- Demo Pricing Blog
- Get started free
.webp)

DOE vs Bayesian Optimization: A Comparison
%20(1).png)
Design of Experiments (DOE) and Bayesian Optimization are two popular methods employed in various fields for optimizing processes, systems, or products. They are particularly useful in scenarios where the objective function is expensive to evaluate, noisy, or lacks a closed-form expression. In this article, we will provide a comparison between these two methods, including their basic principles, strengths, weaknesses, and potential applications.
Design of Experiments (DOE)
DOE is a statistical method used to plan, conduct, analyze, and interpret controlled tests to evaluate the factors that affect the performance of a system or process. It allows researchers to systematically vary input factors and analyze the impact on the response variable, with the ultimate goal of optimizing performance.
Basic principles
DOE is based on three fundamental principles:
- Randomization: Randomizing the order of experiments helps minimize the effects of uncontrolled factors or experimental errors.
- Replication: Performing multiple experiments under the same conditions ensures that the results are reliable and accounts for variability.
- Blocking: Grouping similar experimental units together helps control the effects of external factors that could affect the response variable.
- DOE is a well-established and widely-used methodology in various fields, such as engineering, agriculture, and pharmaceuticals.
- It is particularly useful when there are multiple input factors or interactions between factors that need to be considered.
- DOE can be combined with other optimization techniques to improve the efficiency of the process.
- DOE might require a large number of experiments, which can be costly and time-consuming.
- It is less effective when dealing with high-dimensional problems.
- DOE can be challenging to apply when the objective function is non-linear or non-convex.
Bayesian Optimization
Bayesian Optimization is a global optimization method for expensive black-box functions, based on the principles of Bayesian statistics and Gaussian process regression. It provides a probabilistic model of the objective function and uses it to guide the search for the optimal solution.
Bayesian Optimization involves two main components:
- Surrogate model: A probabilistic model (typically Gaussian process regression) is used to approximate the objective function based on the available data points.
- Acquisition function: An acquisition function is used to determine the next sampling point based on the surrogate model. It balances exploration (sampling in less explored regions) and exploitation (sampling around the current best estimate).
- Bayesian Optimization is highly efficient at handling expensive, noisy, or black-box objective functions.
- It is suitable for high-dimensional problems.
- The surrogate model provides a measure of uncertainty, which is useful for managing risk in the optimization process.
- Bayesian Optimization can be computationally expensive, particularly when dealing with a large number of observations or high-dimensional spaces.
- The performance of Bayesian Optimization is sensitive to the choice of surrogate model and acquisition function.
- It might require expert knowledge to select appropriate hyperparameters and tune the optimization algorithm.
Quantum Boost Platform: Overcoming Bayesian Optimization Weaknesses
Our proprietary software platform has been developed to address the weaknesses of the Bayesian Optimization approach, making it an ideal tool for users who want to harness the power of optimization without the need for extensive expertise. We have carefully designed our platform to mitigate the three main challenges associated with Bayesian Optimization.
- Computationally expensive: We handle the entire computation process on behalf of our users. Utilizing an advanced distributed computing approach, we efficiently parallelize the optimization process across cloud-based infrastructure to expedite the calculations. As a result, users can concentrate on interpreting the optimization results and making informed decisions, while our platform takes care of managing computational resources.
- Sensitive to the choice of surrogate model: Our team of experts, with deep knowledge in the chemical industry, has carefully selected and pre-configured surrogate models that are best suited for this specific domain. This not only takes care of the model selection process for our users but also ensures that the platform delivers reliable and accurate optimization results tailored to the unique requirements of the chemical industry.
- Tuning hyperparameters and optimization algorithm: Our platform intelligently adjusts the hyperparameters and optimization algorithm based on the problem's characteristics and the available data, eliminating the need for users to possess expert knowledge or spend time on manual tuning.
In addition to addressing the above challenges, our platform's intuitive user interface allows users to tap into the benefits of Bayesian Optimization without requiring expert knowledge in statistics or optimization. With a guided workflow, visualizations, and interactive tools, users can quickly set up optimization tasks, monitor progress, and analyze results, enabling them to make data-driven decisions with ease and confidence.
By offering a comprehensive solution that handles the weaknesses of Bayesian Optimization, our software platform empowers users to harness the full potential of this powerful optimization technique, driving innovation and efficiency across the chemical industry.
Design of Experiments (DOE) and Bayesian Optimization are powerful optimization techniques, each with their own unique strengths and weaknesses. While the choice between these methods depends on the problem's specifics and the nature of the objective function, understanding their nuances is critical for effective decision-making and optimization.
Bayesian Optimization inherently requires fewer experiments than DOE, making it an attractive choice for many applications. However, its applicability can be limited due to challenges such as being computationally prohibitive and the complexity involved in choosing the right models for the problem at hand. Quantum Boost platform addresses these challenges, offering a comprehensive and user-friendly solution that allows users to fully harness potential of the Bayesian Optimization even in scenarios where it would have been difficult to apply otherwise.
Related posts
.png)
How to use Quantum Boost to accelerate the development of chemicals and materials
This guide provides a comprehensive overview of Quantum Boost's essential features to help accelerating your projects.
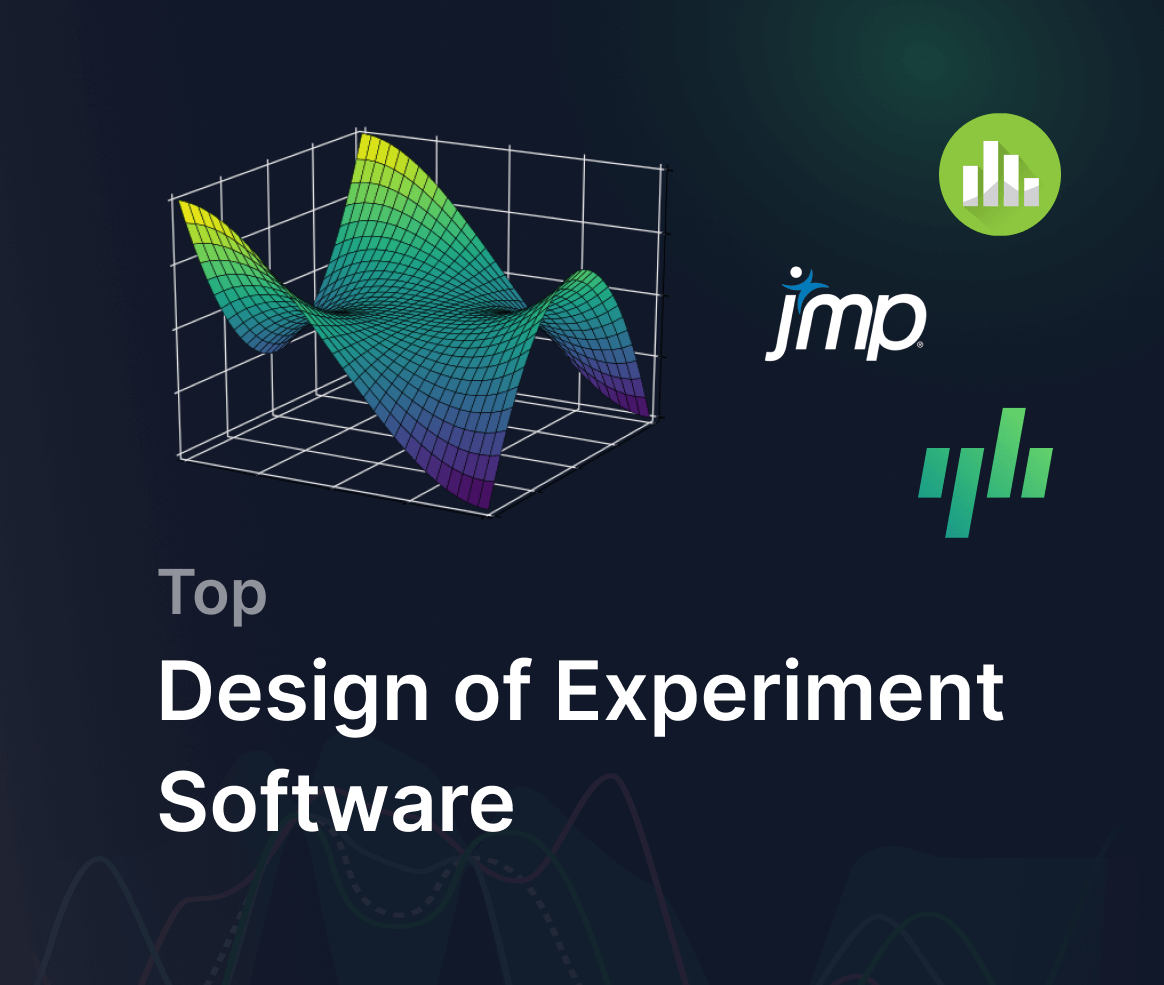
The 5 Best Design of Experiment (DoE) software
DoE software is a tool that facilitates the Design of Experiments process. In this article, we will explore the top 5 best DoE software.
- Privacy policy
Introduction to Bayesian Optimal Experimental Design
Static and adaptive design strategies
What questions should we ask in an online survey? Which point should we query next in an active learning loop? Where should we place sensors, e.g. to detect faults and defects most efficiently? These, and many more, seemingly distinct questions constitute the same fundamental problem—designing experiments to collect data that will help us learn about an underlying process. Bayesian Optimal Experimental Design (BOED) is a powerful yet elegant mathematical framework for tackling such problems. This introductory post describes the BOED framework and highlights some of the computational challenges of deploying it in applications. For an extensive review, see Ryan et al. (2016).
In the BOED framework, the relationship between the parameters $\theta$ of the underlying process, the controllable designs $\xi$, and the outcomes $y$ are described using a likelihood function (or simulator) $p(y|\xi, \theta)$. Our initial knowledge or beliefs about the parameters $\theta$ are encapsulated in a prior $p(\theta)$.
We assume $p(\theta)$ and $p(y|\theta, \xi)$ as given, so our task is to choose experiments $\xi$ that enable us to learn about $\theta$ as efficiently as possible . One possible measure of efficiency is the Expected Information Gain (EIG), proposed by Lindley (1956). Indeed, the guiding principle in BOED is that of information maximisation, and EIG is the central quantity of interest, so we introduce it below in detail.
Information Gain and Expected Information Gain (EIG)
Formally, we quantify the information gain (IG) of an experiment as the reduction in Shannon entropy from the prior to the posterior $$ \text{IG}(\xi, y) = H\big[ p(\theta) \big] - H \big[ p(\theta | y, \xi) \big]. $$ Using this metric, we can now compare and rank design-outcome pairs; for example, we can say the pair $(\xi_2, y_2)$ is more informative about $\theta$ than $(\xi_1, y_1)$. However, we can’t use IG to find the optimal design as it depends on the outcome $y$ and hence can’t calculate it until after the experiment has been performed. An easy way to fix that is to take the expectation with respect to all possible outcomes $y$ for an experiment $\xi$, which defines the expected information gain $$ \text{EIG}(\xi) = \mathbb{E}_{p(y|\xi)}[\text{IG}(\xi, y)], $$ where $p(y|\xi) = \mathbb{E}_{p(\theta)} \big[ p(y|\theta, \xi) \big]$ is the marginal distribution of $y$. The optimal design is then $\xi^* = \arg\max_\xi \text{EIG}(\xi)$
Unfortunately, optimising the EIG is an extremely challenging task. In fact, even computing $\text{EIG}(\xi)$ for a fixed $\xi$ is very computationally costly, as both the posterior $p(\theta | y, \xi)$ and the marginal $p(y|\xi)$ are in general intractable (i.e. not known in closed form). How to deal with these intractabilities will be the topic of another post. For now, we assume that there are computational methods that allow us to estimate and optimise the EIG efficiently.
Multiple experiments
More often than not, we wish to perform multiple experiments $\xi_1, \xi_2, \dots, \xi_T$. Broadly speaking, there are two ways to approach the problem of designing multiple experiments: static (aka batch or open-loop) and sequential (aka adaptive or closed-loop).
Static experimentation
Instead optimising $\text{EIG}(\xi)$ with respect to a single design $\xi$, we can set $\underline{\xi} := (\xi_1, \xi_2, \dots, \xi_T)$ and optimise $\text{EIG}(\underline{\xi})$ to give us an optimal batch of designs. Static design strategies are useful when experiments need to be performed in parallel, which can be suitable, for example, in applications where it takes a long time to obtain the outcomes after the experiment has been performed. As a result, the outcome of any experiment cannot affect the design of the other experiments.
Sequential experimentation
In practice, we are often more interested in performing multiple experiments in a sequence $\xi_1, \xi_2, \dots, \xi_T$, and allowing the choice of each design $\xi_{t+1}$ to be guided by the data from past experiments, which we call the history and denote by $h_t:= (\xi_1, y_1, \dots, \xi_t, y_t)$.
Myopic design (one-step lookahead)
The traditional approach to designing adaptive experiments is to fit a posterior $p(\theta| h_t)$ and use that as a prior in the EIG optimisation, which would give the next design $\xi_{t+1}$. This is known as greedy or myopic design strategy: at each step of the experiment, we are optimising for the next best choice of $\xi$, without taking into account that there are $T-1$ future experiments to be performed. Despite being suboptimal, the sequential myopic strategy can bring huge improvements over static designs.
Unfortunately, the computational cost required to run the myopic strategy severely limits its applicability. At each stage $t+1$ of the experiment, we need to perform compute (or rather approximate) the posterior $p(\theta|h_t)$, which is expensive and cannot be done in advance as it depends on $y_{1:t}$. Furthermore, to obtain $\xi_{t+1}$, we need to maximise the EIG, which is computationally even more challenging. Both of these steps need to be performed during the live experiment. Unless we are dealing with unusually simple models, it will be infeasible to run the myopic (or non-myopic $K$-step lookahead) strategy in real-time experiments.
Amortized sequential BOED
Until recently, to perform BOED in real-time applications, one had to forego adaptivity and use static strategies instead. (Or alternatively, forego being Bayesian optimal and use an adaptive heuristic).
Deep Adaptive Design (DAD, Foster et al. 2021) is a method developed by our group at Oxford that has opened the door to running adaptive BOED in real-time. At the heart of the DAD approach is a design policy network , which is trained prior to the live experiment. The network takes a history $h_t$ as an input and outputs the design $\xi_{t+1}$ to be used for the next experiment. Design decisions are thus made in milliseconds, using a single forward pass through the network.
The key methodological difference, which marks a critical change from previous BOED approaches, is that DAD learns a policy , instead of learning designs. Put differently, previous methods optimise individual designs, while DAD optimises the parameters of a neural network, which is trained to propose optimal designs. DAD is thus the first policy-based approach to BOED.
To be able to train the design network, we addressed some major technical challenges, including formulating a unified training objective that doesn’t require calculating intermediate posteriors and EIGs, introducing a tractable surrogate for that objective and suggesting an appropriate architecture for the design network.
I’ll probably write another blog post to talk about our work on policy-based BOED at greater length. In the meantime, if you’re interested in learning more about DAD, you can watch our ICML talk or have a look at the full paper .
Foster A., D. R. Ivanova, I. Malik, and T. Rainforth, “Deep adaptive design: Amortizing sequential Bayesian experimental design”, International Conference on Machine Learning (ICML), 2021.
Lindley D. V., “On a measure of the information provided by an experiment”, The Annals of Mathematical Statistics, pages 986–1005, 1956.
Ryan E. G., C. C. Drovandi, J. M. McGree, and A. N. Pettitt, “A review of modern computational algorithms for Bayesian optimal design”, International Statistical Review, 2016.
Desi R. Ivanova
Research fellow in ml.
Research Fellow @OxCSML . Former quant, former former gymnast.
- Introduction to Neural Compression
- DOI: 10.1109/ACCESS.2020.2966228
- Corpus ID: 210970589
Bayesian Optimization for Adaptive Experimental Design: A Review
- S. Greenhill , Santu Rana , +2 authors S. Venkatesh
- Published in IEEE Access 13 January 2020
- Engineering, Mathematics, Computer Science
Figures and Tables from this paper
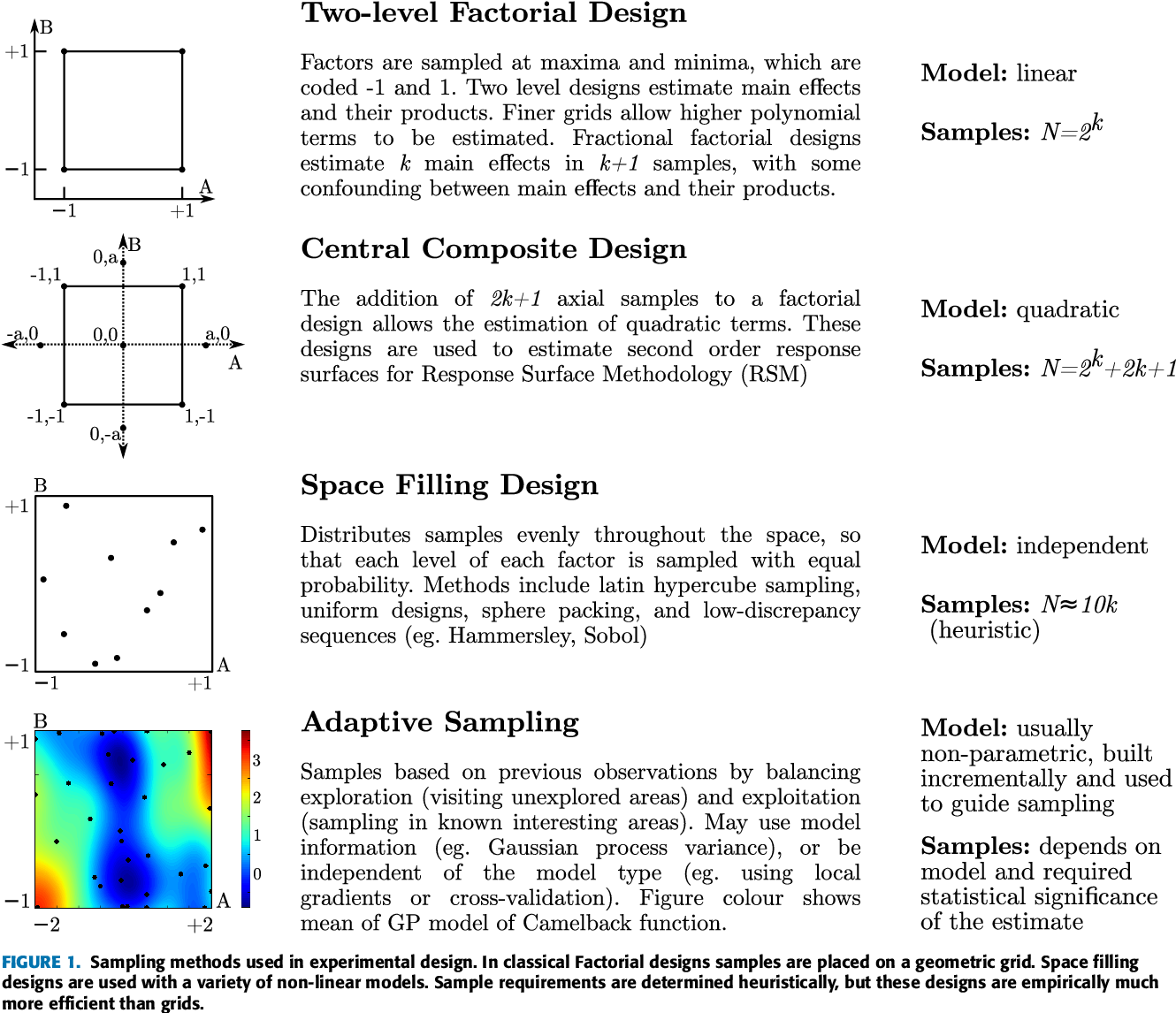
216 Citations
Bayesian optimization with fixed constraints using acceptance functions, transfer learning for bayesian optimization: a survey, bayesian optimisation for sequential experimental design with applications in additive manufacturing, bayesian optimization with informative covariance, bayesian adaptive calibration and optimal design, batch bayesian optimization via maximizing variance change, quantifying uncertainty with ensembles of surrogates for blackbox optimization, enhanced bayesian optimization via preferential modeling of abstract properties, proximal biasing for bayesian optimization and characterization of physical systems, initial sample selection in bayesian optimization for combinatorial optimization of chemical compounds, 121 references, bayesopt: a bayesian optimization library for nonlinear optimization, experimental design and bandits.
- Highly Influential
Bayesian optimization for materials design
Bayesian optimization with inequality constraints, bayesian optimization with shape constraints, a tutorial on bayesian optimization, multi-fidelity bayesian optimisation with continuous approximations, batch bayesian optimization via simulation matching, a tutorial on bayesian optimization of expensive cost functions, with application to active user modeling and hierarchical reinforcement learning, bayesian optimization with unknown constraints, exploiting strategy-space diversity for batch bayesian optimization, related papers.
Showing 1 through 3 of 0 Related Papers
9 th & 10 th July 2025 NEC, Birmingham, UK
Doe vs bayesian optimization: a comparison.
Friday, 28 April 2023

Design of Experiments (DOE) and Bayesian Optimization are two popular methods employed in various fields for optimizing processes, systems, or products. They are particularly useful in scenarios where the objective function is expensive to evaluate, noisy, or lacks a closed-form expression. In this article, we will provide a comparison between these two methods, including their basic principles, strengths, weaknesses, and potential applications.
Design of Experiments (DOE)
DOE is a statistical method used to plan, conduct, analyse, and interpret controlled tests to evaluate the factors that affect the performance of a system or process. It allows researchers to systematically vary input factors and analyse the impact on the response variable, with the ultimate goal of optimizing performance.
Basic principles
DOE is based on three fundamental principles:
- Randomization: Randomizing the order of experiments helps minimize the effects of uncontrolled factors or experimental errors.
- Replication: Performing multiple experiments under the same conditions ensures that the results are reliable and accounts for variability.
- Blocking: Grouping similar experimental units together helps control the effects of external factors that could affect the response variable.
- DOE is a well-established and widely-used methodology in various fields, such as engineering, agriculture, and pharmaceuticals.
- It is particularly useful when there are multiple input factors or interactions between factors that need to be considered.
- DOE can be combined with other optimization techniques to improve the efficiency of the process.
Weaknesses
- DOE might require a large number of experiments, which can be costly and time-consuming.
- It is less effective when dealing with high-dimensional problems.
- DOE can be challenging to apply when the objective function is non-linear or non-convex.
Bayesian Optimization
Bayesian Optimization is a global optimization method for expensive black-box functions, based on the principles of Bayesian statistics and Gaussian process regression. It provides a probabilistic model of the objective function and uses it to guide the search for the optimal solution.
Bayesian Optimization involves two main components:
- Surrogate model: A probabilistic model (typically Gaussian process regression) is used to approximate the objective function based on the available data points.
- Acquisition function: An acquisition function is used to determine the next sampling point based on the surrogate model. It balances exploration (sampling in less explored regions) and exploitation (sampling around the current best estimate).
- Bayesian Optimization is highly efficient at handling expensive, noisy, or black-box objective functions.
- It is suitable for high-dimensional problems.
- The surrogate model provides a measure of uncertainty, which is useful for managing risk in the optimization process.
- Bayesian Optimization can be computationally expensive, particularly when dealing with a large number of observations or high-dimensional spaces.
- The performance of Bayesian Optimization is sensitive to the choice of surrogate model and acquisition function.
- It might require expert knowledge to select appropriate hyperparameters and tune the optimization algorithm.
Quantum Boost Platform: Overcoming Bayesian Optimization Weaknesses
The Quantum Boost platform has been developed to address the weaknesses of the Bayesian Optimization, making it an ideal tool for users who want to harness the power of optimization without the need for extensive expertise. We have carefully designed our platform to mitigate the three main challenges associated with Bayesian Optimization.
- Computationally expensive: We handle the entire computation process on behalf of our users. Utilizing an advanced distributed computing approach, we efficiently parallelize the optimization process across cloud-based infrastructure to expedite the calculations. As a result, users can concentrate on interpreting the optimization results and making informed decisions, while our platform takes care of managing computational resources.
- Sensitive to the choice of surrogate model: Our team of experts, with deep knowledge in the chemical industry, has carefully selected and pre-configured surrogate models that are best suited for this specific domain. This not only takes care of the model selection process for our users but also ensures that the platform delivers reliable and accurate optimization results tailored to the unique requirements of the chemical industry.
- Tuning hyperparameters and optimization algorithm: Our platform intelligently adjusts the hyperparameters and optimization algorithm based on the problem’s characteristics and the available data, eliminating the need for users to possess expert knowledge or spend time on manual tuning.
In addition to addressing the above challenges, our platform’s intuitive user interface allows users to tap into the benefits of Bayesian Optimization without requiring expert knowledge in statistics or optimization. With a guided workflow, visualizations, and interactive tools, users can quickly set up optimization tasks, monitor progress, and analyze results, enabling them to make data-driven decisions with ease and confidence.
By offering a comprehensive solution that handles the weaknesses of Bayesian Optimization, our software platform empowers users to harness the full potential of this powerful optimization technique, driving innovation and efficiency across the chemical industry.
Conclusion
Design of Experiments (DOE) and Bayesian Optimization are powerful optimization techniques, each with their own unique strengths and weaknesses. While the choice between these methods depends on the problem’s specifics and the nature of the objective function, understanding their nuances is critical for effective decision-making and optimization.
Bayesian Optimization inherently requires fewer experiments than DOE, making it an attractive choice for many applications. However, its applicability can be limited due to challenges such as being computationally prohibitive and the complexity involved in choosing the right models for the problem at hand. Quantum Boost platform addresses these challenges, offering a comprehensive and user-friendly solution that allows users to fully harness potential of the Bayesian Optimization even in scenarios where it would have been difficult to apply otherwise.
- All categories
- Exhibitor Spotlight
- Expert Interviews
- Press Releases
- Uncategorized
Latest News
Update nec conference data.
By clickinhg on the buttons below you will be updating the NEC Conference data! This will start the API call to the EP : HUB site to gather the data.
Note : The process can take a few moments to action! So ONLY PRESS A BUTTON ONCE .
When the API call is complete and the data has been updated, the web page will refresh.
Bayesian Optimization and DOE
Read the NASBOT paper. Read the MPS paper. Read the Dragonfly paper. --> Get the code. -->
Bayesian Optimization (BO) and Bayesian Goal-Oriented Design of Experiments (DOE).
Read the papers.
[1] Neural Architecture Search with Bayesian Optimisation and Optimal Transport [ paper ].  Kirthevasan Kandasamy ,  Willie Neiswanger ,  Jeff Schneider ,  Barnabas Póczos ,  Eric Xing .  Accepted at NeurIPS 2018 . Code on github .
[2] Myopic Posterior Sampling for Adaptive Goal Oriented Design of Experiments [ paper ].  Kirthevasan Kandasamy ,  Willie Neiswanger ,  Reed Zhang .  Akshay Krishnamurthy .  Jeff Schneider ,  Barnabas Póczos ,  Accepted at ICML 2019 . Code on github .
[3] Tuning Hyperparameters without Grad Students: Scalable and Robust Bayesian Optimisation with Dragonfly [ paper ].  Kirthevasan Kandasamy ,  Karun Raju Vysyaraju ,  Willie Neiswanger ,  Biswajit Paria ,  Christopher R. Collins ,  Jeff Schneider ,  Barnabas Póczos ,  Eric Xing .  AISTATS 2015 .--> Code on github .