
An official website of the United States government
The .gov means it’s official. Federal government websites often end in .gov or .mil. Before sharing sensitive information, make sure you’re on a federal government site.
The site is secure. The https:// ensures that you are connecting to the official website and that any information you provide is encrypted and transmitted securely.
- Publications
- Account settings
- My Bibliography
- Collections
- Citation manager

Save citation to file
Email citation, add to collections.
- Create a new collection
- Add to an existing collection
Add to My Bibliography
Your saved search, create a file for external citation management software, your rss feed.
- Search in PubMed
- Search in NLM Catalog
- Add to Search
Secondary Analysis Research
Affiliation.
- 1 Rush University College of Nursing, Chicago, Illinois.
- PMID: 33343987
- PMCID: PMC7520737
- DOI: 10.6004/jadpro.2019.10.4.7
In secondary data analysis (SDA) studies, investigators use data collected by other researchers to address different questions. Like primary data researchers, SDA investigators must be knowledgeable about their research area to identify datasets that are a good fit for an SDA. Several sources of datasets may be useful for SDA, and examples of some of these will be discussed. Advanced practice providers must be aware of possible advantages, such as economic savings, the ability to examine clinically significant research questions in large datasets that may have been collected over time (longitudinal data), generating new hypotheses or clarifying research questions, and avoiding overburdening sensitive populations or investigating sensitive areas. When reading an SDA report, the reader should be able to determine that the authors identified the limitation or disadvantages of their research. For example, a primary dataset cannot "fit" an SDA researcher's study exactly, SDAs are inherently limited by the inability to definitively examine causality given their retrospective nature, and data may be too old to address current issues.
© 2019 Harborside™.
PubMed Disclaimer
Conflict of interest statement
The author has no conflicts of interest to disclose.
Similar articles
- Translational Metabolomics of Head Injury: Exploring Dysfunctional Cerebral Metabolism with Ex Vivo NMR Spectroscopy-Based Metabolite Quantification. Wolahan SM, Hirt D, Glenn TC. Wolahan SM, et al. In: Kobeissy FH, editor. Brain Neurotrauma: Molecular, Neuropsychological, and Rehabilitation Aspects. Boca Raton (FL): CRC Press/Taylor & Francis; 2015. Chapter 25. In: Kobeissy FH, editor. Brain Neurotrauma: Molecular, Neuropsychological, and Rehabilitation Aspects. Boca Raton (FL): CRC Press/Taylor & Francis; 2015. Chapter 25. PMID: 26269925 Free Books & Documents. Review.
- Maximizing research opportunities: secondary data analysis. Castle JE. Castle JE. J Neurosci Nurs. 2003 Oct;35(5):287-90. doi: 10.1097/01376517-200310000-00008. J Neurosci Nurs. 2003. PMID: 14593941 Review.
- Specific dynamic action: a century of investigation. McCue MD. McCue MD. Comp Biochem Physiol A Mol Integr Physiol. 2006 Aug;144(4):381-94. doi: 10.1016/j.cbpa.2006.03.011. Epub 2006 Mar 30. Comp Biochem Physiol A Mol Integr Physiol. 2006. PMID: 16716621 Review.
- Effect of removable partial dentures on oral health-related quality of life in subjects with shortened dental arches: a 2-center cross-sectional study. Armellini DB, Heydecke G, Witter DJ, Creugers NH. Armellini DB, et al. Int J Prosthodont. 2008 Nov-Dec;21(6):524-30. Int J Prosthodont. 2008. PMID: 19149071
- Measuring hot flashes: summary of a National Institutes of Health workshop. Miller HG, Li RM. Miller HG, et al. Mayo Clin Proc. 2004 Jun;79(6):777-81. doi: 10.4065/79.6.777. Mayo Clin Proc. 2004. PMID: 15182093
- Effect of post-storage filters vs. pre-storage filters for leukoreduction of blood components on clinical outcomes: a systematic review and meta-analysis. Dejigov Monteiro da Silva N, Nukui Y, Takahashi J, de Almeida Lopes Monteiro da Cruz D, de Souza Nogueira L. Dejigov Monteiro da Silva N, et al. Syst Rev. 2024 Jul 25;13(1):196. doi: 10.1186/s13643-024-02615-z. Syst Rev. 2024. PMID: 39054473 Free PMC article.
- Cross-border data sharing through the lens of research ethics committee members in sub-Saharan Africa. Cengiz N, Kabanda SM, Moodley K. Cengiz N, et al. PLoS One. 2024 May 23;19(5):e0303828. doi: 10.1371/journal.pone.0303828. eCollection 2024. PLoS One. 2024. PMID: 38781141 Free PMC article.
- Intimate partner violence and its correlates in middle-aged and older adults during the COVID-19 pandemic: A multi-country secondary analysis. Chang G, Tucker JD, Walker K, Chu C, Miall N, Tan RKJ, Wu D. Chang G, et al. PLOS Glob Public Health. 2024 May 16;4(5):e0002500. doi: 10.1371/journal.pgph.0002500. eCollection 2024. PLOS Glob Public Health. 2024. PMID: 38753815 Free PMC article.
- Adolescent pregnancy persists in Nigeria: Does household heads' age matter? Mbulu CO, Yang L, Wallen GR. Mbulu CO, et al. PLOS Glob Public Health. 2024 May 15;4(5):e0003212. doi: 10.1371/journal.pgph.0003212. eCollection 2024. PLOS Glob Public Health. 2024. PMID: 38748678 Free PMC article.
- Interpapillary muscle distance independently predicts recurrent mitral regurgitation. Gambardella I, Spadaccio C, Singh SSA, Shingu Y, Kunihara T, Wakasa S, Nappi F. Gambardella I, et al. J Cardiothorac Surg. 2024 Mar 20;19(1):147. doi: 10.1186/s13019-024-02631-z. J Cardiothorac Surg. 2024. PMID: 38509555 Free PMC article. Clinical Trial.
- Ascierto P. A., Del Vecchio M., Robert C., Mackiewicz A., Chiarion-Sileni V., Arance A.,…Maio M. (2017). Ipilimumab 10 mg/kg versus ipilimumab 3 mg/kg in patients with unresectable or metastatic melanoma: A randomised, double-blind, multicentre, phase 3 trial. Lancet Oncology, 18(5), 611–622. 10.1016/S1470-2045(17)30231-0 - DOI - PubMed
- Burkhalter J. E., Margolies L., Sigurdsson H. O., Walland J., Radix A., Rice D.,…Maingi S. (2016). The National LGBT Cancer Action Plan: A white paper of the 2014 National Summit on Cancer in the LGBT Communities. LGBT Health, 3(1), 19–31. 10.1089/lgbt.2015.0118 - DOI
- Castle J. E. (2003). Maximizing research opportunities: Secondary data analysis. Journal of Neuroscience Nursing, 35(5), 287–290. Retrieved from https://www.ncbi.nlm.nih.gov/pubmed/14593941 - PubMed
- Cheng H. G., & Phillips M. R. (2014). Secondary analysis of existing data: Opportunities and implementation. Shanghai Archives of Psychiatry, 26(6), 371–375. https://dx.doi.org/10.11919%2Fj.issn.1002-0829.214171 - PMC - PubMed
- Heaton J. (2008). Secondary analysis of qualitative data: An overview. Historical Social Research, 33(3), 33–45.
LinkOut - more resources
Full text sources.
- Europe PubMed Central
- PubMed Central
- Citation Manager
NCBI Literature Resources
MeSH PMC Bookshelf Disclaimer
The PubMed wordmark and PubMed logo are registered trademarks of the U.S. Department of Health and Human Services (HHS). Unauthorized use of these marks is strictly prohibited.
Have a language expert improve your writing
Run a free plagiarism check in 10 minutes, generate accurate citations for free.
- Knowledge Base
Methodology
- Data Collection | Definition, Methods & Examples
Data Collection | Definition, Methods & Examples
Published on June 5, 2020 by Pritha Bhandari . Revised on June 21, 2023.
Data collection is a systematic process of gathering observations or measurements. Whether you are performing research for business, governmental or academic purposes, data collection allows you to gain first-hand knowledge and original insights into your research problem .
While methods and aims may differ between fields, the overall process of data collection remains largely the same. Before you begin collecting data, you need to consider:
- The aim of the research
- The type of data that you will collect
- The methods and procedures you will use to collect, store, and process the data
To collect high-quality data that is relevant to your purposes, follow these four steps.
Table of contents
Step 1: define the aim of your research, step 2: choose your data collection method, step 3: plan your data collection procedures, step 4: collect the data, other interesting articles, frequently asked questions about data collection.
Before you start the process of data collection, you need to identify exactly what you want to achieve. You can start by writing a problem statement : what is the practical or scientific issue that you want to address and why does it matter?
Next, formulate one or more research questions that precisely define what you want to find out. Depending on your research questions, you might need to collect quantitative or qualitative data :
- Quantitative data is expressed in numbers and graphs and is analyzed through statistical methods .
- Qualitative data is expressed in words and analyzed through interpretations and categorizations.
If your aim is to test a hypothesis , measure something precisely, or gain large-scale statistical insights, collect quantitative data. If your aim is to explore ideas, understand experiences, or gain detailed insights into a specific context, collect qualitative data. If you have several aims, you can use a mixed methods approach that collects both types of data.
- Your first aim is to assess whether there are significant differences in perceptions of managers across different departments and office locations.
- Your second aim is to gather meaningful feedback from employees to explore new ideas for how managers can improve.
Here's why students love Scribbr's proofreading services
Discover proofreading & editing
Based on the data you want to collect, decide which method is best suited for your research.
- Experimental research is primarily a quantitative method.
- Interviews , focus groups , and ethnographies are qualitative methods.
- Surveys , observations, archival research and secondary data collection can be quantitative or qualitative methods.
Carefully consider what method you will use to gather data that helps you directly answer your research questions.
Method | When to use | How to collect data |
---|---|---|
Experiment | To test a causal relationship. | Manipulate variables and measure their effects on others. |
Survey | To understand the general characteristics or opinions of a group of people. | Distribute a list of questions to a sample online, in person or over-the-phone. |
Interview/focus group | To gain an in-depth understanding of perceptions or opinions on a topic. | Verbally ask participants open-ended questions in individual interviews or focus group discussions. |
Observation | To understand something in its natural setting. | Measure or survey a sample without trying to affect them. |
Ethnography | To study the culture of a community or organization first-hand. | Join and participate in a community and record your observations and reflections. |
Archival research | To understand current or historical events, conditions or practices. | Access manuscripts, documents or records from libraries, depositories or the internet. |
Secondary data collection | To analyze data from populations that you can’t access first-hand. | Find existing datasets that have already been collected, from sources such as government agencies or research organizations. |
When you know which method(s) you are using, you need to plan exactly how you will implement them. What procedures will you follow to make accurate observations or measurements of the variables you are interested in?
For instance, if you’re conducting surveys or interviews, decide what form the questions will take; if you’re conducting an experiment, make decisions about your experimental design (e.g., determine inclusion and exclusion criteria ).
Operationalization
Sometimes your variables can be measured directly: for example, you can collect data on the average age of employees simply by asking for dates of birth. However, often you’ll be interested in collecting data on more abstract concepts or variables that can’t be directly observed.
Operationalization means turning abstract conceptual ideas into measurable observations. When planning how you will collect data, you need to translate the conceptual definition of what you want to study into the operational definition of what you will actually measure.
- You ask managers to rate their own leadership skills on 5-point scales assessing the ability to delegate, decisiveness and dependability.
- You ask their direct employees to provide anonymous feedback on the managers regarding the same topics.
You may need to develop a sampling plan to obtain data systematically. This involves defining a population , the group you want to draw conclusions about, and a sample, the group you will actually collect data from.
Your sampling method will determine how you recruit participants or obtain measurements for your study. To decide on a sampling method you will need to consider factors like the required sample size, accessibility of the sample, and timeframe of the data collection.
Standardizing procedures
If multiple researchers are involved, write a detailed manual to standardize data collection procedures in your study.
This means laying out specific step-by-step instructions so that everyone in your research team collects data in a consistent way – for example, by conducting experiments under the same conditions and using objective criteria to record and categorize observations. This helps you avoid common research biases like omitted variable bias or information bias .
This helps ensure the reliability of your data, and you can also use it to replicate the study in the future.
Creating a data management plan
Before beginning data collection, you should also decide how you will organize and store your data.
- If you are collecting data from people, you will likely need to anonymize and safeguard the data to prevent leaks of sensitive information (e.g. names or identity numbers).
- If you are collecting data via interviews or pencil-and-paper formats, you will need to perform transcriptions or data entry in systematic ways to minimize distortion.
- You can prevent loss of data by having an organization system that is routinely backed up.
Finally, you can implement your chosen methods to measure or observe the variables you are interested in.
The closed-ended questions ask participants to rate their manager’s leadership skills on scales from 1–5. The data produced is numerical and can be statistically analyzed for averages and patterns.
To ensure that high quality data is recorded in a systematic way, here are some best practices:
- Record all relevant information as and when you obtain data. For example, note down whether or how lab equipment is recalibrated during an experimental study.
- Double-check manual data entry for errors.
- If you collect quantitative data, you can assess the reliability and validity to get an indication of your data quality.
If you want to know more about statistics , methodology , or research bias , make sure to check out some of our other articles with explanations and examples.
- Student’s t -distribution
- Normal distribution
- Null and Alternative Hypotheses
- Chi square tests
- Confidence interval
- Cluster sampling
- Stratified sampling
- Data cleansing
- Reproducibility vs Replicability
- Peer review
- Likert scale
Research bias
- Implicit bias
- Framing effect
- Cognitive bias
- Placebo effect
- Hawthorne effect
- Hindsight bias
- Affect heuristic
Data collection is the systematic process by which observations or measurements are gathered in research. It is used in many different contexts by academics, governments, businesses, and other organizations.
When conducting research, collecting original data has significant advantages:
- You can tailor data collection to your specific research aims (e.g. understanding the needs of your consumers or user testing your website)
- You can control and standardize the process for high reliability and validity (e.g. choosing appropriate measurements and sampling methods )
However, there are also some drawbacks: data collection can be time-consuming, labor-intensive and expensive. In some cases, it’s more efficient to use secondary data that has already been collected by someone else, but the data might be less reliable.
Quantitative research deals with numbers and statistics, while qualitative research deals with words and meanings.
Quantitative methods allow you to systematically measure variables and test hypotheses . Qualitative methods allow you to explore concepts and experiences in more detail.
Reliability and validity are both about how well a method measures something:
- Reliability refers to the consistency of a measure (whether the results can be reproduced under the same conditions).
- Validity refers to the accuracy of a measure (whether the results really do represent what they are supposed to measure).
If you are doing experimental research, you also have to consider the internal and external validity of your experiment.
Operationalization means turning abstract conceptual ideas into measurable observations.
For example, the concept of social anxiety isn’t directly observable, but it can be operationally defined in terms of self-rating scores, behavioral avoidance of crowded places, or physical anxiety symptoms in social situations.
Before collecting data , it’s important to consider how you will operationalize the variables that you want to measure.
In mixed methods research , you use both qualitative and quantitative data collection and analysis methods to answer your research question .
Cite this Scribbr article
If you want to cite this source, you can copy and paste the citation or click the “Cite this Scribbr article” button to automatically add the citation to our free Citation Generator.
Bhandari, P. (2023, June 21). Data Collection | Definition, Methods & Examples. Scribbr. Retrieved September 9, 2024, from https://www.scribbr.com/methodology/data-collection/
Is this article helpful?
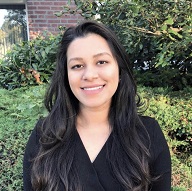
Pritha Bhandari
Other students also liked, qualitative vs. quantitative research | differences, examples & methods, sampling methods | types, techniques & examples, "i thought ai proofreading was useless but..".
I've been using Scribbr for years now and I know it's a service that won't disappoint. It does a good job spotting mistakes”
Instant insights, infinite possibilities
Data collection in research: Your complete guide
Last updated
31 January 2023
Reviewed by
Cathy Heath
Short on time? Get an AI generated summary of this article instead
In the late 16th century, Francis Bacon coined the phrase "knowledge is power," which implies that knowledge is a powerful force, like physical strength. In the 21st century, knowledge in the form of data is unquestionably powerful.
But data isn't something you just have - you need to collect it. This means utilizing a data collection process and turning the collected data into knowledge that you can leverage into a successful strategy for your business or organization.
Believe it or not, there's more to data collection than just conducting a Google search. In this complete guide, we shine a spotlight on data collection, outlining what it is, types of data collection methods, common challenges in data collection, data collection techniques, and the steps involved in data collection.
Analyze all your data in one place
Uncover hidden nuggets in all types of qualitative data when you analyze it in Dovetail
- What is data collection?
There are two specific data collection techniques: primary and secondary data collection. Primary data collection is the process of gathering data directly from sources. It's often considered the most reliable data collection method, as researchers can collect information directly from respondents.
Secondary data collection is data that has already been collected by someone else and is readily available. This data is usually less expensive and quicker to obtain than primary data.
- What are the different methods of data collection?
There are several data collection methods, which can be either manual or automated. Manual data collection involves collecting data manually, typically with pen and paper, while computerized data collection involves using software to collect data from online sources, such as social media, website data, transaction data, etc.
Here are the five most popular methods of data collection:
Surveys are a very popular method of data collection that organizations can use to gather information from many people. Researchers can conduct multi-mode surveys that reach respondents in different ways, including in person, by mail, over the phone, or online.
As a method of data collection, surveys have several advantages. For instance, they are relatively quick and easy to administer, you can be flexible in what you ask, and they can be tailored to collect data on various topics or from certain demographics.
However, surveys also have several disadvantages. For instance, they can be expensive to administer, and the results may not represent the population as a whole. Additionally, survey data can be challenging to interpret. It may also be subject to bias if the questions are not well-designed or if the sample of people surveyed is not representative of the population of interest.
Interviews are a common method of collecting data in social science research. You can conduct interviews in person, over the phone, or even via email or online chat.
Interviews are a great way to collect qualitative and quantitative data . Qualitative interviews are likely your best option if you need to collect detailed information about your subjects' experiences or opinions. If you need to collect more generalized data about your subjects' demographics or attitudes, then quantitative interviews may be a better option.
Interviews are relatively quick and very flexible, allowing you to ask follow-up questions and explore topics in more depth. The downside is that interviews can be time-consuming and expensive due to the amount of information to be analyzed. They are also prone to bias, as both the interviewer and the respondent may have certain expectations or preconceptions that may influence the data.
Direct observation
Observation is a direct way of collecting data. It can be structured (with a specific protocol to follow) or unstructured (simply observing without a particular plan).
Organizations and businesses use observation as a data collection method to gather information about their target market, customers, or competition. Businesses can learn about consumer behavior, preferences, and trends by observing people using their products or service.
There are two types of observation: participatory and non-participatory. In participatory observation, the researcher is actively involved in the observed activities. This type of observation is used in ethnographic research , where the researcher wants to understand a group's culture and social norms. Non-participatory observation is when researchers observe from a distance and do not interact with the people or environment they are studying.
There are several advantages to using observation as a data collection method. It can provide insights that may not be apparent through other methods, such as surveys or interviews. Researchers can also observe behavior in a natural setting, which can provide a more accurate picture of what people do and how and why they behave in a certain context.
There are some disadvantages to using observation as a method of data collection. It can be time-consuming, intrusive, and expensive to observe people for extended periods. Observations can also be tainted if the researcher is not careful to avoid personal biases or preconceptions.
Automated data collection
Business applications and websites are increasingly collecting data electronically to improve the user experience or for marketing purposes.
There are a few different ways that organizations can collect data automatically. One way is through cookies, which are small pieces of data stored on a user's computer. They track a user's browsing history and activity on a site, measuring levels of engagement with a business’s products or services, for example.
Another way organizations can collect data automatically is through web beacons. Web beacons are small images embedded on a web page to track a user's activity.
Finally, organizations can also collect data through mobile apps, which can track user location, device information, and app usage. This data can be used to improve the user experience and for marketing purposes.
Automated data collection is a valuable tool for businesses, helping improve the user experience or target marketing efforts. Businesses should aim to be transparent about how they collect and use this data.
Sourcing data through information service providers
Organizations need to be able to collect data from a variety of sources, including social media, weblogs, and sensors. The process to do this and then use the data for action needs to be efficient, targeted, and meaningful.
In the era of big data, organizations are increasingly turning to information service providers (ISPs) and other external data sources to help them collect data to make crucial decisions.
Information service providers help organizations collect data by offering personalized services that suit the specific needs of the organizations. These services can include data collection, analysis, management, and reporting. By partnering with an ISP, organizations can gain access to the newest technology and tools to help them to gather and manage data more effectively.
There are also several tools and techniques that organizations can use to collect data from external sources, such as web scraping, which collects data from websites, and data mining, which involves using algorithms to extract data from large data sets.
Organizations can also use APIs (application programming interface) to collect data from external sources. APIs allow organizations to access data stored in another system and share and integrate it into their own systems.
Finally, organizations can also use manual methods to collect data from external sources. This can involve contacting companies or individuals directly to request data, by using the right tools and methods to get the insights they need.
- What are common challenges in data collection?
There are many challenges that researchers face when collecting data. Here are five common examples:
Big data environments
Data collection can be a challenge in big data environments for several reasons. It can be located in different places, such as archives, libraries, or online. The sheer volume of data can also make it difficult to identify the most relevant data sets.
Second, the complexity of data sets can make it challenging to extract the desired information. Third, the distributed nature of big data environments can make it difficult to collect data promptly and efficiently.
Therefore it is important to have a well-designed data collection strategy to consider the specific needs of the organization and what data sets are the most relevant. Alongside this, consideration should be made regarding the tools and resources available to support data collection and protect it from unintended use.
Data bias is a common challenge in data collection. It occurs when data is collected from a sample that is not representative of the population of interest.
There are different types of data bias, but some common ones include selection bias, self-selection bias, and response bias. Selection bias can occur when the collected data does not represent the population being studied. For example, if a study only includes data from people who volunteer to participate, that data may not represent the general population.
Self-selection bias can also occur when people self-select into a study, such as by taking part only if they think they will benefit from it. Response bias happens when people respond in a way that is not honest or accurate, such as by only answering questions that make them look good.
These types of data bias present a challenge because they can lead to inaccurate results and conclusions about behaviors, perceptions, and trends. Data bias can be avoided by identifying potential sources or themes of bias and setting guidelines for eliminating them.
Lack of quality assurance processes
One of the biggest challenges in data collection is the lack of quality assurance processes. This can lead to several problems, including incorrect data, missing data, and inconsistencies between data sets.
Quality assurance is important because there are many data sources, and each source may have different levels of quality or corruption. There are also different ways of collecting data, and data quality may vary depending on the method used.
There are several ways to improve quality assurance in data collection. These include developing clear and consistent goals and guidelines for data collection, implementing quality control measures, using standardized procedures, and employing data validation techniques. By taking these steps, you can ensure that your data is of adequate quality to inform decision-making.
Limited access to data
Another challenge in data collection is limited access to data. This can be due to several reasons, including privacy concerns, the sensitive nature of the data, security concerns, or simply the fact that data is not readily available.
Legal and compliance regulations
Most countries have regulations governing how data can be collected, used, and stored. In some cases, data collected in one country may not be used in another. This means gaining a global perspective can be a challenge.
For example, if a company is required to comply with the EU General Data Protection Regulation (GDPR), it may not be able to collect data from individuals in the EU without their explicit consent. This can make it difficult to collect data from a target audience.
Legal and compliance regulations can be complex, and it's important to ensure that all data collected is done so in a way that complies with the relevant regulations.
- What are the key steps in the data collection process?
There are five steps involved in the data collection process. They are:
1. Decide what data you want to gather
Have a clear understanding of the questions you are asking, and then consider where the answers might lie and how you might obtain them. This saves time and resources by avoiding the collection of irrelevant data, and helps maintain the quality of your datasets.
2. Establish a deadline for data collection
Establishing a deadline for data collection helps you avoid collecting too much data, which can be costly and time-consuming to analyze. It also allows you to plan for data analysis and prompt interpretation. Finally, it helps you meet your research goals and objectives and allows you to move forward.
3. Select a data collection approach
The data collection approach you choose will depend on different factors, including the type of data you need, available resources, and the project timeline. For instance, if you need qualitative data, you might choose a focus group or interview methodology. If you need quantitative data , then a survey or observational study may be the most appropriate form of collection.
4. Gather information
When collecting data for your business, identify your business goals first. Once you know what you want to achieve, you can start collecting data to reach those goals. The most important thing is to ensure that the data you collect is reliable and valid. Otherwise, any decisions you make using the data could result in a negative outcome for your business.
5. Examine the information and apply your findings
As a researcher, it's important to examine the data you're collecting and analyzing before you apply your findings. This is because data can be misleading, leading to inaccurate conclusions. Ask yourself whether it is what you are expecting? Is it similar to other datasets you have looked at?
There are many scientific ways to examine data, but some common methods include:
looking at the distribution of data points
examining the relationships between variables
looking for outliers
By taking the time to examine your data and noticing any patterns, strange or otherwise, you can avoid making mistakes that could invalidate your research.
- How qualitative analysis software streamlines the data collection process
Knowledge derived from data does indeed carry power. However, if you don't convert the knowledge into action, it will remain a resource of unexploited energy and wasted potential.
Luckily, data collection tools enable organizations to streamline their data collection and analysis processes and leverage the derived knowledge to grow their businesses. For instance, qualitative analysis software can be highly advantageous in data collection by streamlining the process, making it more efficient and less time-consuming.
Secondly, qualitative analysis software provides a structure for data collection and analysis, ensuring that data is of high quality. It can also help to uncover patterns and relationships that would otherwise be difficult to discern. Moreover, you can use it to replace more expensive data collection methods, such as focus groups or surveys.
Overall, qualitative analysis software can be valuable for any researcher looking to collect and analyze data. By increasing efficiency, improving data quality, and providing greater insights, qualitative software can help to make the research process much more efficient and effective.
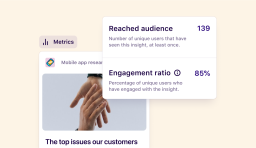
Learn more about qualitative research data analysis software
Should you be using a customer insights hub.
Do you want to discover previous research faster?
Do you share your research findings with others?
Do you analyze research data?
Start for free today, add your research, and get to key insights faster
Editor’s picks
Last updated: 18 April 2023
Last updated: 27 February 2023
Last updated: 22 August 2024
Last updated: 5 February 2023
Last updated: 16 August 2024
Last updated: 9 March 2023
Last updated: 30 April 2024
Last updated: 12 December 2023
Last updated: 11 March 2024
Last updated: 4 July 2024
Last updated: 6 March 2024
Last updated: 5 March 2024
Last updated: 13 May 2024
Latest articles
Related topics, .css-je19u9{-webkit-align-items:flex-end;-webkit-box-align:flex-end;-ms-flex-align:flex-end;align-items:flex-end;display:-webkit-box;display:-webkit-flex;display:-ms-flexbox;display:flex;-webkit-flex-direction:row;-ms-flex-direction:row;flex-direction:row;-webkit-box-flex-wrap:wrap;-webkit-flex-wrap:wrap;-ms-flex-wrap:wrap;flex-wrap:wrap;-webkit-box-pack:center;-ms-flex-pack:center;-webkit-justify-content:center;justify-content:center;row-gap:0;text-align:center;max-width:671px;}@media (max-width: 1079px){.css-je19u9{max-width:400px;}.css-je19u9>span{white-space:pre;}}@media (max-width: 799px){.css-je19u9{max-width:400px;}.css-je19u9>span{white-space:pre;}} decide what to .css-1kiodld{max-height:56px;display:-webkit-box;display:-webkit-flex;display:-ms-flexbox;display:flex;-webkit-align-items:center;-webkit-box-align:center;-ms-flex-align:center;align-items:center;}@media (max-width: 1079px){.css-1kiodld{display:none;}} build next, decide what to build next, log in or sign up.
Get started for free

- Research Data Lifecycle Guide
Data Collection
Data collection is the process of gathering and measuring information used for research. Collecting data is one of the most important steps in the research process, and is part of all disciplines including physical and social sciences, humanities, business, etc. Data comes in many forms with different ways to store and record data, either written in a lab notebook and or recorded digitally on a computer system.
While methods may differ across disciplines, good data management processes begin with accurately and clearly describing the information recorded, the process used to collect the data, practices that ensure the quality of the data, and sharing data to enable reproducibility. This section breaks down different topics that need to be addressed while collecting and managing data for research.
Learn more about what’s required for data collection as a researcher at Case Western Reserve University.
Ensuring Accurate and Appropriate Data Collection
Accurate data collection is vital to ensure the integrity of research . It is important when planning and executing a research project to consider methods collection and the storage of data to ensure that results can be used for publications and reporting. The consequences from improper data collection include:
- inability to answer research questions accurately
- inability to repeat and validate the study
- distorted findings resulting in wasted resources
- misleading other researchers to pursue fruitless avenues of investigation
- compromising decisions for public policy
- causing harm to human participants and animal subjects
While the degree of impact from inaccurate data may vary by discipline, there is a potential to cause disproportionate harm when data is misrepresented and misused. This includes fraud or scientific misconduct.
Any data collected in the course of your research should follow RDM best practices to ensure accurate and appropriate data collection. This includes as appropriate, developing data collection protocols and processes to ensure inconsistencies and other errors are caught and corrected in a timely manner.
Examples of Research Data
Research data is any information that has been collected, observed, generated or created in association with research processes and findings.
Much research data is digital in format, but research data can also be extended to include non-digital formats such as laboratory notebook, diaries, or written responses to surveys. Examples may include (but are not limited to):
- Excel spreadsheets that contains instrument data
- Documents (text, Word), containing study results
- Laboratory notebooks, field notebooks, diaries
- Questionnaires, transcripts, codebooks
- Audiotapes, videotapes
- Photographs, films
- Protein or genetic sequences
- Test responses
- Slides, artifacts, specimens, samples
- Collection of digital objects acquired and generated during the process of research
- Database contents (video, audio, text, images)
- Models, algorithms, scripts
- Contents of an application (input, output, logfiles for analysis software, simulation software, schemas)
- Source code used in application development
To ensure reproducibility of experiments and results, be sure to include and document information such as:
- Methodologies and workflows
- Standard operating procedures and protocols
Data Use Agreements
When working with data it is important to understand any restrictions that need to be addressed due to the sensitivity of the data. This includes how you download and share with other collaborators, and how it needs to be properly secured.
Datasets can include potentially sensitive data that needs to be protected, and not openly shared. In this case, the dataset cannot be shared and or downloaded without permission from CWRU Research Administration and may require an agreement between collaborators and their institutions. All parties will need to abide by the agreement terms including the destruction of data once the collaboration is complete.
Storage Options
UTech provides cloud and on-premise storage to support the university research mission. This includes Google Drive , Box , Microsoft 365 , and various on-premise solutions for high speed access and mass storage. A listing of supported options can be found on UTech’s website .
In addition to UTech-supported storage solutions, CWRU also maintains an institutional subscription to OSF (Open Science Framework) . OSF is a cloud-based data storage, sharing, and project collaboration platform that connects to many other cloud services like Drive, Box, and Github to amplify your research and data visibility and discoverability. OSF storage is functionally unlimited.
When selecting a storage platform it is important to understand how you plan to analyze and store your data. Cloud storage provides the ability to store and share data effortlessly and provides capabilities such as revisioning and other means to protect your data. On-premise storage is useful when you have large storage demands and require a high speed connection to instruments that generate data and systems that process data. Both types of storage have their advantages and disadvantages that you should consider when planning your research project.
Data Security
Data security is a set of processes and ongoing practices designed to protect information and the systems used to store and process data. This includes computer systems, files, databases, applications, user accounts, networks, and services on institutional premises, in the cloud, and remotely at the location of individual researchers.
Effective data security takes into account the confidentiality, integrity, and availability of the information and its use. This is especially important when data contains personally identifiable information, intellectual property, trade secrets, and or technical data supporting technology transfer agreements (before public disclosure decisions have been made).
Data Categorization
CWRU uses a 3-tier system to categorize research data based on information types and sensitivity . Determination is based upon risk to the University in the areas of confidentiality, integrity, and availability of data in support of the University's research mission. In this context, confidentiality measures to what extent information can be disclosed to others, integrity is the assurance that the information is trustworthy and accurate, and availability is a guarantee of reliable access to the information by authorized users.
Information (or data) owners are responsible for determining the impact levels of their information, i.e. what happens if the data is improperly accessed or lost accidentally, implementing the necessary security controls, and managing the risk of negative events including data loss and unauthorized access.
Classification | Examples |
---|---|
| |
| |
|
Loss, corruption, or inappropriate access to information can interfere with CWRU's mission, interrupt business and damage reputations or finances.
Securing Data
The classification of data requires certain safeguards or countermeasures, known as controls, to be applied to systems that store data. This can include restricting access to the data, detecting unauthorized access, preventative measures to avoid loss of data, encrypting the transfer and storage of data, keeping the system and data in a secure location, and receiving training on best practices for handling data. Controls are classified according to their characteristics, for example:
- Physical controls e.g. doors, locks, climate control, and fire extinguishers;
- Procedural or administrative controls e.g. policies, incident response processes, management oversight, security awareness and training;
- Technical or logical controls e.g. user authentication (login) and logical access controls, antivirus software, firewalls;
- Legal and regulatory or compliance controls e.g. privacy laws, policies and clauses.
Principal Investigator (PI) Responsibilities
The CWRU Faculty Handbook provides guidelines for PIs regarding the custody of research data. This includes, where applicable, appropriate measures to protect confidential information. It is everyone’s responsibility to ensure that our research data is kept securely and available for reproducibility and future research opportunities.
University Technology provides many services and resources related to data security including assistance with planning and securing data. This includes processing and storing restricted information used in research.
Data Collected as Part of Human Subject Research
To ensure the privacy and safety of the individual participating in a human subject research study, additional rules and processes are in place that describe how one can use and disclose data collected, The Office of Research Administration provides information relevant to conducting this type of research. This includes:
- Guidance on data use agreements and processes for agreements that involve human-related data or human-derived samples coming in or going out of CWRU.
- Compliance with human subject research rules and regulations.
According to 45 CFR 46 , a human subject is "a living individual about whom an investigator (whether professional or student) conducting research:
- Obtains information or biospecimens through intervention or interaction with the individual, and uses, studies, or analyzes the information or biospecimens; or
- Obtains, uses, studies, analyzes, or generates identifiable private information or identifiable biospecimens."
The CWRU Institutional Review Board reviews social science/behavioral studies, and low-risk biomedical research not conducted in a hospital setting for all faculty, staff, and students of the University. This includes data collected and used for human subjects research.
Research conducted in a hospital setting including University Hospitals requires IRB protocol approval.
Questions regarding the management of human subject research data should be addressed to the CWRU Institutional Review Board .
Getting Help With Data Collection
If you are looking for datasets and other resources for your research you can contact your subject area librarian for assistance.
- Kelvin Smith Library
If you need assistance with administrative items such as data use agreements or finding the appropriate storage solution please contact the following offices.
- Research Administration
- UTech Research Computing
- Information Security Office
Guidance and Resources
- Information Security Policy
- Research Data Protection
- CWRU Faculty Handbook
- CWRU IRB Guidance
Have a language expert improve your writing
Run a free plagiarism check in 10 minutes, automatically generate references for free.
- Knowledge Base
- Methodology
- Data Collection Methods | Step-by-Step Guide & Examples
Data Collection Methods | Step-by-Step Guide & Examples
Published on 4 May 2022 by Pritha Bhandari .
Data collection is a systematic process of gathering observations or measurements. Whether you are performing research for business, governmental, or academic purposes, data collection allows you to gain first-hand knowledge and original insights into your research problem .
While methods and aims may differ between fields, the overall process of data collection remains largely the same. Before you begin collecting data, you need to consider:
- The aim of the research
- The type of data that you will collect
- The methods and procedures you will use to collect, store, and process the data
To collect high-quality data that is relevant to your purposes, follow these four steps.
Table of contents
Step 1: define the aim of your research, step 2: choose your data collection method, step 3: plan your data collection procedures, step 4: collect the data, frequently asked questions about data collection.
Before you start the process of data collection, you need to identify exactly what you want to achieve. You can start by writing a problem statement : what is the practical or scientific issue that you want to address, and why does it matter?
Next, formulate one or more research questions that precisely define what you want to find out. Depending on your research questions, you might need to collect quantitative or qualitative data :
- Quantitative data is expressed in numbers and graphs and is analysed through statistical methods .
- Qualitative data is expressed in words and analysed through interpretations and categorisations.
If your aim is to test a hypothesis , measure something precisely, or gain large-scale statistical insights, collect quantitative data. If your aim is to explore ideas, understand experiences, or gain detailed insights into a specific context, collect qualitative data.
If you have several aims, you can use a mixed methods approach that collects both types of data.
- Your first aim is to assess whether there are significant differences in perceptions of managers across different departments and office locations.
- Your second aim is to gather meaningful feedback from employees to explore new ideas for how managers can improve.
Prevent plagiarism, run a free check.
Based on the data you want to collect, decide which method is best suited for your research.
- Experimental research is primarily a quantitative method.
- Interviews , focus groups , and ethnographies are qualitative methods.
- Surveys , observations, archival research, and secondary data collection can be quantitative or qualitative methods.
Carefully consider what method you will use to gather data that helps you directly answer your research questions.
Method | When to use | How to collect data |
---|---|---|
Experiment | To test a causal relationship. | Manipulate variables and measure their effects on others. |
Survey | To understand the general characteristics or opinions of a group of people. | Distribute a list of questions to a sample online, in person, or over the phone. |
Interview/focus group | To gain an in-depth understanding of perceptions or opinions on a topic. | Verbally ask participants open-ended questions in individual interviews or focus group discussions. |
Observation | To understand something in its natural setting. | Measure or survey a sample without trying to affect them. |
Ethnography | To study the culture of a community or organisation first-hand. | Join and participate in a community and record your observations and reflections. |
Archival research | To understand current or historical events, conditions, or practices. | Access manuscripts, documents, or records from libraries, depositories, or the internet. |
Secondary data collection | To analyse data from populations that you can’t access first-hand. | Find existing datasets that have already been collected, from sources such as government agencies or research organisations. |
When you know which method(s) you are using, you need to plan exactly how you will implement them. What procedures will you follow to make accurate observations or measurements of the variables you are interested in?
For instance, if you’re conducting surveys or interviews, decide what form the questions will take; if you’re conducting an experiment, make decisions about your experimental design .
Operationalisation
Sometimes your variables can be measured directly: for example, you can collect data on the average age of employees simply by asking for dates of birth. However, often you’ll be interested in collecting data on more abstract concepts or variables that can’t be directly observed.
Operationalisation means turning abstract conceptual ideas into measurable observations. When planning how you will collect data, you need to translate the conceptual definition of what you want to study into the operational definition of what you will actually measure.
- You ask managers to rate their own leadership skills on 5-point scales assessing the ability to delegate, decisiveness, and dependability.
- You ask their direct employees to provide anonymous feedback on the managers regarding the same topics.
You may need to develop a sampling plan to obtain data systematically. This involves defining a population , the group you want to draw conclusions about, and a sample, the group you will actually collect data from.
Your sampling method will determine how you recruit participants or obtain measurements for your study. To decide on a sampling method you will need to consider factors like the required sample size, accessibility of the sample, and time frame of the data collection.
Standardising procedures
If multiple researchers are involved, write a detailed manual to standardise data collection procedures in your study.
This means laying out specific step-by-step instructions so that everyone in your research team collects data in a consistent way – for example, by conducting experiments under the same conditions and using objective criteria to record and categorise observations.
This helps ensure the reliability of your data, and you can also use it to replicate the study in the future.
Creating a data management plan
Before beginning data collection, you should also decide how you will organise and store your data.
- If you are collecting data from people, you will likely need to anonymise and safeguard the data to prevent leaks of sensitive information (e.g. names or identity numbers).
- If you are collecting data via interviews or pencil-and-paper formats, you will need to perform transcriptions or data entry in systematic ways to minimise distortion.
- You can prevent loss of data by having an organisation system that is routinely backed up.
Finally, you can implement your chosen methods to measure or observe the variables you are interested in.
The closed-ended questions ask participants to rate their manager’s leadership skills on scales from 1 to 5. The data produced is numerical and can be statistically analysed for averages and patterns.
To ensure that high-quality data is recorded in a systematic way, here are some best practices:
- Record all relevant information as and when you obtain data. For example, note down whether or how lab equipment is recalibrated during an experimental study.
- Double-check manual data entry for errors.
- If you collect quantitative data, you can assess the reliability and validity to get an indication of your data quality.
Data collection is the systematic process by which observations or measurements are gathered in research. It is used in many different contexts by academics, governments, businesses, and other organisations.
When conducting research, collecting original data has significant advantages:
- You can tailor data collection to your specific research aims (e.g., understanding the needs of your consumers or user testing your website).
- You can control and standardise the process for high reliability and validity (e.g., choosing appropriate measurements and sampling methods ).
However, there are also some drawbacks: data collection can be time-consuming, labour-intensive, and expensive. In some cases, it’s more efficient to use secondary data that has already been collected by someone else, but the data might be less reliable.
Quantitative research deals with numbers and statistics, while qualitative research deals with words and meanings.
Quantitative methods allow you to test a hypothesis by systematically collecting and analysing data, while qualitative methods allow you to explore ideas and experiences in depth.
Reliability and validity are both about how well a method measures something:
- Reliability refers to the consistency of a measure (whether the results can be reproduced under the same conditions).
- Validity refers to the accuracy of a measure (whether the results really do represent what they are supposed to measure).
If you are doing experimental research , you also have to consider the internal and external validity of your experiment.
In mixed methods research , you use both qualitative and quantitative data collection and analysis methods to answer your research question .
Operationalisation means turning abstract conceptual ideas into measurable observations.
For example, the concept of social anxiety isn’t directly observable, but it can be operationally defined in terms of self-rating scores, behavioural avoidance of crowded places, or physical anxiety symptoms in social situations.
Before collecting data , it’s important to consider how you will operationalise the variables that you want to measure.
Cite this Scribbr article
If you want to cite this source, you can copy and paste the citation or click the ‘Cite this Scribbr article’ button to automatically add the citation to our free Reference Generator.
Bhandari, P. (2022, May 04). Data Collection Methods | Step-by-Step Guide & Examples. Scribbr. Retrieved 9 September 2024, from https://www.scribbr.co.uk/research-methods/data-collection-guide/
Is this article helpful?
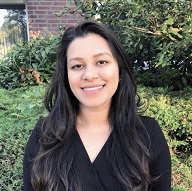
Pritha Bhandari
Other students also liked, qualitative vs quantitative research | examples & methods, triangulation in research | guide, types, examples, what is a conceptual framework | tips & examples.
- Privacy Policy

Home » Data Collection – Methods Types and Examples
Data Collection – Methods Types and Examples
Table of Contents

Data Collection
Definition:
Data collection is the process of gathering and collecting information from various sources to analyze and make informed decisions based on the data collected. This can involve various methods, such as surveys, interviews, experiments, and observation.
In order for data collection to be effective, it is important to have a clear understanding of what data is needed and what the purpose of the data collection is. This can involve identifying the population or sample being studied, determining the variables to be measured, and selecting appropriate methods for collecting and recording data.
Types of Data Collection
Types of Data Collection are as follows:
Primary Data Collection
Primary data collection is the process of gathering original and firsthand information directly from the source or target population. This type of data collection involves collecting data that has not been previously gathered, recorded, or published. Primary data can be collected through various methods such as surveys, interviews, observations, experiments, and focus groups. The data collected is usually specific to the research question or objective and can provide valuable insights that cannot be obtained from secondary data sources. Primary data collection is often used in market research, social research, and scientific research.
Secondary Data Collection
Secondary data collection is the process of gathering information from existing sources that have already been collected and analyzed by someone else, rather than conducting new research to collect primary data. Secondary data can be collected from various sources, such as published reports, books, journals, newspapers, websites, government publications, and other documents.
Qualitative Data Collection
Qualitative data collection is used to gather non-numerical data such as opinions, experiences, perceptions, and feelings, through techniques such as interviews, focus groups, observations, and document analysis. It seeks to understand the deeper meaning and context of a phenomenon or situation and is often used in social sciences, psychology, and humanities. Qualitative data collection methods allow for a more in-depth and holistic exploration of research questions and can provide rich and nuanced insights into human behavior and experiences.
Quantitative Data Collection
Quantitative data collection is a used to gather numerical data that can be analyzed using statistical methods. This data is typically collected through surveys, experiments, and other structured data collection methods. Quantitative data collection seeks to quantify and measure variables, such as behaviors, attitudes, and opinions, in a systematic and objective way. This data is often used to test hypotheses, identify patterns, and establish correlations between variables. Quantitative data collection methods allow for precise measurement and generalization of findings to a larger population. It is commonly used in fields such as economics, psychology, and natural sciences.
Data Collection Methods
Data Collection Methods are as follows:
Surveys involve asking questions to a sample of individuals or organizations to collect data. Surveys can be conducted in person, over the phone, or online.
Interviews involve a one-on-one conversation between the interviewer and the respondent. Interviews can be structured or unstructured and can be conducted in person or over the phone.
Focus Groups
Focus groups are group discussions that are moderated by a facilitator. Focus groups are used to collect qualitative data on a specific topic.
Observation
Observation involves watching and recording the behavior of people, objects, or events in their natural setting. Observation can be done overtly or covertly, depending on the research question.
Experiments
Experiments involve manipulating one or more variables and observing the effect on another variable. Experiments are commonly used in scientific research.
Case Studies
Case studies involve in-depth analysis of a single individual, organization, or event. Case studies are used to gain detailed information about a specific phenomenon.
Secondary Data Analysis
Secondary data analysis involves using existing data that was collected for another purpose. Secondary data can come from various sources, such as government agencies, academic institutions, or private companies.
How to Collect Data
The following are some steps to consider when collecting data:
- Define the objective : Before you start collecting data, you need to define the objective of the study. This will help you determine what data you need to collect and how to collect it.
- Identify the data sources : Identify the sources of data that will help you achieve your objective. These sources can be primary sources, such as surveys, interviews, and observations, or secondary sources, such as books, articles, and databases.
- Determine the data collection method : Once you have identified the data sources, you need to determine the data collection method. This could be through online surveys, phone interviews, or face-to-face meetings.
- Develop a data collection plan : Develop a plan that outlines the steps you will take to collect the data. This plan should include the timeline, the tools and equipment needed, and the personnel involved.
- Test the data collection process: Before you start collecting data, test the data collection process to ensure that it is effective and efficient.
- Collect the data: Collect the data according to the plan you developed in step 4. Make sure you record the data accurately and consistently.
- Analyze the data: Once you have collected the data, analyze it to draw conclusions and make recommendations.
- Report the findings: Report the findings of your data analysis to the relevant stakeholders. This could be in the form of a report, a presentation, or a publication.
- Monitor and evaluate the data collection process: After the data collection process is complete, monitor and evaluate the process to identify areas for improvement in future data collection efforts.
- Ensure data quality: Ensure that the collected data is of high quality and free from errors. This can be achieved by validating the data for accuracy, completeness, and consistency.
- Maintain data security: Ensure that the collected data is secure and protected from unauthorized access or disclosure. This can be achieved by implementing data security protocols and using secure storage and transmission methods.
- Follow ethical considerations: Follow ethical considerations when collecting data, such as obtaining informed consent from participants, protecting their privacy and confidentiality, and ensuring that the research does not cause harm to participants.
- Use appropriate data analysis methods : Use appropriate data analysis methods based on the type of data collected and the research objectives. This could include statistical analysis, qualitative analysis, or a combination of both.
- Record and store data properly: Record and store the collected data properly, in a structured and organized format. This will make it easier to retrieve and use the data in future research or analysis.
- Collaborate with other stakeholders : Collaborate with other stakeholders, such as colleagues, experts, or community members, to ensure that the data collected is relevant and useful for the intended purpose.
Applications of Data Collection
Data collection methods are widely used in different fields, including social sciences, healthcare, business, education, and more. Here are some examples of how data collection methods are used in different fields:
- Social sciences : Social scientists often use surveys, questionnaires, and interviews to collect data from individuals or groups. They may also use observation to collect data on social behaviors and interactions. This data is often used to study topics such as human behavior, attitudes, and beliefs.
- Healthcare : Data collection methods are used in healthcare to monitor patient health and track treatment outcomes. Electronic health records and medical charts are commonly used to collect data on patients’ medical history, diagnoses, and treatments. Researchers may also use clinical trials and surveys to collect data on the effectiveness of different treatments.
- Business : Businesses use data collection methods to gather information on consumer behavior, market trends, and competitor activity. They may collect data through customer surveys, sales reports, and market research studies. This data is used to inform business decisions, develop marketing strategies, and improve products and services.
- Education : In education, data collection methods are used to assess student performance and measure the effectiveness of teaching methods. Standardized tests, quizzes, and exams are commonly used to collect data on student learning outcomes. Teachers may also use classroom observation and student feedback to gather data on teaching effectiveness.
- Agriculture : Farmers use data collection methods to monitor crop growth and health. Sensors and remote sensing technology can be used to collect data on soil moisture, temperature, and nutrient levels. This data is used to optimize crop yields and minimize waste.
- Environmental sciences : Environmental scientists use data collection methods to monitor air and water quality, track climate patterns, and measure the impact of human activity on the environment. They may use sensors, satellite imagery, and laboratory analysis to collect data on environmental factors.
- Transportation : Transportation companies use data collection methods to track vehicle performance, optimize routes, and improve safety. GPS systems, on-board sensors, and other tracking technologies are used to collect data on vehicle speed, fuel consumption, and driver behavior.
Examples of Data Collection
Examples of Data Collection are as follows:
- Traffic Monitoring: Cities collect real-time data on traffic patterns and congestion through sensors on roads and cameras at intersections. This information can be used to optimize traffic flow and improve safety.
- Social Media Monitoring : Companies can collect real-time data on social media platforms such as Twitter and Facebook to monitor their brand reputation, track customer sentiment, and respond to customer inquiries and complaints in real-time.
- Weather Monitoring: Weather agencies collect real-time data on temperature, humidity, air pressure, and precipitation through weather stations and satellites. This information is used to provide accurate weather forecasts and warnings.
- Stock Market Monitoring : Financial institutions collect real-time data on stock prices, trading volumes, and other market indicators to make informed investment decisions and respond to market fluctuations in real-time.
- Health Monitoring : Medical devices such as wearable fitness trackers and smartwatches can collect real-time data on a person’s heart rate, blood pressure, and other vital signs. This information can be used to monitor health conditions and detect early warning signs of health issues.
Purpose of Data Collection
The purpose of data collection can vary depending on the context and goals of the study, but generally, it serves to:
- Provide information: Data collection provides information about a particular phenomenon or behavior that can be used to better understand it.
- Measure progress : Data collection can be used to measure the effectiveness of interventions or programs designed to address a particular issue or problem.
- Support decision-making : Data collection provides decision-makers with evidence-based information that can be used to inform policies, strategies, and actions.
- Identify trends : Data collection can help identify trends and patterns over time that may indicate changes in behaviors or outcomes.
- Monitor and evaluate : Data collection can be used to monitor and evaluate the implementation and impact of policies, programs, and initiatives.
When to use Data Collection
Data collection is used when there is a need to gather information or data on a specific topic or phenomenon. It is typically used in research, evaluation, and monitoring and is important for making informed decisions and improving outcomes.
Data collection is particularly useful in the following scenarios:
- Research : When conducting research, data collection is used to gather information on variables of interest to answer research questions and test hypotheses.
- Evaluation : Data collection is used in program evaluation to assess the effectiveness of programs or interventions, and to identify areas for improvement.
- Monitoring : Data collection is used in monitoring to track progress towards achieving goals or targets, and to identify any areas that require attention.
- Decision-making: Data collection is used to provide decision-makers with information that can be used to inform policies, strategies, and actions.
- Quality improvement : Data collection is used in quality improvement efforts to identify areas where improvements can be made and to measure progress towards achieving goals.
Characteristics of Data Collection
Data collection can be characterized by several important characteristics that help to ensure the quality and accuracy of the data gathered. These characteristics include:
- Validity : Validity refers to the accuracy and relevance of the data collected in relation to the research question or objective.
- Reliability : Reliability refers to the consistency and stability of the data collection process, ensuring that the results obtained are consistent over time and across different contexts.
- Objectivity : Objectivity refers to the impartiality of the data collection process, ensuring that the data collected is not influenced by the biases or personal opinions of the data collector.
- Precision : Precision refers to the degree of accuracy and detail in the data collected, ensuring that the data is specific and accurate enough to answer the research question or objective.
- Timeliness : Timeliness refers to the efficiency and speed with which the data is collected, ensuring that the data is collected in a timely manner to meet the needs of the research or evaluation.
- Ethical considerations : Ethical considerations refer to the ethical principles that must be followed when collecting data, such as ensuring confidentiality and obtaining informed consent from participants.
Advantages of Data Collection
There are several advantages of data collection that make it an important process in research, evaluation, and monitoring. These advantages include:
- Better decision-making : Data collection provides decision-makers with evidence-based information that can be used to inform policies, strategies, and actions, leading to better decision-making.
- Improved understanding: Data collection helps to improve our understanding of a particular phenomenon or behavior by providing empirical evidence that can be analyzed and interpreted.
- Evaluation of interventions: Data collection is essential in evaluating the effectiveness of interventions or programs designed to address a particular issue or problem.
- Identifying trends and patterns: Data collection can help identify trends and patterns over time that may indicate changes in behaviors or outcomes.
- Increased accountability: Data collection increases accountability by providing evidence that can be used to monitor and evaluate the implementation and impact of policies, programs, and initiatives.
- Validation of theories: Data collection can be used to test hypotheses and validate theories, leading to a better understanding of the phenomenon being studied.
- Improved quality: Data collection is used in quality improvement efforts to identify areas where improvements can be made and to measure progress towards achieving goals.
Limitations of Data Collection
While data collection has several advantages, it also has some limitations that must be considered. These limitations include:
- Bias : Data collection can be influenced by the biases and personal opinions of the data collector, which can lead to inaccurate or misleading results.
- Sampling bias : Data collection may not be representative of the entire population, resulting in sampling bias and inaccurate results.
- Cost : Data collection can be expensive and time-consuming, particularly for large-scale studies.
- Limited scope: Data collection is limited to the variables being measured, which may not capture the entire picture or context of the phenomenon being studied.
- Ethical considerations : Data collection must follow ethical principles to protect the rights and confidentiality of the participants, which can limit the type of data that can be collected.
- Data quality issues: Data collection may result in data quality issues such as missing or incomplete data, measurement errors, and inconsistencies.
- Limited generalizability : Data collection may not be generalizable to other contexts or populations, limiting the generalizability of the findings.
About the author
Muhammad Hassan
Researcher, Academic Writer, Web developer
You may also like

Research Project – Definition, Writing Guide and...

Research Recommendations – Examples and Writing...


Theoretical Framework – Types, Examples and...

Institutional Review Board – Application Sample...

Research Design – Types, Methods and Examples

Significance of the Study – Examples and Writing...
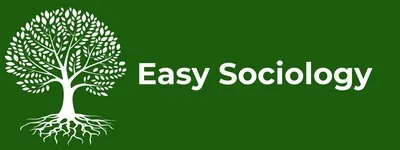
- Books, Journals, Papers
- Guides & How To’s
- Life Around The World
- Research Methods
- Functionalism
- Postmodernism
- Social Constructionism
- Structuralism
- Symbolic Interactionism
- Sociology Theorists
- General Sociology
- Social Policy
- Social Work
- Sociology of Childhood
- Sociology of Crime & Deviance
- Sociology of Art
- Sociology of Dance
- Sociology of Food
- Sociology of Sport
- Sociology of Disability
- Sociology of Economics
- Sociology of Education
- Sociology of Emotion
- Sociology of Family & Relationships
- Sociology of Gender
- Sociology of Health
- Sociology of Identity
- Sociology of Ideology
- Sociology of Inequalities
- Sociology of Knowledge
- Sociology of Language
- Sociology of Law
- Sociology of Anime
- Sociology of Film
- Sociology of Gaming
- Sociology of Literature
- Sociology of Music
- Sociology of TV
- Sociology of Migration
- Sociology of Nature & Environment
- Sociology of Politics
- Sociology of Power
- Sociology of Race & Ethnicity
- Sociology of Religion
- Sociology of Sexuality
- Sociology of Social Movements
- Sociology of Technology
- Sociology of the Life Course
- Sociology of Travel & Tourism
- Sociology of Violence & Conflict
- Sociology of Work
- Urban Sociology
- Changing Relationships Within Families
- Conjugal Role Relationships
- Criticisms of Families
- Family Forms
- Functions of the Family
- Featured Articles
- Privacy Policy
- Terms & Conditions
Understanding a Univariate Analysis
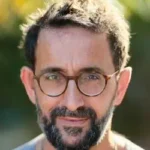
Table of Contents
What is a univariate analysis, importance of univariate analysis in sociological research, common methods used in univariate analysis.
- Applying Univariate Analysis in Sociological Research
- Limitations of Univariate Analysis
In sociological research, data analysis plays a crucial role in uncovering patterns, relationships, and explanations for various social phenomena. One of the most fundamental forms of statistical analysis used in sociology is the univariate analysis. At its core, univariate analysis involves the examination of a single variable at a time. This type of analysis serves as the foundation for more complex statistical methods and provides an essential understanding of the data before moving on to more advanced analyses. In this article, we will explore what univariate analysis entails, its importance in sociology, the methods used to perform it, and how it is applied to social research.
Univariate analysis refers to the process of analyzing one variable in a dataset without considering relationships with other variables. The word “univariate” can be broken down into “uni,” meaning “one,” and “variate,” meaning “variable.” Thus, univariate analysis focuses on a single dimension of the data, helping sociologists and researchers to describe the basic features of that specific variable.
The primary goal of univariate analysis is descriptive. It seeks to summarize and understand the characteristics of the variable in question, such as its distribution, central tendency (e.g., mean, median, and mode), and dispersion (e.g., range, variance, and standard deviation). For instance, if a sociologist is interested in the age distribution of a particular population, they might conduct a univariate analysis of the “age” variable to uncover patterns such as the average age, the most common age, or the spread of ages within the group.
Univariate analysis is a crucial first step in data analysis because it allows researchers to gain an understanding of each variable individually before exploring how variables relate to one another in more complex analyses such as bivariate or multivariate analysis. This helps to ensure that the data is understood at a basic level and allows for the identification of any potential anomalies, outliers, or data entry errors.
In sociology, univariate analysis serves multiple essential purposes. First and foremost, it provides a straightforward approach to exploring data, enabling researchers to summarize large datasets and communicate key findings clearly. Sociologists often work with extensive datasets, such as survey results, census data, or observational studies. Conducting a univariate analysis allows researchers to efficiently organize and describe these datasets, providing the groundwork for further inquiry.
Another important reason for conducting univariate analysis is that it facilitates data cleaning and preparation. Through univariate analysis, sociologists can identify missing values , outliers, or inaccuracies in the data. For example, if a researcher is studying income levels within a population, and the univariate analysis reveals some income values that are impossibly high or low, these outliers can be investigated and potentially corrected or removed from the dataset.
Moreover, univariate analysis enables sociologists to explore social inequalities and distributions of resources or experiences across different groups. For instance, examining the distribution of education levels or income across a population may reveal patterns of social stratification and inequality. This can then prompt further research to investigate the causes and consequences of these disparities, ultimately contributing to the development of sociological theory.
Lastly, univariate analysis provides a foundation for comparison. Understanding the basic characteristics of a variable, such as its central tendency and variability, is essential before comparing it with other variables in a bivariate or multivariate analysis . For example, understanding the average income of a population is a prerequisite before comparing it with education levels, gender, or other factors that might explain income disparities.
Several statistical methods and tools are commonly employed in univariate analysis to describe the characteristics of a single variable. These methods focus on summarizing the data through measures of central tendency , dispersion, and distribution.
Measures of Central Tendency
One of the key components of univariate analysis is understanding the central tendency of a variable. Central tendency refers to the point around which the values of a variable cluster. In sociology, three primary measures of central tendency are often used:
- Mean : The mean is the arithmetic average of a set of values. It is calculated by summing all the values of the variable and dividing by the number of observations. The mean is useful for understanding the overall level of the variable, but it can be sensitive to outliers. For instance, when studying income, a few extremely high-income individuals can inflate the mean, making it appear that the population is wealthier than it actually is.
- Median : The median is the middle value when the data is ordered from least to greatest. It is a useful measure of central tendency when the data is skewed or contains outliers, as it is not affected by extreme values. For example, if the researcher is studying home prices in a city, the median may provide a better indication of typical home prices than the mean, especially if there are a few extremely expensive properties.
- Mode : The mode is the value that occurs most frequently in the dataset. While the mode may not always provide deep insights into the distribution of data, it can be helpful in certain cases. For example, in survey research, the mode can reveal the most commonly selected response to a question, offering a clear representation of majority opinions or behaviors.
Measures of Dispersion
In addition to central tendency, univariate analysis also examines how the values of a variable are spread out or dispersed. Measures of dispersion provide insights into the variability or spread of the data. Common measures of dispersion include:
- Range : The range is the difference between the maximum and minimum values in a dataset. It gives a basic sense of how spread out the data is, but it can be heavily influenced by outliers. A variable with a large range may suggest significant differences within the population being studied.
- Variance : Variance measures the average squared deviation from the mean. It provides a more sophisticated understanding of dispersion than the range. A higher variance indicates that the data points are more spread out from the mean, while a lower variance suggests that the data points are closer to the mean.
- Standard Deviation : The standard deviation is the square root of the variance. It offers an intuitive understanding of how much the values deviate from the mean. In sociological research, a high standard deviation for a variable like income would indicate that there is substantial inequality within the population, whereas a low standard deviation suggests that incomes are more similar.
Frequency Distributions
Membership required.
You must be a member to access this content.
View Membership Levels
Mr Edwards has a PhD in sociology and 10 years of experience in sociological knowledge
Related Articles
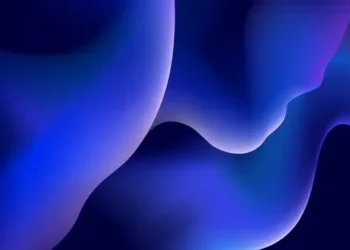
What is Theory Building in Sociology?
Theory building is a fundamental process in the field of sociology that involves the systematic development of theoretical frameworks to...
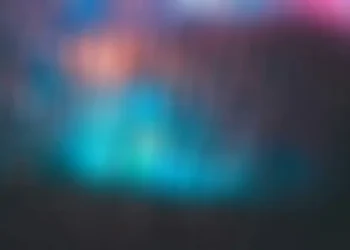
Understanding Heuristic Devices in Sociology
In the realm of sociology, a heuristic device is an invaluable conceptual tool that aids in the exploration, explanation, and...

How to Conduct a Chi-Square Test

Institutionalization: An Overview

Total Institutions Explained
Get the latest sociology.
Would you be interested in enrolling in courses from Easy Sociology?
Recommended
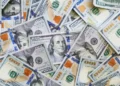
(Watch) Paywall: The Business of Scholarship
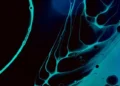
24 Hour Trending
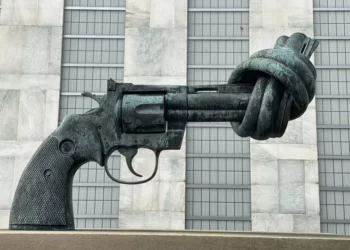
Pierre Bourdieu’s Symbolic Violence: An Outline and Explanation
The work and contributions of emile durkheim in sociology, the connection between education and social stratification, robert merton’s strain theory: understanding societal pressure and deviance, the role and functions of the education system: exploring its relationship to the economy and class structure.
Easy Sociology makes sociology as easy as possible. Our aim is to make sociology accessible for everybody. © 2023 Easy Sociology
© 2023 Easy Sociology
Advertisement
U-shaped association between hemoglobin levels and albuminuria in US adults: a cross-sectional study
- Nephrology – Original Paper
- Published: 08 September 2024
Cite this article
- Rong Yin 1 , 2 &
- Zhangxue Hu 1
This study aimed to explore the correlation between hemoglobin levels and albuminuria in US adults.
This cross-sectional investigation analyzed the National Health and Nutrition Examination Survey (NHANES) information from 2011 to 2020. Data on hemoglobin, albuminuria, and other variables were collected from all participants. The logistic-regression analyses and smoothed curves were used to substantiate the research objectives.
The average age of the 8,868 participants was 49.5 ± 17.3 years, and 49.3% were men. The prevalence of albuminuria was 12.1%. After adjusting for potential variables in the logistic-regression analysis models, hemoglobin (per 1 g/dL increase) was inversely associated with the presence of albuminuria (odds ratio [OR], 0.92; 95% confidence interval [95%CI], 0.87–0.97). Compared with participants in quartile 3 (Q3, 14.1–15.0 g/dL) for hemoglobin levels, those in the lowest quartile 1 (Q1, 6.1–13.0 g/dL) and highest quartile 4 (Q4, 15.1–19.6 g/dL) had adjusted ORs for albuminuria of 1.48 (95% CI, 1.19–1.85) and 1.11 (95% CI, 0.9–1.38), respectively. Our observations indicated a U-shaped association between hemoglobin levels and albuminuria, with a point of inflection at approximately 15.5 g/dL. The effect sizes and CIs below and above this point were 0.853 (95% CI, 0.798–0.912) and 1.377 (95% CI, 1.055–1.797), respectively.
This study indicates that the presence of albuminuria is linked to both low and high hemoglobin levels in US adults. The management of hemoglobin may benefit kidney health.
This is a preview of subscription content, log in via an institution to check access.
Access this article
Subscribe and save.
- Get 10 units per month
- Download Article/Chapter or eBook
- 1 Unit = 1 Article or 1 Chapter
- Cancel anytime
Price includes VAT (Russian Federation)
Instant access to the full article PDF.
Rent this article via DeepDyve
Institutional subscriptions
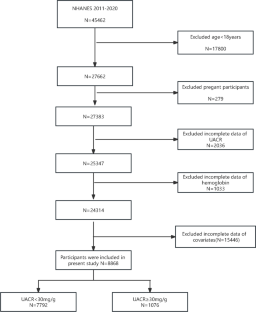
Data availability
The study was analyzed with publicly available datasets from NHANES at https://www.cdc.gov/nchs/nhanes/ .
Matsushita K, Ballew SH, Wang AY, Kalyesubula R, Schaeffner E, Agarwal R (2022) Epidemiology and risk of cardiovascular disease in populations with chronic kidney disease. Nat Rev Nephrol 18(11):696–707. https://doi.org/10.1038/s41581-022-00616-6
Article PubMed Google Scholar
Usherwood T, Lee V (2021) Advances in chronic kidney disease pathophysiology and management. Aust J Gen Pract. 50:188–192. https://doi.org/10.31128/AJGP-11-20-5735
Collaboration GBDCKD (2020) Global, regional, and national burden of chronic kidney disease, 1990–2017: a systematic analysis for the Global Burden of Disease Study 2017. Lancet 395(10225):709–733. https://doi.org/10.1016/S0140-6736(20)30045-3
Article Google Scholar
Ataga KI, Zhou Q, Derebail VK, Saraf SL, Hankins JS, Loehr LR, Garrett ME, Ashley-Koch AE, Cai J, Telen MJ (2021) Rapid decline in estimated glomerular filtration rate in sickle cell anemia: results of a multicenter pooled analysis. Haematologica 106(6):1749. https://doi.org/10.3324/haematol.2020.267419
Article CAS PubMed Google Scholar
Elsherif L, Kanthakumar P, Afolabi J, Stratton AF, Ogu U, Nelson M, Mukhopadhyay A, Smeltzer MP, Adebiyi A, Ataga KI (2023) Urinary angiotensinogen is associated with albuminuria in adults with sickle cell anaemia. Br J Haematol 202(3):669–673. https://doi.org/10.1111/bjh.18862
Niss O, Lane A, Asnani MR, Yee ME, Raj A, Creary S, Fitzhugh C, Bodas P, Saraf SL, Sarnaik S, Devarajan P, Malik P (2020) Progression of albuminuria in patients with sickle cell anemia: a multicenter, longitudinal study. Blood Adv 4(7):1501–1511. https://doi.org/10.1182/bloodadvances.2019001378
Article PubMed PubMed Central Google Scholar
Okada H, Hasegawa G, Tanaka M, Osaka T, Shiotsu Y, Narumiya H, Inoue M, Nakano K, Nakamura N, Fukui M (2015) Association between hemoglobin concentration and the progression or development of albuminuria in diabetic kidney disease. PLoS ONE 10(5):e0129192. https://doi.org/10.1371/journal.pone.0129192
Article CAS PubMed PubMed Central Google Scholar
Adetunji OR, Mani H, Olujohungbe A, Abraham KA, Gill GV (2009) ‘Microalbuminuric anaemia’—the relationship between haemoglobin levels and albuminuria in diabetes. Diabetes Res Clin Pract 85(2):179–182. https://doi.org/10.1016/j.diabres.2009.04.028
Wang H, Tang C, Dang Z, Yong A, Liu L, Wang S, Zhao M (2022) Clinicopathological characteristics of high-altitude polycythemia-related kidney disease in Tibetan inhabitants. Kidney Int 102(1):196–206. https://doi.org/10.1016/j.kint.2022.03.027
Jefferson JA, Escudero E, Hurtado ME, Kelly JP, Swenson ER, Wener MH, Burnier M, Maillard M, Schreiner GF, Schoene RB, Hurtado A, Johnson RJ (2002) Hyperuricemia, hypertension, and proteinuria associated with high-altitude polycythemia. Am J Kidney Dis 39(6):1135–1142. https://doi.org/10.1053/ajkd.2002.33380
Liu H, Wang D, Wu F, Dong Z, Yu S (2023) Association between inflammatory potential of diet and self-reported severe headache or migraine: a cross-sectional study of the National Health and Nutrition Examination Survey. Nutrition. https://doi.org/10.1016/j.nut.2023.112098
Chu CD, Xia F, Du YX, Singh R, Tuot DS, Lamprea-Montealegre JA, Gualtieri R, Liao N, Kong SX, Williamson T, Shlipak MG, Estrella MM (2023) Estimated prevalence and testing for albuminuria in US adults at risk for chronic kidney disease. Jama Netw Open. https://doi.org/10.1001/jamanetworkopen.2023.26230
Stevens PE, Levin A (2013) Evaluation and management of chronic kidney disease: synopsis of the kidney disease: improving global outcomes 2012 clinical practice guideline. Ann Intern Med 158(11):825–830. https://doi.org/10.7326/0003-4819-158-11-201306040-00007
Oparil S, Acelajado MC, Bakris GL, Berlowitz DR, Cifkova R, Dominiczak AF, Grassi G, Jordan J, Poulter NR, Rodgers A, Whelton PK (2018) Hypertension. Nat Rev Dis Prim 4:18014. https://doi.org/10.1038/nrdp.2018.14
ElSayed NA, Aleppo G, Bannuru RR, Bruemmer D, Collins BS, Ekhlaspour L, Gaglia JL, Hilliard ME, Johnson EL, Khunti K, Lingvay I, Matfin G, McCoy RG, Perry ML, Pilla SJ, Polsky S, Prahalad P, Pratley RE, Segal AR, Gabbay RA (2024) Diagnosis and classification of diabetes: standards of care in diabetes—2024. Diabetes Care 47(1):S20–S42. https://doi.org/10.2337/dc24-S002
González-Muniesa P, Mártinez-González M-A, Hu FB, Després J-P, Matsuzawa Y, Loos RJF, Moreno LA, Bray GA, Martinez JA (2017) Obesity. Nature Rev Dis. https://doi.org/10.1038/nrdp.2017.34
Lippi G, Guidi GC, Targher G (2009) Relationship between albuminuria and hemoglobin level. Diabetes Res Clin Pract 86(3):e62–e63. https://doi.org/10.1016/j.diabres.2009.09.021
Shimosawa T, Han JS, Lee MJ, Park KS, Han SH, Yoo T-H, Oh K-H, Park SK, Lee J, Hyun YY, Chung W, Kim YH, Ahn C, Choi KH (2015) Albuminuria as a risk factor for anemia in chronic kidney disease: result from the Korean Cohort study for outcomes in patients with chronic kidney disease (KNOW-CKD). PLoS ONE. https://doi.org/10.1371/journal.pone.0139747
Warnecke C, Zaborowska Z, Kurreck J, Erdmann VA, Frei U, Wiesener M, Eckardt KU (2004) Differentiating the functional role of hypoxia-inducible factor (HIF)-1α and HIF-2α (EPAS-1) by the use of RNA interference: erythropoietin is a HIF-2α target gene in Hep3B and Kelly cells. FASEB J 18(12):1462–1464
Rosenberger C, Mandriota S, Jurgensen JS, Wiesener MS, Horstrup JH, Frei U, Ratcliffe PJ, Maxwell PH, Bachmann S, Eckardt KU (2002) Expression of hypoxia-inducible factor-1alpha and -2alpha in hypoxic and ischemic rat kidneys. J Am Soc Nephrol 13(7):1721–1732. https://doi.org/10.1097/01.asn.0000017223.49823.2a
Lu H, Kapur G, Mattoo TK, Lyman WD (2012) Hypoxia decreases podocyte expression of slit diaphragm proteins. Int J Nephrol Renovasc Dis 5:101–107. https://doi.org/10.2147/IJNRD.S27332
Singh AK, Kolligundla LP, Francis J, Pasupulati AK (2021) Detrimental effects of hypoxia on glomerular podocytes. J Physiol Biochem 77(2):193–203. https://doi.org/10.1007/s13105-021-00788-y
Takahashi N, Yoshida H, Kimura H, Kamiyama K, Kurose T, Sugimoto H, Imura T, Yokoi S, Mikami D, Kasuno K, Kurosawa H, Hirayama Y, Naiki H, Hara M, Iwano M (2020) Chronic hypoxia exacerbates diabetic glomerulosclerosis through mesangiolysis and podocyte injury in db/db mice. Nephrol Dial Transpl 35(10):1678–1688. https://doi.org/10.1093/ndt/gfaa074
Article CAS Google Scholar
Kagami S (2012) Involvement of glomerular renin-angiotensin system (RAS) activation in the development and progression of glomerular injury. Clin Exp Nephrol 16(2):214–220. https://doi.org/10.1007/s10157-011-0568-0
Dittrich S, Buhrer C, Muller C, Dahnert I, Lange PE (1998) Renal impairment in patients with long-standing cyanotic congenital heart disease. Acta Paediatr 87:949–954. https://doi.org/10.1080/080352598750031608
Shayo FK, Lutale J (2018) Albuminuria in patients with chronic obstructive pulmonary disease: a cross-sectional study in an African patient cohort. BMC Pulm Med. https://doi.org/10.1186/s12890-018-0694-5
Xu L, Chen Y, Xie Z, He Q, Chen S, Wang W, Liu G, Liao Y, Lu C, Hao L, Sun J, Shi W, Liang X (2019) High hemoglobin is associated with increased in-hospital death in patients with chronic obstructive pulmonary disease and chronic kidney disease: a retrospective multicenter population-based study. BMC Pulm Med. https://doi.org/10.1186/s12890-019-0933-4
Palubiski LM, O’Halloran KD, O’Neill J (2020) Renal physiological adaptation to high altitude: a systematic review. Front Physiol 11:756. https://doi.org/10.3389/fphys.2020.00756
Brenner BM, Lawler EV, Mackenzie HS (1996) The hyperfiltration theory: a paradigm shift in nephrology. Kidney Int 49(6):1774–1777. https://doi.org/10.1038/ki.1996.265
Srivastava T, Hariharan S, Alon US, McCarthy ET, Sharma R, El-Meanawy A, Savin VJ, Sharma M (2018) Hyperfiltration-mediated Injury in the remaining kidney of a transplant donor. Transplantation 102(10):1624–1635. https://doi.org/10.1097/TP.0000000000002304
Wiggins JE, Goyal M, Sanden SK, Wharram BL, Shedden KA, Misek DE, Kuick RD, Wiggins RC (2005) Podocyte hypertrophy, “adaptation,” and “decompensation” associated with glomerular enlargement and glomerulosclerosis in the aging rat: prevention by calorie restriction. J Am Soc Nephrol 16(10):2953–2966. https://doi.org/10.1681/ASN.2005050488
Download references
Acknowledgements
We are grateful for the technical support and useful tools provided by the Free Statistics Team for data analysis and visualization.
No funding was received for conducting this study.
Author information
Authors and affiliations.
Department of Nephrology, West China Hospital, Sichuan University, Chengdu, China
Rong Yin & Zhangxue Hu
Department of Nephrology, Hospital of Chengdu Office of People’s Government of Tibet Autonomous Region, Chengdu, Sichuan, China
You can also search for this author in PubMed Google Scholar
Contributions
Conception and design: YR and HZX; Data collection, statistical analysis, and writing: YR, Supervision and review: HZX. All authors contributed to the manuscript and acknowledge the supplied version.
Corresponding author
Correspondence to Zhangxue Hu .
Ethics declarations
Conflict of interest.
The authors declare no competing interests.
Ethics statement
The NCHS Research Institutional Review Board approved all of the research. The data from the user agreement is available online.
Informed consent
The qualifying subjects gave their informed consent before the data collection and NHANES health screening started.
Additional information
Publisher's note.
Springer Nature remains neutral with regard to jurisdictional claims in published maps and institutional affiliations.
Supplementary Information
Below is the link to the electronic supplementary material.
11255_2024_4200_MOESM1_ESM.tiff
Supplementary Information Fig. 1 Effect size of hemoglobin on albuminuria in each subgroup OR, odds ratio; CI, confidence interval; BMI, body mass index. Adjustment factors included age, sex, race, education, marital status, ratio of family income to poverty, smoking, physical activity, body mass index, waist circumference, fasting blood glucose, serum uric acid, low-density lipoprotein cholesterol, total cholesterol, high-density lipoprotein cholesterol, systolic blood pressure, diastolic blood pressure, estimated glomerular filtration rate, triglycerides, serum creatinine, hypertension, and diabetes (TIFF 21060 kb)
Rights and permissions
Springer Nature or its licensor (e.g. a society or other partner) holds exclusive rights to this article under a publishing agreement with the author(s) or other rightsholder(s); author self-archiving of the accepted manuscript version of this article is solely governed by the terms of such publishing agreement and applicable law.
Reprints and permissions
About this article
Yin, R., Hu, Z. U-shaped association between hemoglobin levels and albuminuria in US adults: a cross-sectional study. Int Urol Nephrol (2024). https://doi.org/10.1007/s11255-024-04200-8
Download citation
Received : 20 June 2024
Accepted : 02 September 2024
Published : 08 September 2024
DOI : https://doi.org/10.1007/s11255-024-04200-8
Share this article
Anyone you share the following link with will be able to read this content:
Sorry, a shareable link is not currently available for this article.
Provided by the Springer Nature SharedIt content-sharing initiative
- Albuminuria
- National health and nutrition examination survey (NHANES)
- Cross-sectional study
- Find a journal
- Publish with us
- Track your research
- Open access
- Published: 04 September 2024
Genome-wide identification of the phenylalanine ammonia-lyase gene from Epimedium Pubescens Maxim. (Berberidaceae): novel insight into the evolution of the PAL gene family
- Chaoqun Xu 1 na1 ,
- Xuelan Fan 1 , 2 na1 ,
- Guoan Shen 1 &
- Baolin Guo 1
BMC Plant Biology volume 24 , Article number: 831 ( 2024 ) Cite this article
Metrics details
Phenylalanine ammonia-lyase (PAL) serves as a key gateway enzyme, bridging primary metabolism and the phenylpropanoid pathway, and thus playing an indispensable role in flavonoid, anthocyanin and lignin biosynthesis. PAL gene families have been extensively studied across species using public genomes. However, a comprehensive exploration of PAL genes in Epimedium species, especially those involved in prenylated flavonol glycoside, anthocyanin, or lignin biosynthesis, is still lacking. Moreover, an in-depth investigation into PAL gene family evolution is warranted.
Seven PAL genes ( EpPAL1 - EpPAL7 ) were identified. EpPAL2 and EpPAL3 exhibit low sequence identity to other EpPALs (ranging from 61.09 to 64.38%) and contain two unique introns, indicating distinct evolutionary origins. They evolve at a rate ~ 10 to ~ 54 times slower compared to EpPAL1 and EpPAL4-7 , suggesting strong purifying selection. EpPAL1 evolved independently and is another ancestral gene. EpPAL1 formed EpPAL4 through segmental duplication, which lead to EpPAL5 and EpPAL6 through tandem duplications, and EpPAL7 through transposed duplication, shaping modern EpPALs . Correlation analysis suggests EpPAL1 , EpPAL2 and EpPAL3 play important roles in prenylated flavonol glycosides biosynthesis, with EpPAL2 and EpPAL3 strongly correlated with both Epimedin C and total prenylated flavonol glycosides. EpPAL1 , EpPAL2 and EpPAL3 may play a role in anthocyanin biosynthesis in leaves. EpPAL2 , EpPAL3 , EpPAL6 , and EpPAL7 might be engaged in anthocyanin production in petals, and EpPAL2 and EpPAL3 might also contribute to anthocyanin synthesis in sepals. Further experiments are needed to confirm these hypotheses. Novel insights into the evolution of PAL gene family suggest that it might have evolved from a monophyletic group in bryophytes to large-scale sequence differentiation in gymnosperms, basal angiosperms, and Magnoliidae. Ancestral gene duplications and vertical inheritance from gymnosperms to angiosperms likely occurred during PAL evolution. Most early-diverging eudicotyledons and monocotyledons have distinct histories, while modern angiosperm PAL gene families share similar patterns and lack distant gene types.
Conclusions
EpPAL2 and EpPAL3 may play crucial roles in biosynthesis of prenylated flavonol glycosides and anthocyanins in leaves and flowers. This study provides novel insights into PAL gene family evolution. The findings on PAL genes in E. pubescens will aid in synthetic biology research on prenylated flavonol glycosides production.
Peer Review reports
Introduction
Phenylalanine ammonia-lyase (PAL, EC 4.3.1.24) is the first critical enzyme in the phenylpropanoid pathway, catalyzing the biotransformation of L-phenylalanine to trans-cinnamic acid. Acting as a bridge, PAL mediates carbon flux from primary to secondary metabolism, leading to the production of flavonoids, anthocyanins, tannins, lignins, phytoalexins and other benzene-based compounds with pharmaceutical value [ 1 , 2 ]. The phenylpropanoid derivatives play crucial roles in plant defense against a range of biotic (e.g., insects and pathogens) and abiotic stresses (e.g., UV light, low temperature and nutrient stress). These compounds function as regulatory molecules, participating in signal transduction and communication with other organisms [ 3 ]. Furthermore, they contribute to lignin biosynthesis, which is essential for maintaining stem rigidity, vascular integrity, and providing a physical barrier against invading pathogens in plants [ 4 , 5 ].
PAL enzymes in dicots typically exhibit monofunctionality, specifically catalyzing the PAL reaction. However, in certain monocots, particularly those belonging to the grass family Poaceae, PAL enzymes can display bifunctionality, catalyzing both PAL and TAL reactions with phenylalanine and tyrosine as substrates, respectively. Notably, PAL and TAL enzymes are absent in animals, where they have been replaced by HAL (L-histidine ammonia-lyase) [ 2 , 6 , 7 ]. The PAL gene family typically consists of 2–6 copies, although some species possess significantly more members [ 8 , 9 ]. Over the course of evolution, the expression of PAL genes in response to biotic and abiotic stresses has become highly regulated in a temporal and spatial manner, often resulting in the diversification of gene copies with redundant functions [ 10 , 11 , 12 ]. Given the diverse functions of PAL gene copies, it can be challenging to determine which copy predominantly modulates the biosynthesis of different branch end-products.
Herba Epimedii, a valued traditional Chinese medicine (TCM), is sourced exclusively from the dried leaves of four Epimedium species: namely E. pubescens , E. sagittatum , E. brevicornu and E. koreanum . Besides its traditional uses as a kidney tonic and antirheumatic agent [ 13 , 14 ], the aglycone of its primary bioactive components, known as prenylated flavonol glycosides (PFGs), particularly icaritin, has garnered recognition as a novel drug effective in inhibiting hepatocellular carcinoma (HCC) initiation and malignant growth [ 15 , 16 ]. However, the genes involved in PFGs biosynthesis in Epimedium , including PAL, remain fragmented. To date, only three PALs ( EsPAL1 , EsPAL2 and EsPAL3 ) in E. sagittatum [ 17 ] and one PAL ( EwPAL ) in E. wushanense [ 18 ] have been reported. Through qRT-PCR and correlation analysis, only EsPAL3 has been implicated in both PFGs and anthocyanin pathways. Meanwhile, EsPAL1 is suspected to play a role in lignin biosynthesis, while EsPAL2 demonstrates constitutive expression across all tissues, hinting at its potential involvement in lignin, PFGs and anthocyanin pathways. EwPAL , on the other hand, has been solely linked to the biosynthesis of naringenin. Further research into the PALs responsible for PFGs, anthocyanin or lignin biosynthesis needs to be clearly explored, which would facilitate more efficient synthesis of PFGs.
Previous studies on the evolution of the PAL gene family are limited, with only a few notable investigations reported: in Nelumbo nucifera by Wu et al. (2014) [ 19 ] and in three Cucurbitaceae plants by Dong et al. (2016) [ 8 ]. While the former study identified a distinct ancient NnPAL1 gene in the early-diverging dicotyledonous plant N. nucifera , it failed to explore similar patterns in other early-diverging dicotyledonous species. On the other hand, the latter study exclusively examined PAL evolution within Cucurbitaceae plants without delving into the evolutionary origins of the discovered PAL genes. E. pubescens , as another early-diverging dicotyledonous plant, emerges as a valuable subject for studying the evolution of the PAL gene family. Therefore, conducting in-depth research on this species becomes particularly significant.
In this study, a genome-wide search of E. pubescens led to the identification of 7 PAL genes. Among these, EpPAL2 and EpPAL3 exhibit significant sequence divergences, yet there is a lack of research exploring their distinct functional traits. This study aims to delve deeper into the gene functions of each EpPAL , with a particular focus on EpPAL2 and EpPAL3 . Furthermore, by utilizing E. pubescens as a representative of an early-diverging angiosperm, we aim to integrate prior research and employ a comprehensive set of 24 representative species to thoroughly examine the evolutionary history of the PAL gene family. The findings of this study have implications for deepening our understanding of the molecular functions of various EpPALs and providing valuable insights into the evolution of the PAL gene family.
Identification and chromosomal localization of EpPAL s
Putative PAL genes were retrieved and identified from E. pubescens by HMM search and BLASTP. A total of 7 PAL genes were identified. The full length of candidate genes was further confirmed to be correct using available transcriptome data of E. pubescens . According to the subcellular localization predictions, all EpPALs are in the cytoplasm. Detailed information of those genes is presented in Table 1 , including gene IDs, gene names, exon numbers, chromosome locations and protein length. The naming of EpPAL s was done according to the order of PAL on the chromosome of E. pubescens . 7 EpPALs were unevenly distributed across the whole genome. Specifically, EpPAL1 was located on chromosome 1, EpPAL2 and EpPAL3 were on chromosome 4, while EpPAL4 - EpPAL7 were allocated on chromosome 6 (Table 1 ).
Evolutionary analysis of PAL genes
To gain a deeper understanding of the evolution of PAL genes, we conducted a comprehensive analysis utilizing a diverse set of PAL members from 24 species, including Chara braunii (a charophyte), Physcomitrium patens (a bryophyte), Ceratopteris richardii (a fern), Ginkgo biloba and Sequoiadendron giganteum (gymnosperms), Amborella trichopoda and Nymphaea colorata (basal angiosperms), Ceratophyllum demersum (Ceratophyllales), Cinnamomum kanehirae , Liriodendron chinense and Piper nigrum (Magnoliidae), Spirodela polyrhiza (a basal monocot), Papaver somniferum , N. nucifera , Tetracentron sinense , Macadamia integrifolia , Aquilegia coerulea , Kingdonia uniflora and E. pubescens (early-diverging eudicotyledons), Brachypodium distachyon (an early-diverging monocotyledon), Oryza sativa (a representative modern monocotyledon), as well as A. thaliana , Cucumis sativus and Vitis vinifera (representative modern dicotyledons). Detailed gene mining instructions and sequences of the PAL genes from these 24 species are provided in Table S1 and Table S2 , respectively.
We constructed a phylogenetic tree using the maximum likelihood method to illustrate the differentiation profile of PAL genes among the aforementioned taxa (Fig. 1 ). As shown in Fig. 1 , the tree clearly divides all PAL genes into six distinct clusters (Cluster 1–6). Cluster 1 represents an evolutionary branch unique to charophytes, with a significantly longer branch length compared to other clusters, indicating that its PAL evolutionary clade is the farthest from the others. Cluster 2 and 4 exclusively contain gymnosperm genes, suggesting they may represent specific evolutionary branches unique to gymnosperms. Cluster 3 and 5 mainly include PAL genes from bryophytes, ferns, gymnosperms, basal angiosperms, Ceratophyllales, Magnoliidae, and early-diverging eudicotyledons, indicating a more primitive group of PALs. Cluster 6 primarily encompasses PAL clades from typical modern dicotyledons and monocotyledons, as well as homologous genes in ferns, gymnosperms, basal angiosperms, Magnoliidae, Ceratophyllales, and other taxa corresponding to modern PAL genes.
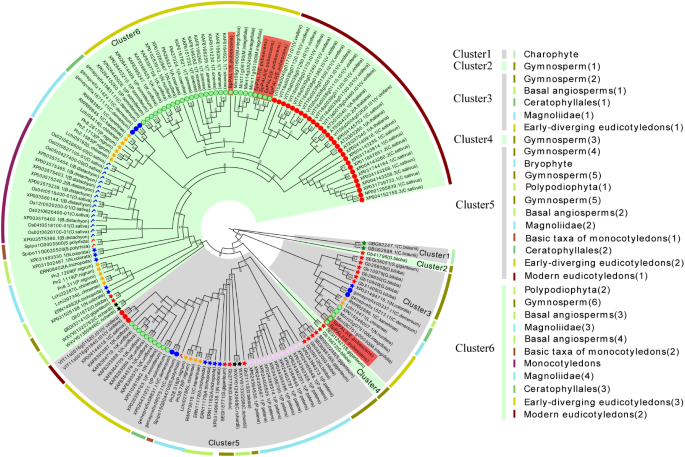
Phylogenetic tree of PAL sequences of 24 plant species with typical evolutionary relationships. EpPALs are marked with red box. The representation of the markings at the branch nodes is as follows: green five-pointed star: charophyte, red five-pointed star: gymnosperm, blue five-pointed star: basal angiosperms, orange five-pointed star: Magnoliidae, pink five-pointed star: bryophyte, black five-pointed star: polypodiophyta, green circle: early differentiated angiosperms, blue circle: Ceratophyllales, red circle: modern eudicotyledons, red checkmark: basic taxa of monocotyledons, and blue checkmark: monocotyledons. The attributes of each gene in the six clusters represented on the right side, and the numbers in parentheses represent branches of corresponding attributes. Taking gymnosperm as an example, it can be subdivided into six branches, represented by Gymnosperm (1) to Gymnosperm (6) respectively. Detailed species source information of PAL, as well as sequences can be referred to Table S1 and Table S2 , respectively
Based on these 12 different evolutionary branches (charophyte, bryophyte, fern, gymnosperms, basal angiosperms, Ceratophyllales, Magnoliidae, basic taxa of eudicotyledon, early-diverging eudicotyledons, an early-diverging monocotyledon, a representative modern monocotyledon, and representative modern dicotyledons), we can roughly outline the differentiation profile of PAL genes among various taxonomic groups. The PAL genes of charophytes and bryophytes each form a monophyletic group, respectively. Ferns exhibit two major sequence divergences. Significant differentiation occurred in gymnosperm, resulting in five branches: two unique to gymnosperms, two shared by some primitive taxa and early-diverging angiosperm ancestors, and one clustering with modern taxa. Both basal angiosperms and Magnoliidae also show substantial differentiation. Most early-diverging angiosperms, including Epimedium , and ancestral monocotyledon taxa possess ancestral primitive group genes. Modern monocotyledons and dicotyledons, represented by O. sativa and A. thaliana , mostly form monophyletic groups, and most of them do not have ancestral group genes. Thus, the evolution of PAL genes has progressed from a monophyletic group in bryophyte with small-scale functional differentiation to large-scale sequence differentiation in gymnosperms, basal angiosperms, and Magnoliidae. Most early-diverging eudicotyledons and monocotyledons have different evolutionary histories, while modern angiosperm taxa tend to be monophyletic with few ancestral group genes.
Among the seven EpPALs identified in our study, two genes ( EpPAL2 and EpPAL3 ) cluster together with the ancestral type PAL genes represented by Cluster 3, supported by high bootstrap values. The remaining five genes ( EpPAL1 and EpPAL4 - 7 ) form a separate monophyletic group (Cluster 6) with equally strong bootstrap support (Fig. 1 ). These findings suggest that the PAL genes in Epimedium originated from at least two ancestral PAL homologous genes. The presence of EpPALs in different branches of the phylogenetic tree indicates their diverse evolutionary histories and potential functional diversification within the species.
Gene structure analysis of PAL genes
Statistical analysis of 167 PAL genes from 24 species primarily revealed three distinct intron insertion patterns. Pattern 1 (59/167) lacked introns, Pattern 2 (65/167) had a single intron with a shorter front-end exon (~ 400 bp) compared to the back-end (~ 1750 bp), and Pattern 3 (7/167) contained two introns with exon lengths of ~ 1140 bp, ~ 540 bp, and ~ 540 bp, respectively (Figure S1 ). Notably, in E. pubescens , intron lengths varied widely, from 422 bp in EpPAL5 to 2862 bp in EpPAL4 , while exon lengths were highly conserved. EpPAL2 and EpPAL3 followed Pattern 3, but differed in intron phase: EpPAL2 had two phase 0 introns, whereas EpPAL3 had one phase 2 and one phase 0 intron. Both genes had conserved glutamine codon (CAG) at the second exon/intron boundary. All other EpPALs exhibited Pattern 2, with conserved intron insertion sites between the second and third bases of specific codons: arginine (CGA) for EpPAL1 , EpPAL6 , and EpPAL7 , and isoleucine (AUU) for EpPAL4 and EpPAL5 (Fig. 2 b and Figure S1 ). These differences suggest independent origins for the intron insertion events in these two gene sets.
Using a fungal PAL gene from Neurospora tetrasperma (NCBI accession number: EGZ69514.1) as an outgroup, we determined the root position of the phylogenetic tree composed of EpPALs to infer their lineage. The research results support the division of EpPALs into two major clades, with Clade1 being further subdivided into two sub-clades (clade1_1 and clade1_2). Detailed homology detection and structural alignment among EpPALs were provided (Fig. 2 b and Table S3 ). Pairwise identity between EpPALs (excluding EpPAL2 and EpPAL3 ) ranged from 85.10 to 100%, indicating close relatedness. However, protein identity between Clade2 and Clade1 was lower, ranging from 61.09 to 64.38% (Fig. 2 a and Table S3 ), suggesting multiple ancestral origins for the formation of EpPALs . Phylogenetic analysis (Fig. 1 ), sequence alignment (Table S3 ) and gene structure (Fig. 2 b) collectively provide compelling evidence supporting the hypothesis that EpPAL2 and EpPAL3 trace their origins to a unique ancestral gene, whereas the other EpPALs descended from a different ancestral gene.
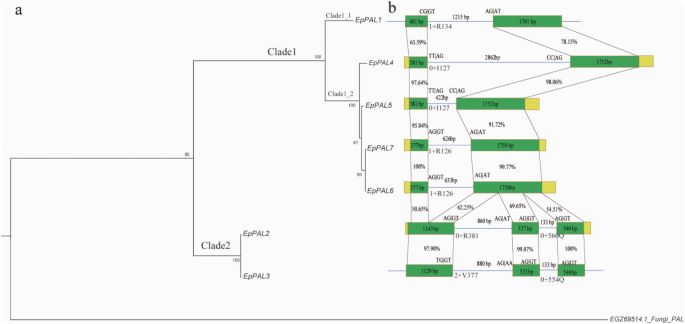
Phylogenetic tree and genomic structure of EpPALs . ( a ) Phylogenetic tree of EpPALs . The numbers below the branches represent the bootstrap values; ( b ) Gene structure of EpPALs . Green boxes, lines and yellow boxes represent the exon, intron and UTR, respectively. The percentages indicate the similarity of fragments between each pair of EpPALs . The exon/intron borders were displayed on top of the structural model, the intron phase (the numbers 0, 1 and 2, which represent introns between codons, introns between the first and second bases of a codon, introns between the second and the third bases of a codon, respectively), the amino acid residues affected by intron insertion events and its position were displayed under the structural model
Collinearity analysis of PALs
To determine the origin of EpPALs through duplication events, both inter- and intra-specific collinearity analyses were conducted (Fig. 3 ). We selected three species from different evolutionary branches ( A. trichopoda , N. nucifera , and O. sativa ) along with E. pubescens for microsynteny analysis of interspecies local regions related to PAL genes. Collinear blocks were detected for EpPAL1 to EpPAL6 , except for EpPAL7 . The analysis of EpPAL1 , EpPAL2 and EpPAL3 revealed collinear blocks only among E. pubescens , A. trichopoda , and N. nucifera , with no collinear block detected in O. sativa . Notably, homologous genes corresponding to EpPAL2 and EpPAL3 were not detected in A. trichopoda . Considering the detection of collinear blocks for EpPAL1 - 3 only in relatively primitive evolutionary branches and not in more modern species, it is speculated that the EpPAL1 - 3 may represent primitive types of the PAL family in E. pubescens . Further referencing Fig. 1 , we infer that the ancestral genes of EpPAL2 and EpPAL3 originated differently from that of EpPAL1 . The ancestral genes of EpPAL2 and EpPAL3 belong to Clade 3, a more primitive branch, while the ancestral gene of EpPAL1 belongs to Cluster 6, a branch present in modern monocots and dicots. For EpPAL4 - 6 , we detected relevant collinear blocks in species from three different evolutionary branches. Combining this with the evolutionary positions of these three genes in Fig. 1 , we infer that they may have originated from gene duplication of either the EpPAL1 branch or the EpPAL2 - 3 branch.
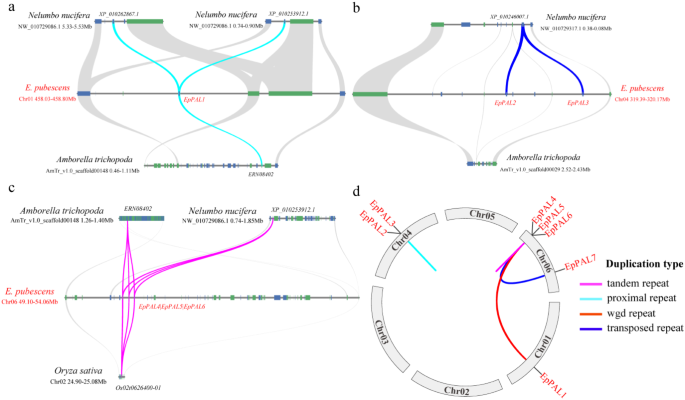
Inter- and intra-specific collinearity analysis of EpPALs . ( a ) Microsynteny analysis between the EpPAL1 loci and their respective collinear counterparts in A.trichopoda and N. nucifera . The collinear PAL genes and the syntenic flanking genes are connected by colored and gray lines, respectively; ( b ) Microsynteny analysis of EpPAL2 and EpPAL3 ; ( c ) Microsynteny analysis of EpPAL4 , EpPAL5 and EpPAL6 ; ( d ) Intraspecific collinearity analysis of EpPALs. Red line represents a collinear gene pair of EpPALs (the two ends were EpPAL1 and EpPAL4 , respectively). Purple line represents a tandem repeat between EpPAL4 and EpPAL5 - 6 . Cyan line represents a proximal repeat between EpPAL2 and EpPAL3 . Blue line represents a transposed repeat between EpPAL4 and EpPAL7
To gain a deeper understanding of the relationships among these genes, we conducted further intraspecific collinearity analysis in E. pubescens and analyzed gene duplication patterns using DupGen-finder (Fig. 3 d and Table S4 ). The results indicated that the gene pair EpPAL1 and EpPAL4 underwent segmental/whole-genome duplication (Fig. 3 d). EpPAL5 and EpPAL6 originated from tandem gene duplication of EpPAL4 , while EpPAL7 emerged from a transposed gene duplication event involving EpPAL4 (Fig. 3 d, Figure S2 and Table S4 ). Therefore, duplication events have played a significant role in the evolution of EpPALs . The evolutionary profile of EpPALs can be summarized as follows: the ancestral genes of E. pubescens are EpPAL2 and EpPAL3 , with EpPAL1 originating independently. EpPAL4 was then acquired through intraspecific whole-genome duplication from EpPAL1 . EpPAL4 underwent tandem duplication to produce EpPAL5 and EpPAL6 , and transposition duplication to generate EpPAL7 .
Conserved motif identification and cis- regulatory elements analysis
Eight conserved motifs followed a consistent distribution pattern of 6-4-7-3-8-1-2-5 among all EpPAL proteins (Figure S3 ). These motifs, ranging from 26 to 100 amino acids in length, were identified across all EpPALs (Figure S3 and Table S5 ). Notably, the MIO (4-methylidene-imidazolone-5-one) domain, characterized by the highly conserved signature sequence GTITASGDLVPLSYIA, contained the enzymatic active site Ala-Ser-Gly, which was preserved in all EpPAL proteins (Figure S3 ). With the exception of EpPAL3, which lacked the active site 158 L, the 388 F substrate-selective binding site, and the 350R phosphorylation site, both the active sites and substrate-specific binding sites were conserved across all EpPAL proteins (Figure S4 ). Additionally, while the 538 S phosphorylation site was serine in EpPAL2, EpPAL3 and EpPAL4, it was replaced by threonine in the remaining EpPAL proteins.
A total of 56 cis -regulatory elements (CREs) with known functions were identified, including 17, 19, 12, and 8 CREs related to light, stress, hormone, growth, and development responses, respectively (Fig. 4 ). With the exception of EpPAL4 , all EpPAL genes possess at least one AC element essential for lignin synthesis. However, no MBSI element related to the regulation of flavonoid biosynthetic genes was found. ARE (anaerobic induction), ABRE (abscisic acid-responsiveness), CGTCA-motif, and TGACG-motif (MeJA-responsiveness) were identified in all EpPAL genes, suggesting that responses to oxygen, abscisic acid, and methyl jasmonate are essential functions shared by all EpPALs . Additionally, ERE (ethylene-responsiveness) was exclusively present in EpPAL1 , while the CCGTCC-box was only found in EpPAL4 . These findings suggest that EpPAL1 and EpPAL4 may be specifically associated with ethylene response and activation of the meristem, respectively. Overall, these results imply that different EpPAL genes may have distinct yet overlapping biological functions, playing roles in processes such as growth and development, hormone response, and environmental stress response.
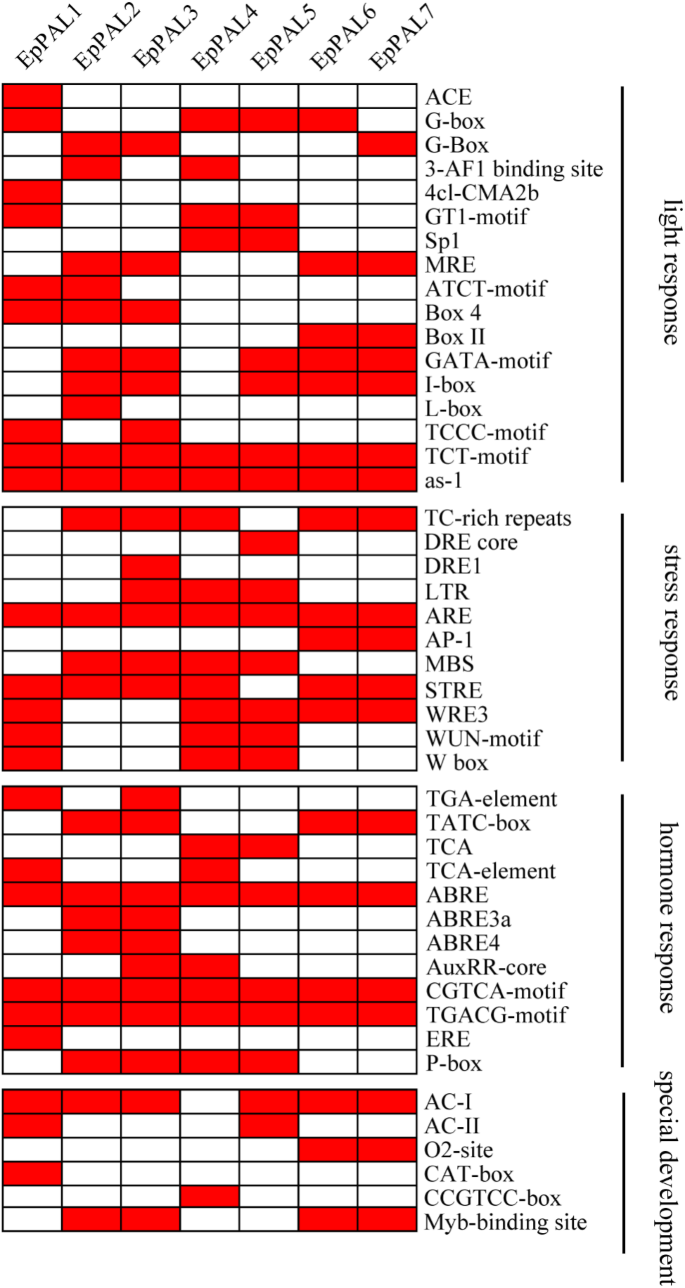
Cis -regulatory elements of EpPAL s in upstream region of 1500 bp. Four different types of cis -regulatory elements and different cis -regulatory elements of all EpPALs were provided
Natural selection analysis
Natural selection tests were conducted using PAML (v.4.1) under different hypotheses. For the branch-specific model, four hypotheses were tested as outlined in Table S6 . The three-ratio model, which assigns distinct ω values to each of the three clades (ω[Clade1_1] ≠ ω[Clade1_2] ≠ ω[Clade2]), emerged as a significantly better fit to the dataset compared to the other models tested (df = 1, P = 1.13e-07). Notably, the two-ratio model also outperformed the one-ratio model (df = 1, P = 2.62e-08), as illustrated in Fig. 2 a and detailed in Table S6 . These findings suggest that each clade experienced unique selection pressures, with ω ratios of 0.20, 0.037, and 0.0037 for Clade1_1, Clade1_2, and Clade2, respectively (Fig. 2 a and Table S6 ). Notably, Clade2 exhibited strong purifying selection, evolving approximately 54 and 10 times slower than Clade1_1 and Clade1_2, respectively. Clade1_2 followed in terms of purifying selection, while EpPAL1 , originating from Clade1_1, exhibited relatively higher divergence.
To further investigate whether ω varied across all amino acid sites or specific sites within particular branches, both the site-specific model and the branch-site model were employed. Under the site-specific model, three amino acid residues under positive selection when comparing selection M1 versus neutral M1, as well as M7 versus M8. Additionally, when setting Clade1_1, Clade1_2, and Clade2 as the foreground branches, 8, 7, and 76 amino acid sites were found under positive selection, respectively (Table S6 ). These candidate positively selected sites provide valuable insights into the evolutionary history of EpPALs .
Expression patterns of EpPALs and determination of EpPALs related to prenylated flavonol glycosides
The EpPALs were identified in five distinct tissues, as depicted in Figure S5 , with varying expression patterns across these tissues. EpPAL1 demonstrated high expression levels in all tested tissues, peaking in the leaf and flower, and gradually decreasing in the root, fruit, and stem, suggesting a fundamental role. In contrast, EpPAL2 and EpPAL3 showed elevated expression in leaf but were barely detectable in the stem, while EpPAL5 was predominantly expressed in leaf and root. EpPAL4 , EpPAL6 , and EpPAL7 had minimal or low levels of expression.
To further investigate the relationship between EpPAL expression and the contents of key bioactive compounds, a transcriptome analysis using samples from five different leaf development stages. The findings, along with the corresponding EpPAL expression levels and bioactive compound contents, are outlined in Table S7 . Additionally, a correlation analysis was performed, and the results are visually presented in Fig. 5 .
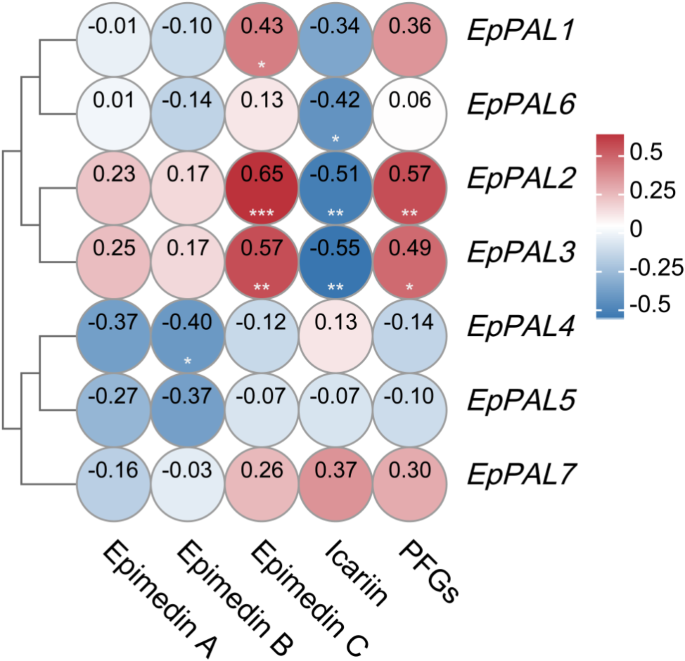
Correlation heatmap of EpPAL s with Epimedin A , Epimedin B , Epimedin C , Icariin and total PFGs. The significance levels were set as follows: unmarked stands for P > = 0.05, * stands for 0.01 < P < 0.05, ** stands for 0.001 < P < 0.01, *** stands for P < = 0.001
By integrating transcriptome data with targeted metabolite measurements, significant correlations were discovered between the expression of EpPAL2 and EpPAL3 with Epimedin C ( EpPAL2 : r = 0.65, P < 0.001; EpPAL3 : r = 0.57, P < 0.01) and total PFGs ( EpPAL2 : r = 0.57, P < 0.01; EpPAL3 : r = 0.49, P < 0.05). Notably, EpPAL1 holds the third position in terms of correlation strength. However, a notable negative correlation was observed between these genes and Icariin ( EpPAL2 : r = -0.51, P < 0.01; EpPAL3 : r = -0.55, P < 0.01). The remaining EpPALs demonstrated either weak ( EpPAL6 ) or no correlation ( EpPAL4 , EpPAL5 and EpPAL7 ), implying that EpPAL1 , EpPAL2 , and EpPAL3 , particularly the latter two, might have a pivotal role in the biosynthesis of PFGs.
Determination of EpPALs related to anthocyanin biosynthesis in flowers and leaves
To further identity the EpPAL isoforms responsible for anthocyanin biosynthesis, various groups of Epimedium species with distinct petal, sepal, and leaf colors were studied (Fig. 6 a). In leaves, EpPAL1 - 3 align with the observed color phenotypes, with EpPAL2 and EpPAL3 showing significantly higher expression in magenta leaves compared to green leaves, while EpPAL1 did not exhibit a notably high expression pattern (Fig. 6 b). Co-expression analysis with EpANSs and EpDFRs revealed a significant positive correlation between EpPAL1 - EpPAL3 and the expression of DFR and ANS genes, suggesting their involvement in anthocyanin biosynthesis in leaves, whereas EpPAL6 and EpPAL7 showed a significant negative correlation (Fig. 6 e and Table S9 ).
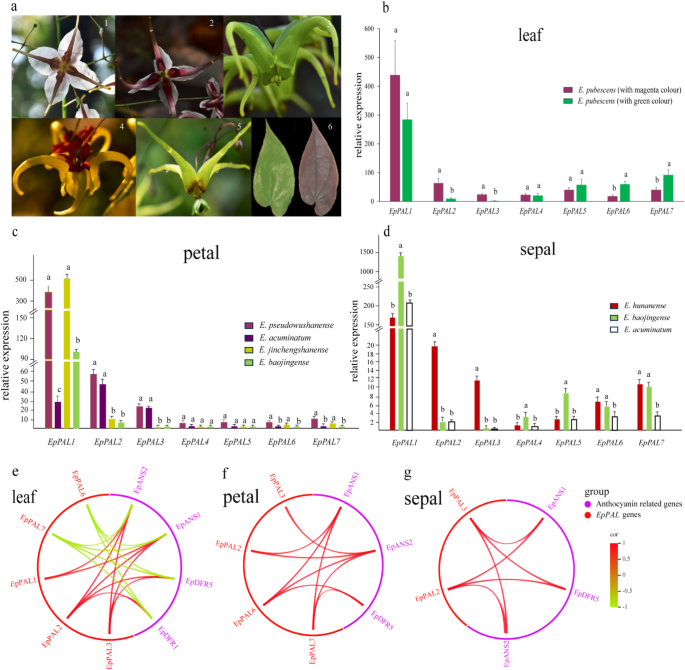
Phenotype of flower and leaf colors in different species of Epimedium plants, their PAL expression profiles, and the co-expression relationship with major anthocyanin-related genes. ( a ) Different colors in leaf, petal and sepal. a1 ~ a5 showed E. pseudowushanense , E. acuminatum , E. baojingense , E. hunanense and E. jinchengshanense , respectively. a6 showed leaf colors in E. pubescens with green and magenta, respectively. ( b ) Expression levels of EpPALs with different leaf colors. Significance tests for each EpPAL gene in two types of leaves were conducted and were labled with italicized ‘ a ’ and ‘ b ’ accordingly; ( c ) Expression levels of EpPALs in E. pseudowushanense , E. acuminatum , E. jinchengshanense and E. baojingense , with petal colors of magenta, magenta, yellow, and green, respectively. ( d ) Expression levels of EpPALs in E. hunanense , E. baojingense and E. acuminatum with sepal colors of red, green and white, respectively. Significance tests for each EpPAL gene in different colours of petals or sepals were conducted and were labeled with regular ‘a’ and ‘b’ accordingly. ( e-g ) Co-expression relationship between EpPALs and anthocyanin-related genes in leaf, petal and sepal, respectively. Three biological replicates were provided, and each repeat represent a mixing sample originated from three individuals
Similarly, in flowers, EpPAL2 and EpPAL3 showed significantly elevated expression in magenta petals compared to yellow petals and green petals (Fig. 6 c). Both genes exhibited similar expression patterns across different sepal colors (Fig. 6 d). Co-expression analysis in petals and sepals revealed a significant positive correlation between EpPAL2 , EpPAL3 , EpPAL6 and EpPAL7 with EpANSs and EpDFRs in petals (Fig. 6 f and g and Table S9 ), and between EpPAL2 and EpPAL3 with EpANSs and EpDFRs in sepals (Fig. 6 g and Table S9 ). Based on these expression profiles of EpPALs and co-expression patterns, we speculate that EpPAL2 and EpPAL3 may primarily be involved in anthocyanin synthesis in petals and sepals, while EpPAL6 and EpPAL7 may also play a role in anthocyanin synthesis in petals.
Repeatability verification of PFGs content dynamics and expression levels
To further validate the casual PAL genes involved in PFGs biosynthesis, a parallel validation experiment was conducted, comprising the determination of Epimedin C and total PFGs using the UPLC method (Fig. 7 c and Table S8 ), and the absolute quantification of seven EpPALs gene expressions across five developmental stages (S1 to S5) using the qRT-PCR method (Fig. 7 b). Six pairs of specific primers were designed for this purpose, with a single pair used for EpPAL6 and EpPAL7 due to their high sequence conservation (Table S10 ).
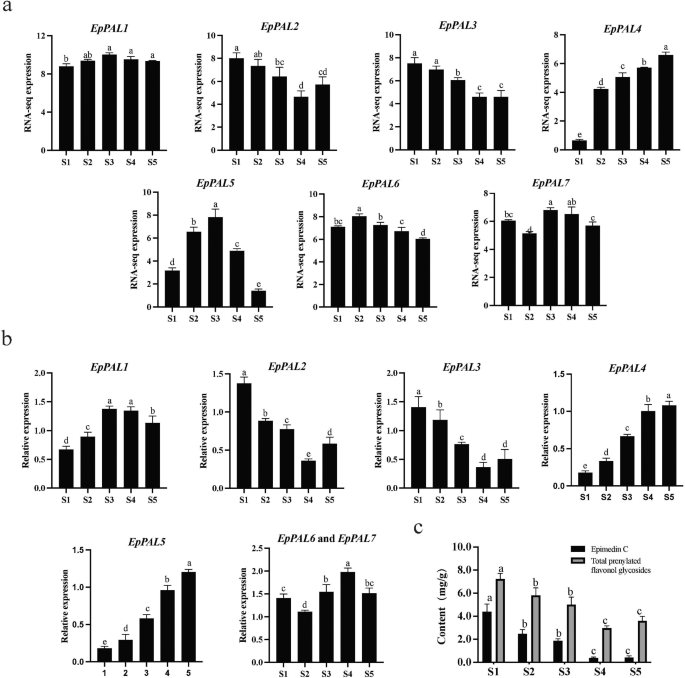
Expression patterns of EpPALs . ( a ) Expression patterns of EpPALs in S1-S5 by RNA-seq. The replicates can be referred to Table S7 . ( b ) Expression levels of EpPALs in S1-S5 by qRT-PCR. Three biological replicates were provided, and each repeat represent a mixing sample originated from three individuals. ( c ) The content of Epimedin C and total PFGs in S1-S5. S1-S5 represent the different stages of leaf development in E. pubescens . Error bars indicate standard error. The significance level is 0.05
The results revealed that EpPAL2 and EpPAL3 exhibited peak expression in S1, followed by a continuous decrease from S2 to S4, and a slight increase in S5 (Fig. 7 b), consistent with the relative quantification observed in transcriptome results (Fig. 7 a). A similar trend was observed for other EpPALs , except for EpPAL5 , indicating the overall reliability of the transcriptome data.
Importantly, the dynamic expression patterns of EpPAL2 and EpPAL3 across the five stages aligned with the dynamic changes in the content of Epimedin C and total PFGs (Fig. 7 ). However, no apparent correlation was found between the PFGs contents and qRT-PCR results for other EpPALs . For instance, EpPAL1 showed an initial increase (S1 to S3) followed by a decrease (S3 to S5), while EpPAL4 and EpPAL5 exhibited a continuous increasing trend. EpPAL6 and EpPAL7 fluctuated significantly, with the lowest expression in S2 and the highest in S4, respectively (Fig. 7 b), and did not align with the dynamic changes observed in Epimedin C and total PFGs content (Fig. 7 c).
Overall, our findings suggest that EpPAL2 and EpPAL3 may be the most critical genes responsible for the biosynthesis of PFGs in E. pubescens .
Characterization of the PAL gene family in E. pubescens
In the present study, the high-quality chromosome-level genome of E. pubescens served as a valuable resource for PAL research. A comprehensive exploration identified seven PAL genes ( EpPAL1 to EpPAL7 ), offering a more extensive analysis compared to previous studies in E. sagittatum [ 17 ]. All seven EpPALs were located in the cytoplasm, consistent with current PAL studies [ 8 , 9 , 12 , 19 ]. Despite the widely recognized conservation among EpPALs , two genes, EpPAL2 and EpPAL3 , exhibited lower identity (61.09–64.38%) to other EpPALs , reminiscent of ClPAL2 in Citrullus lanatus [ 20 ], which was proven to be an ancestral PAL, sharing only ~ 60% identity with other ClPALs despite having pairwise identities ranging from 71.2 to 99.0% [ 8 ]. Significant differences in PAL evolution with distinct origins were observed in E. pubescens , contributing to the varying sequence identities.
This study included a substantial proportion (20 out of 24) of primitive taxa which is different from previous studies [ 8 , 19 ]. This facilitated an investigation into intron insertion events in E. pubescens . While similar exon/intron structures tend to cluster together, as observed in E. sagittatum [ 17 ], pear [ 21 ], and common walnut [ 22 ], EpPAL2 and EpPAL3 gained two introns (Fig. 2 b and Figure S1 ), whereas other EpPALs possess only one intron insertion [ 8 , 9 , 11 , 12 , 17 , 23 , 24 ], suggesting significant structural divergence within the PAL gene family in E. pubescens .
Differences in intron/exon structures between EpPAL2-3 and other angiosperms were also detected (Figure S1 ), further indicating their ancient with distinct evolutionary origins. The clustering of the ancient gene NnPAL1 ( XP_010246007.1 in this study) with EpPAL2 and EpPAL3 supports this conclusion [ 19 ]. The increased intron number in ancient EpPAL genes may be significant, as intron-gain events can enhance mRNA stability or harbor regulatory elements without disrupting the coding frame of genes [ 25 , 26 , 27 , 28 ]. However, intron insertions in EpPAL2 , EpPAL3 and other EpPALs may have occurred independently based on their phylogenetic positions (Fig. 1 ).
Evolution of the plant PAL gene family
Previous studies have primarily focused on research at the species or family level [ 20 , 21 , 23 , 24 , 29 ], with limited investigations on the evolution of PAL genes, except for those in N. nucifera [ 19 ] and cucurbit species [ 8 ]. To bridge this gap, we conducted a phylogenomic study to elucidate the evolution of plant PAL (Fig. 1 ). Our findings reveal a large-scale gene duplication event in gymnosperms, with two gymnosperm-specific branches (cluster2 and cluster 4), emphasizing their unique origin. The presence of PALs in gymnosperms across other branches (cluster3, cluster5 and cluster6) suggests ancestral gene duplication and vertical inheritance during evolution, supporting widespread differentiation [ 19 ]. We hypothesize that the multigene families of PAL in gymnosperms may confer diverse functions, such as producing additional trans-cinnamic acid for downstream metabolic pathways or enhancing lignin biosynthesis to defend against adverse environments like insect and pathogen attacks [ 25 ], contributing to their widespread habitat adaptation.
Furthermore, our results indicate that the origin of PAL in angiosperm plants may not be monophyletic. This is evident from the evolutionary tree, where PAL genes of dicots like cucumber and lotus are clustered within cluster6 and also share clustering with PAL genes of primitive types within cluster3 and cluster5 (Fig. 1 ). This finding validates previous conclusions in N. nucifera [ 19 ]. Except for A. coerulea (only in cluster6), the PALs of other early-diverging eudicotyledons including E. pubescens (in cluster3 and 6), P. somniferum (in cluster5 and 6), M. integrifolia (in cluster3 and 6), K. uniflora (in cluster5 and 6), N. nucifera (in cluster3, 5 and 6), and T. sinense (cluster5 and 6) are divided into two clusters or three clusters ( N. nucifera ), with one clustering with cluster3 or cluster5, speculated to be relatively ancient genes, and the other with PALs of modern dicots (cluster6). This suggests different evolutionary origins may underlie the PAL gene evolution in early-diverging dicots [ 19 ]. Additionally, we speculate that ancient genes like EpPAL2 and EpPAL3 may have played a crucial role in the survival of these “pioneer species” in harsh environments during evolution, highlighting their importance for related plants. For instance, in E. pubescens , the positive selection test indicates that EpPAL2 and EpPAL3 experienced the strictest selection pressure, evolving ~ 10 and ~ 54 times slower than genes of Clade 1_2 ( EpPAL4 ~ 7 ) and Clade 1_1 ( EpPAL1 ), respectively (Table S6 ).
Expression profiles of EpPALs
Duplicate genes can lead to pseudogenization, subfunctionalization and neofunctionalization [ 2 ]. Gene expression patterns offer valuable insights into the functional differentiation. Previous research has shown that AtPAL1 and AtPAL2 in A. thaliana are highly expressed in roots and involved in stress-induced flavonoid biosynthesis [ 30 , 31 ]. In Pyrus bretschneideri , PbPAL1 and PbPAL2 are predominantly expressed in stems and roots, suggesting involvement in lignin synthesis and stone cell development [ 21 ].
In this study, the expression levels of EpPAL2 and EpPAL3 gradually decreased in correlation with the PFGs content during leaf development, indicating a potential significant role in PFGs biosynthesis. Our findings support the speculation that both genes positively correlate with Epimedin C and total PFGs content (Figs. 5 and 7 ). EpPAL1 may also be involved in PFGs biosynthesis in leaves, as evidenced by the third-highest correlation with Epimedin C and total PFGs (Fig. 5 ). As an ancestral gene with an independent origin, EpPAL1 is constitutively highly expressed across tissues, suggesting divergent functionality. Similar expression patterns of PAL can be observed in Cuminum cyminum and cucurbit plants [ 8 , 32 ]. Given the pivotal role of PAL in both primary and secondary metabolism, constitutive multifunctional expression may be crucial for maintaining diverse biological processes and adapting to changing environments.
In E. sagittatum , the expression patterns of EsPAL1 , EsPAL2 , and EsPAL3 align with lignification and active components accumulation [ 17 ]. In our study, EpPALs exhibited distinct yet overlapping expression patterns during different stages of leaf development (Fig. 5 and Table S7 ), hinting at possible functional diversification and redundancy. EpPAL2 and EpPAL3 demonstrated overlapping expression in PFGs and anthocyanin biosynthesis, ensuring functional redundancy, preventing the complete loss of weaker gene copies, a phenomenon often observed in key genes involved in different metabolic pathways [ 33 , 34 ]. In contrast, EpPAL1 and other EpPALs displayed distinct expression patterns, potentially stemming from functional differentiation among duplicate EpPAL genes, ultimately leading to their subfunctionalization and neofunctionalization.
In summary, this study comprehensively investigated PAL genes in E. pubescens . We speculate that EpPAL2 and EpPAL3 may participate in PFGs and anthocyanin pathways in leaves and flowers, while EpPAL1 , characterized by its constitutively high expression, may not only be involved in the biosynthesis of PFGs and anthocyanins in leaves, but also play a significant role in defense and protection. EpPAL6 and EpPAL7 may participate in anthocyanin synthesis in petals, but there is no evidence of their involvement in PFGs biosynthesis. Given the low expression levels, we hypothesize that EpPAL4 to EpPAL7 may function as stress responders or have become nonfunctional following duplication events. Further experimental validation is needed to confirm these speculations.
Materials and methods
Experimental materials.
The experimental materials were sourced from the Epimedium Germplasm Resources Nursery located in Xiuwen County, Guizhou Province. The plant species used in our studies are authenticated by Professor Baolin Guo. Specially, the plant selected for genome sequencing, as well as RNA-seq analysis across different tissues and leaf developmental stages, qRT-PCR and UPLC experimentation, was confirmed as E. pubescens . Additionally, Professor Baolin Guo identified the plants utilized in RNA-seq investigations of various flower hues, including E. pseudowushanense , E. acuminatum , E. jinchengshanense , E. baojingense and E. hunanense . Voucher specimens for all plant samples are preserved at the Plant Specimen Museum, part of the Institute of Medicinal Plant Resources Development, Chinese Academy of Medical Sciences (coded as IMD). The deposition numbers assigned to E. pubescens , E. pseudowushanense , E. acuminatum , E. jinchengshanense , E. baojingense and E. hunanense were B. L. Guo 0711-3, B. L. Guo 0312, B. L. Guo 0342, B. L. Guo 0524, B. L. Guo 0332 and B. L. Guo 0402, respectively.
PAL gene identification and sequence analysis
The g enome sequences of E. pubescens were accessed from the National Center for Biotechnology Information (NCBI) under project PRJNA747870 [ 35 ]. Utilizing APG IV [ 36 ] as a reference, genome sequences of 23 species were located and retrieved from Phytozome ( http://www.phytozome.net ) and the NCBI database ( https://www.ncbi.nlm.nih.gov/ ) (Table S1 and Table S2 ). The analyzed species are as follows: one algae ( Chara braunii ), one bryophyte ( Physcomitrella patens ), one fern ( Ceratopteris richardii ), two gymnosperms ( Ginkgo biloba and Sequoiadendron giganteum ), two basal angiosperms ( Amborella trichopoda and Nymphaea colorata ), four Magnoliidae ( Ceratophyllum demersum , Cinnamomum kanehirae , Liriodendron chinense , Piper nigrum ), a basal monocotyledon ( Spirodela polyrrhiza ), six early-diverging eudicotyledons ( Nelumbo nucifera , Macadamia integrifolia , Tetracentron sinense , Aquilegia coerulea , Kingdonia uniflora , Papaver somniferum , E. pubescens ), three typical dicotyledons ( Vitis vinifera , Cucumis sativus , Arabidopsis thaliana ), an early-diverging monocotyledons ( Brachypodium distachyon ) and a typical monocotyledon ( Oryza sativa ). Predicted proteins from these genomes underwent screening with HMMER v3 [ 37 ], employing the Hidden Markov Model (HMM) corresponding to Pfam [ 38 ] (PF00221; http://pfam.sanger.ac.uk/ ). Among the proteins identified using the PAL HMM, a subset of high-quality proteins (E-value < 1e-20 and confirmed integrity of the PAL domain) was selected for alignment. In cases where only genome information was available for certain species, a localized TBLASTN search was conducted against the PAL genes of A. thaliana and O. sativa , considering records with maximum identity > 95%, length > 400 bp, and E-value < 1e-20. To validate the results of the HMM and BLAST searches, all potential PAL genes were further subjected to analysis in the NCBI-CDD database ( https://www.ncbi.nlm.nih.gov/cdd/ ) to confirm the presence of conserved domains, and candidates lacking the “PAL-HAL” shorthand designation were discarded. Protein sequences were excluded if the PAL domain appeared truncated or if the PAL domain match E-value exceeded 1e-5. Following these stringent criteria, 167 PAL genes were ultimately identified across the nine species studied (Table S2 ). Sequences of the 7 EpPALs have been submitted to China National Center for Bioinformation (CNCB), with the accession numbers for EpPAL1 - EpPAL7 are C_AA071439.1, C_AA071440.1, C_AA071441.1, C_AA071442.1, C_AA071443.1, C_AA071444.1 and C_AA071445.1, respectively. The accession number of Ep-actin gene used in qPCR is C_AA071459.1.
Protein sequence properties analysis, conserved domain and motifs analysis
The physiological and biochemical characteristics of the full-length proteins were determined using the ProtParam tool ( http://web.expasy.org/protparam/ ) [ 39 ]. SignalP (V.4.1) ( http://www.cbs.dtu.dk/services/SignalP/ ) [ 40 ] and Euk-mPLoc (V.2.0) ( http://www.csbio.sjtu.edu.cn/bioinf/euk-multi-2/# ) [ 41 ] were utilized to analyze the signal peptide and subcellular localization of each protein, respectively. Additionally, MEME (V.5.0.2) ( http://meme-suite.org/ ) [ 42 ] was employed to identify conserved motifs, including the PAL domain, using optimized parameters: a maximum of 10 motifs were searched for, with each motif ranging from 6 to 50 residues in width.
Phylogenetic analysis, synteny block identification and gene duplication pattern analysis
The protein sequences were aligned using ClustalW2 [ 43 ] with its default settings. Phylogenetic trees were inferred using the maximum likelihood (ML) method with the JTT + R9 model, which was automatic selected by IQ-TREE [ 44 ]. The evolutionary tree was then visualized and further refined using iTOL ( https://itol.embl.de/ ) [ 45 ]. Synteny blocks between genomes and intra-specific collinearity analysis were identified using the jcvi pipeline ( https://github.com/tanghaibao/jcvi ). BLASTP was performed to identify paralogous or orthologous gene pairs, with an E-value cutoff of 1e-05. To identify patterns of gene duplication, DupGen-finder ( https://github.com/qiao-xin/DupGen_finder ) [ 46 ] was employed.
Chromosome location, cis -acting element and gene structure analysis
The gene location map was constructed using MapChart V.2.0 ( http://mg2c.iask.in/mg2c_v2.0/ ) [ 47 ]. Cis -acting elements located within the 1.5 kb upstream sequences of the 5′ regulatory region, starting from the transcriptional start site, were identified using PlantCARE ( http://bioinformatics.psb.ugent.be/webtools/plantcare/html/ ). To assess the divergence between upstream sequences of each paralogous gene pair, the GATA program [ 48 ] was employed with a window size of seven and a lower cut-off score of 12 bits. Lastly, the visualization of gene structure was facilitated by TBtools software [ 49 ].
Natural selection test
Codeml program in PAML (V.4.8) [ 50 ] was conducted to detect changes in evolutionary rates and signatures of positive selection. Four levels of positive selection tests were performed. (1) Detection of positive selection in pairwise genes of all EpPALs . For this, the main parameter settings were: runmode = -2 and NSsites = 0; (2) Site-specific model was applied for positive selection detection of sites in genes. This model assumes a constant ω (ω = dN/dS; where dN is the non-synonymous substitution rate and dS is the synonymous substitution rate) across all branches. The main parameters were set as runmode = 0 and NSites = 0 1 2 7 8. To determine the most suitable model for detection, we compared Neutral M1 vs. Selection M1 and M7 vs. M8; (3) Branch model was applied to detect the rapidly evolving genes in the target branch. This model assumes a constant ω for all sites with a gene. Three scenarios were tested: the one-ratio model (assuming a constant ω for all branches with parameters as model = 0 and NSites = 0), the two-ratio model (assuming a foreground ω for designated branches and a background ω for all others with parameters as model = 2 and Nsites = 0), and the free-ratio model (allowing different ω for each branch with parameters as model = 1 and Nsites = 0) [ 51 ]. Models were compared using likelihood ratio tests based on the log likelihood (lnL). The chi-square test with a significance threshold of P < 0.05 was used to compare 2|ΔlnL| values between models; (4) Branch-site model was applied to detect whether there exist positive selection sites in a specific branch. This model assumes one ω for the target branch and another constant ω for all other branches. We compared the branch-site model A (model = 2, NSites = 2, fix_omega = 0, omega = 2) with its null model (model = 2, NSites = 2, fix_omega = 1, omega = 1). If the chi-square test yielded a significance of P < 0.05, we employed the Bayes Empirical Bayes (BEB) method to calculate the posterior probability. Genes in the specific branch were considered under positive selection if this value exceeded 0.95 [ 52 ].
RNA-seq and correlation analysis
To identify the gene expression profiles of EpPALs , we conducted three independent RNA-seq experiments. Firstly, we sampled different tissues (roots, stems, leaves, flowers, and fruits) from E. pubescens . Secondly, we collected leaves from various developmental stages of E. pubescens , specifically: Stage 1 (S1) with leaf width of 0.5–1 cm and low leatheriness; Stage 2 (S2) with leaf width of 1.5–2 cm and low leatheriness; Stage 3 (S3) with leaf width of 2.5 ~ 4 cm and low leatheriness; Stage 4 (S4) with leaf width of 5 cm and medium leatheriness; and Stage 5 (S5) with leaf width of 5 cm and high leatheriness. Thirdly, we included six species of Epimedium with diverse petal colors (magenta in E. pseudowushanense and E. acuminatum , yellow in E. jinchengshanense , and green in E. baojingense ), sepal colors (red in E. hunanense , green in E. baojingense , and white in E. acuminatum ), and leaf colors (green and magenta in E. pubescens ). The RNA-seq protocol and classification criteria followed Xu et al. (2023) [ 53 ]. Initial protein contamination screening was performed using the NanoDrop ND 1000 (Nanodrop technologies), ensuring a tightly controlled OD260/OD280 ratio within the range of 1.9 to 2.1. Subsequently, the RNA Integrity Number (RIN) was evaluated using the Agilent Technologies 2100 bioanalyzer (Agilent, Santa Clara, CA). Sequencing was only initiated if the RIN exceeded 8 and the 28 S/18S ratio was greater than or equal to 0.7. Software tools including Trimmomatic (version 0.36) [ 54 ], HISAT2 [ 55 ], and the R package Rsubread [ 56 ] were utilized for quality control, sequence alignments, and gene expression quantification, respectively. The reference genome utilized was that of E. pubescens , as published by Shen et al. (2022) [ 35 ]. All samples were collected between 10:00–11:30 am on a sunny day and immediately treated with liquid nitrogen before being stored in dry ice for transport to Beijing. All samples were conserved at -80 °C under ultralow temperature for subsequent RNA extraction and chemical component identification. We used the R packages Tidyverse and ggcor to compute the pearson correlation between PALs and the relative content of PFGs.
UPLC experiment
For the analysis of PFGs content, approximately 0.1 g of ground sample was soaked in 10 ml of 50% ethanol and ultrasonicated for 30 min before being filtrated through 0.22 μm filter membrane (Millipore, Nylon) for UPLC analysis. UPLC under 270 nm was conducted at a flow rate of 0.3 ml/min using the ACQUITY UPLC system (UPLC I-class; Waters, Milford, MA, USA) equipped with an ACQUITY UPLC BEH C18 column (2.1 × 100 mm. 1.7 μm; Waters, Milford, MA, USA) maintained at 25 °C. The mobile phase comprised of water (eluent A) and 100% acetonitrile (eluent B). The authentic flavonoids were purchased from the Shanghai Yuanye Bio-Technology Co., Ltd., Shanghai, China.
To ensure the reliability of our transcriptome data, we conducted qRT-PCR analysis on leaves from five distinct developmental stages of E. pubescens , focusing on six selected EpPALs . Total RNA was extracted using a plant total RNA extraction kit from Aidlab (China). We assessed RNA integrity on a 1.2% agarose gel and quantified it using a NanoDrop 2000 C Spectrophotometer from Thermo Scientific (USA). cDNA synthesis was achieved using the TransScript One-Step gDNA Removal and cDNA Synthesis SuperMix Kit from Transgen Biotech (China). qRT-PCR reactions were performed for each tissue sample with gene-specific primers (Table S10 ). The qRT-PCR program consisted of pre-denaturation at 95 °C for 2 min, followed by 40 cycles of amplification at 95 °C for 15 s, 60 °C for 30 s, and 72 °C for 30 s. We analyzed the relative abundance of transcripts using the comparative Ct method, applying the formula 2-ΔΔCt for relative quantification. Our gene expression results were calculated based on the 2-ΔΔCt method, and the reported data represents the average of three biological and three technical replicates.
7 PALs were firstly and comprehensively identified based on the genome of E. pubescens . EpPAL2 , EpPAL3 and EpPAL1 were identified as the ancient isoforms. EpPAL2 and EpPAL3 exhibited a homology range of 61.09 to 64.38%, contained two introns and underwent strong purifying selection, evolving at a rate ~ 10 to ~ 54 times slower compared to EpPAL1 and modern EpPALs ( EpPAL4-7 ). The evolutionary trajectory of modern EpPALs was shaped by multiple duplication events. Initially, EpPAL4 emerged through intraspecific whole-genome duplication from EpPAL1 . This was followed by a sequence of tandem duplications resulting in EpPAL5 and EpPAL6 , and transposed duplications that gave rise to EpPAL7 , all originating from EpPAL4 . Analysis of expression profiles through RNA-seq and UPLC techniques revealed that EpPAL2 and EpPAL3 are key genes involved in the biosynthesis of prenylated flavonol glycosides. This finding was further validated through parallel UPLC and qRT-PCR experiments. Novel insights into the evolution of 24 PAL gene families were provided, revealing the evolutionary characteristics of 12 different evolutionary clade groups. Overall, this study offers a unique perspective on PAL evolution and clarifies the role of PAL genes in Epimedium plants.
Data availability
The experimental materials were stored at the Institute of Medicinal Plant Development, Chinese Academy of Medical Sciences. The datasets containing the E. pubescens genome sequences can be accessed from the National Center for Biotechnology Information (NCBI) repository using the accession number PRJNA747870. Additionally, the RNA-seq datasets generated in this study have been deposited in the China National Center for Bioinformation (CNCB) repository. Specifically, the RNA-seq data related to different tissues of E. pubescens, various developmental stages of E. pubescens leaves, and six species of Epimedium with distinct petal colors can be retrieved using the accession numbers CRA014527, CRA014549, and CRA014550, respectively.
Naoumkina M, Zhao Q, Gallego-Giraldo L, Dai X, Zhao PX, Dixon R. Genome-wide analysis of phenylpropanoid defence pathways. Mol Plant Pathol. 2010;11(6):829–46.
Article CAS PubMed PubMed Central Google Scholar
Barros J, Dixon RA. Plant phenylalanine/tyrosine ammonia-lyases. Trends Plant Sci. 2020;25(1):66–79.
Article CAS PubMed Google Scholar
Bennici A. Origin and early evolution of land plants: problems and considerations. Commun Integr Biol. 2008;1(2):212–8.
Article PubMed PubMed Central Google Scholar
Shalaby S, Horwitz BA. Plant phenolic compounds and oxidative stress: integrated signals in fungal-plant interactions. Curr Genet. 2015;61(3):347–57.
Liu CW, Murray JD. The role of flavonoids in nodulation host-range specificity: an update. Plants (Basel). 2016;5(3):33.
MacDonald MJ, D’Cunha GB. A modern view of phenylalanine ammonia lyase. Biochem Cell Biol. 2007;85(3):273–82.
Schwede TF, Rétey J, Schulz GE. Crystal structure of histidine ammonia-lyase revealing a novel polypeptide modification as the catalytic electrophile. Biochemistry. 1999;38(17):5355–61.
Dong C, Cao N, Zhang Z, Shang Q. Phenylalanine ammonia-lyase gene families in cucurbit species: structure, evolution, and expression. J Integr Agric. 2016;15(6):1239–55.
Article CAS Google Scholar
Chang A, Lim MH, Lee SW, Robb EJ, Nazar RN. Tomato phenylalanine ammonia-lyase gene family, highly redundant but strongly underutilized. J Biol Chem. 2008;283(48):33591–601.
Bate NJ, Orr J, Ni W, Meromi A, Nadler-Hassar T, Doerner PW, et al. Quantitative relationship between phenylalanine ammonia-lyase levels and phenylpropanoid accumulation in transgenic tobacco identifies a rate-determining step in natural product synthesis. J Clin Periodontol. 1994;91(16):7608–12.
CAS Google Scholar
Huang J, Gu M, Lai Z, Fan B, Shi K, Zhou YH, et al. Functional analysis of the arabidopsis PAL gene family in plant growth, development, and response to environmental stress. Plant Physiol. 2010;153(4):1526–38.
de Jong F, Hanley SJ, Beale MH, Karp A. Characterisation of the willow phenylalanine ammonia-lyase (PAL) gene family reveals expression differences compared with poplar. Phytochemistry. 2015;117:90–7.
Ma H, He X, Yang Y, Li M, Hao D, Jia Z. The genus Epimedium : an ethnopharmacological and phytochemical review. J Ethnopharmacol. 2011;134(3):519–41.
Jiang J, Zhao Bj, Song J, Jia X. Pharmacology and clinical application of plants in Epimedium L. Chin Herb Med. 2016;8(1):12–23.
Zhu Jf L, Zj Z, Gs, Meng K, Kuang Wy, Li J, et al. Icaritin shows potent anti-leukemia activity on chronic myeloid leukemia in vitro and in vivo by regulating MAPK/ERK/JNK and JAK2/STAT3 /AKT signalings. PLoS ONE. 2011;6(8):e23720.
Zhao H, Guo Y, Li S, Han R, Ying J, Zhu H, et al. A novel anti-cancer agent Icaritin suppresses hepatocellular carcinoma initiation and malignant growth through the IL-6/Jak2/Stat3 pathway. Oncotarget. 2015;6(31):31927–43.
Zeng S, Liu Y, Zou C, Huang W, Wang Y. Cloning and characterization of phenylalanine ammonia-lyase in medicinal Epimedium species. Plant Cell Tissue Organ Cult. 2013;113(2):257–67.
Liu Y, Wu L, Deng Z, Yu Y. Two putative parallel pathways for naringenin biosynthesis in Epimedium wushanense . RSC Adv. 2021;11(23):13919–27.
Wu Z, Gui S, Wang S, Ding Y. Molecular evolution and functional characterisation of an ancient phenylalanine ammonia-lyase gene (NnPAL1) from Nelumbo nucifera : novel insight into the evolution of the PAL family in angiosperms. BMC Evol Biol. 2014;14:100.
Dong CJ, Shang QM. Genome-wide characterization of phenylalanine ammonia-lyase gene family in watermelon ( Citrullus lanatus ). Planta. 2013;238(1):35–49.
Li G, Wang H, Cheng X, Su X, Zhao Y, Jiang T, et al. Comparative genomic analysis of the PAL genes in five Rosaceae species and functional identification of Chinese white pear. PeerJ. 2019;7:e8064.
Yan F, Li H, Zhao P. Genome-wide identification and transcriptional expression of the PAL gene family in common walnut ( Juglans Regia L). Genes. 2019;10(1):46.
Hou X, Shao F, Ma Y, Lu S. The phenylalanine ammonia-lyase gene family in Salvia miltiorrhiza : genome-wide characterization, molecular cloning and expression analysis. Mol Biol Rep. 2013;40(7):4301–10.
Thiyagarajan K, Vitali F, Tolaini V, Galeffi P, Cantale C, Vikram P, et al. Genomic characterization of phenylalanine ammonia lyase gene in buckwheat. PLoS ONE. 2016;11(3):e0151187.
Bagal UR, Leebens-Mack JH, Lorenz WW, Dean JFD. The phenylalanine ammonia lyase (PAL) gene family shows a gymnosperm-specific lineage. BMC Genomics. 2012;13(3):S1.
Duret L. Why do genes have introns? Recombination might add a new piece to the puzzle. Trends Genet. 2001;17(4):172–5.
Wang HF, Feng L, Niu DK. Relationship between mRNA stability and intron presence. Biochem Biophys Res Commun. 2007;354(1):203–8.
Vogt T. Phenylpropanoid biosynthesis. Mol Plant. 2010;3(1):2–20.
Hu GS, Jia JM, Hur YJ, Chung YS, Lee JH, Yun DJ, et al. Molecular characterization of phenylalanine ammonia lyase gene from Cistanche deserticola . Mol Biol Rep. 2011;38(6):3741–50.
Wanner LA, Li G, Ware D, Somssich IE, Davis KR. The phenylalanine ammonia-lyase gene family in Arabidopsis thaliana . Plant Mol Biol. 1995;27(2):327–38.
Olsen KM, Lea US, Slimestad R, Verheul M, Lillo C. Differential expression of four Arabidopsis PAL genes; PAL1 and PAL2 have functional specialization in abiotic environmental-triggered flavonoid synthesis. J Plant Physiol. 2008;165(14):1491–9.
Habibollahi M, Kavousi HR, Lohrasbi-Nejad A, Rahpeyma SA. Cloning, characterization and expression of a phenylalanine ammonia-lyase gene ( CcPAL ) from cumin ( Cuminum cyminum L). J Appl Res Med Aromat Plants. 2020;18:100253.
Google Scholar
Duarte JM, Cui L, Wall PK, Zhang Q, Zhang X, Leebens-Mack J, et al. Expression pattern shifts following duplication indicative of subfunctionalization and neofunctionalization in regulatory genes of Arabidopsis . Mol Biol Evol. 2006;23(2):469–78.
Lei L, Zhou SL, Ma H, Zhang LS. Expansion and diversification of the SET domain gene family following whole-genome duplications in Populus trichocarpa . BMC Evol Biol. 2012;12:51.
Shen G, Luo Y, Yao Y, Meng G, Zhang Y, Wang Y, et al. The discovery of a key prenyltransferase gene assisted by a chromosome-level Epimedium pubescens genome. Front Plant Sci. 2022;13:1034943.
Group TAP, Chase MW, Christenhusz MJM, Fay MF, Byng JW, Judd WS, et al. An update of the angiosperm phylogeny group classification for the orders and families of flowering plants: APG IV. Bot J Linn Soc. 2016;181(1):1–20.
Article Google Scholar
Finn RD, Clements J, Eddy SR. HMMER web server: interactive sequence similarity searching. Nucleic Acids Res. 2011; 39(Web Server issue):W29–37.
Finn RD, Bateman A, Clements J, Coggill P, Eberhardt RY, Eddy SR, et al. Pfam: the protein families database. Nucleic Acids Res. 2014;42(Database issue):D222–230.
Wang L, Wang L, Zhang Z, Ma M, Wang R, Qian M, et al. Genome-wide identification and comparative analysis of the superoxide dismutase gene family in pear and their functions during fruit ripening. Postharvest Biol Technol. 2018;143:68–77.
Petersen TN, Brunak S, von Heijne G, Nielsen H. SignalP 4.0: discriminating signal peptides from transmembrane regions. Nat Methods. 2011;8(10):785–6.
Chou KC, Shen HB. A new method for predicting the subcellular localization of eukaryotic proteins with both single and multiple sites: Euk-mPLoc 2.0. PLoS ONE. 2010;5(4):e9931.
Bailey TL, Johnson J, Grant CE, Noble WS. The MEME suite. Nucleic Acids Res. 2015;43(W1):W39–49.
Larkin MA, Blackshields G, Brown NP, Chenna R, McGettigan PA, McWilliam H, et al. Clustal W and Clustal X version 2.0. Bioinformatics. 2007;23(21):2947–8.
Minh BQ, Schmidt HA, Chernomor O, Schrempf D, Woodhams MD, von Haeseler A, et al. IQ-TREE 2: new models and efficient methods for phylogenetic inference in the genomic era. Mol Biol Evol. 2020;37(5):1530–4.
Letunic I, Bork P. Interactive tree of life (iTOL) v4: recent updates and new developments. Nucleic Acids Res. 2019;47(W1):W256–9.
Qiao X, Li Q, Yin H, Qi K, Li L, Wang R, et al. Gene duplication and evolution in recurring polyploidization-diploidization cycles in plants. Genome Biol. 2019;20(1):38.
Voorrips RE. MapChart: software for the graphical presentation of linkage maps and QTLs. J Heredity. 2002;93(1):77–8.
Nix D, Eisen M. GATA: a graphic alignment tool for comparative sequence analysis. BMC Bioinformatics. 2005;6:9.
Chen C, Chen H, Zhang Y, Thomas HR, Frank MH, He Y, et al. TBtools: an integrative toolkit developed for interactive analyses of big biological data. Mol Plant. 2020;13(8):1194–202.
Yang Z. PAML 4: phylogenetic analysis by maximum likelihood. Mol Biol Evol. 2007;24(8):1586–91.
Yang Z. Likelihood ratio tests for detecting positive selection and application to primate lysozyme evolution. Mol Biol Evol. 1998;15(5):568–73.
Yang Z, Nielsen R. Codon-substitution models for detecting molecular adaptation at individual sites along specific lineages. Mol Biol Evol. 2002;19(6):908–17.
Xu C, Liu X, Shen G, Fan X, Zhang Y, Sun C, et al. Time-series transcriptome provides insights into the gene regulation network involved in the icariin-flavonoid metabolism during the leaf development of Epimedium pubescens . Front Plant Sci. 2023;14:1183481.
Anthony M, Marc L, Bjoern U. Trimmomatic: a flexible trimmer for illumina sequence data. Bioinformatics. 2014;30(15):2114–20.
Kim D, Langmead B, Salzberg SL. HISAT: a fast spliced aligner with low memory requirements. Nat Methods. 2015;12:357–60.
Liao Y, Smyth GK, Shi W. The r package rsubread is easier, faster, cheaper and better for alignment and quantification of RNA sequencing reads. Nuc Acids Res. 2019;47(8):e47.
Download references
Acknowledgements
We thank all our colleagues for providing useful discussions and technical assistance. We are very grateful to the editor and reviewers for critically evaluating the manuscript and providing constructive comments for its improvement.
This work was financially supported by the CAMS Innovation Fund for Medical Sciences (CIFMS) (2021-I2M-1-031).
Author information
Chaoqun Xu and Xuelan Fan contributed equally to this work and share the first authorship.
Authors and Affiliations
Key Laboratory of Bioactive Substances and Resources Utilization of Chinese Herbal Medicines, Ministry of Education, Institute of Medicinal Plant Development, Peking Union Medical College and Chinese Academy of Medical Sciences, No.151 MaLianWa North Road, Haidian District, Beijing, 100193, China
Chaoqun Xu, Xuelan Fan, Guoan Shen & Baolin Guo
College of Pharmacy, Jiangxi University of Chinese Medicine, Nanchang, 330004, China
You can also search for this author in PubMed Google Scholar
Contributions
Chaoqun Xu conceived and designed the study, put into effect the main bioinformatics analyses, wrote the manuscript, and prepared the figures and tables. Xuelan Fan prepared the materials, conducted the experiments, data analysis, and revised the manuscript drafts. Guoan Shen participated in the design of this study and revised the manuscript. Baolin Guo conceived and designed the study, involved in data interpretation and finalizing the manuscript draft. The authors read and approved the final manuscript.
Corresponding author
Correspondence to Baolin Guo .
Ethics declarations
Ethics approval and consent to participate.
All the experimental materials in this study have obtained the authority. The experimental research and method on all the experimental materials, including the collection of plant material, comply with relevant institutional, national, and international guidelines.
Consent for publication
Not applicable.
Competing interests
The authors declare no competing interests.
Additional information
Publisher’s note.
Springer Nature remains neutral with regard to jurisdictional claims in published maps and institutional affiliations.
Electronic supplementary material
Below is the link to the electronic supplementary material.
Supplementary Material 1
Supplementary material 2, supplementary material 3, supplementary material 4, supplementary material 5, supplementary material 6, rights and permissions.
Open Access This article is licensed under a Creative Commons Attribution-NonCommercial-NoDerivatives 4.0 International License, which permits any non-commercial use, sharing, distribution and reproduction in any medium or format, as long as you give appropriate credit to the original author(s) and the source, provide a link to the Creative Commons licence, and indicate if you modified the licensed material. You do not have permission under this licence to share adapted material derived from this article or parts of it. The images or other third party material in this article are included in the article’s Creative Commons licence, unless indicated otherwise in a credit line to the material. If material is not included in the article’s Creative Commons licence and your intended use is not permitted by statutory regulation or exceeds the permitted use, you will need to obtain permission directly from the copyright holder. To view a copy of this licence, visit http://creativecommons.org/licenses/by-nc-nd/4.0/ .
Reprints and permissions
About this article
Cite this article.
Xu, C., Fan, X., Shen, G. et al. Genome-wide identification of the phenylalanine ammonia-lyase gene from Epimedium Pubescens Maxim. (Berberidaceae): novel insight into the evolution of the PAL gene family. BMC Plant Biol 24 , 831 (2024). https://doi.org/10.1186/s12870-024-05480-z
Download citation
Received : 09 January 2024
Accepted : 01 August 2024
Published : 04 September 2024
DOI : https://doi.org/10.1186/s12870-024-05480-z
Share this article
Anyone you share the following link with will be able to read this content:
Sorry, a shareable link is not currently available for this article.
Provided by the Springer Nature SharedIt content-sharing initiative
- Epimedium pubescens
- Phenylalanine ammonia-lyase gene
- Prenylated flavonol glycoside
- Expression profiling
BMC Plant Biology
ISSN: 1471-2229
- General enquiries: [email protected]

An official website of the United States government
The .gov means it’s official. Federal government websites often end in .gov or .mil. Before sharing sensitive information, make sure you’re on a federal government site.
The site is secure. The https:// ensures that you are connecting to the official website and that any information you provide is encrypted and transmitted securely.
- Publications
- Account settings
Preview improvements coming to the PMC website in October 2024. Learn More or Try it out now .
- Advanced Search
- Journal List
- HCA Healthc J Med
- v.1(2); 2020
- PMC10324782

Introduction to Research Statistical Analysis: An Overview of the Basics
Christian vandever.
1 HCA Healthcare Graduate Medical Education
Description
This article covers many statistical ideas essential to research statistical analysis. Sample size is explained through the concepts of statistical significance level and power. Variable types and definitions are included to clarify necessities for how the analysis will be interpreted. Categorical and quantitative variable types are defined, as well as response and predictor variables. Statistical tests described include t-tests, ANOVA and chi-square tests. Multiple regression is also explored for both logistic and linear regression. Finally, the most common statistics produced by these methods are explored.
Introduction
Statistical analysis is necessary for any research project seeking to make quantitative conclusions. The following is a primer for research-based statistical analysis. It is intended to be a high-level overview of appropriate statistical testing, while not diving too deep into any specific methodology. Some of the information is more applicable to retrospective projects, where analysis is performed on data that has already been collected, but most of it will be suitable to any type of research. This primer will help the reader understand research results in coordination with a statistician, not to perform the actual analysis. Analysis is commonly performed using statistical programming software such as R, SAS or SPSS. These allow for analysis to be replicated while minimizing the risk for an error. Resources are listed later for those working on analysis without a statistician.
After coming up with a hypothesis for a study, including any variables to be used, one of the first steps is to think about the patient population to apply the question. Results are only relevant to the population that the underlying data represents. Since it is impractical to include everyone with a certain condition, a subset of the population of interest should be taken. This subset should be large enough to have power, which means there is enough data to deliver significant results and accurately reflect the study’s population.
The first statistics of interest are related to significance level and power, alpha and beta. Alpha (α) is the significance level and probability of a type I error, the rejection of the null hypothesis when it is true. The null hypothesis is generally that there is no difference between the groups compared. A type I error is also known as a false positive. An example would be an analysis that finds one medication statistically better than another, when in reality there is no difference in efficacy between the two. Beta (β) is the probability of a type II error, the failure to reject the null hypothesis when it is actually false. A type II error is also known as a false negative. This occurs when the analysis finds there is no difference in two medications when in reality one works better than the other. Power is defined as 1-β and should be calculated prior to running any sort of statistical testing. Ideally, alpha should be as small as possible while power should be as large as possible. Power generally increases with a larger sample size, but so does cost and the effect of any bias in the study design. Additionally, as the sample size gets bigger, the chance for a statistically significant result goes up even though these results can be small differences that do not matter practically. Power calculators include the magnitude of the effect in order to combat the potential for exaggeration and only give significant results that have an actual impact. The calculators take inputs like the mean, effect size and desired power, and output the required minimum sample size for analysis. Effect size is calculated using statistical information on the variables of interest. If that information is not available, most tests have commonly used values for small, medium or large effect sizes.
When the desired patient population is decided, the next step is to define the variables previously chosen to be included. Variables come in different types that determine which statistical methods are appropriate and useful. One way variables can be split is into categorical and quantitative variables. ( Table 1 ) Categorical variables place patients into groups, such as gender, race and smoking status. Quantitative variables measure or count some quantity of interest. Common quantitative variables in research include age and weight. An important note is that there can often be a choice for whether to treat a variable as quantitative or categorical. For example, in a study looking at body mass index (BMI), BMI could be defined as a quantitative variable or as a categorical variable, with each patient’s BMI listed as a category (underweight, normal, overweight, and obese) rather than the discrete value. The decision whether a variable is quantitative or categorical will affect what conclusions can be made when interpreting results from statistical tests. Keep in mind that since quantitative variables are treated on a continuous scale it would be inappropriate to transform a variable like which medication was given into a quantitative variable with values 1, 2 and 3.
Categorical vs. Quantitative Variables
Categorical Variables | Quantitative Variables |
---|---|
Categorize patients into discrete groups | Continuous values that measure a variable |
Patient categories are mutually exclusive | For time based studies, there would be a new variable for each measurement at each time |
Examples: race, smoking status, demographic group | Examples: age, weight, heart rate, white blood cell count |
Both of these types of variables can also be split into response and predictor variables. ( Table 2 ) Predictor variables are explanatory, or independent, variables that help explain changes in a response variable. Conversely, response variables are outcome, or dependent, variables whose changes can be partially explained by the predictor variables.
Response vs. Predictor Variables
Response Variables | Predictor Variables |
---|---|
Outcome variables | Explanatory variables |
Should be the result of the predictor variables | Should help explain changes in the response variables |
One variable per statistical test | Can be multiple variables that may have an impact on the response variable |
Can be categorical or quantitative | Can be categorical or quantitative |
Choosing the correct statistical test depends on the types of variables defined and the question being answered. The appropriate test is determined by the variables being compared. Some common statistical tests include t-tests, ANOVA and chi-square tests.
T-tests compare whether there are differences in a quantitative variable between two values of a categorical variable. For example, a t-test could be useful to compare the length of stay for knee replacement surgery patients between those that took apixaban and those that took rivaroxaban. A t-test could examine whether there is a statistically significant difference in the length of stay between the two groups. The t-test will output a p-value, a number between zero and one, which represents the probability that the two groups could be as different as they are in the data, if they were actually the same. A value closer to zero suggests that the difference, in this case for length of stay, is more statistically significant than a number closer to one. Prior to collecting the data, set a significance level, the previously defined alpha. Alpha is typically set at 0.05, but is commonly reduced in order to limit the chance of a type I error, or false positive. Going back to the example above, if alpha is set at 0.05 and the analysis gives a p-value of 0.039, then a statistically significant difference in length of stay is observed between apixaban and rivaroxaban patients. If the analysis gives a p-value of 0.91, then there was no statistical evidence of a difference in length of stay between the two medications. Other statistical summaries or methods examine how big of a difference that might be. These other summaries are known as post-hoc analysis since they are performed after the original test to provide additional context to the results.
Analysis of variance, or ANOVA, tests can observe mean differences in a quantitative variable between values of a categorical variable, typically with three or more values to distinguish from a t-test. ANOVA could add patients given dabigatran to the previous population and evaluate whether the length of stay was significantly different across the three medications. If the p-value is lower than the designated significance level then the hypothesis that length of stay was the same across the three medications is rejected. Summaries and post-hoc tests also could be performed to look at the differences between length of stay and which individual medications may have observed statistically significant differences in length of stay from the other medications. A chi-square test examines the association between two categorical variables. An example would be to consider whether the rate of having a post-operative bleed is the same across patients provided with apixaban, rivaroxaban and dabigatran. A chi-square test can compute a p-value determining whether the bleeding rates were significantly different or not. Post-hoc tests could then give the bleeding rate for each medication, as well as a breakdown as to which specific medications may have a significantly different bleeding rate from each other.
A slightly more advanced way of examining a question can come through multiple regression. Regression allows more predictor variables to be analyzed and can act as a control when looking at associations between variables. Common control variables are age, sex and any comorbidities likely to affect the outcome variable that are not closely related to the other explanatory variables. Control variables can be especially important in reducing the effect of bias in a retrospective population. Since retrospective data was not built with the research question in mind, it is important to eliminate threats to the validity of the analysis. Testing that controls for confounding variables, such as regression, is often more valuable with retrospective data because it can ease these concerns. The two main types of regression are linear and logistic. Linear regression is used to predict differences in a quantitative, continuous response variable, such as length of stay. Logistic regression predicts differences in a dichotomous, categorical response variable, such as 90-day readmission. So whether the outcome variable is categorical or quantitative, regression can be appropriate. An example for each of these types could be found in two similar cases. For both examples define the predictor variables as age, gender and anticoagulant usage. In the first, use the predictor variables in a linear regression to evaluate their individual effects on length of stay, a quantitative variable. For the second, use the same predictor variables in a logistic regression to evaluate their individual effects on whether the patient had a 90-day readmission, a dichotomous categorical variable. Analysis can compute a p-value for each included predictor variable to determine whether they are significantly associated. The statistical tests in this article generate an associated test statistic which determines the probability the results could be acquired given that there is no association between the compared variables. These results often come with coefficients which can give the degree of the association and the degree to which one variable changes with another. Most tests, including all listed in this article, also have confidence intervals, which give a range for the correlation with a specified level of confidence. Even if these tests do not give statistically significant results, the results are still important. Not reporting statistically insignificant findings creates a bias in research. Ideas can be repeated enough times that eventually statistically significant results are reached, even though there is no true significance. In some cases with very large sample sizes, p-values will almost always be significant. In this case the effect size is critical as even the smallest, meaningless differences can be found to be statistically significant.
These variables and tests are just some things to keep in mind before, during and after the analysis process in order to make sure that the statistical reports are supporting the questions being answered. The patient population, types of variables and statistical tests are all important things to consider in the process of statistical analysis. Any results are only as useful as the process used to obtain them. This primer can be used as a reference to help ensure appropriate statistical analysis.
Alpha (α) | the significance level and probability of a type I error, the probability of a false positive |
Analysis of variance/ANOVA | test observing mean differences in a quantitative variable between values of a categorical variable, typically with three or more values to distinguish from a t-test |
Beta (β) | the probability of a type II error, the probability of a false negative |
Categorical variable | place patients into groups, such as gender, race or smoking status |
Chi-square test | examines association between two categorical variables |
Confidence interval | a range for the correlation with a specified level of confidence, 95% for example |
Control variables | variables likely to affect the outcome variable that are not closely related to the other explanatory variables |
Hypothesis | the idea being tested by statistical analysis |
Linear regression | regression used to predict differences in a quantitative, continuous response variable, such as length of stay |
Logistic regression | regression used to predict differences in a dichotomous, categorical response variable, such as 90-day readmission |
Multiple regression | regression utilizing more than one predictor variable |
Null hypothesis | the hypothesis that there are no significant differences for the variable(s) being tested |
Patient population | the population the data is collected to represent |
Post-hoc analysis | analysis performed after the original test to provide additional context to the results |
Power | 1-beta, the probability of avoiding a type II error, avoiding a false negative |
Predictor variable | explanatory, or independent, variables that help explain changes in a response variable |
p-value | a value between zero and one, which represents the probability that the null hypothesis is true, usually compared against a significance level to judge statistical significance |
Quantitative variable | variable measuring or counting some quantity of interest |
Response variable | outcome, or dependent, variables whose changes can be partially explained by the predictor variables |
Retrospective study | a study using previously existing data that was not originally collected for the purposes of the study |
Sample size | the number of patients or observations used for the study |
Significance level | alpha, the probability of a type I error, usually compared to a p-value to determine statistical significance |
Statistical analysis | analysis of data using statistical testing to examine a research hypothesis |
Statistical testing | testing used to examine the validity of a hypothesis using statistical calculations |
Statistical significance | determine whether to reject the null hypothesis, whether the p-value is below the threshold of a predetermined significance level |
T-test | test comparing whether there are differences in a quantitative variable between two values of a categorical variable |
Funding Statement
This research was supported (in whole or in part) by HCA Healthcare and/or an HCA Healthcare affiliated entity.
Conflicts of Interest
The author declares he has no conflicts of interest.
Christian Vandever is an employee of HCA Healthcare Graduate Medical Education, an organization affiliated with the journal’s publisher.
This research was supported (in whole or in part) by HCA Healthcare and/or an HCA Healthcare affiliated entity. The views expressed in this publication represent those of the author(s) and do not necessarily represent the official views of HCA Healthcare or any of its affiliated entities.

IMAGES
VIDEO
COMMENTS
Qualitative Research: Data Collection, Analysis, and ...
Learn how to use existing data to answer new research questions in pediatric health care. Explore the process, advantages, and limitations of secondary data analysis, as well as public datasets and examples.
Secondary Analysis Research - PMC
How to use and assess qualitative research methods - PMC
Learn what secondary data analysis is, how it can be used to answer new research questions, and what benefits and limitations it has. See an exemplar of pediatric focused research using a publicly available dataset from the National Survey of Children's Health.
Learning to Do Qualitative Data Analysis: A Starting Point
We offer best-practice recommendations for journal reviewers, editors, and authors regarding data collection and preparation. Our recommendations are applicable to research adopting different epistemological and ontological perspectives—including both quantitative and qualitative approaches—as well as research addressing micro (i.e., individuals, teams) and macro (i.e., organizations ...
In secondary data analysis (SDA) studies, investigators use data collected by other researchers to address different questions. Like primary data researchers, SDA investigators must be knowledgeable about their research area to identify datasets that are a good fit for an SDA. Several sources of datasets may be useful for SDA, and examples of ...
Methods. Data collection. Qualitative content analysis. Observation protocol. Interpretation of results. Communicative validation. In this section we elaborate on the methods we use to collect and analyse our data. We followed a multi-method data collection process in which we triangulated data obtained via different collection methods. The ...
A practical guide to data analysis in general literature reviews
Learn how to collect data for research purposes, whether quantitative or qualitative, and choose the best methods and procedures. Find out how to define your research aim, choose your data collection method, plan your data collection procedures, and collect the data.
Data Collection Methods and Tools for Research
Protecting against researcher bias in secondary data ...
Learn what data collection is, types of data collection methods, common challenges, and steps involved in data collection. Explore examples of surveys, interviews, observation, automated data collection, and sourcing data through information service providers.
Learn about the six major methods of data collection used by educational researchers: tests, questionnaires, interviews, focus groups, observation, and constructed, secondary, and existing data. Explore the strengths and weaknesses of each method and how to mix them in your research design.
Learn how to collect and manage data for research at CWRU, including data types, storage options, security, and categorization. Data collection is the process of gathering and measuring information used for research, and data itself is just a collection of numbers.
This document provides guidance to investigators conducting research involving the secondary use of existing data, such as medical records, student records, etc. It explains when the secondary use of existing data does not require review, when it is exempt, and when it is non-exempt.
Data Collection Methods and Tools for Research; A Step- ...
Learn how to collect data for research using different methods, such as surveys, interviews, experiments, and archival research. Find out how to operationalise, sample, and standardise your data collection procedures.
Methods of Data Collection, Representation, and Analysis
Data collection is the process of gathering and collecting information from various sources to analyze and make informed decisions. Learn about different types of data collection, such as primary, secondary, qualitative, and quantitative, and the methods to collect them, such as surveys, interviews, experiments, and more.
In sociological research, data analysis plays a crucial role in uncovering patterns, relationships, and explanations for various social phenomena. One of the most fundamental forms of statistical analysis used in sociology is the univariate analysis. At its core, univariate analysis involves the examination of a single variable at a time.
Purpose This study aimed to explore the correlation between hemoglobin levels and albuminuria in US adults. Methods This cross-sectional investigation analyzed the National Health and Nutrition Examination Survey (NHANES) information from 2011 to 2020. Data on hemoglobin, albuminuria, and other variables were collected from all participants. The logistic-regression analyses and smoothed curves ...
Design: Selection of Data Collection Methods - PMC
Background Phenylalanine ammonia-lyase (PAL) serves as a key gateway enzyme, bridging primary metabolism and the phenylpropanoid pathway, and thus playing an indispensable role in flavonoid, anthocyanin and lignin biosynthesis. PAL gene families have been extensively studied across species using public genomes. However, a comprehensive exploration of PAL genes in Epimedium species, especially ...
Introduction. Statistical analysis is necessary for any research project seeking to make quantitative conclusions. The following is a primer for research-based statistical analysis. It is intended to be a high-level overview of appropriate statistical testing, while not diving too deep into any specific methodology.