Correlation in Psychology: Meaning, Types, Examples & coefficient
Saul Mcleod, PhD
Editor-in-Chief for Simply Psychology
BSc (Hons) Psychology, MRes, PhD, University of Manchester
Saul Mcleod, PhD., is a qualified psychology teacher with over 18 years of experience in further and higher education. He has been published in peer-reviewed journals, including the Journal of Clinical Psychology.
Learn about our Editorial Process
Olivia Guy-Evans, MSc
Associate Editor for Simply Psychology
BSc (Hons) Psychology, MSc Psychology of Education
Olivia Guy-Evans is a writer and associate editor for Simply Psychology. She has previously worked in healthcare and educational sectors.
On This Page:
Correlation means association – more precisely, it measures the extent to which two variables are related. There are three possible results of a correlational study: a positive correlation, a negative correlation, and no correlation.
- A positive correlation is a relationship between two variables in which both variables move in the same direction. Therefore, one variable increases as the other variable increases, or one variable decreases while the other decreases. An example of a positive correlation would be height and weight. Taller people tend to be heavier.
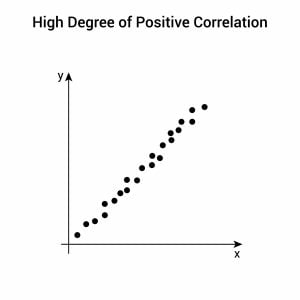
- A negative correlation is a relationship between two variables in which an increase in one variable is associated with a decrease in the other. An example of a negative correlation would be the height above sea level and temperature. As you climb the mountain (increase in height), it gets colder (decrease in temperature).
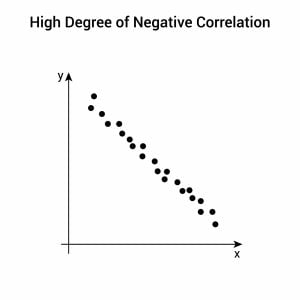
- A zero correlation exists when there is no relationship between two variables. For example, there is no relationship between the amount of tea drunk and the level of intelligence.
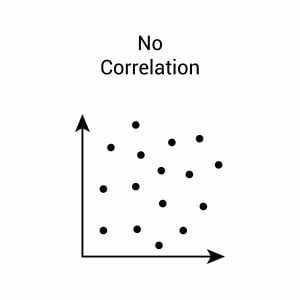

Scatter Plots
A correlation can be expressed visually. This is done by drawing a scatter plot (also known as a scattergram, scatter graph, scatter chart, or scatter diagram).
A scatter plot is a graphical display that shows the relationships or associations between two numerical variables (or co-variables), which are represented as points (or dots) for each pair of scores.
A scatter plot indicates the strength and direction of the correlation between the co-variables.
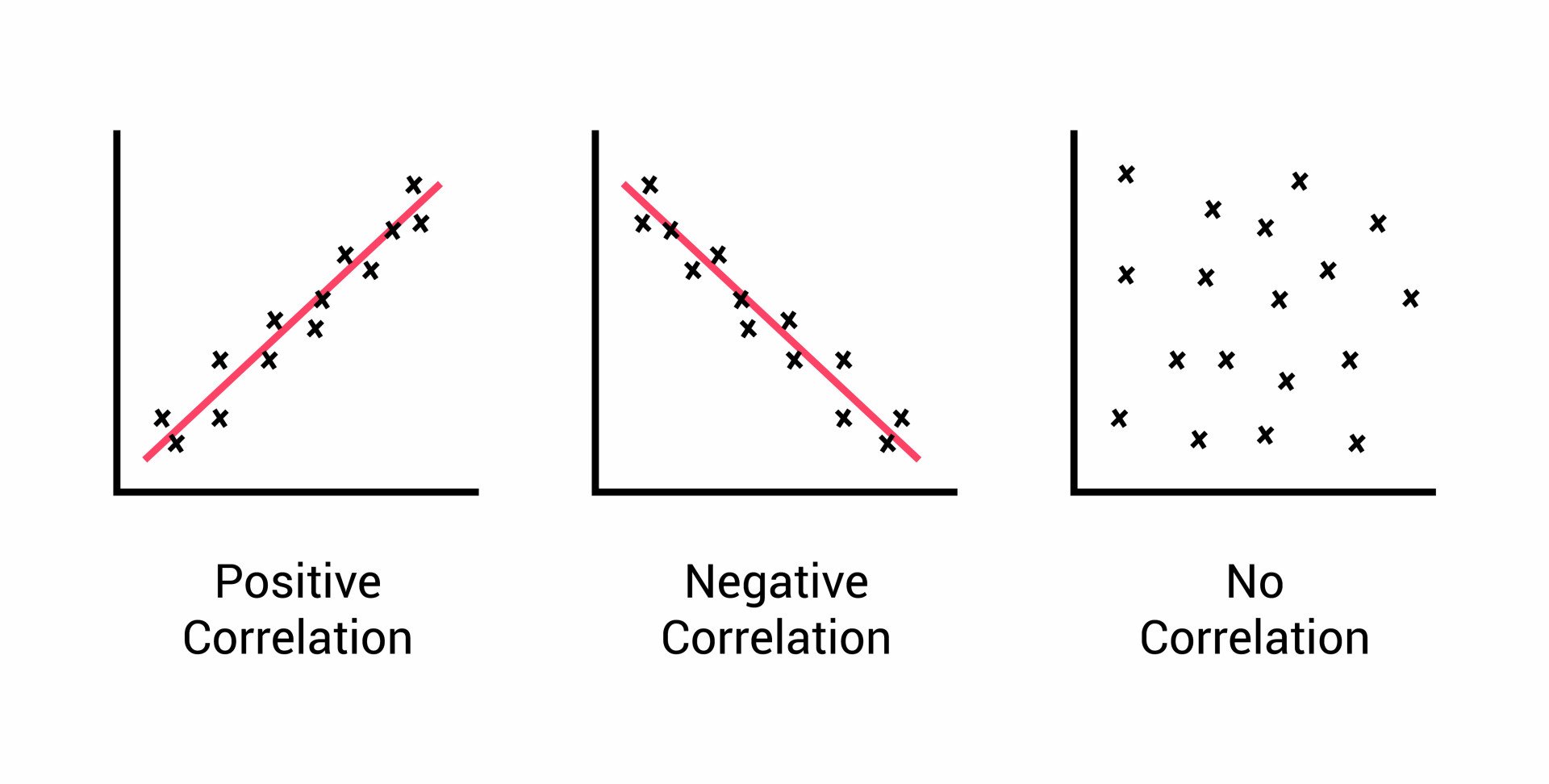
When you draw a scatter plot, it doesn’t matter which variable goes on the x-axis and which goes on the y-axis.
Remember, in correlations, we always deal with paired scores, so the values of the two variables taken together will be used to make the diagram.
Decide which variable goes on each axis and then simply put a cross at the point where the two values coincide.
Uses of Correlations
- If there is a relationship between two variables, we can make predictions about one from another.
- Concurrent validity (correlation between a new measure and an established measure).
Reliability
- Test-retest reliability (are measures consistent?).
- Inter-rater reliability (are observers consistent?).
Theory verification
- Predictive validity.
Correlation Coefficients
Instead of drawing a scatter plot, a correlation can be expressed numerically as a coefficient, ranging from -1 to +1. When working with continuous variables, the correlation coefficient to use is Pearson’s r.
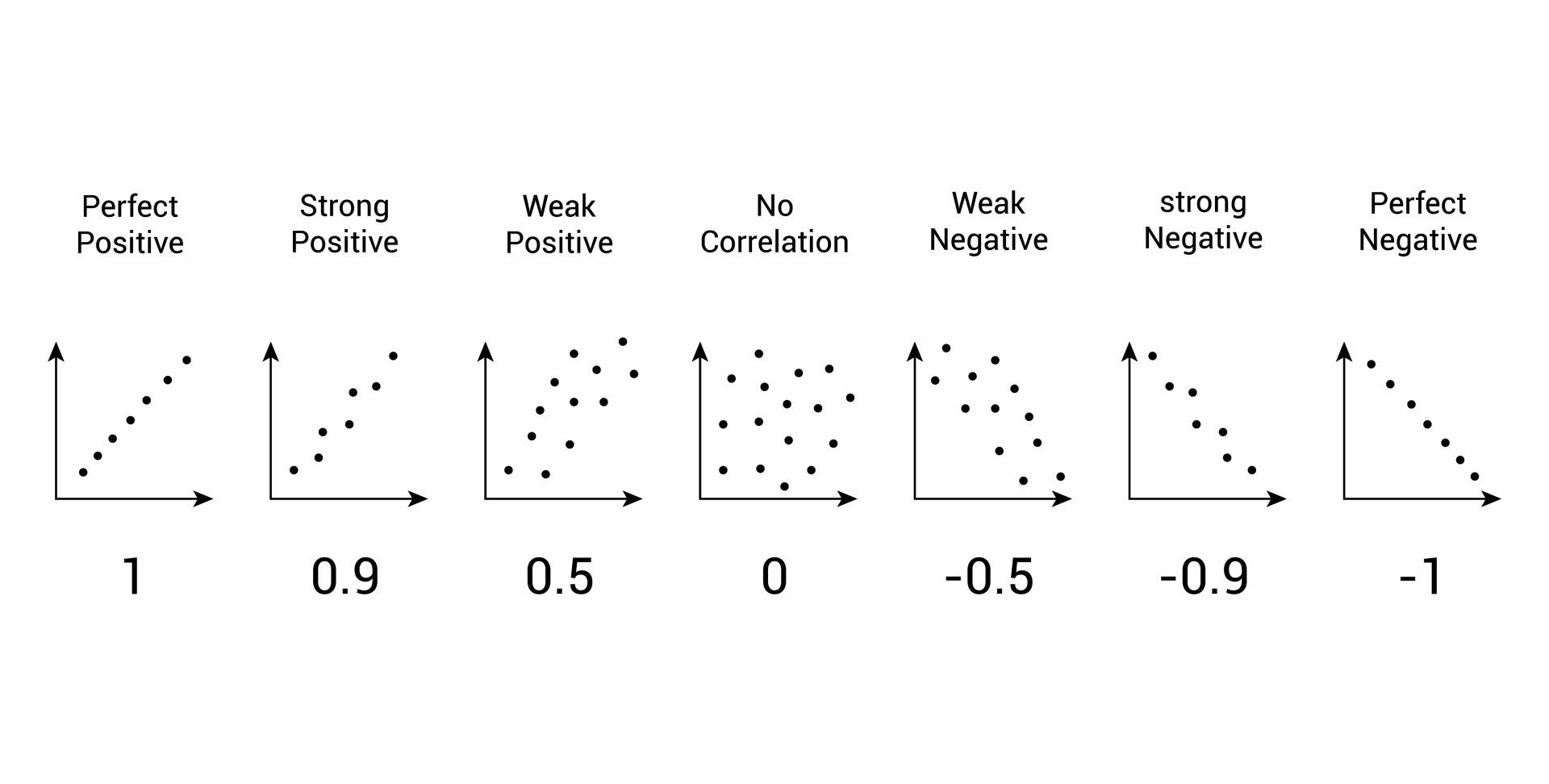
The correlation coefficient ( r ) indicates the extent to which the pairs of numbers for these two variables lie on a straight line. Values over zero indicate a positive correlation, while values under zero indicate a negative correlation.
A correlation of –1 indicates a perfect negative correlation, meaning that as one variable goes up, the other goes down. A correlation of +1 indicates a perfect positive correlation, meaning that as one variable goes up, the other goes up.
There is no rule for determining what correlation size is considered strong, moderate, or weak. The interpretation of the coefficient depends on the topic of study.
When studying things that are difficult to measure, we should expect the correlation coefficients to be lower (e.g., above 0.4 to be relatively strong). When we are studying things that are easier to measure, such as socioeconomic status, we expect higher correlations (e.g., above 0.75 to be relatively strong).)
In these kinds of studies, we rarely see correlations above 0.6. For this kind of data, we generally consider correlations above 0.4 to be relatively strong; correlations between 0.2 and 0.4 are moderate, and those below 0.2 are considered weak.
When we are studying things that are more easily countable, we expect higher correlations. For example, with demographic data, we generally consider correlations above 0.75 to be relatively strong; correlations between 0.45 and 0.75 are moderate, and those below 0.45 are considered weak.
Correlation vs. Causation
Causation means that one variable (often called the predictor variable or independent variable) causes the other (often called the outcome variable or dependent variable).
Experiments can be conducted to establish causation. An experiment isolates and manipulates the independent variable to observe its effect on the dependent variable and controls the environment in order that extraneous variables may be eliminated.
A correlation between variables, however, does not automatically mean that the change in one variable is the cause of the change in the values of the other variable. A correlation only shows if there is a relationship between variables.
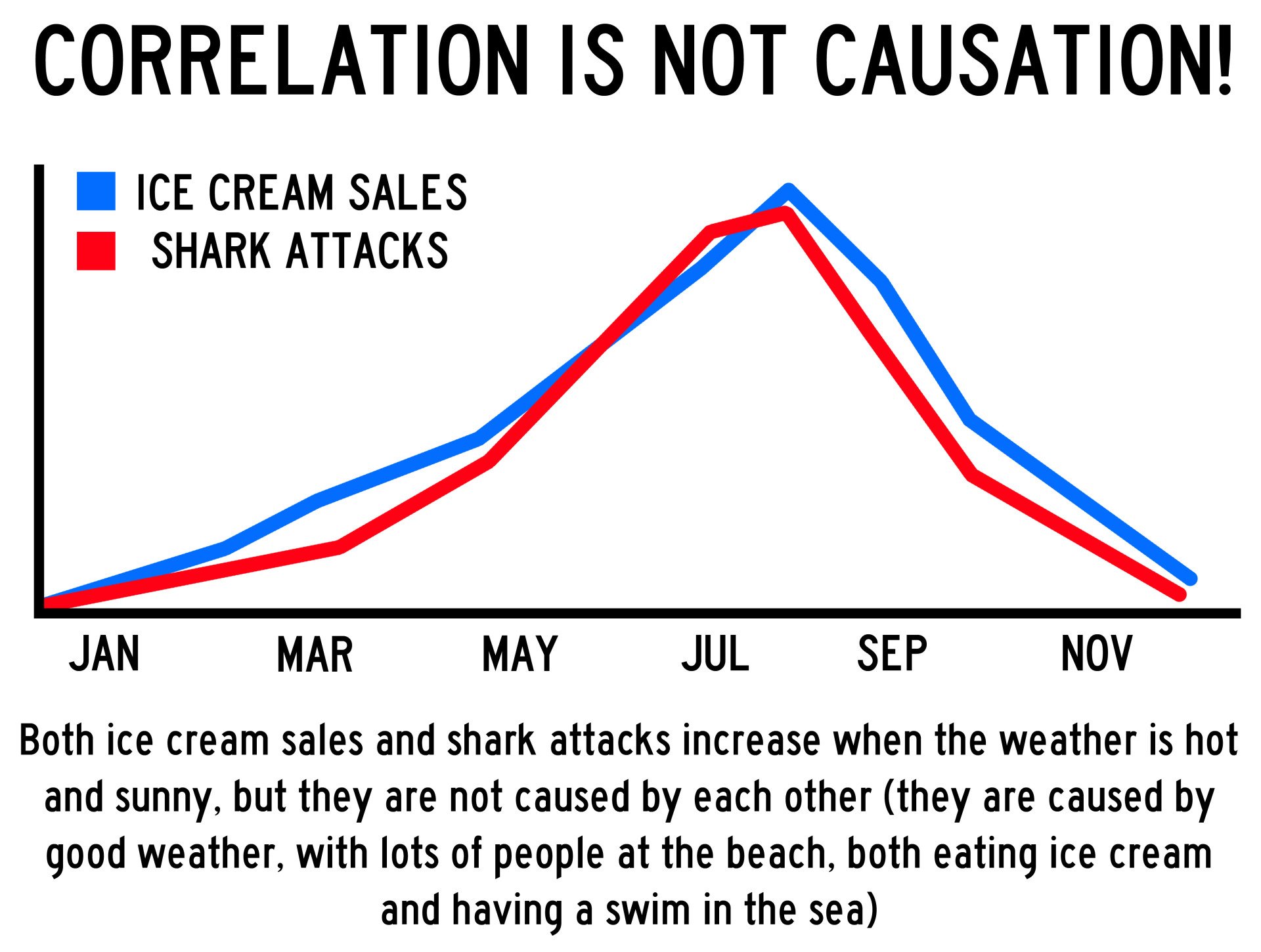
While variables are sometimes correlated because one does cause the other, it could also be that some other factor, a confounding variable , is actually causing the systematic movement in our variables of interest.
Correlation does not always prove causation, as a third variable may be involved. For example, being a patient in a hospital is correlated with dying, but this does not mean that one event causes the other, as another third variable might be involved (such as diet and level of exercise).
“Correlation is not causation” means that just because two variables are related it does not necessarily mean that one causes the other.
A correlation identifies variables and looks for a relationship between them. An experiment tests the effect that an independent variable has upon a dependent variable but a correlation looks for a relationship between two variables.
This means that the experiment can predict cause and effect (causation) but a correlation can only predict a relationship, as another extraneous variable may be involved that it not known about.
1. Correlation allows the researcher to investigate naturally occurring variables that may be unethical or impractical to test experimentally. For example, it would be unethical to conduct an experiment on whether smoking causes lung cancer.
2 . Correlation allows the researcher to clearly and easily see if there is a relationship between variables. This can then be displayed in a graphical form.
Limitations
1 . Correlation is not and cannot be taken to imply causation. Even if there is a very strong association between two variables, we cannot assume that one causes the other.
For example, suppose we found a positive correlation between watching violence on T.V. and violent behavior in adolescence.
It could be that the cause of both these is a third (extraneous) variable – for example, growing up in a violent home – and that both the watching of T.V. and the violent behavior is the outcome of this.
2 . Correlation does not allow us to go beyond the given data. For example, suppose it was found that there was an association between time spent on homework (1/2 hour to 3 hours) and the number of G.C.S.E. passes (1 to 6).
It would not be legitimate to infer from this that spending 6 hours on homework would likely generate 12 G.C.S.E. passes.
How do you know if a study is correlational?
A study is considered correlational if it examines the relationship between two or more variables without manipulating them. In other words, the study does not involve the manipulation of an independent variable to see how it affects a dependent variable.
One way to identify a correlational study is to look for language that suggests a relationship between variables rather than cause and effect.
For example, the study may use phrases like “associated with,” “related to,” or “predicts” when describing the variables being studied.
Another way to identify a correlational study is to look for information about how the variables were measured. Correlational studies typically involve measuring variables using self-report surveys, questionnaires, or other measures of naturally occurring behavior.
Finally, a correlational study may include statistical analyses such as correlation coefficients or regression analyses to examine the strength and direction of the relationship between variables.
Why is a correlational study used?
Correlational studies are particularly useful when it is not possible or ethical to manipulate one of the variables.
For example, it would not be ethical to manipulate someone’s age or gender. However, researchers may still want to understand how these variables relate to outcomes such as health or behavior.
Additionally, correlational studies can be used to generate hypotheses and guide further research.
If a correlational study finds a significant relationship between two variables, this can suggest a possible causal relationship that can be further explored in future research.
What is the goal of correlational research?
The ultimate goal of correlational research is to increase our understanding of how different variables are related and to identify patterns in those relationships.
This information can then be used to generate hypotheses and guide further research aimed at establishing causality.

Related Articles
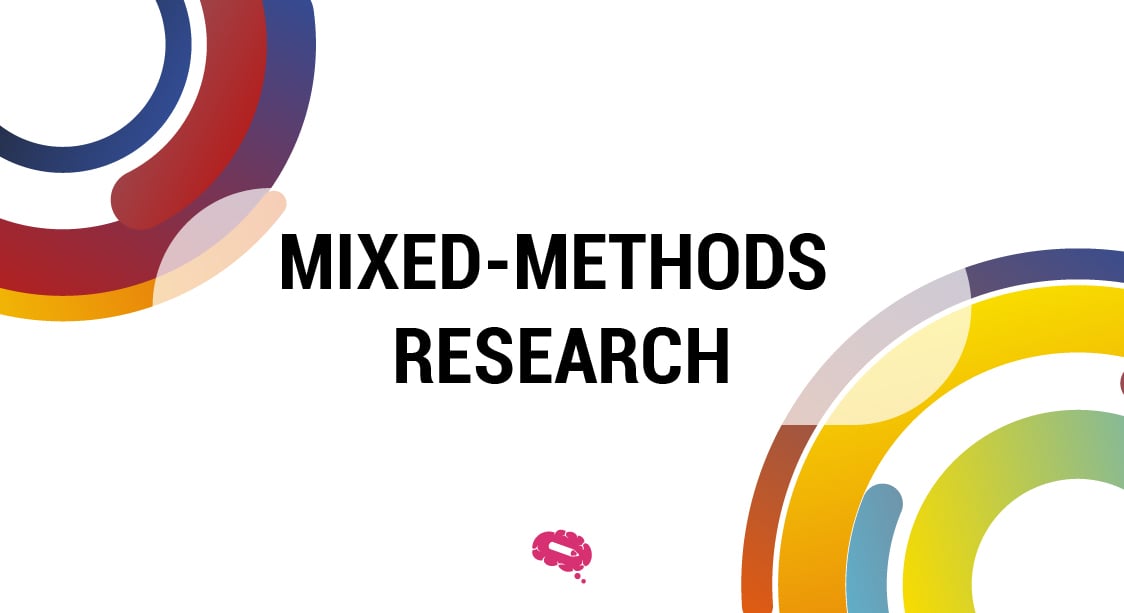
Research Methodology
Mixed Methods Research
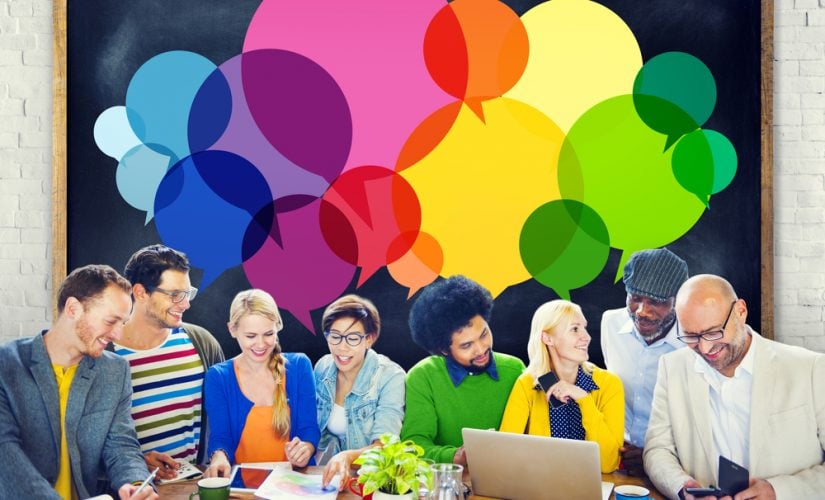
Conversation Analysis
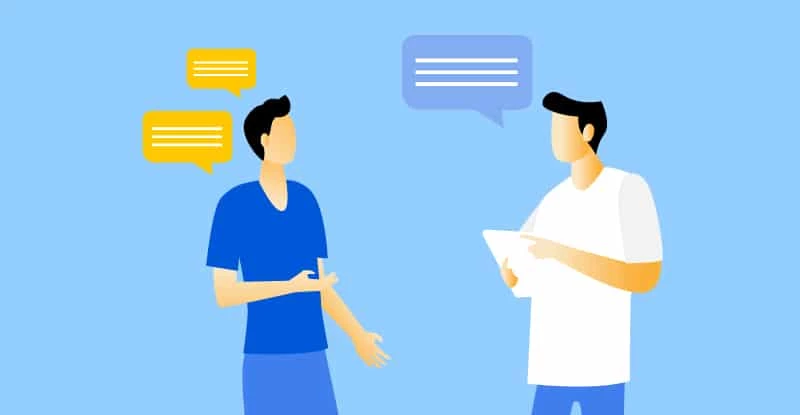
Discourse Analysis
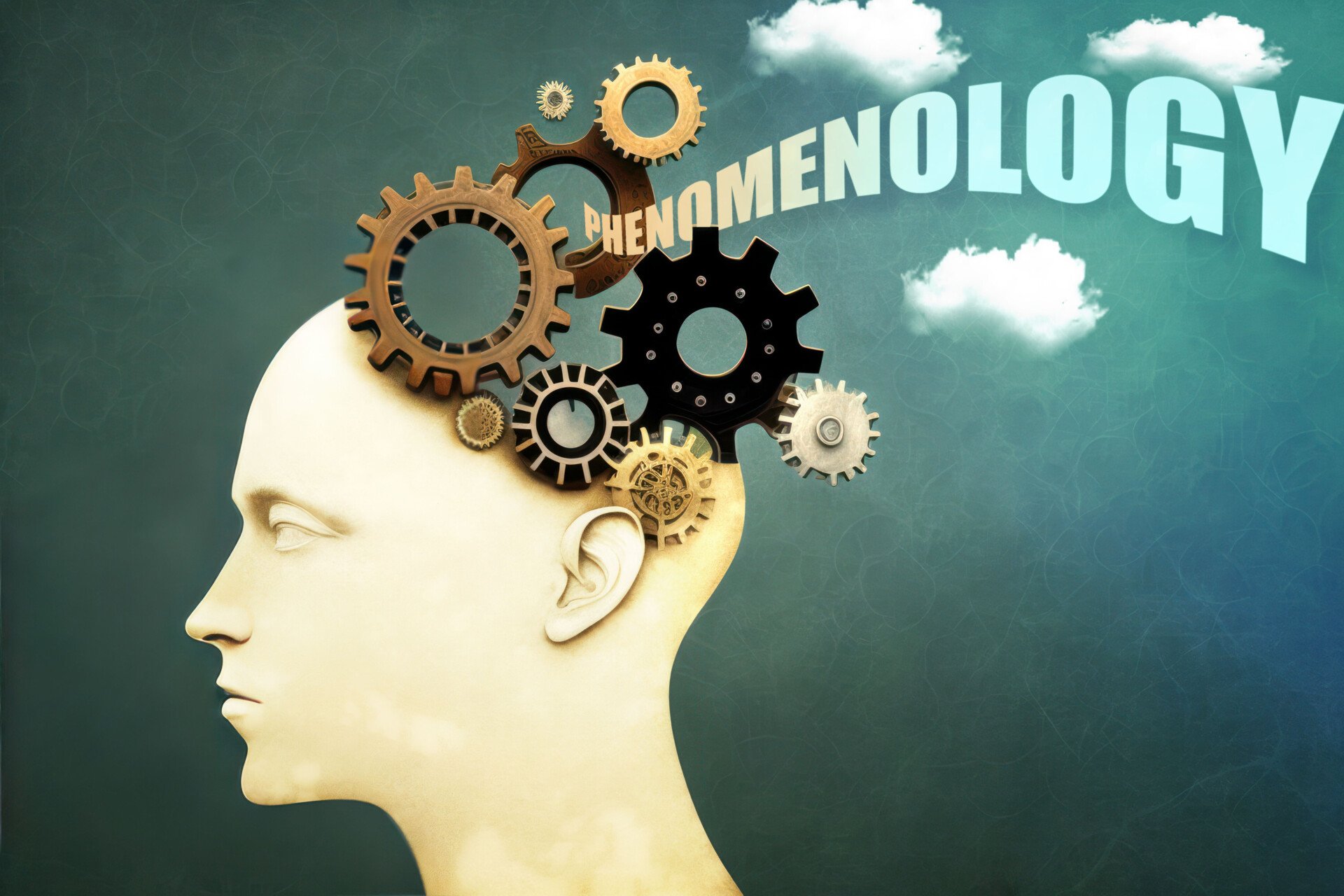
Phenomenology In Qualitative Research
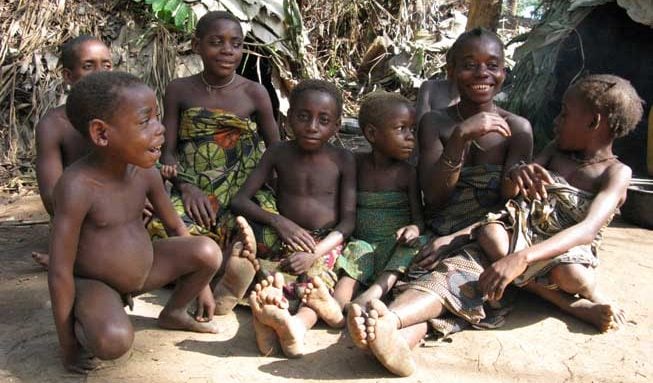
Ethnography In Qualitative Research
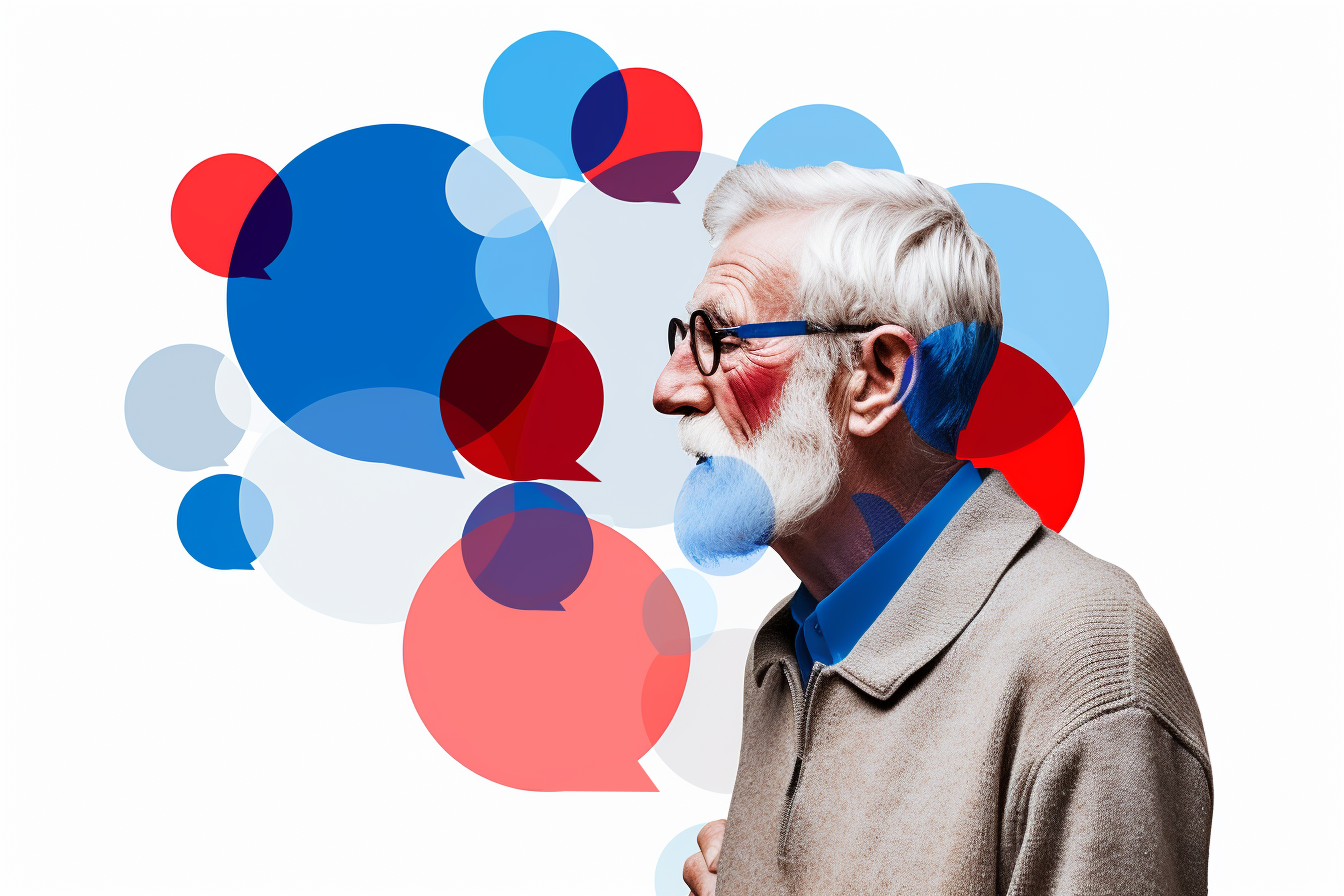
Narrative Analysis In Qualitative Research
- Bipolar Disorder
- Therapy Center
- When To See a Therapist
- Types of Therapy
- Best Online Therapy
- Best Couples Therapy
- Best Family Therapy
- Managing Stress
- Sleep and Dreaming
- Understanding Emotions
- Self-Improvement
- Healthy Relationships
- Student Resources
- Personality Types
- Guided Meditations
- Verywell Mind Insights
- 2024 Verywell Mind 25
- Mental Health in the Classroom
- Editorial Process
- Meet Our Review Board
- Crisis Support
What Is a Correlation?
Kendra Cherry, MS, is a psychosocial rehabilitation specialist, psychology educator, and author of the "Everything Psychology Book."
:max_bytes(150000):strip_icc():format(webp)/IMG_9791-89504ab694d54b66bbd72cb84ffb860e.jpg)
James Lacy, MLS, is a fact-checker and researcher.
:max_bytes(150000):strip_icc():format(webp)/James-Lacy-1000-73de2239670146618c03f8b77f02f84e.jpg)
What Is a Correlation Coefficient?
Scatter plots and correlation, strong vs. weak correlations, correlation does not equal causation, illusory correlations, frequently asked questions.
A correlation means that there is a relationship between two or more variables. This does not imply, however, that there is necessarily a cause or effect relationship between them. Instead, it simply means that there is some type of relationship, meaning they change together at a constant rate.
A correlation coefficient is a number that expresses the strength of the relationship between the two variables.
At a Glance
Correlation can help researchers understand if there is an association between two variables of interest. Such relationships can be positive, meaning they move in the same direction together, or negative, meaning that as one goes up, the other goes down. Correlations can be visualized using scatter plots to show how measurements of a variable change along an x- and y-axis.
It is important to remember that while correlations can help show a relationship, correlation does not indicate causation.
A correlation coefficient, often expressed as r , indicates a measure of the direction and strength of a relationship between two variables. When the r value is closer to +1 or -1, it indicates that there is a stronger linear relationship between the two variables.
Correlational studies are quite common in psychology, particularly because some things are impossible to recreate or research in a lab setting .
Instead of performing an experiment , researchers may collect data to look at possible relationships between variables. From the data they collect and its analysis, researchers then make inferences and predictions about the nature of the relationships between variables.
Helpful Hint
A correlation is a statistical measurement of the relationship between two variables. Remember this handy rule: The closer the correlation is to 0, the weaker it is. The closer it is to +/-1, the stronger it is.
Types of Correlation
Correlation strength ranges from -1 to +1.
Positive Correlation
A correlation of +1 indicates a perfect positive correlation, meaning that both variables move in the same direction together. In other words, +1 is the strong positive correlation you can find.
Negative Correlation
A correlation of –1 indicates a perfect negative correlation, meaning that as one variable goes up, the other goes down.
Zero Correlation
A zero correlation suggests that the correlation statistic does not indicate a relationship between the two variables. This does not mean that there is no relationship at all; it simply means that there is not a linear relationship. A zero correlation is often indicated using the abbreviation r = 0.
Scatter plots (also called scatter charts, scattergrams, and scatter diagrams) are used to plot variables on a chart to observe the associations or relationships between them. The horizontal axis represents one variable, and the vertical axis represents the other.
Investopedia
Each point on the plot is a different measurement. From those measurements, a trend line can be calculated. The correlation coefficient is the slope of that line. When the correlation is weak ( r is close to zero), the line is hard to distinguish. When the correlation is strong ( r is close to 1), the line will be more apparent.
Correlations can be confusing, and many people equate positive with strong and negative with weak. A relationship between two variables can be negative, but that doesn't mean that the relationship isn't strong.
- A weak positive correlation indicates that, although both variables tend to go up in response to one another, the relationship is not very strong.
- A strong negative correlation , on the other hand, indicates a strong connection between the two variables, but that one goes up whenever the other one goes down.
For example, a correlation of -0.97 is a strong negative correlation, whereas a correlation of 0.10 indicates a weak positive correlation. A correlation of +0.10 is weaker than -0.74, and a correlation of -0.98 is stronger than +0.79.
Correlation does not equal causation. Just because two variables have a relationship does not mean that changes in one variable cause changes in the other.
Correlations tell us that there is a relationship between variables, but this does not necessarily mean that one variable causes the other to change.
An oft-cited example is the correlation between ice cream consumption and homicide rates. Studies have found a correlation between increased ice cream sales and spikes in homicides. However, eating ice cream does not cause you to commit murder. Instead, there is a third variable: heat. Both variables increase during summertime .
An illusory correlation is the perception of a relationship between two variables when only a minor relationship—or none at all—actually exists. An illusory correlation does not always mean inferring causation; it can also mean inferring a relationship between two variables when one does not exist.
For example, people sometimes assume that, because two events occurred together at one point in the past, one event must be the cause of the other. These illusory correlations can occur both in scientific investigations and in real-world situations.
Stereotypes are a good example of illusory correlations. Research has shown that people tend to assume that certain groups and traits occur together and frequently overestimate the strength of the association between the two variables.
For example, suppose someone holds the mistaken belief that all people from small towns are extremely kind. When they meet a very kind person, their immediate assumption might be that the person is from a small town, despite the fact that kindness is not related to city population.
What This Means For You
Psychology research frequently uses correlations, but it's essential to understand that correlation is not the same as causation. Confusing correlation with causation assumes a cause-effect relationship that might not exist. While correlation can help you see that there is a relationship (and tell you how strong that relationship is), only experimental research can reveal a causal connection.
You can calculate the correlation coefficient in a few different ways, with the same result. The general formula is r XY =COV XY /(S X S Y ) , which is the covariance between the two variables, divided by the product of their standard deviations:
In the cell in which you want the correlation coefficient to appear, enter =CORREL(A2:A7,B2:B7), where A2:A7 and B2:B7 are the variable lists to compare. Press Enter .
Finding the linear correlation coefficient requires a long, difficult calculation, so most people use a calculator or software such as Excel or a statistics program.
Correlations range from -1.00 to +1.00. The correlation coefficient (expressed as r ) shows the direction and strength of a relationship between two variables. The closer the r value is to +1 or -1, the stronger the linear relationship between the two variables is.
Correlations indicate a relationship between two variables, but one doesn't necessarily cause the other to change.
Mukaka M. A guide to appropriate use of correlation coefficient in medical research . Malawi Med J . 2012;24(3):69-71.
Heath W. Psychology Research Methods: Connecting Research to Students’ Lives . Cambridge University Press.
Chen DT. When correlation does not imply causation: Why your gut microbes may not (yet) be a silver bullet to all your problems . Harvard University.
Association for Psychological Science. Research states that prejudice comes from a basic human need and way of thinking .
Correlation and regression . In: Swinscow TDV. Statistics at Square One . The BMJ.
By Kendra Cherry, MSEd Kendra Cherry, MS, is a psychosocial rehabilitation specialist, psychology educator, and author of the "Everything Psychology Book."
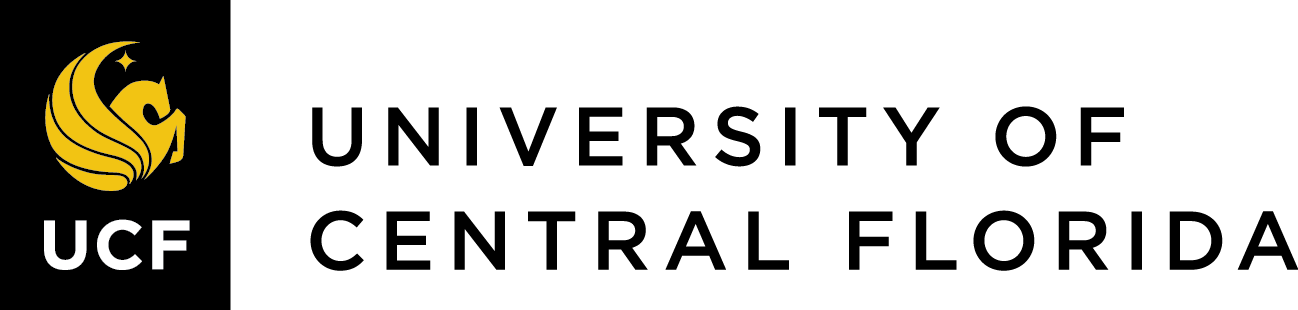
Psychological Research
Correlational Research
Learning objectives.
- Explain what a correlation coefficient tells us about the relationship between variables
- Describe why correlation does not mean causation
Did you know that as sales in ice cream increase, so does the overall rate of crime? Is it possible that indulging in your favorite flavor of ice cream could send you on a crime spree? Or, after committing crime do you think you might decide to treat yourself to a cone? There is no question that a relationship exists between ice cream and crime (e.g., Harper, 2013), but it would be pretty foolish to decide that one thing actually caused the other to occur.
It is much more likely that both ice cream sales and crime rates are related to the temperature outside. When the temperature is warm, there are lots of people out of their houses, interacting with each other, getting annoyed with one another, and sometimes committing crimes. Also, when it is warm outside, we are more likely to seek a cool treat like ice cream. How do we determine if there is indeed a relationship between two things? And when there is a relationship, how can we discern whether it is attributable to coincidence or causation?
Correlation means that there is a relationship between two or more variables (such as ice cream consumption and crime), but this relationship does not necessarily imply cause and effect. When two variables are correlated, it simply means that as one variable changes, so does the other. We can measure correlation by calculating a statistic known as a correlation coefficient. A correlation coefficient is a number from -1 to +1 that indicates the strength and direction of the relationship between variables. The correlation coefficient is usually represented by the letter r .
The number portion of the correlation coefficient indicates the strength of the relationship. The closer the number is to 1 (be it negative or positive), the more strongly related the variables are, and the more predictable changes in one variable will be as the other variable changes. The closer the number is to zero, the weaker the relationship, and the less predictable the relationships between the variables becomes. For instance, a correlation coefficient of 0.9 indicates a far stronger relationship than a correlation coefficient of 0.3. If the variables are not related to one another at all, the correlation coefficient is 0. The example above about ice cream and crime is an example of two variables that we might expect to have no relationship to each other.
The sign—positive or negative—of the correlation coefficient indicates the direction of the relationship (Figure 1). A positive correlation means that the variables move in the same direction. Put another way, it means that as one variable increases so does the other, and conversely, when one variable decreases so does the other. A negative correlation means that the variables move in opposite directions. If two variables are negatively correlated, a decrease in one variable is associated with an increase in the other and vice versa.
The example of ice cream and crime rates is a positive correlation because both variables increase when temperatures are warmer. Other examples of positive correlations are the relationship between an individual’s height and weight or the relationship between a person’s age and number of wrinkles. One might expect a negative correlation to exist between someone’s tiredness during the day and the number of hours they slept the previous night: the amount of sleep decreases as the feelings of tiredness increase. In a real-world example of negative correlation, student researchers at the University of Minnesota found a weak negative correlation ( r = -0.29) between the average number of days per week that students got fewer than 5 hours of sleep and their GPA (Lowry, Dean, & Manders, 2010). Keep in mind that a negative correlation is not the same as no correlation. For example, we would probably find no correlation between hours of sleep and shoe size.
As mentioned earlier, correlations have predictive value. Imagine that you are on the admissions committee of a major university. You are faced with a huge number of applications, but you are able to accommodate only a small percentage of the applicant pool. How might you decide who should be admitted? You might try to correlate your current students’ college GPA with their scores on standardized tests like the SAT or ACT. By observing which correlations were strongest for your current students, you could use this information to predict relative success of those students who have applied for admission into the university.
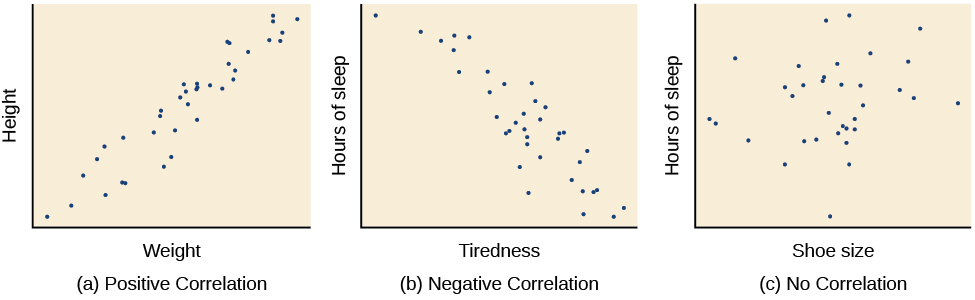
Correlation Does Not Indicate Causation
Correlational research is useful because it allows us to discover the strength and direction of relationships that exist between two variables. However, correlation is limited because establishing the existence of a relationship tells us little about cause and effect . While variables are sometimes correlated because one does cause the other, it could also be that some other factor, a confounding variable , is actually causing the systematic movement in our variables of interest. In the ice cream/crime rate example mentioned earlier, temperature is a confounding variable that could account for the relationship between the two variables.
Even when we cannot point to clear confounding variables, we should not assume that a correlation between two variables implies that one variable causes changes in another. This can be frustrating when a cause-and-effect relationship seems clear and intuitive. Think back to our discussion of the research done by the American Cancer Society and how their research projects were some of the first demonstrations of the link between smoking and cancer. It seems reasonable to assume that smoking causes cancer, but if we were limited to correlational research , we would be overstepping our bounds by making this assumption.
Unfortunately, people mistakenly make claims of causation as a function of correlations all the time. Such claims are especially common in advertisements and news stories. For example, recent research found that people who eat cereal on a regular basis achieve healthier weights than those who rarely eat cereal (Frantzen, Treviño, Echon, Garcia-Dominic, & DiMarco, 2013; Barton et al., 2005). Guess how the cereal companies report this finding. Does eating cereal really cause an individual to maintain a healthy weight, or are there other possible explanations, such as, someone at a healthy weight is more likely to regularly eat a healthy breakfast than someone who is obese or someone who avoids meals in an attempt to diet (Figure 2)? While correlational research is invaluable in identifying relationships among variables, a major limitation is the inability to establish causality. Psychologists want to make statements about cause and effect, but the only way to do that is to conduct an experiment to answer a research question. The next section describes how scientific experiments incorporate methods that eliminate, or control for, alternative explanations, which allow researchers to explore how changes in one variable cause changes in another variable.
Watch this clip from Freakonomics for an example of how correlation does not indicate causation.
You can view the transcript for “Correlation vs. Causality: Freakonomics Movie” here (opens in new window) .
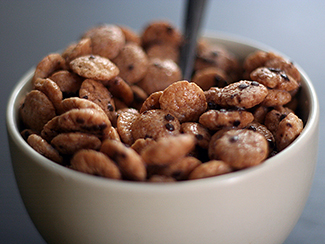
Illusory Correlations
The temptation to make erroneous cause-and-effect statements based on correlational research is not the only way we tend to misinterpret data. We also tend to make the mistake of illusory correlations, especially with unsystematic observations. Illusory correlations , or false correlations, occur when people believe that relationships exist between two things when no such relationship exists. One well-known illusory correlation is the supposed effect that the moon’s phases have on human behavior. Many people passionately assert that human behavior is affected by the phase of the moon, and specifically, that people act strangely when the moon is full (Figure 3).
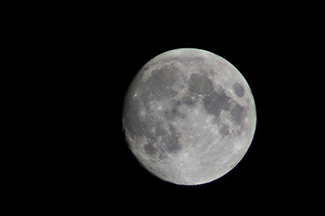
There is no denying that the moon exerts a powerful influence on our planet. The ebb and flow of the ocean’s tides are tightly tied to the gravitational forces of the moon. Many people believe, therefore, that it is logical that we are affected by the moon as well. After all, our bodies are largely made up of water. A meta-analysis of nearly 40 studies consistently demonstrated, however, that the relationship between the moon and our behavior does not exist (Rotton & Kelly, 1985). While we may pay more attention to odd behavior during the full phase of the moon, the rates of odd behavior remain constant throughout the lunar cycle.
Why are we so apt to believe in illusory correlations like this? Often we read or hear about them and simply accept the information as valid. Or, we have a hunch about how something works and then look for evidence to support that hunch, ignoring evidence that would tell us our hunch is false; this is known as confirmation bias . Other times, we find illusory correlations based on the information that comes most easily to mind, even if that information is severely limited. And while we may feel confident that we can use these relationships to better understand and predict the world around us, illusory correlations can have significant drawbacks. For example, research suggests that illusory correlations—in which certain behaviors are inaccurately attributed to certain groups—are involved in the formation of prejudicial attitudes that can ultimately lead to discriminatory behavior (Fiedler, 2004).
Think It Over
CC licensed content, Shared previously
- Analyzing Findings. Authored by : OpenStax College. Located at : https://openstax.org/books/psychology-2e/pages/2-3-analyzing-findings . License : CC BY: Attribution . License Terms : Download for free at https://openstax.org/books/psychology-2e/pages/1-introduction.
All rights reserved content
- Correlation vs. Causality: Freakonomics Movie. Located at : https://www.youtube.com/watch?v=lbODqslc4Tg . License : Other . License Terms : Standard YouTube License
relationship between two or more variables; when two variables are correlated, one variable changes as the other does
number from -1 to +1, indicating the strength and direction of the relationship between variables, and usually represented by r
two variables change in the same direction, both becoming either larger or smaller
two variables change in different directions, with one becoming larger as the other becomes smaller; a negative correlation is not the same thing as no correlation
related to whether we say one variable is causing changes in the other variable, versus other variables that may be related to these two variables.
unanticipated outside factor that affects both variables of interest, often giving the false impression that changes in one variable causes changes in the other variable, when, in actuality, the outside factor causes changes in both variables
changes in one variable cause the changes in the other variable; can be determined only through an experimental research design
seeing relationships between two things when in reality no such relationship exists
seeking out information that supports our stereotypes while ignoring information that is inconsistent with our stereotypes
General Psychology Copyright © by OpenStax and Lumen Learning is licensed under a Creative Commons Attribution 4.0 International License , except where otherwise noted.
Share This Book
6.2 Correlational Research
Learning objectives.
- Define correlational research and give several examples.
- Explain why a researcher might choose to conduct correlational research rather than experimental research or another type of non-experimental research.
- Interpret the strength and direction of different correlation coefficients.
- Explain why correlation does not imply causation.
What Is Correlational Research?
Correlational research is a type of non-experimental research in which the researcher measures two variables and assesses the statistical relationship (i.e., the correlation) between them with little or no effort to control extraneous variables. There are many reasons that researchers interested in statistical relationships between variables would choose to conduct a correlational study rather than an experiment. The first is that they do not believe that the statistical relationship is a causal one or are not interested in causal relationships. Recall two goals of science are to describe and to predict and the correlational research strategy allows researchers to achieve both of these goals. Specifically, this strategy can be used to describe the strength and direction of the relationship between two variables and if there is a relationship between the variables then the researchers can use scores on one variable to predict scores on the other (using a statistical technique called regression).
Another reason that researchers would choose to use a correlational study rather than an experiment is that the statistical relationship of interest is thought to be causal, but the researcher cannot manipulate the independent variable because it is impossible, impractical, or unethical. For example, while I might be interested in the relationship between the frequency people use cannabis and their memory abilities I cannot ethically manipulate the frequency that people use cannabis. As such, I must rely on the correlational research strategy; I must simply measure the frequency that people use cannabis and measure their memory abilities using a standardized test of memory and then determine whether the frequency people use cannabis use is statistically related to memory test performance.
Correlation is also used to establish the reliability and validity of measurements. For example, a researcher might evaluate the validity of a brief extraversion test by administering it to a large group of participants along with a longer extraversion test that has already been shown to be valid. This researcher might then check to see whether participants’ scores on the brief test are strongly correlated with their scores on the longer one. Neither test score is thought to cause the other, so there is no independent variable to manipulate. In fact, the terms independent variable and dependent variabl e do not apply to this kind of research.
Another strength of correlational research is that it is often higher in external validity than experimental research. Recall there is typically a trade-off between internal validity and external validity. As greater controls are added to experiments, internal validity is increased but often at the expense of external validity. In contrast, correlational studies typically have low internal validity because nothing is manipulated or control but they often have high external validity. Since nothing is manipulated or controlled by the experimenter the results are more likely to reflect relationships that exist in the real world.
Finally, extending upon this trade-off between internal and external validity, correlational research can help to provide converging evidence for a theory. If a theory is supported by a true experiment that is high in internal validity as well as by a correlational study that is high in external validity then the researchers can have more confidence in the validity of their theory. As a concrete example, correlational studies establishing that there is a relationship between watching violent television and aggressive behavior have been complemented by experimental studies confirming that the relationship is a causal one (Bushman & Huesmann, 2001) [1] . These converging results provide strong evidence that there is a real relationship (indeed a causal relationship) between watching violent television and aggressive behavior.
Data Collection in Correlational Research
Again, the defining feature of correlational research is that neither variable is manipulated. It does not matter how or where the variables are measured. A researcher could have participants come to a laboratory to complete a computerized backward digit span task and a computerized risky decision-making task and then assess the relationship between participants’ scores on the two tasks. Or a researcher could go to a shopping mall to ask people about their attitudes toward the environment and their shopping habits and then assess the relationship between these two variables. Both of these studies would be correlational because no independent variable is manipulated.
Correlations Between Quantitative Variables
Correlations between quantitative variables are often presented using scatterplots . Figure 6.3 shows some hypothetical data on the relationship between the amount of stress people are under and the number of physical symptoms they have. Each point in the scatterplot represents one person’s score on both variables. For example, the circled point in Figure 6.3 represents a person whose stress score was 10 and who had three physical symptoms. Taking all the points into account, one can see that people under more stress tend to have more physical symptoms. This is a good example of a positive relationship , in which higher scores on one variable tend to be associated with higher scores on the other. A negative relationship is one in which higher scores on one variable tend to be associated with lower scores on the other. There is a negative relationship between stress and immune system functioning, for example, because higher stress is associated with lower immune system functioning.

Figure 6.3 Scatterplot Showing a Hypothetical Positive Relationship Between Stress and Number of Physical Symptoms. The circled point represents a person whose stress score was 10 and who had three physical symptoms. Pearson’s r for these data is +.51.
The strength of a correlation between quantitative variables is typically measured using a statistic called Pearson’s Correlation Coefficient (or Pearson’s r ) . As Figure 6.4 shows, Pearson’s r ranges from −1.00 (the strongest possible negative relationship) to +1.00 (the strongest possible positive relationship). A value of 0 means there is no relationship between the two variables. When Pearson’s r is 0, the points on a scatterplot form a shapeless “cloud.” As its value moves toward −1.00 or +1.00, the points come closer and closer to falling on a single straight line. Correlation coefficients near ±.10 are considered small, values near ± .30 are considered medium, and values near ±.50 are considered large. Notice that the sign of Pearson’s r is unrelated to its strength. Pearson’s r values of +.30 and −.30, for example, are equally strong; it is just that one represents a moderate positive relationship and the other a moderate negative relationship. With the exception of reliability coefficients, most correlations that we find in Psychology are small or moderate in size. The website http://rpsychologist.com/d3/correlation/ , created by Kristoffer Magnusson, provides an excellent interactive visualization of correlations that permits you to adjust the strength and direction of a correlation while witnessing the corresponding changes to the scatterplot.

Figure 6.4 Range of Pearson’s r, From −1.00 (Strongest Possible Negative Relationship), Through 0 (No Relationship), to +1.00 (Strongest Possible Positive Relationship)
There are two common situations in which the value of Pearson’s r can be misleading. Pearson’s r is a good measure only for linear relationships, in which the points are best approximated by a straight line. It is not a good measure for nonlinear relationships, in which the points are better approximated by a curved line. Figure 6.5, for example, shows a hypothetical relationship between the amount of sleep people get per night and their level of depression. In this example, the line that best approximates the points is a curve—a kind of upside-down “U”—because people who get about eight hours of sleep tend to be the least depressed. Those who get too little sleep and those who get too much sleep tend to be more depressed. Even though Figure 6.5 shows a fairly strong relationship between depression and sleep, Pearson’s r would be close to zero because the points in the scatterplot are not well fit by a single straight line. This means that it is important to make a scatterplot and confirm that a relationship is approximately linear before using Pearson’s r . Nonlinear relationships are fairly common in psychology, but measuring their strength is beyond the scope of this book.

Figure 6.5 Hypothetical Nonlinear Relationship Between Sleep and Depression
The other common situations in which the value of Pearson’s r can be misleading is when one or both of the variables have a limited range in the sample relative to the population. This problem is referred to as restriction of range . Assume, for example, that there is a strong negative correlation between people’s age and their enjoyment of hip hop music as shown by the scatterplot in Figure 6.6. Pearson’s r here is −.77. However, if we were to collect data only from 18- to 24-year-olds—represented by the shaded area of Figure 6.6—then the relationship would seem to be quite weak. In fact, Pearson’s r for this restricted range of ages is 0. It is a good idea, therefore, to design studies to avoid restriction of range. For example, if age is one of your primary variables, then you can plan to collect data from people of a wide range of ages. Because restriction of range is not always anticipated or easily avoidable, however, it is good practice to examine your data for possible restriction of range and to interpret Pearson’s r in light of it. (There are also statistical methods to correct Pearson’s r for restriction of range, but they are beyond the scope of this book).

Figure 6.6 Hypothetical Data Showing How a Strong Overall Correlation Can Appear to Be Weak When One Variable Has a Restricted Range.The overall correlation here is −.77, but the correlation for the 18- to 24-year-olds (in the blue box) is 0.
Correlation Does Not Imply Causation
You have probably heard repeatedly that “Correlation does not imply causation.” An amusing example of this comes from a 2012 study that showed a positive correlation (Pearson’s r = 0.79) between the per capita chocolate consumption of a nation and the number of Nobel prizes awarded to citizens of that nation [2] . It seems clear, however, that this does not mean that eating chocolate causes people to win Nobel prizes, and it would not make sense to try to increase the number of Nobel prizes won by recommending that parents feed their children more chocolate.
There are two reasons that correlation does not imply causation. The first is called the directionality problem . Two variables, X and Y , can be statistically related because X causes Y or because Y causes X . Consider, for example, a study showing that whether or not people exercise is statistically related to how happy they are—such that people who exercise are happier on average than people who do not. This statistical relationship is consistent with the idea that exercising causes happiness, but it is also consistent with the idea that happiness causes exercise. Perhaps being happy gives people more energy or leads them to seek opportunities to socialize with others by going to the gym. The second reason that correlation does not imply causation is called the third-variable problem . Two variables, X and Y , can be statistically related not because X causes Y , or because Y causes X , but because some third variable, Z , causes both X and Y . For example, the fact that nations that have won more Nobel prizes tend to have higher chocolate consumption probably reflects geography in that European countries tend to have higher rates of per capita chocolate consumption and invest more in education and technology (once again, per capita) than many other countries in the world. Similarly, the statistical relationship between exercise and happiness could mean that some third variable, such as physical health, causes both of the others. Being physically healthy could cause people to exercise and cause them to be happier. Correlations that are a result of a third-variable are often referred to as spurious correlations.
Some excellent and funny examples of spurious correlations can be found at http://www.tylervigen.com (Figure 6.7 provides one such example).

“Lots of Candy Could Lead to Violence”
Although researchers in psychology know that correlation does not imply causation, many journalists do not. One website about correlation and causation, http://jonathan.mueller.faculty.noctrl.edu/100/correlation_or_causation.htm , links to dozens of media reports about real biomedical and psychological research. Many of the headlines suggest that a causal relationship has been demonstrated when a careful reading of the articles shows that it has not because of the directionality and third-variable problems.
One such article is about a study showing that children who ate candy every day were more likely than other children to be arrested for a violent offense later in life. But could candy really “lead to” violence, as the headline suggests? What alternative explanations can you think of for this statistical relationship? How could the headline be rewritten so that it is not misleading?
As you have learned by reading this book, there are various ways that researchers address the directionality and third-variable problems. The most effective is to conduct an experiment. For example, instead of simply measuring how much people exercise, a researcher could bring people into a laboratory and randomly assign half of them to run on a treadmill for 15 minutes and the rest to sit on a couch for 15 minutes. Although this seems like a minor change to the research design, it is extremely important. Now if the exercisers end up in more positive moods than those who did not exercise, it cannot be because their moods affected how much they exercised (because it was the researcher who determined how much they exercised). Likewise, it cannot be because some third variable (e.g., physical health) affected both how much they exercised and what mood they were in (because, again, it was the researcher who determined how much they exercised). Thus experiments eliminate the directionality and third-variable problems and allow researchers to draw firm conclusions about causal relationships.
Key Takeaways
- Correlational research involves measuring two variables and assessing the relationship between them, with no manipulation of an independent variable.
- Correlation does not imply causation. A statistical relationship between two variables, X and Y , does not necessarily mean that X causes Y . It is also possible that Y causes X , or that a third variable, Z , causes both X and Y .
- While correlational research cannot be used to establish causal relationships between variables, correlational research does allow researchers to achieve many other important objectives (establishing reliability and validity, providing converging evidence, describing relationships and making predictions)
- Correlation coefficients can range from -1 to +1. The sign indicates the direction of the relationship between the variables and the numerical value indicates the strength of the relationship.
- A cognitive psychologist compares the ability of people to recall words that they were instructed to “read” with their ability to recall words that they were instructed to “imagine.”
- A manager studies the correlation between new employees’ college grade point averages and their first-year performance reports.
- An automotive engineer installs different stick shifts in a new car prototype, each time asking several people to rate how comfortable the stick shift feels.
- A food scientist studies the relationship between the temperature inside people’s refrigerators and the amount of bacteria on their food.
- A social psychologist tells some research participants that they need to hurry over to the next building to complete a study. She tells others that they can take their time. Then she observes whether they stop to help a research assistant who is pretending to be hurt.
2. Practice: For each of the following statistical relationships, decide whether the directionality problem is present and think of at least one plausible third variable.
- People who eat more lobster tend to live longer.
- People who exercise more tend to weigh less.
- College students who drink more alcohol tend to have poorer grades.
- Bushman, B. J., & Huesmann, L. R. (2001). Effects of televised violence on aggression. In D. Singer & J. Singer (Eds.), Handbook of children and the media (pp. 223–254). Thousand Oaks, CA: Sage. ↵
- Messerli, F. H. (2012). Chocolate consumption, cognitive function, and Nobel laureates. New England Journal of Medicine, 367 , 1562-1564. ↵

Share This Book
- Increase Font Size
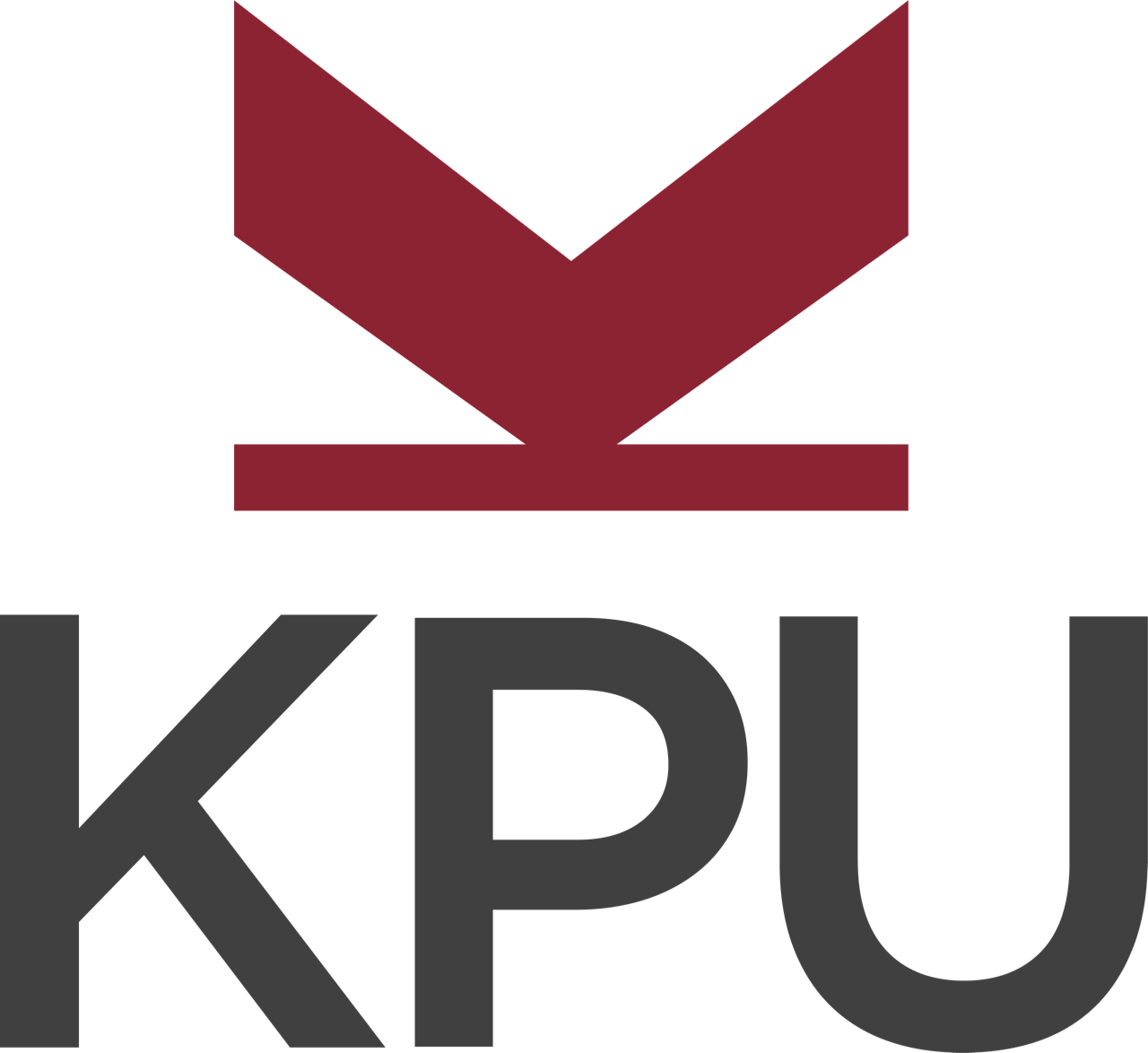
Want to create or adapt books like this? Learn more about how Pressbooks supports open publishing practices.
Non-Experimental Research
29 Correlational Research
Learning objectives.
- Define correlational research and give several examples.
- Explain why a researcher might choose to conduct correlational research rather than experimental research or another type of non-experimental research.
- Interpret the strength and direction of different correlation coefficients.
- Explain why correlation does not imply causation.
What Is Correlational Research?
Correlational research is a type of non-experimental research in which the researcher measures two variables (binary or continuous) and assesses the statistical relationship (i.e., the correlation) between them with little or no effort to control extraneous variables. There are many reasons that researchers interested in statistical relationships between variables would choose to conduct a correlational study rather than an experiment. The first is that they do not believe that the statistical relationship is a causal one or are not interested in causal relationships. Recall two goals of science are to describe and to predict and the correlational research strategy allows researchers to achieve both of these goals. Specifically, this strategy can be used to describe the strength and direction of the relationship between two variables and if there is a relationship between the variables then the researchers can use scores on one variable to predict scores on the other (using a statistical technique called regression, which is discussed further in the section on Complex Correlation in this chapter).
Another reason that researchers would choose to use a correlational study rather than an experiment is that the statistical relationship of interest is thought to be causal, but the researcher cannot manipulate the independent variable because it is impossible, impractical, or unethical. For example, while a researcher might be interested in the relationship between the frequency people use cannabis and their memory abilities they cannot ethically manipulate the frequency that people use cannabis. As such, they must rely on the correlational research strategy; they must simply measure the frequency that people use cannabis and measure their memory abilities using a standardized test of memory and then determine whether the frequency people use cannabis is statistically related to memory test performance.
Correlation is also used to establish the reliability and validity of measurements. For example, a researcher might evaluate the validity of a brief extraversion test by administering it to a large group of participants along with a longer extraversion test that has already been shown to be valid. This researcher might then check to see whether participants’ scores on the brief test are strongly correlated with their scores on the longer one. Neither test score is thought to cause the other, so there is no independent variable to manipulate. In fact, the terms independent variable and dependent variabl e do not apply to this kind of research.
Another strength of correlational research is that it is often higher in external validity than experimental research. Recall there is typically a trade-off between internal validity and external validity. As greater controls are added to experiments, internal validity is increased but often at the expense of external validity as artificial conditions are introduced that do not exist in reality. In contrast, correlational studies typically have low internal validity because nothing is manipulated or controlled but they often have high external validity. Since nothing is manipulated or controlled by the experimenter the results are more likely to reflect relationships that exist in the real world.
Finally, extending upon this trade-off between internal and external validity, correlational research can help to provide converging evidence for a theory. If a theory is supported by a true experiment that is high in internal validity as well as by a correlational study that is high in external validity then the researchers can have more confidence in the validity of their theory. As a concrete example, correlational studies establishing that there is a relationship between watching violent television and aggressive behavior have been complemented by experimental studies confirming that the relationship is a causal one (Bushman & Huesmann, 2001) [1] .
Does Correlational Research Always Involve Quantitative Variables?
A common misconception among beginning researchers is that correlational research must involve two quantitative variables, such as scores on two extraversion tests or the number of daily hassles and number of symptoms people have experienced. However, the defining feature of correlational research is that the two variables are measured—neither one is manipulated—and this is true regardless of whether the variables are quantitative or categorical. Imagine, for example, that a researcher administers the Rosenberg Self-Esteem Scale to 50 American college students and 50 Japanese college students. Although this “feels” like a between-subjects experiment, it is a correlational study because the researcher did not manipulate the students’ nationalities. The same is true of the study by Cacioppo and Petty comparing college faculty and factory workers in terms of their need for cognition. It is a correlational study because the researchers did not manipulate the participants’ occupations.
Figure 6.2 shows data from a hypothetical study on the relationship between whether people make a daily list of things to do (a “to-do list”) and stress. Notice that it is unclear whether this is an experiment or a correlational study because it is unclear whether the independent variable was manipulated. If the researcher randomly assigned some participants to make daily to-do lists and others not to, then it is an experiment. If the researcher simply asked participants whether they made daily to-do lists, then it is a correlational study. The distinction is important because if the study was an experiment, then it could be concluded that making the daily to-do lists reduced participants’ stress. But if it was a correlational study, it could only be concluded that these variables are statistically related. Perhaps being stressed has a negative effect on people’s ability to plan ahead (the directionality problem). Or perhaps people who are more conscientious are more likely to make to-do lists and less likely to be stressed (the third-variable problem). The crucial point is that what defines a study as experimental or correlational is not the variables being studied, nor whether the variables are quantitative or categorical, nor the type of graph or statistics used to analyze the data. What defines a study is how the study is conducted.
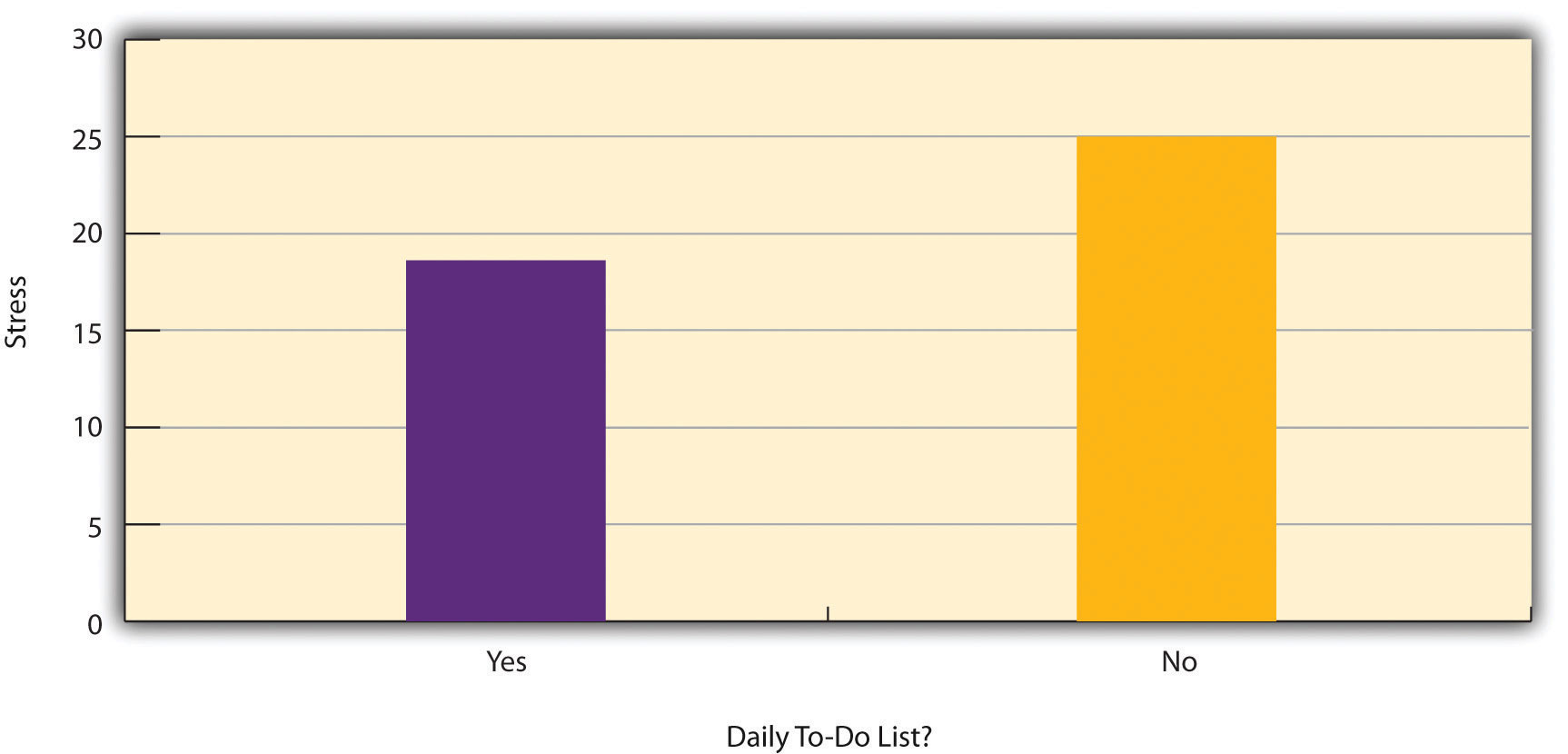
Data Collection in Correlational Research
Again, the defining feature of correlational research is that neither variable is manipulated. It does not matter how or where the variables are measured. A researcher could have participants come to a laboratory to complete a computerized backward digit span task and a computerized risky decision-making task and then assess the relationship between participants’ scores on the two tasks. Or a researcher could go to a shopping mall to ask people about their attitudes toward the environment and their shopping habits and then assess the relationship between these two variables. Both of these studies would be correlational because no independent variable is manipulated.
Correlations Between Quantitative Variables
Correlations between quantitative variables are often presented using scatterplots . Figure 6.3 shows some hypothetical data on the relationship between the amount of stress people are under and the number of physical symptoms they have. Each point in the scatterplot represents one person’s score on both variables. For example, the circled point in Figure 6.3 represents a person whose stress score was 10 and who had three physical symptoms. Taking all the points into account, one can see that people under more stress tend to have more physical symptoms. This is a good example of a positive relationship , in which higher scores on one variable tend to be associated with higher scores on the other. In other words, they move in the same direction, either both up or both down. A negative relationship is one in which higher scores on one variable tend to be associated with lower scores on the other. In other words, they move in opposite directions. There is a negative relationship between stress and immune system functioning, for example, because higher stress is associated with lower immune system functioning.
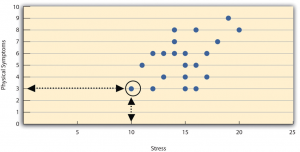
The strength of a correlation between quantitative variables is typically measured using a statistic called Pearson’s Correlation Coefficient (or Pearson's r ) . As Figure 6.4 shows, Pearson’s r ranges from −1.00 (the strongest possible negative relationship) to +1.00 (the strongest possible positive relationship). A value of 0 means there is no relationship between the two variables. When Pearson’s r is 0, the points on a scatterplot form a shapeless “cloud.” As its value moves toward −1.00 or +1.00, the points come closer and closer to falling on a single straight line. Correlation coefficients near ±.10 are considered small, values near ± .30 are considered medium, and values near ±.50 are considered large. Notice that the sign of Pearson’s r is unrelated to its strength. Pearson’s r values of +.30 and −.30, for example, are equally strong; it is just that one represents a moderate positive relationship and the other a moderate negative relationship. With the exception of reliability coefficients, most correlations that we find in Psychology are small or moderate in size. The website http://rpsychologist.com/d3/correlation/ , created by Kristoffer Magnusson, provides an excellent interactive visualization of correlations that permits you to adjust the strength and direction of a correlation while witnessing the corresponding changes to the scatterplot.
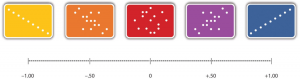
There are two common situations in which the value of Pearson’s r can be misleading. Pearson’s r is a good measure only for linear relationships, in which the points are best approximated by a straight line. It is not a good measure for nonlinear relationships, in which the points are better approximated by a curved line. Figure 6.5, for example, shows a hypothetical relationship between the amount of sleep people get per night and their level of depression. In this example, the line that best approximates the points is a curve—a kind of upside-down “U”—because people who get about eight hours of sleep tend to be the least depressed. Those who get too little sleep and those who get too much sleep tend to be more depressed. Even though Figure 6.5 shows a fairly strong relationship between depression and sleep, Pearson’s r would be close to zero because the points in the scatterplot are not well fit by a single straight line. This means that it is important to make a scatterplot and confirm that a relationship is approximately linear before using Pearson’s r . Nonlinear relationships are fairly common in psychology, but measuring their strength is beyond the scope of this book.
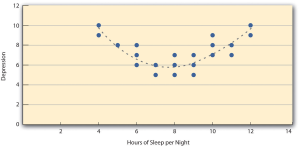
The other common situations in which the value of Pearson’s r can be misleading is when one or both of the variables have a limited range in the sample relative to the population. This problem is referred to as restriction of range . Assume, for example, that there is a strong negative correlation between people’s age and their enjoyment of hip hop music as shown by the scatterplot in Figure 6.6. Pearson’s r here is −.77. However, if we were to collect data only from 18- to 24-year-olds—represented by the shaded area of Figure 6.6—then the relationship would seem to be quite weak. In fact, Pearson’s r for this restricted range of ages is 0. It is a good idea, therefore, to design studies to avoid restriction of range. For example, if age is one of your primary variables, then you can plan to collect data from people of a wide range of ages. Because restriction of range is not always anticipated or easily avoidable, however, it is good practice to examine your data for possible restriction of range and to interpret Pearson’s r in light of it. (There are also statistical methods to correct Pearson’s r for restriction of range, but they are beyond the scope of this book).
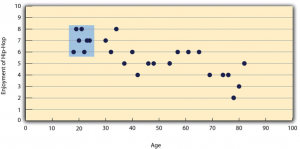
Correlation Does Not Imply Causation
You have probably heard repeatedly that “Correlation does not imply causation.” An amusing example of this comes from a 2012 study that showed a positive correlation (Pearson’s r = 0.79) between the per capita chocolate consumption of a nation and the number of Nobel prizes awarded to citizens of that nation [2] . It seems clear, however, that this does not mean that eating chocolate causes people to win Nobel prizes, and it would not make sense to try to increase the number of Nobel prizes won by recommending that parents feed their children more chocolate.
There are two reasons that correlation does not imply causation. The first is called the directionality problem . Two variables, X and Y , can be statistically related because X causes Y or because Y causes X . Consider, for example, a study showing that whether or not people exercise is statistically related to how happy they are—such that people who exercise are happier on average than people who do not. This statistical relationship is consistent with the idea that exercising causes happiness, but it is also consistent with the idea that happiness causes exercise. Perhaps being happy gives people more energy or leads them to seek opportunities to socialize with others by going to the gym. The second reason that correlation does not imply causation is called the third-variable problem . Two variables, X and Y , can be statistically related not because X causes Y , or because Y causes X , but because some third variable, Z , causes both X and Y . For example, the fact that nations that have won more Nobel prizes tend to have higher chocolate consumption probably reflects geography in that European countries tend to have higher rates of per capita chocolate consumption and invest more in education and technology (once again, per capita) than many other countries in the world. Similarly, the statistical relationship between exercise and happiness could mean that some third variable, such as physical health, causes both of the others. Being physically healthy could cause people to exercise and cause them to be happier. Correlations that are a result of a third-variable are often referred to as spurious correlations .
Some excellent and amusing examples of spurious correlations can be found at http://www.tylervigen.com (Figure 6.7 provides one such example).
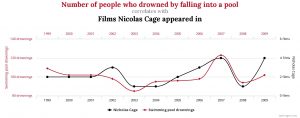
“Lots of Candy Could Lead to Violence”
Although researchers in psychology know that correlation does not imply causation, many journalists do not. One website about correlation and causation, http://jonathan.mueller.faculty.noctrl.edu/100/correlation_or_causation.htm , links to dozens of media reports about real biomedical and psychological research. Many of the headlines suggest that a causal relationship has been demonstrated when a careful reading of the articles shows that it has not because of the directionality and third-variable problems.
One such article is about a study showing that children who ate candy every day were more likely than other children to be arrested for a violent offense later in life. But could candy really “lead to” violence, as the headline suggests? What alternative explanations can you think of for this statistical relationship? How could the headline be rewritten so that it is not misleading?
As you have learned by reading this book, there are various ways that researchers address the directionality and third-variable problems. The most effective is to conduct an experiment. For example, instead of simply measuring how much people exercise, a researcher could bring people into a laboratory and randomly assign half of them to run on a treadmill for 15 minutes and the rest to sit on a couch for 15 minutes. Although this seems like a minor change to the research design, it is extremely important. Now if the exercisers end up in more positive moods than those who did not exercise, it cannot be because their moods affected how much they exercised (because it was the researcher who used random assignment to determine how much they exercised). Likewise, it cannot be because some third variable (e.g., physical health) affected both how much they exercised and what mood they were in. Thus experiments eliminate the directionality and third-variable problems and allow researchers to draw firm conclusions about causal relationships.
Media Attributions
- Nicholas Cage and Pool Drownings © Tyler Viegen is licensed under a CC BY (Attribution) license
- Bushman, B. J., & Huesmann, L. R. (2001). Effects of televised violence on aggression. In D. Singer & J. Singer (Eds.), Handbook of children and the media (pp. 223–254). Thousand Oaks, CA: Sage. ↵
- Messerli, F. H. (2012). Chocolate consumption, cognitive function, and Nobel laureates. New England Journal of Medicine, 367 , 1562-1564. ↵
A graph that presents correlations between two quantitative variables, one on the x-axis and one on the y-axis. Scores are plotted at the intersection of the values on each axis.
A relationship in which higher scores on one variable tend to be associated with higher scores on the other.
A relationship in which higher scores on one variable tend to be associated with lower scores on the other.
A statistic that measures the strength of a correlation between quantitative variables.
When one or both variables have a limited range in the sample relative to the population, making the value of the correlation coefficient misleading.
The problem where two variables, X and Y , are statistically related either because X causes Y, or because Y causes X , and thus the causal direction of the effect cannot be known.
Two variables, X and Y, can be statistically related not because X causes Y, or because Y causes X, but because some third variable, Z, causes both X and Y.
Correlations that are a result not of the two variables being measured, but rather because of a third, unmeasured, variable that affects both of the measured variables.
Research Methods in Psychology Copyright © 2019 by Rajiv S. Jhangiani, I-Chant A. Chiang, Carrie Cuttler, & Dana C. Leighton is licensed under a Creative Commons Attribution-NonCommercial-ShareAlike 4.0 International License , except where otherwise noted.
Share This Book

Positive Correlation
Definition:
A positive correlation refers to a statistical relationship between two variables where an increase in one variable is accompanied by an increase in the other variable. In other words, when the value of one variable goes up, the value of the other variable also tends to go up.
Characteristics of a Positive Correlation:
1. Direct Relationship:
In a positive correlation, the variables move in the same direction. If the value of one variable increases, the value of the other variable also increases. Similarly, if the value of one variable decreases, the value of the other variable also decreases.
2. Scatterplot:
A scatterplot is often used to visually represent a positive correlation. It shows the data points on a graph with one variable plotted on the x-axis and the other variable plotted on the y-axis. The scatterplot typically exhibits an upward trend, indicating a positive relationship between the variables.
3. Correlation Coefficient:
The correlation coefficient is a numerical measure that quantifies the strength and direction of a correlation. In a positive correlation, the correlation coefficient value ranges from 0 to +1. A value close to +1 indicates a strong positive correlation, while a value close to 0 suggests a weak positive correlation.
4. Causation:
A positive correlation does not imply causation. It only indicates that the variables tend to move together. Establishing a cause-and-effect relationship requires further analysis and evidence.
A study is conducted to evaluate the relationship between hours of study and exam scores. The results show a positive correlation between the two variables. Students who study for more hours tend to achieve higher scores, while those who study for fewer hours tend to have lower scores.
In an analysis of income and education levels, it is found that individuals with higher education tend to have higher incomes. This demonstrates a positive correlation – as education level increases, income level also tends to increase.
Using Science to Inform Educational Practices
Correlational Research
Correlation means that there is a relationship between two or more variables (such as ice cream consumption and crime), but this relationship does not necessarily imply cause and effect. When two variables are correlated, it simply means that as one variable changes, so does the other. We can measure correlation by calculating a statistic known as a correlation coefficient. A correlation coefficient is a number from -1 to +1 that indicates the strength and direction of the relationship between variables. The correlation coefficient is usually represented by the letter r .
The number portion of the correlation coefficient indicates the strength of the relationship. The closer the number is to 1 (be it negative or positive), the more strongly related the variables are, and the more predictable changes in one variable will be as the other variable changes. The closer the number is to zero, the weaker the relationship and the less predictable the relationship between the variables becomes. For instance, a correlation coefficient of 0.9 indicates a far stronger relationship than a correlation coefficient of 0.3. If the variables are not related to one another at all, the correlation coefficient is 0. The example above about ice cream and crime is an example of two variables that we might expect to have no relationship to each other.
The sign—positive or negative—of the correlation coefficient indicates the direction of the relationship (Figure 2.2). A positive correlation means that the variables move in the same direction. Put another way, it means that as one variable increases so does the other, and conversely, when one variable decreases so does the other. A negative correlation means that the variables move in opposite directions. If two variables are negatively correlated, a decrease in one variable is associated with an increase in the other and vice versa.
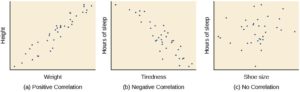
Figure 2.7.1. Scatterplots are a graphical view of the strength and direction of correlations. The stronger the correlation, the closer the data points are to a straight line. In these examples, we see that there is (a) a positive correlation between weight and height, (b) a negative correlation between tiredness and hours of sleep, and (c) no correlation between shoe size and hours of sleep.
The example of ice cream and crime rates is a positive correlation because both variables increase when temperatures are warmer. Other examples of positive correlations are the relationship between an individual’s height and weight or the relationship between a person’s age and number of wrinkles. One might expect a negative correlation to exist between someone’s tiredness during the day and the number of hours they slept the previous night: the amount of sleep decreases as the feelings of tiredness increase. In a real-world example of negative correlation, student researchers at the University of Minnesota found a weak negative correlation ( r = -0.29) between the average number of days per week that students got fewer than 5 hours of sleep and their GPA (Lowry, Dean, & Manders, 2010). Keep in mind that a negative correlation is not the same as no correlation. For example, we would probably find no correlation between hours of sleep and shoe size.
Video 2.7.1. Correlational Research Design provides explanation and examples for correlational research. A closed-captioned version of this video is available here .
Exercise 2.1. Manipulating Scatterplots
Manipulate this interactive scatterplot to practice your understanding of positive and negative correlations.
As mentioned earlier, correlations have predictive value. Imagine that you are on the admissions committee of a major university. You are faced with a massive number of applications, but you are able to accommodate only a small percentage of the applicant pool. How might you decide who should be admitted? You might try to correlate your current students’ college GPA with their scores on standardized tests like the SAT or ACT. By observing which correlations were strongest for your current students, you could use this information to predict the relative success of those students who have applied for admission into the university.
Correlation Does Not Indicate Causation
Correlational research is useful because it allows us to discover the strength and direction of relationships that exist between two variables. However, correlation is limited because establishing the existence of a relationship tells us little about cause and effect. While variables are sometimes correlated because one does cause the other, it could also be that some other factor, a confounding variable, is actually causing the systematic movement in our variables of interest. In the ice cream/crime rate example mentioned earlier, temperature is a confounding variable that could account for the relationship between the two variables.
Even when we cannot point to clear confounding variables, we should not assume that a correlation between two variables implies that one variable causes changes in another. This can be frustrating when a cause-and-effect relationship seems clear and intuitive. Think back to our discussion of the research done by the American Cancer Society and how their research projects were some of the first demonstrations of the link between smoking and cancer. It seems reasonable to assume that smoking causes cancer, but if we were limited to correlational research, we would be overstepping our bounds by making this assumption.
Unfortunately, people mistakenly make claims of causation as a function of correlations all the time. Such claims are especially common in advertisements and news stories. For example, recent research found that people who eat cereal on a regular basis achieve healthier weights than those who rarely eat cereal (Frantzen, Treviño, Echon, Garcia-Dominic, & DiMarco, 2013; Barton et al., 2005). Guess how the cereal companies report this finding. Does eating cereal really cause an individual to maintain a healthy weight, or are there other possible explanations, such as, someone at a healthy weight is more likely to regularly eat a healthy breakfast than someone who is obese or someone who avoids meals in an attempt to diet? While correlational research is invaluable in identifying relationships among variables, a significant limitation is the inability to establish causality. Psychologists want to make statements about cause and effect, but the only way to do that is to conduct an experiment to answer a research question. The next section describes how scientific experiments incorporate methods that eliminate or control for alternative explanations, which allow researchers to explore how changes in one variable cause changes in another variable.
Video 2.7.2. Correlation and Causality provides explanationfor why correlation does not imply causality.
Illusory Correlations
The temptation to make erroneous cause-and-effect statements based on correlational research is not the only way we tend to misinterpret data. We also tend to make the mistake of illusory correlations, especially with unsystematic observations. Illusory correlations , or false correlations, occur when people believe that relationships exist between two things when no such relationship exists. One well-known illusory correlation is the supposed effect that the moon’s phases have on human behavior. Many people passionately assert that human behavior is affected by the phase of the moon, and specifically, that people act strangely when the moon is full (Figure 2).
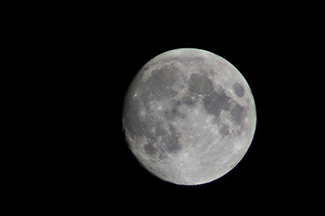
There is no denying that the moon exerts a powerful influence on our planet. The ebb and flow of the ocean’s tides are tightly tied to the gravitational forces of the moon. Many people believe, therefore, that it is logical that we are affected by the moon as well. After all, our bodies are largely made up of water. A meta-analysis of nearly 40 studies consistently demonstrated, however, that the relationship between the moon and our behavior does not exist (Rotton & Kelly, 1985). While we may pay more attention to odd behavior during the full phase of the moon, the rates of odd behavior remain constant throughout the lunar cycle. Why are we so apt to believe in illusory correlations like this? Often we read or hear about them and simply accept the information as valid. Or, we have a hunch about how something works and then look for evidence to support that hunch, ignoring evidence that would tell us our hunch is false; this is known as confirmation bias . Other times, we find illusory correlations based on the information that comes most easily to mind, even if that information is severely limited. And while we may feel confident that we can use these relationships to better understand and predict the world around us, illusory correlations can have significant drawbacks. For example, research suggests that illusory correlations—in which certain behaviors are inaccurately attributed to certain groups—are involved in the formation of prejudicial attitudes that can ultimately lead to discriminatory behavior (Fiedler, 2004).
Candela Citations
- Correlational Research. Authored by : Nicole Arduini-Van Hoose. Provided by : Hudson Valley Community College. Retrieved from : https://courses.lumenlearning.com/edpsy/chapter/correlational-research/. License : CC BY-NC-SA: Attribution-NonCommercial-ShareAlike
- Correlational Research. Authored by : Nicole Arduini-Van Hoose. Provided by : Hudson Valley Community College. Retrieved from : https://courses.lumenlearning.com/adolescent/chapter/correlational-research/. License : CC BY-NC-SA: Attribution-NonCommercial-ShareAlike
Educational Psychology Copyright © 2020 by Nicole Arduini-Van Hoose is licensed under a Creative Commons Attribution-NonCommercial-ShareAlike 4.0 International License , except where otherwise noted.
Share This Book
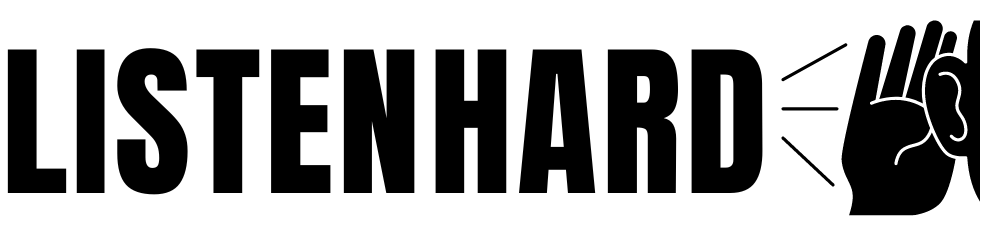
Exploring Positive Correlation in Psychology
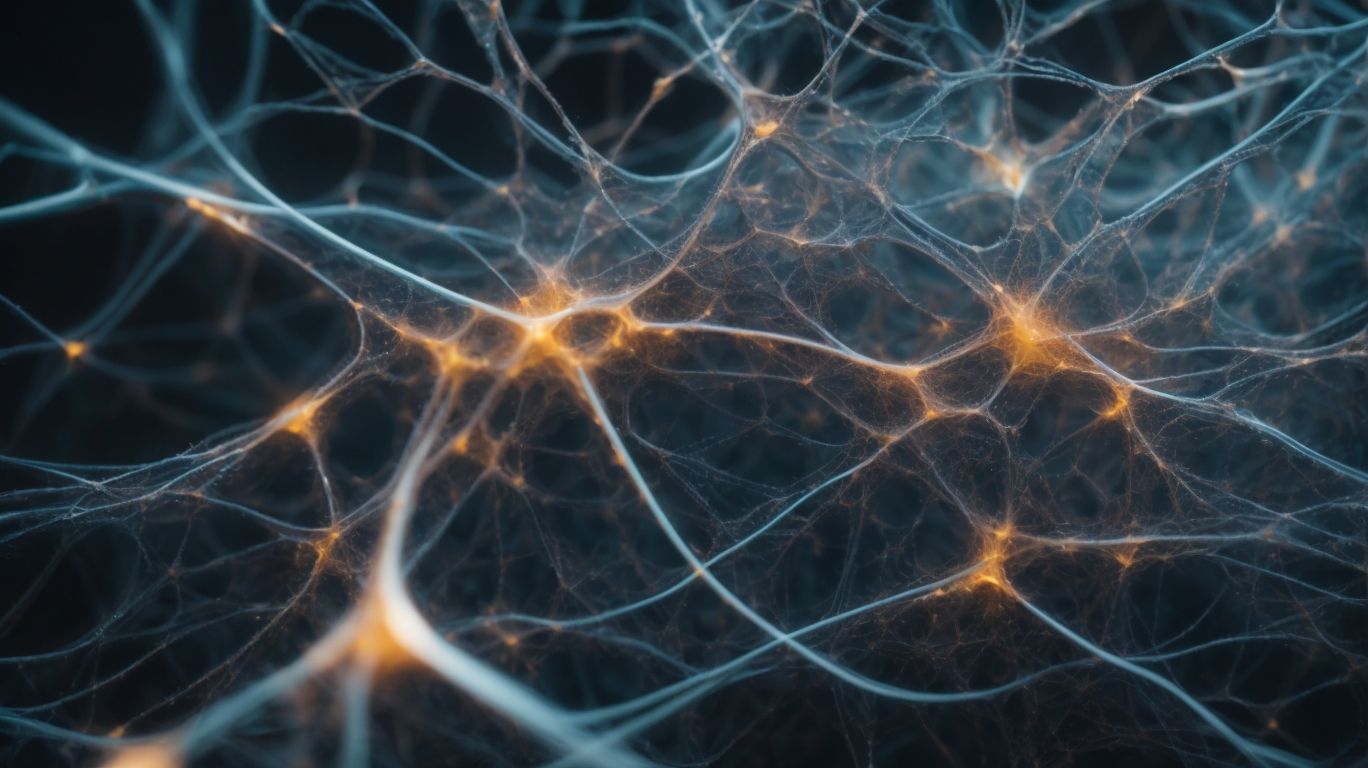
If you’ve ever wondered about the connection between variables in psychology, positive correlation is a key concept to understand. In this article, we will delve into what positive correlation is, how it differs from negative correlation, and how it is measured.
We will also explore the factors that can affect positive correlation, the benefits and limitations of this relationship, and how it is utilized in the field of psychology. So, let’s dive into the world of positive correlation and uncover its significance in psychological research.
- 1 What Is Positive Correlation?
- 2 What Is The Difference Between Positive And Negative Correlation?
- 3 How Is Positive Correlation Measured?
- 4.1 Sample Size
- 4.2 Outliers
- 4.3 Causation vs. Correlation
- 5.1 Predictive Power
- 5.2 Identifying Relationships
- 5.3 Research Purposes
- 6.1 Inaccurate Interpretation
- 6.2 Confounding Variables
- 6.3 Limited Causal Inference
- 7.1 Studying Relationships Between Variables
- 7.2 Identifying Patterns In Data
- 7.3 Predicting Outcomes
- 8.1 What is positive correlation in psychology?
- 8.2 Why is exploring positive correlation important in psychology?
- 8.3 How is positive correlation different from negative correlation in psychology?
- 8.4 Can there be a perfect positive correlation in psychology?
- 8.5 What are some examples of positive correlation in psychology?
- 8.6 How can understanding positive correlation benefit individuals in their daily lives?
What Is Positive Correlation?
Positive correlation refers to a relationship between two variables where they move in the same direction, meaning an increase in one variable corresponds to an increase in the other variable.
For example, a classic illustration of positive correlation is the relationship between ice cream consumption and crime rate. As ice cream sales increase in the summer, so does the crime rate. This doesn’t imply that ice cream causes crime; rather, both variables are influenced by a common factor, such as warmer weather. The strength of a positive correlation is often quantified using the correlation coefficient, which ranges from -1 to 1. A value close to 1 indicates a strong positive correlation.
Understanding correlations is crucial in research and analysis as it helps predict and interpret relationships between variables, enabling knowledge-based decision making and effective strategies.
What Is The Difference Between Positive And Negative Correlation?
The key difference between positive and negative correlation lies in the direction of the relationship they represent; positive correlation indicates that two variables move in the same direction while negative correlation signifies that they move in opposite directions.
For instance, in a scenario of positive correlation, as the amount of rainfall increases, the crop yield also increases. On the other hand, negative correlation can be exemplified by the relationship between exercise time and body weight; as exercise time increases, body weight decreases.
A zero correlation suggests a lack of relationship between two variables, regardless of the nature of changes in one variable and the other. This absence of correlation doesn’t imply lack of significance; it merely indicates that there is no linear relationship between the variables.
The correlation coefficient is a numerical measure that quantifies the strength and direction of the relationship between variables. It ranges from -1 to 1, with the value closer to 1 indicating a strong positive correlation, closer to -1 indicating a strong negative correlation, and a value around 0 suggesting no correlation at all.
Identifying and understanding the different types of relationships, be it positive, negative, or zero correlation, are crucial for proper data analysis. By recognizing these patterns, researchers can draw accurate conclusions and make informed decisions based on the data they are analyzing.
How Is Positive Correlation Measured?
Positive correlation is quantified using a statistical measure known as the correlation coefficient, which provides a numerical value indicating the strength and direction of the relationship between two variables.
When calculating correlation coefficients, values range between -1 and 1. A positive correlation exists when both variables move in the same direction. A coefficient close to 1 suggests a strong positive relationship, signifying that as one variable increases, the other also tends to increase proportionally. Conversely, a coefficient near 0 implies weak or no correlation. It’s important to use statistical tools like regression analysis to accurately determine the degree of association between variables.
What Are The Factors That Affect Positive Correlation?
Several factors can influence the presence and strength of positive correlation between variables, including sample size, outliers, and the distinction between causation and correlation.
In statistical analysis, the sample size plays a crucial role in determining the reliability of correlation results. A larger sample size generally leads to more accurate and representative findings, reducing the likelihood of random fluctuations skewing the correlation.
For instance, when examining the relationship between ice cream consumption and crime rate, a study with a small sample size might show a positive correlation purely by chance. Outliers, on the other hand, are data points that deviate significantly from the overall pattern, potentially misleading the correlation analysis.
If an outlier, such as an exceptionally hot summer affecting both ice cream sales and crime rates, is not properly identified and handled, it can distort the perceived relationship between the variables.
Sample Size
Sample size plays a crucial role in determining the reliability and significance of positive correlation results between variables.
A larger sample size often leads to more robust correlation outcomes as it provides a broader representation of the population under study. When researchers use a small sample size, the results might not accurately reflect the true relationship between variables, leading to potentially misleading conclusions.
For instance, in a study on consumer behavior, a small sample size may not capture the diverse preferences and purchasing patterns of the entire target market, resulting in skewed correlation findings. On the contrary, a representative sample with sufficient size can offer insights that are more generalizable and applicable in real-world scenarios.
Outliers are data points that deviate significantly from the general pattern and can distort the strength and direction of positive correlation between variables.
Identifying outliers is crucial in correlation studies to ensure the accuracy of conclusions drawn from the data. One common method for detecting outliers is through visualization techniques such as scatter plots, where these data points appear as distant from the main cluster. Statistical methods like Z-Score, Tukey’s method, or leverage statistics can also help pinpoint outliers. Once identified, researchers can choose to either remove outliers, transform the data, or use robust statistical measures that are less affected by outliers.
For instance, in a study examining the relationship between income and spending habits, an outlier with exceptionally high income could skew the correlation results, giving a false impression of a stronger relationship between income and spending. By addressing outliers appropriately, researchers can obtain more accurate insights from their correlation analyses.
Causation vs. Correlation
Distinguishing between causation and correlation is crucial when interpreting positive correlation findings, as correlation does not imply causation.
Although two variables can have a strong positive correlation, it does not necessarily mean that one variable causes the other to change. For instance, a study might find a positive correlation between the ice cream sales and drowning incidents during the summer months. While these two variables are correlated, it would be erroneous to conclude that higher ice cream sales cause more drownings.
Researchers must proceed with caution and thoroughly analyze data before inferring causation from correlation. One classic example is the spurious correlation between the divorce rate in Maine and the per capita consumption of margarine. While these two variables had a strong positive correlation, it’s far-fetched to assume that eating margarine affects divorce rates.
What Are The Benefits Of Positive Correlation?
Positive correlation offers several advantages, including predictive power in forecasting outcomes, identifying meaningful relationships between variables, and serving research purposes in various fields.
When there is a positive correlation between two variables, it implies that as one variable increases, the other also tends to increase. This relationship becomes a valuable tool for analysts and researchers as they delve into the realm of predictive modeling. By leveraging this correlation, analysts can use historical data to project future trends accurately.
Identifying significant relationships in data analysis is crucial for drawing meaningful conclusions. Positive correlation helps researchers pinpoint connections that might not be apparent at first glance, enabling them to make informed decisions based on concrete evidence rather than mere speculation.
Across disciplines such as economics, psychology, and philosophy, the utility of positive correlation cannot be understated. In economics, for instance, understanding how different economic factors correlate can assist in developing effective policies and strategies to foster growth and stability.
Predictive Power
The predictive power of positive correlation enables researchers to anticipate trends or outcomes based on the observed relationships between variables.
Positive correlation plays a vital role in enhancing predictive modeling as it indicates that when one variable increases, the other variable also tends to increase. This relationship provides valuable insights into how changes in one variable impact the other, allowing researchers to make more accurate predictions.
For example, in financial markets, a positive correlation between two assets can help investors forecast how changes in one asset’s value may affect the other. By understanding these relationships, researchers can leverage predictive modeling to make informed decisions and mitigate risks effectively.
Identifying Relationships
Positive correlation assists in identifying and understanding meaningful relationships between variables, shedding light on connections that may influence each other.
By recognizing a positive correlation between two variables, data analysts can determine whether an increase in one variable corresponds with an increase in the other, or vice versa. This correlation can highlight patterns and dependencies that might not be immediately apparent, allowing for deeper insights into the underlying mechanisms at play.
For example, in a study on exercise and cardiovascular health, researchers may find a strong positive correlation between the amount of physical activity a person engages in and their overall heart health. This correlation suggests that increasing exercise levels could lead to improvements in cardiovascular fitness.
Research Purposes
Positive correlation serves as a valuable tool for research purposes across disciplines, allowing researchers to explore associations and patterns between variables.
Researchers leverage positive correlation analysis to uncover relationships where an increase in one variable corresponds to an increase in another. In economics, it helps in determining how two economic indicators move together, like the positive correlation between employment rates and consumer spending. In psychology, researchers may use positive correlation to study the relationship between stress levels and health outcomes. In philosophy, positive correlation assists in analyzing how certain philosophical constructs relate to each other in a coherent manner.
What Are The Limitations Of Positive Correlation?
Despite its benefits , positive correlation has limitations such as the potential for inaccurate interpretation, confounding variables affecting results, and restrictions on making causal inferences.
Interpreting positive correlation results inaccurately can lead to erroneous conclusions, as observers may mistakenly assume a causal relationship when none exists. This misinterpretation can have far-reaching consequences, especially in scientific studies or decision-making processes.
Confounding variables, which are external factors that are not taken into account during the analysis, can significantly distort correlation findings. These variables can create a false impression of a direct relationship between the variables being studied, leading to misleading interpretations.
One must be cautious when inferring causation solely based on positive correlation data, as correlation does not imply causation. The common phrase ‘correlation does not imply causation’ underscores the importance of recognizing that a correlation between two variables does not necessarily mean that one causes the other.
Inaccurate Interpretation
One of the limitations of positive correlation is the risk of misinterpreting the results, leading to erroneous conclusions or assumptions about the relationship between variables.
This misinterpretation can occur when individuals mistakenly equate correlation with causation, assuming that just because two variables are correlated, one must cause the other. For example, in a study that shows a positive correlation between ice cream sales and swimming pool accidents, it would be incorrect to conclude that eating ice cream leads to an increase in accidents. This flawed interpretation can have serious consequences, especially in fields like public health or policy-making.
Confounding Variables
Confounding variables pose a significant challenge in positive correlation studies, as they can introduce biases and distort the true relationship between variables.
These variables, often unnoticed or unaccounted for, can lead to incorrect conclusions and flawed interpretations. Researchers must be diligent in identifying potential confounders that could impact their results. One strategy to address this issue is conducting thorough literature reviews to understand previous studies’ findings and potential confounders. Utilizing statistical techniques such as regression analysis can help control for confounding variables by including them as covariates.
Controlling for confounders is crucial for ensuring the validity and reliability of correlation analyses. For example, in a study examining the relationship between coffee consumption and heart health, failing to account for confounding variables like age, exercise habits, or diet could result in a misleadingly strong positive correlation between coffee intake and heart disease risk.
Limited Causal Inference
Positive correlation does not imply causation, limiting the extent to which researchers can infer direct causal relationships between variables based solely on correlation findings.
It is crucial for researchers to remember that correlation simply indicates a relationship between two variables and does not confirm a cause-and-effect relationship. This challenge of making causal inferences from positive correlation data is evident in numerous scientific studies.
For example, in a study that found a positive correlation between ice cream sales and shark attacks, it would be inaccurate to conclude that buying ice cream directly leads to an increase in shark attacks. The confusion between correlation and causation can lead to faulty assumptions and misinterpretations of data.
How Is Positive Correlation Used In Psychology?
Positive correlation plays a vital role in psychology by enabling researchers to study relationships between variables, identify patterns in data, and predict outcomes based on observed correlations.
Psychologists utilize positive correlation when studying behavior and cognition to understand how two variables change together in a systematic manner. For example, in a study examining the relationship between exercise and mood, a positive correlation may show that as physical activity increases, reported feelings of happiness also increase. This helps psychologists make predictions about how certain behaviors or factors impact mental well-being. By establishing positive correlations in research, psychologists can gain valuable insights into the underlying mechanisms of various psychological phenomena.
Studying Relationships Between Variables
Psychologists utilize positive correlation to investigate connections between variables such as memory and educational performance, enabling insights into the underlying mechanisms of behavior.
By analyzing positive correlations, psychologists can uncover patterns that suggest a direct relationship between two or more variables. For example, a study examining the correlation between exercise frequency and stress levels found a positive relationship, indicating that higher exercise frequency was associated with lower stress levels. This type of correlation allows researchers to make predictions and draw conclusions about how changes in one variable may impact another. Understanding positive correlations is essential in psychological research as it helps in establishing the strength and direction of relationships, providing a foundation for further exploration and experimentation.
Identifying Patterns In Data
Positive correlation assists psychologists in identifying consistent patterns in data, allowing for the recognition of trends or relationships that influence psychological processes.
In psychological research, understanding data patterns is crucial for gaining insights into human behavior and cognition. Through correlation analysis, researchers can quantify the strength and direction of relationships between variables, unveiling underlying connections that may not be apparent at first glance.
Correlation is especially useful when studying variables that are interrelated, such as stress levels and academic performance. For example, a study might find a positive correlation between high stress levels and lower academic achievement, indicating a potential relationship between the two factors.
Another case study demonstrating the power of correlation analysis is in the field of addiction research. By examining the correlation between genetic predispositions and substance abuse tendencies, psychologists can better understand the risk factors involved and develop targeted intervention strategies.
The ability to recognize patterns through correlation analysis is invaluable in psychology as it allows researchers to make informed decisions, predict outcomes, and ultimately improve the well-being of individuals through evidence-based practices.
Predicting Outcomes
In psychology, positive correlation aids in predicting future outcomes or behaviors based on the observed relationships between variables, offering insights into potential patterns or trends.
For example, researchers have utilized positive correlation to forecast the impact of stress levels on academic performance among students. By establishing a positive relationship between stress scores and grade point averages, they could predict how changes in stress may affect academic achievement.
In clinical psychology, positive correlation analysis has been instrumental in anticipating treatment outcomes based on patients’ adherence to therapy sessions and their reported progress. This predictive modeling not only helps in assessing individual progress but also contributes to shaping treatment plans to cater to specific needs.
Frequently Asked Questions
What is positive correlation in psychology.
Positive correlation in psychology refers to a relationship between two variables where an increase in one variable is associated with an increase in the other variable. This means that as one variable goes up, the other variable also tends to go up.
Why is exploring positive correlation important in psychology?
Exploring positive correlation is important in psychology because it allows researchers to understand how two variables are related and how changes in one variable can affect the other. This can help in predicting behavior and developing effective interventions or treatments.
How is positive correlation different from negative correlation in psychology?
Positive correlation and negative correlation are two types of relationships between variables. Positive correlation means that as one variable increases, the other variable also tends to increase, while negative correlation means that as one variable increases, the other variable tends to decrease.
Can there be a perfect positive correlation in psychology?
Yes, there can be a perfect positive correlation in psychology. This means that the two variables are perfectly related and as one variable increases, the other variable also increases by a specific amount. However, perfect correlations are rare in real-world research.
What are some examples of positive correlation in psychology?
An example of positive correlation in psychology is the relationship between self-esteem and academic achievement. Research has shown that as self-esteem increases, academic achievement also tends to increase.
How can understanding positive correlation benefit individuals in their daily lives?
Understanding positive correlation can benefit individuals in their daily lives by helping them make better decisions. For example, if someone knows that positive correlation exists between exercise and mood, they may choose to exercise more to improve their mood.
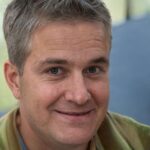
Gabriel Silva is a cultural psychologist interested in how cultural contexts influence individual psychology and vice versa. His fieldwork spans multiple continents, studying the diversity of human experience through the lens of psychology. Gabriel’s writings reflect his journey, offering readers a global perspective on the ways culture shapes our identity, values, and interactions with the world.
Similar Posts
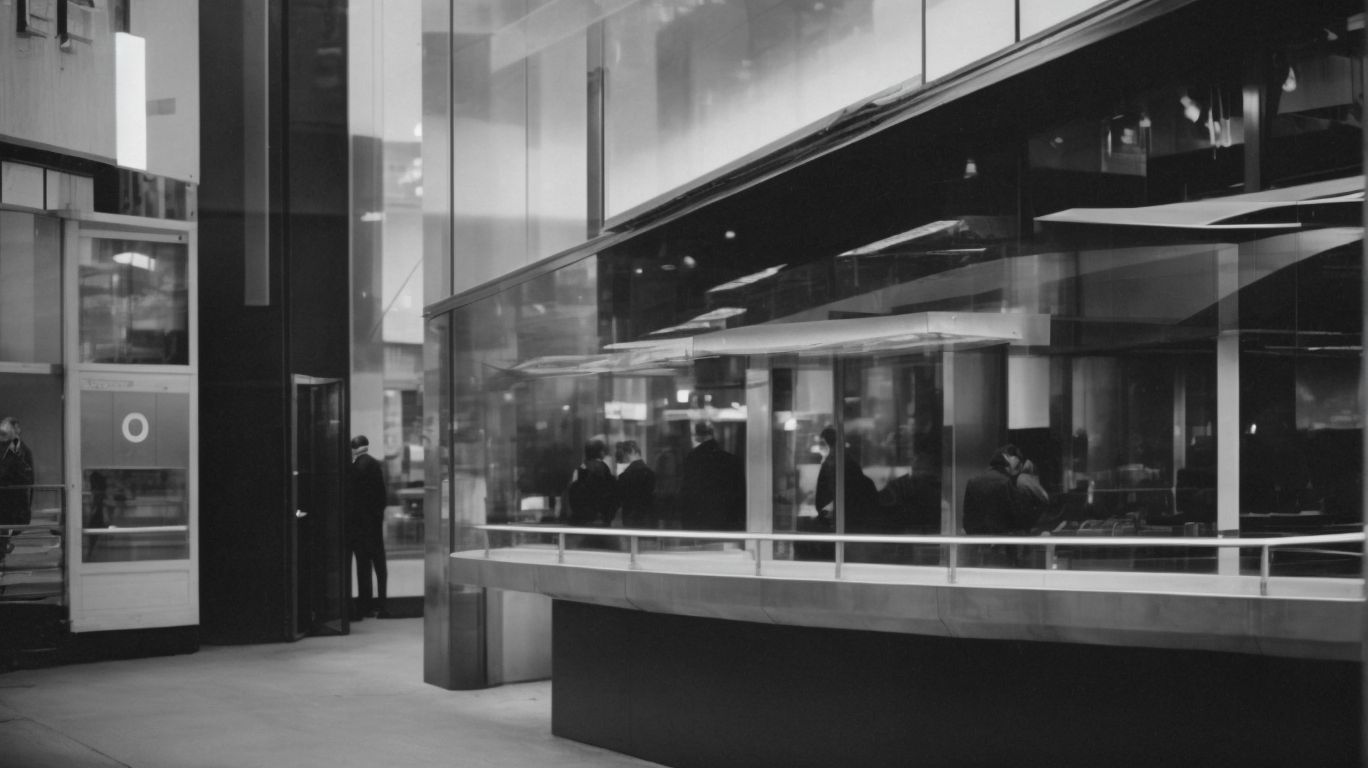
Understanding Self Report in Psychology: Definition and Importance
The article was last updated by Alicia Rhodes on February 4, 2024. Self-report measures are a crucial tool in psychological research, providing valuable insights into…
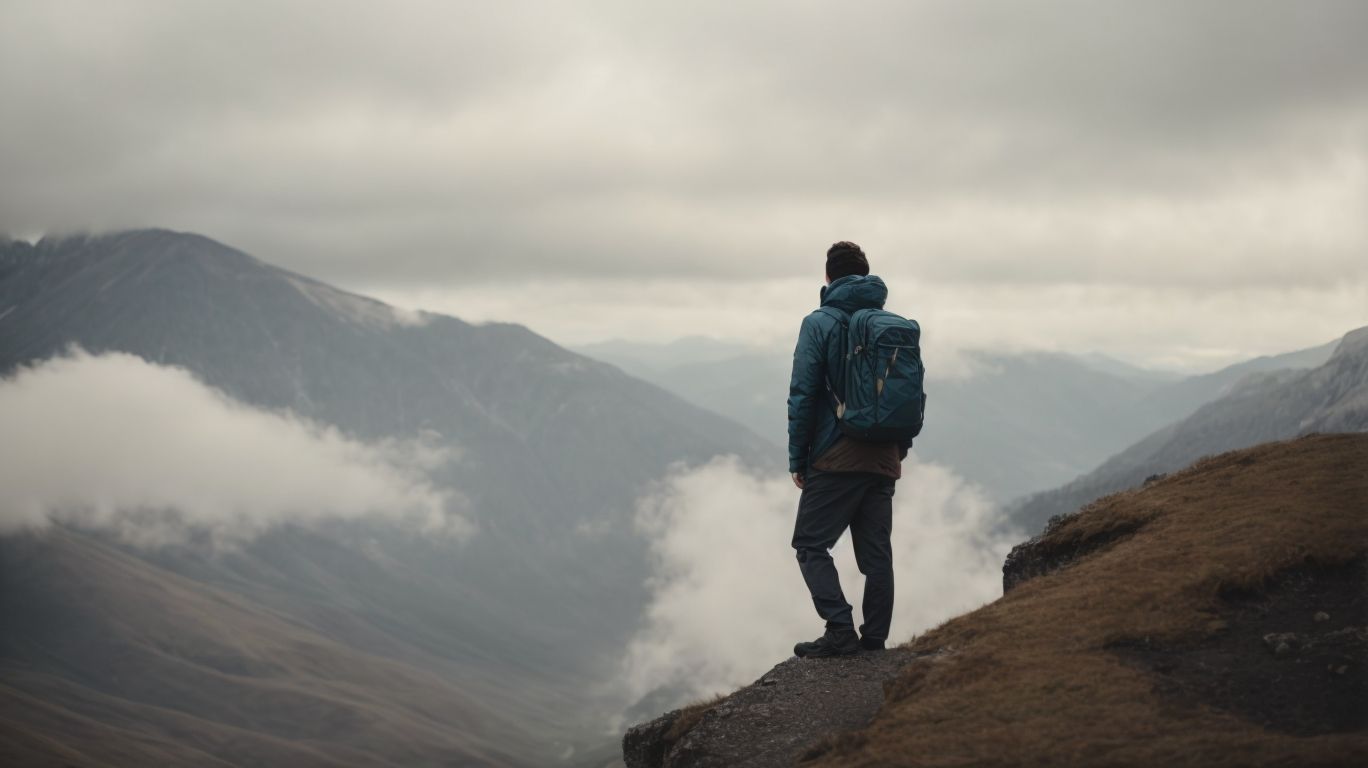
Identifying and Overcoming Limitations in Psychology
The article was last updated by Dr. Henry Foster on February 6, 2024. Understanding the limitations in psychology research is crucial for the accuracy and…
Understanding the Concept of Test Bias in Psychology
The article was last updated by Emily (Editor) on February 5, 2024. Test bias is a crucial concept in the field of psychology, as it…
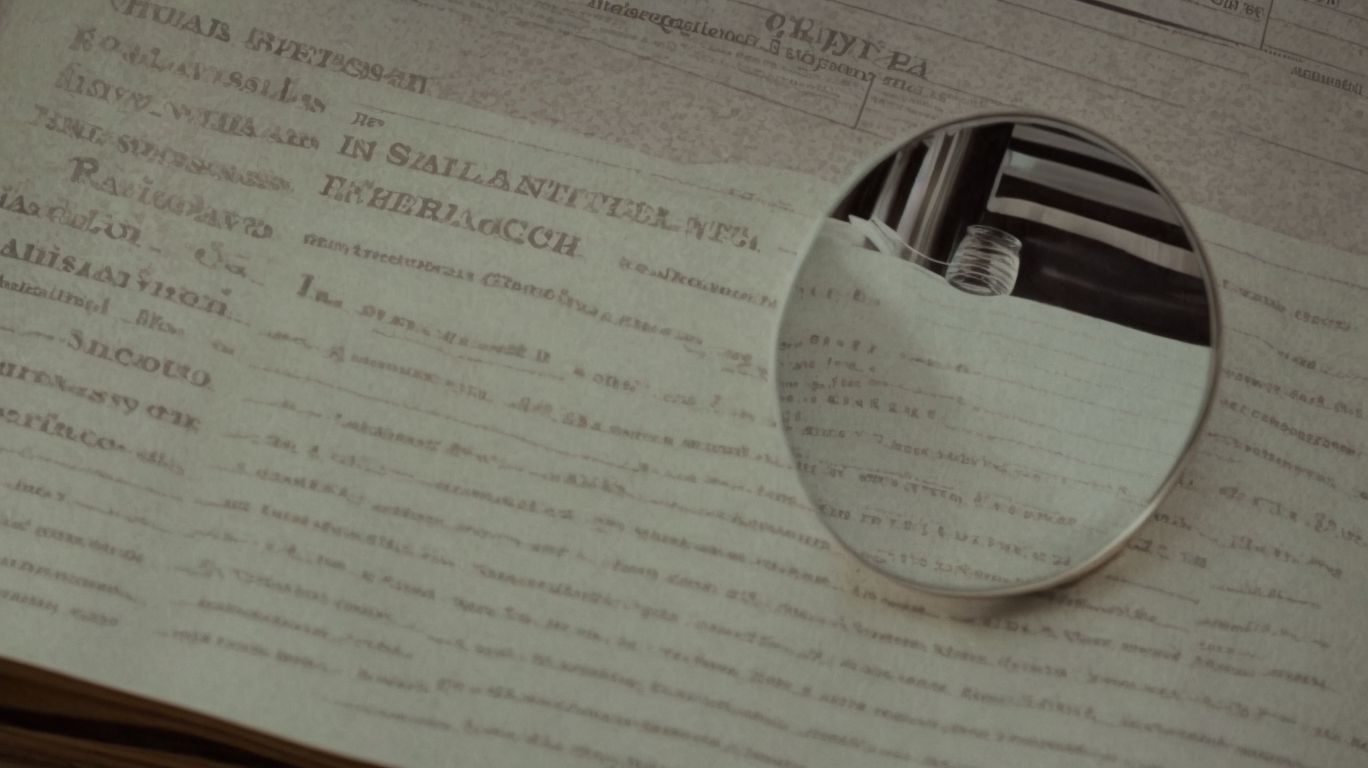
Uncovering the Significance and Applications of Natural Experiments in Psychology
The article was last updated by Ethan Clarke on February 9, 2024. Have you ever wondered how psychologists conduct research in real-life settings to understand…
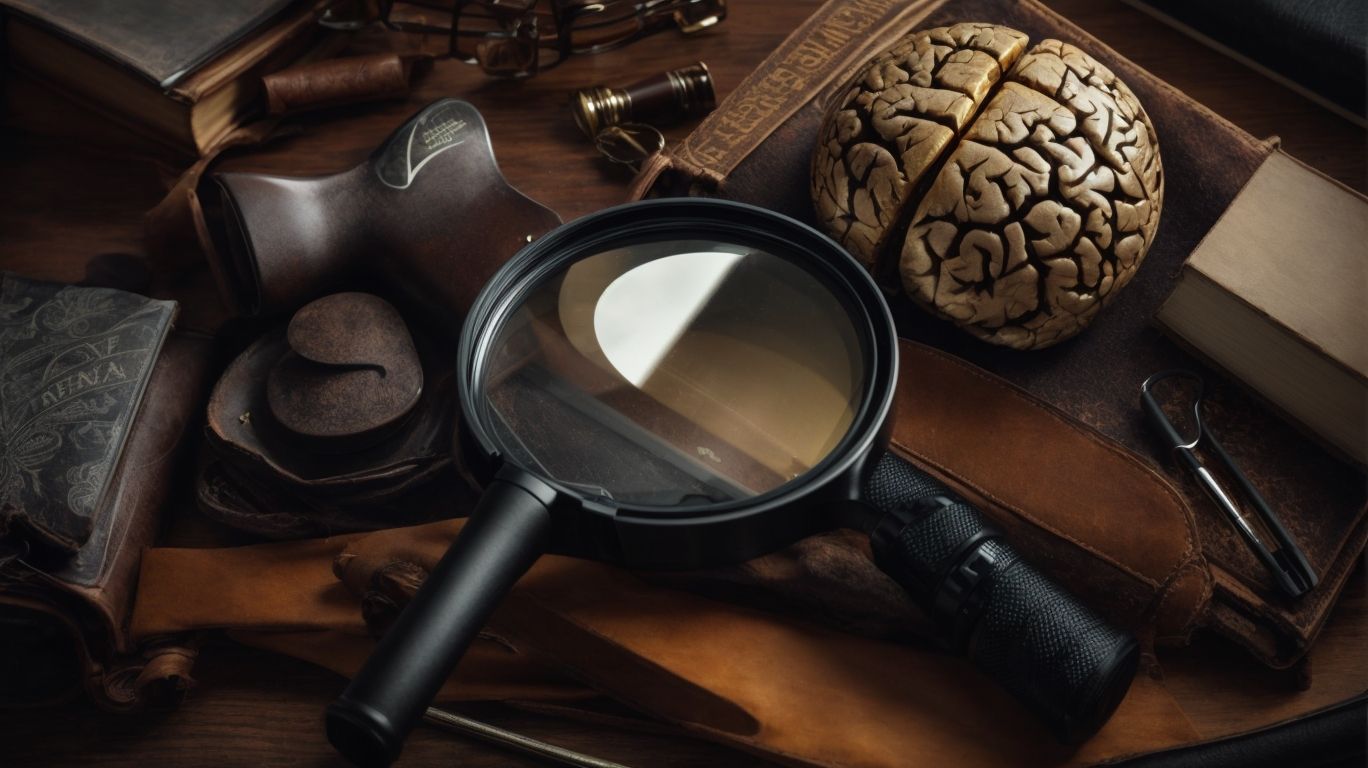
Exploring Tools and Technology in Psychology: A Comprehensive Guide
The article was last updated by Dr. Henry Foster on February 9, 2024. Curious about the world of psychology and how it can benefit you…
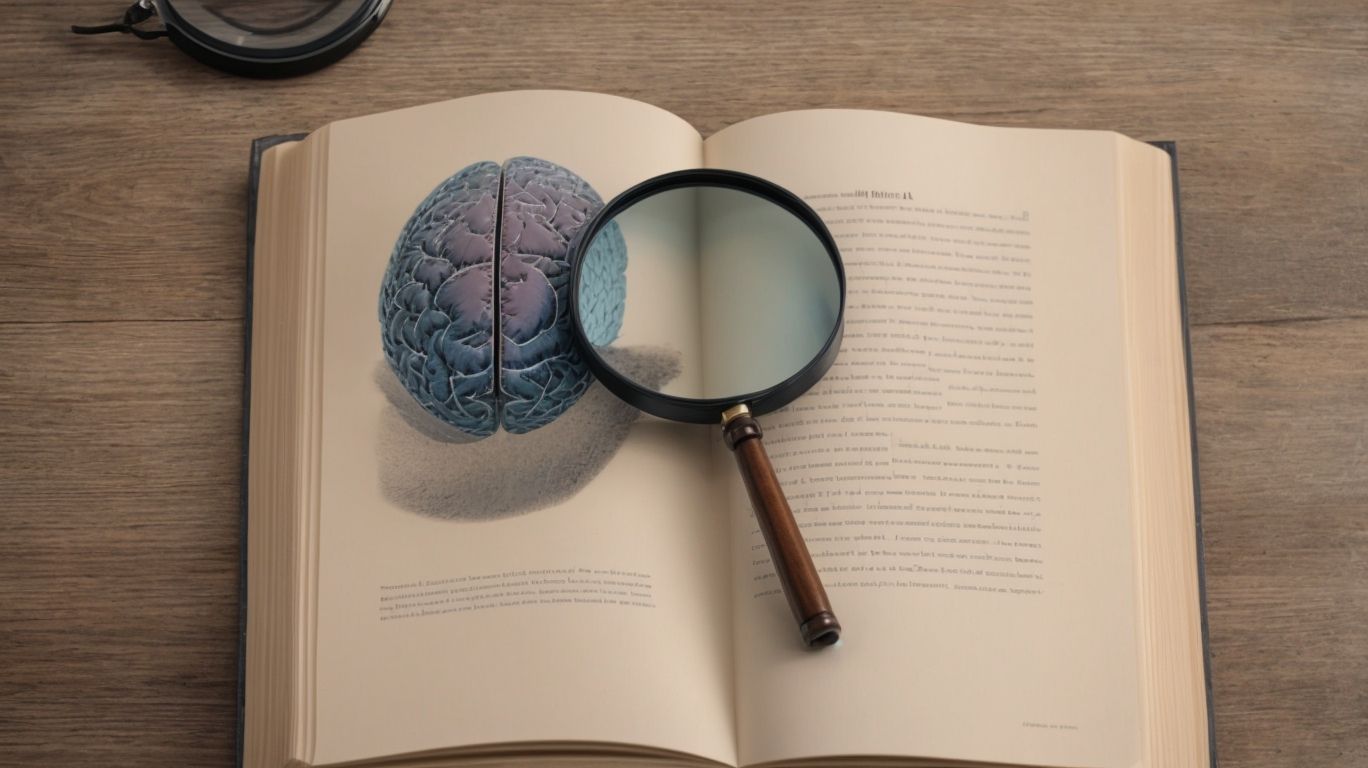
Guide to Designing Psychological Experiments
The article was last updated by Ethan Clarke on February 8, 2024. Are you curious about how psychological experiments are conducted and what their purpose…
2.3 Analyzing Findings
Learning objectives.
By the end of this section, you will be able to:
- Explain what a correlation coefficient tells us about the relationship between variables
- Recognize that correlation does not indicate a cause-and-effect relationship between variables
- Discuss our tendency to look for relationships between variables that do not really exist
- Explain random sampling and assignment of participants into experimental and control groups
- Discuss how experimenter or participant bias could affect the results of an experiment
- Identify independent and dependent variables
Did you know that as sales in ice cream increase, so does the overall rate of crime? Is it possible that indulging in your favorite flavor of ice cream could send you on a crime spree? Or, after committing crime do you think you might decide to treat yourself to a cone? There is no question that a relationship exists between ice cream and crime (e.g., Harper, 2013), but it would be pretty foolish to decide that one thing actually caused the other to occur.
It is much more likely that both ice cream sales and crime rates are related to the temperature outside. When the temperature is warm, there are lots of people out of their houses, interacting with each other, getting annoyed with one another, and sometimes committing crimes. Also, when it is warm outside, we are more likely to seek a cool treat like ice cream. How do we determine if there is indeed a relationship between two things? And when there is a relationship, how can we discern whether it is attributable to coincidence or causation?
Correlational Research
Correlation means that there is a relationship between two or more variables (such as ice cream consumption and crime), but this relationship does not necessarily imply cause and effect. When two variables are correlated, it simply means that as one variable changes, so does the other. We can measure correlation by calculating a statistic known as a correlation coefficient. A correlation coefficient is a number from -1 to +1 that indicates the strength and direction of the relationship between variables. The correlation coefficient is usually represented by the letter r .
The number portion of the correlation coefficient indicates the strength of the relationship. The closer the number is to 1 (be it negative or positive), the more strongly related the variables are, and the more predictable changes in one variable will be as the other variable changes. The closer the number is to zero, the weaker the relationship, and the less predictable the relationship between the variables becomes. For instance, a correlation coefficient of 0.9 indicates a far stronger relationship than a correlation coefficient of 0.3. If the variables are not related to one another at all, the correlation coefficient is 0. The example above about ice cream and crime is an example of two variables that we might expect to have no relationship to each other.
The sign—positive or negative—of the correlation coefficient indicates the direction of the relationship ( Figure 2.12 ). A positive correlation means that the variables move in the same direction. Put another way, it means that as one variable increases so does the other, and conversely, when one variable decreases so does the other. A negative correlation means that the variables move in opposite directions. If two variables are negatively correlated, a decrease in one variable is associated with an increase in the other and vice versa.
The example of ice cream and crime rates is a positive correlation because both variables increase when temperatures are warmer. Other examples of positive correlations are the relationship between an individual’s height and weight or the relationship between a person’s age and number of wrinkles. One might expect a negative correlation to exist between someone’s tiredness during the day and the number of hours they slept the previous night: the amount of sleep decreases as the feelings of tiredness increase. In a real-world example of negative correlation, student researchers at the University of Minnesota found a weak negative correlation ( r = -0.29) between the average number of days per week that students got fewer than 5 hours of sleep and their GPA (Lowry, Dean, & Manders, 2010). Keep in mind that a negative correlation is not the same as no correlation. For example, we would probably find no correlation between hours of sleep and shoe size.
As mentioned earlier, correlations have predictive value. Imagine that you are on the admissions committee of a major university. You are faced with a huge number of applications, but you are able to accommodate only a small percentage of the applicant pool. How might you decide who should be admitted? You might try to correlate your current students’ college GPA with their scores on standardized tests like the SAT or ACT. By observing which correlations were strongest for your current students, you could use this information to predict relative success of those students who have applied for admission into the university.
Link to Learning
Manipulate this interactive scatterplot to practice your understanding of positive and negative correlation.
Correlation Does Not Indicate Causation
Correlational research is useful because it allows us to discover the strength and direction of relationships that exist between two variables. However, correlation is limited because establishing the existence of a relationship tells us little about cause and effect . While variables are sometimes correlated because one does cause the other, it could also be that some other factor, a confounding variable , is actually causing the systematic movement in our variables of interest. In the ice cream/crime rate example mentioned earlier, temperature is a confounding variable that could account for the relationship between the two variables.
Even when we cannot point to clear confounding variables, we should not assume that a correlation between two variables implies that one variable causes changes in another. This can be frustrating when a cause-and-effect relationship seems clear and intuitive. Think back to our discussion of the research done by the American Cancer Society and how their research projects were some of the first demonstrations of the link between smoking and cancer. It seems reasonable to assume that smoking causes cancer, but if we were limited to correlational research , we would be overstepping our bounds by making this assumption.
Unfortunately, people mistakenly make claims of causation as a function of correlations all the time. Such claims are especially common in advertisements and news stories. For example, research found that people who eat certain breakfast cereal may have a reduced risk of heart disease (Anderson, Hanna, Peng, & Kryscio, 2000). Cereal companies are likely to share this information in a way that maximizes and perhaps overstates the positive aspects of eating cereal. But does cereal really cause better health, or are there other possible explanations for the health of those who eat cereal? While correlational research is invaluable in identifying relationships among variables, a major limitation is the inability to establish causality. Psychologists want to make statements about cause and effect, but the only way to do that is to conduct an experiment to answer a research question. The next section describes how scientific experiments incorporate methods that eliminate, or control for, alternative explanations, which allow researchers to explore how changes in one variable cause changes in another variable.
Illusory Correlations
The temptation to make erroneous cause-and-effect statements based on correlational research is not the only way we tend to misinterpret data. We also tend to make the mistake of illusory correlations, especially with unsystematic observations. Illusory correlations , or false correlations, occur when people believe that relationships exist between two things when no such relationship exists. One well-known illusory correlation is the supposed effect that the moon’s phases have on human behavior. Many people passionately assert that human behavior is affected by the phase of the moon, and specifically, that people act strangely when the moon is full ( Figure 2.14 ).
There is no denying that the moon exerts a powerful influence on our planet. The ebb and flow of the ocean’s tides are tightly tied to the gravitational forces of the moon. Many people believe, therefore, that it is logical that we are affected by the moon as well. After all, our bodies are largely made up of water. A meta-analysis of nearly 40 studies consistently demonstrated, however, that the relationship between the moon and our behavior does not exist (Rotton & Kelly, 1985). While we may pay more attention to odd behavior during the full phase of the moon, the rates of odd behavior remain constant throughout the lunar cycle.
Why are we so apt to believe in illusory correlations like this? Often we read or hear about them and simply accept the information as valid. Or, we have a hunch about how something works and then look for evidence to support that hunch, ignoring evidence that would tell us our hunch is false; this is known as confirmation bias . Other times, we find illusory correlations based on the information that comes most easily to mind, even if that information is severely limited. And while we may feel confident that we can use these relationships to better understand and predict the world around us, illusory correlations can have significant drawbacks. For example, research suggests that illusory correlations—in which certain behaviors are inaccurately attributed to certain groups—are involved in the formation of prejudicial attitudes that can ultimately lead to discriminatory behavior (Fiedler, 2004).
Causality: Conducting Experiments and Using the Data
As you’ve learned, the only way to establish that there is a cause-and-effect relationship between two variables is to conduct a scientific experiment . Experiment has a different meaning in the scientific context than in everyday life. In everyday conversation, we often use it to describe trying something for the first time, such as experimenting with a new hair style or a new food. However, in the scientific context, an experiment has precise requirements for design and implementation.
The Experimental Hypothesis
In order to conduct an experiment, a researcher must have a specific hypothesis to be tested. As you’ve learned, hypotheses can be formulated either through direct observation of the real world or after careful review of previous research. For example, if you think that the use of technology in the classroom has negative impacts on learning, then you have basically formulated a hypothesis—namely, that the use of technology in the classroom should be limited because it decreases learning. How might you have arrived at this particular hypothesis? You may have noticed that your classmates who take notes on their laptops perform at lower levels on class exams than those who take notes by hand, or those who receive a lesson via a computer program versus via an in-person teacher have different levels of performance when tested ( Figure 2.15 ).
These sorts of personal observations are what often lead us to formulate a specific hypothesis, but we cannot use limited personal observations and anecdotal evidence to rigorously test our hypothesis. Instead, to find out if real-world data supports our hypothesis, we have to conduct an experiment.
Designing an Experiment
The most basic experimental design involves two groups: the experimental group and the control group. The two groups are designed to be the same except for one difference— experimental manipulation. The experimental group gets the experimental manipulation—that is, the treatment or variable being tested (in this case, the use of technology)—and the control group does not. Since experimental manipulation is the only difference between the experimental and control groups, we can be sure that any differences between the two are due to experimental manipulation rather than chance.
In our example of how the use of technology should be limited in the classroom, we have the experimental group learn algebra using a computer program and then test their learning. We measure the learning in our control group after they are taught algebra by a teacher in a traditional classroom. It is important for the control group to be treated similarly to the experimental group, with the exception that the control group does not receive the experimental manipulation.
We also need to precisely define, or operationalize, how we measure learning of algebra. An operational definition is a precise description of our variables, and it is important in allowing others to understand exactly how and what a researcher measures in a particular experiment. In operationalizing learning, we might choose to look at performance on a test covering the material on which the individuals were taught by the teacher or the computer program. We might also ask our participants to summarize the information that was just presented in some way. Whatever we determine, it is important that we operationalize learning in such a way that anyone who hears about our study for the first time knows exactly what we mean by learning. This aids peoples’ ability to interpret our data as well as their capacity to repeat our experiment should they choose to do so.
Once we have operationalized what is considered use of technology and what is considered learning in our experiment participants, we need to establish how we will run our experiment. In this case, we might have participants spend 45 minutes learning algebra (either through a computer program or with an in-person math teacher) and then give them a test on the material covered during the 45 minutes.
Ideally, the people who score the tests are unaware of who was assigned to the experimental or control group, in order to control for experimenter bias. Experimenter bias refers to the possibility that a researcher’s expectations might skew the results of the study. Remember, conducting an experiment requires a lot of planning, and the people involved in the research project have a vested interest in supporting their hypotheses. If the observers knew which child was in which group, it might influence how they interpret ambiguous responses, such as sloppy handwriting or minor computational mistakes. By being blind to which child is in which group, we protect against those biases. This situation is a single-blind study , meaning that one of the groups (participants) are unaware as to which group they are in (experiment or control group) while the researcher who developed the experiment knows which participants are in each group.
In a double-blind study , both the researchers and the participants are blind to group assignments. Why would a researcher want to run a study where no one knows who is in which group? Because by doing so, we can control for both experimenter and participant expectations. If you are familiar with the phrase placebo effect , you already have some idea as to why this is an important consideration. The placebo effect occurs when people's expectations or beliefs influence or determine their experience in a given situation. In other words, simply expecting something to happen can actually make it happen.
The placebo effect is commonly described in terms of testing the effectiveness of a new medication. Imagine that you work in a pharmaceutical company, and you think you have a new drug that is effective in treating depression. To demonstrate that your medication is effective, you run an experiment with two groups: The experimental group receives the medication, and the control group does not. But you don’t want participants to know whether they received the drug or not.
Why is that? Imagine that you are a participant in this study, and you have just taken a pill that you think will improve your mood. Because you expect the pill to have an effect, you might feel better simply because you took the pill and not because of any drug actually contained in the pill—this is the placebo effect.
To make sure that any effects on mood are due to the drug and not due to expectations, the control group receives a placebo (in this case a sugar pill). Now everyone gets a pill, and once again neither the researcher nor the experimental participants know who got the drug and who got the sugar pill. Any differences in mood between the experimental and control groups can now be attributed to the drug itself rather than to experimenter bias or participant expectations ( Figure 2.16 ).
Independent and Dependent Variables
In a research experiment, we strive to study whether changes in one thing cause changes in another. To achieve this, we must pay attention to two important variables, or things that can be changed, in any experimental study: the independent variable and the dependent variable. An independent variable is manipulated or controlled by the experimenter. In a well-designed experimental study, the independent variable is the only important difference between the experimental and control groups. In our example of how technology use in the classroom affects learning, the independent variable is the type of learning by participants in the study ( Figure 2.17 ). A dependent variable is what the researcher measures to see how much effect the independent variable had. In our example, the dependent variable is the learning exhibited by our participants.
We expect that the dependent variable will change as a function of the independent variable. In other words, the dependent variable depends on the independent variable. A good way to think about the relationship between the independent and dependent variables is with this question: What effect does the independent variable have on the dependent variable? Returning to our example, what is the effect of being taught a lesson through a computer program versus through an in-person instructor?
Selecting and Assigning Experimental Participants
Now that our study is designed, we need to obtain a sample of individuals to include in our experiment. Our study involves human participants so we need to determine whom to include. Participants are the subjects of psychological research, and as the name implies, individuals who are involved in psychological research actively participate in the process. Often, psychological research projects rely on college students to serve as participants. In fact, the vast majority of research in psychology subfields has historically involved students as research participants (Sears, 1986; Arnett, 2008). But are college students truly representative of the general population? College students tend to be younger, more educated, more liberal, and less diverse than the general population. Although using students as test subjects is an accepted practice, relying on such a limited pool of research participants can be problematic because it is difficult to generalize findings to the larger population.
Our hypothetical experiment involves high school students, and we must first generate a sample of students. Samples are used because populations are usually too large to reasonably involve every member in our particular experiment ( Figure 2.18 ). If possible, we should use a random sample (there are other types of samples, but for the purposes of this chapter, we will focus on random samples). A random sample is a subset of a larger population in which every member of the population has an equal chance of being selected. Random samples are preferred because if the sample is large enough we can be reasonably sure that the participating individuals are representative of the larger population. This means that the percentages of characteristics in the sample—sex, ethnicity, socioeconomic level, and any other characteristics that might affect the results—are close to those percentages in the larger population.
In our example, let’s say we decide our population of interest is algebra students. But all algebra students is a very large population, so we need to be more specific; instead we might say our population of interest is all algebra students in a particular city. We should include students from various income brackets, family situations, races, ethnicities, religions, and geographic areas of town. With this more manageable population, we can work with the local schools in selecting a random sample of around 200 algebra students who we want to participate in our experiment.
In summary, because we cannot test all of the algebra students in a city, we want to find a group of about 200 that reflects the composition of that city. With a representative group, we can generalize our findings to the larger population without fear of our sample being biased in some way.
Now that we have a sample, the next step of the experimental process is to split the participants into experimental and control groups through random assignment. With random assignment , all participants have an equal chance of being assigned to either group. There is statistical software that will randomly assign each of the algebra students in the sample to either the experimental or the control group.
Random assignment is critical for sound experimental design . With sufficiently large samples, random assignment makes it unlikely that there are systematic differences between the groups. So, for instance, it would be very unlikely that we would get one group composed entirely of males, a given ethnic identity, or a given religious ideology. This is important because if the groups were systematically different before the experiment began, we would not know the origin of any differences we find between the groups: Were the differences preexisting, or were they caused by manipulation of the independent variable? Random assignment allows us to assume that any differences observed between experimental and control groups result from the manipulation of the independent variable.
Use this online random number generator to learn more about random sampling and assignments.
Issues to Consider
While experiments allow scientists to make cause-and-effect claims, they are not without problems. True experiments require the experimenter to manipulate an independent variable, and that can complicate many questions that psychologists might want to address. For instance, imagine that you want to know what effect sex (the independent variable) has on spatial memory (the dependent variable). Although you can certainly look for differences between males and females on a task that taps into spatial memory, you cannot directly control a person’s sex. We categorize this type of research approach as quasi-experimental and recognize that we cannot make cause-and-effect claims in these circumstances.
Experimenters are also limited by ethical constraints. For instance, you would not be able to conduct an experiment designed to determine if experiencing abuse as a child leads to lower levels of self-esteem among adults. To conduct such an experiment, you would need to randomly assign some experimental participants to a group that receives abuse, and that experiment would be unethical.
Interpreting Experimental Findings
Once data is collected from both the experimental and the control groups, a statistical analysis is conducted to find out if there are meaningful differences between the two groups. A statistical analysis determines how likely it is that any difference found is due to chance (and thus not meaningful). For example, if an experiment is done on the effectiveness of a nutritional supplement, and those taking a placebo pill (and not the supplement) have the same result as those taking the supplement, then the experiment has shown that the nutritional supplement is not effective. Generally, psychologists consider differences to be statistically significant if there is less than a five percent chance of observing them if the groups did not actually differ from one another. Stated another way, psychologists want to limit the chances of making “false positive” claims to five percent or less.
The greatest strength of experiments is the ability to assert that any significant differences in the findings are caused by the independent variable. This occurs because random selection, random assignment, and a design that limits the effects of both experimenter bias and participant expectancy should create groups that are similar in composition and treatment. Therefore, any difference between the groups is attributable to the independent variable, and now we can finally make a causal statement. If we find that watching a violent television program results in more violent behavior than watching a nonviolent program, we can safely say that watching violent television programs causes an increase in the display of violent behavior.
Reporting Research
When psychologists complete a research project, they generally want to share their findings with other scientists. The American Psychological Association (APA) publishes a manual detailing how to write a paper for submission to scientific journals. Unlike an article that might be published in a magazine like Psychology Today, which targets a general audience with an interest in psychology, scientific journals generally publish peer-reviewed journal articles aimed at an audience of professionals and scholars who are actively involved in research themselves.
The Online Writing Lab (OWL) at Purdue University can walk you through the APA writing guidelines.
A peer-reviewed journal article is read by several other scientists (generally anonymously) with expertise in the subject matter. These peer reviewers provide feedback—to both the author and the journal editor—regarding the quality of the draft. Peer reviewers look for a strong rationale for the research being described, a clear description of how the research was conducted, and evidence that the research was conducted in an ethical manner. They also look for flaws in the study's design, methods, and statistical analyses. They check that the conclusions drawn by the authors seem reasonable given the observations made during the research. Peer reviewers also comment on how valuable the research is in advancing the discipline’s knowledge. This helps prevent unnecessary duplication of research findings in the scientific literature and, to some extent, ensures that each research article provides new information. Ultimately, the journal editor will compile all of the peer reviewer feedback and determine whether the article will be published in its current state (a rare occurrence), published with revisions, or not accepted for publication.
Peer review provides some degree of quality control for psychological research. Poorly conceived or executed studies can be weeded out, and even well-designed research can be improved by the revisions suggested. Peer review also ensures that the research is described clearly enough to allow other scientists to replicate it, meaning they can repeat the experiment using different samples to determine reliability. Sometimes replications involve additional measures that expand on the original finding. In any case, each replication serves to provide more evidence to support the original research findings. Successful replications of published research make scientists more apt to adopt those findings, while repeated failures tend to cast doubt on the legitimacy of the original article and lead scientists to look elsewhere. For example, it would be a major advancement in the medical field if a published study indicated that taking a new drug helped individuals achieve better health without changing their behavior. But if other scientists could not replicate the results, the original study’s claims would be questioned.
In recent years, there has been increasing concern about a “replication crisis” that has affected a number of scientific fields, including psychology. Some of the most well-known studies and scientists have produced research that has failed to be replicated by others (as discussed in Shrout & Rodgers, 2018). In fact, even a famous Nobel Prize-winning scientist has recently retracted a published paper because she had difficulty replicating her results (Nobel Prize-winning scientist Frances Arnold retracts paper, 2020 January 3). These kinds of outcomes have prompted some scientists to begin to work together and more openly, and some would argue that the current “crisis” is actually improving the ways in which science is conducted and in how its results are shared with others (Aschwanden, 2018).
The Vaccine-Autism Myth and Retraction of Published Studies
Some scientists have claimed that routine childhood vaccines cause some children to develop autism, and, in fact, several peer-reviewed publications published research making these claims. Since the initial reports, large-scale epidemiological research has indicated that vaccinations are not responsible for causing autism and that it is much safer to have your child vaccinated than not. Furthermore, several of the original studies making this claim have since been retracted.
A published piece of work can be rescinded when data is called into question because of falsification, fabrication, or serious research design problems. Once rescinded, the scientific community is informed that there are serious problems with the original publication. Retractions can be initiated by the researcher who led the study, by research collaborators, by the institution that employed the researcher, or by the editorial board of the journal in which the article was originally published. In the vaccine-autism case, the retraction was made because of a significant conflict of interest in which the leading researcher had a financial interest in establishing a link between childhood vaccines and autism (Offit, 2008). Unfortunately, the initial studies received so much media attention that many parents around the world became hesitant to have their children vaccinated ( Figure 2.19 ). Continued reliance on such debunked studies has significant consequences. For instance, between January and October of 2019, there were 22 measles outbreaks across the United States and more than a thousand cases of individuals contracting measles (Patel et al., 2019). This is likely due to the anti-vaccination movements that have risen from the debunked research. For more information about how the vaccine/autism story unfolded, as well as the repercussions of this story, take a look at Paul Offit’s book, Autism’s False Prophets: Bad Science, Risky Medicine, and the Search for a Cure.
Reliability and Validity
Reliability and validity are two important considerations that must be made with any type of data collection. Reliability refers to the ability to consistently produce a given result. In the context of psychological research, this would mean that any instruments or tools used to collect data do so in consistent, reproducible ways. There are a number of different types of reliability. Some of these include inter-rater reliability (the degree to which two or more different observers agree on what has been observed), internal consistency (the degree to which different items on a survey that measure the same thing correlate with one another), and test-retest reliability (the degree to which the outcomes of a particular measure remain consistent over multiple administrations).
Unfortunately, being consistent in measurement does not necessarily mean that you have measured something correctly. To illustrate this concept, consider a kitchen scale that would be used to measure the weight of cereal that you eat in the morning. If the scale is not properly calibrated, it may consistently under- or overestimate the amount of cereal that’s being measured. While the scale is highly reliable in producing consistent results (e.g., the same amount of cereal poured onto the scale produces the same reading each time), those results are incorrect. This is where validity comes into play. Validity refers to the extent to which a given instrument or tool accurately measures what it’s supposed to measure, and once again, there are a number of ways in which validity can be expressed. Ecological validity (the degree to which research results generalize to real-world applications), construct validity (the degree to which a given variable actually captures or measures what it is intended to measure), and face validity (the degree to which a given variable seems valid on the surface) are just a few types that researchers consider. While any valid measure is by necessity reliable, the reverse is not necessarily true. Researchers strive to use instruments that are both highly reliable and valid.
Everyday Connection
How valid are the sat and act.
Standardized tests like the SAT and ACT are supposed to measure an individual’s aptitude for a college education, but how reliable and valid are such tests? Research conducted by the College Board suggests that scores on the SAT have high predictive validity for first-year college students’ GPA (Kobrin, Patterson, Shaw, Mattern, & Barbuti, 2008). In this context, predictive validity refers to the test’s ability to effectively predict the GPA of college freshmen. Given that many institutions of higher education require the SAT or ACT for admission, this high degree of predictive validity might be comforting.
However, the emphasis placed on SAT or ACT scores in college admissions is changing based on a number of factors. For one, some researchers assert that these tests are biased, and students from historically marginalized populations are at a disadvantage that unfairly reduces the likelihood of being admitted into a college (Santelices & Wilson, 2010). Additionally, some research has suggested that the predictive validity of these tests is grossly exaggerated in how well they are able to predict the GPA of first-year college students. In fact, it has been suggested that the SAT’s predictive validity may be overestimated by as much as 150% (Rothstein, 2004). Many institutions of higher education are beginning to consider de-emphasizing the significance of SAT scores in making admission decisions (Rimer, 2008).
Recent examples of high profile cheating scandals both domestically and abroad have only increased the scrutiny being placed on these types of tests, and as of March 2019, more than 1000 institutions of higher education have either relaxed or eliminated the requirements for SAT or ACT testing for admissions (Strauss, 2019, March 19).
As an Amazon Associate we earn from qualifying purchases.
This book may not be used in the training of large language models or otherwise be ingested into large language models or generative AI offerings without OpenStax's permission.
Want to cite, share, or modify this book? This book uses the Creative Commons Attribution License and you must attribute OpenStax.
Access for free at https://openstax.org/books/psychology-2e/pages/1-introduction
- Authors: Rose M. Spielman, William J. Jenkins, Marilyn D. Lovett
- Publisher/website: OpenStax
- Book title: Psychology 2e
- Publication date: Apr 22, 2020
- Location: Houston, Texas
- Book URL: https://openstax.org/books/psychology-2e/pages/1-introduction
- Section URL: https://openstax.org/books/psychology-2e/pages/2-3-analyzing-findings
© Jan 6, 2024 OpenStax. Textbook content produced by OpenStax is licensed under a Creative Commons Attribution License . The OpenStax name, OpenStax logo, OpenStax book covers, OpenStax CNX name, and OpenStax CNX logo are not subject to the Creative Commons license and may not be reproduced without the prior and express written consent of Rice University.

In order to continue enjoying our site, we ask that you confirm your identity as a human. Thank you very much for your cooperation.
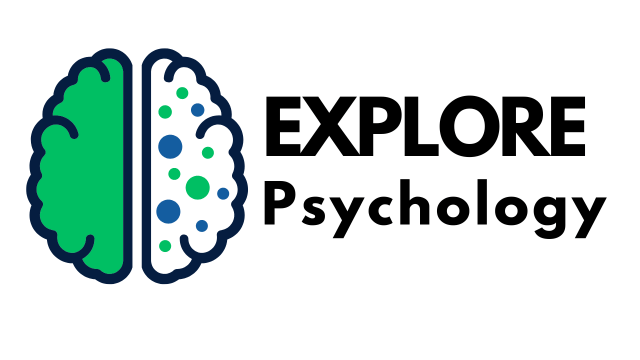
Correlational Research in Psychology: Definition and How It Works
Categories Research Methods
Correlational research is a type of scientific investigation in which a researcher looks at the relationships between variables but does not vary, manipulate, or control them. It can be a useful research method for evaluating the direction and strength of the relationship between two or more different variables.
When examining how variables are related to one another, researchers may find that the relationship is positive or negative. Or they may also find that there is no relationship at all.
Table of Contents
How Does Correlational Research Work?
In correlational research, the researcher measures the values of the variables of interest and calculates a correlation coefficient, which quantifies the strength and direction of the relationship between the variables.
The correlation coefficient ranges from -1.0 to +1.0, where -1.0 represents a perfect negative correlation, 0 represents no correlation, and +1.0 represents a perfect positive correlation.
A negative correlation indicates that as the value of one variable increases, the value of the other variable decreases, while a positive correlation indicates that as the value of one variable increases, the value of the other variable also increases. A zero correlation indicates that there is no relationship between the variables.
The variables both increase together | The more you walk on a treadmill, the more calories you burn. | |
The variables decrease together | The less you study, the lower your grades will be. | |
No relationship exists between the variables | How much you walk on a treadmill is not associated with grades on exams. |
Correlational Research vs. Experimental Research
Correlational research differs from experimental research in that it does not involve manipulating variables. Instead, it focuses on analyzing the relationship between two or more variables.
In other words, correlational research seeks to determine whether there is a relationship between two variables and, if so, the nature of that relationship.
Experimental research, on the other hand, involves manipulating one or more variables to determine the effect on another variable. Because of this manipulation and control of variables, experimental research allows for causal conclusions to be drawn, while correlational research does not.
Both types of research are important in understanding the world around us, but they serve different purposes and are used in different situations.
Utilized to assess the strength and direction of the relationship between variables | Utilized to look for cause-and-effect relationships between variables |
Involves measuring but not manipulating variables | Involves manipulating an independent variable and measuring the effect on the dependent variable |
Results may be influenced by other variables that the researcher cannot control | Researchers are better able to control extraneous variables that might impact results |
Types of Correlational Research
There are three main types of correlational studies:
Cohort Correlational Study
This type of study involves following a cohort of participants over a period of time. This type of research can be useful for understanding how certain events might influence outcomes.
For example, researchers might study how exposure to a traumatic natural disaster influences the mental health of a group of people over time.
By examining the data collected from these individuals, researchers can determine whether there is a correlation between the two variables under investigation. This information can be used to develop strategies for preventing or treating certain conditions or illnesses.
Cross-Sectional Correlational Study
A cross-sectional design is a research method that examines a group of individuals at a single time. This type of study collects information from a diverse group of people, usually from different backgrounds and age groups, to gain insight into a particular phenomenon or issue.
The data collected from this type of study is used to analyze relationships between variables and identify patterns and trends within the group.
Cross-sectional studies can help identify potential risk factors for certain conditions or illnesses, and can also be used to evaluate the prevalence of certain behaviors, attitudes, or beliefs within a population.
Case-Control Correlational Study
A case-control correlational study is a type of research design that investigates the relationship between exposure and health outcomes. In this study, researchers identify a group of individuals with the health outcome of interest (cases) and another group of individuals without the health outcome (controls).
The researchers then compare the exposure history of the cases and controls to determine whether the exposure and health outcome correlate.
This type of study design is often used in epidemiology and can provide valuable information about potential risk factors for a particular disease or condition.
When to Use Correlational Research
There are a number of situations where researchers might opt to use a correlational study instead of some other research design.
Correlational research can be used to investigate a wide range of psychological phenomena, including the relationship between personality traits and academic performance, the association between sleep duration and mental health, and the correlation between parental involvement and child outcomes.
To Generate Hypotheses
Correlational research can also be used to generate hypotheses for further research by identifying variables that are associated with each other.
To Investigate Variables Without Manipulating Them
Researchers should use correlational research when they want to investigate the relationship between two variables without manipulating them. This type of research is useful when the researcher cannot or should not manipulate one of the variables or when it is impossible to conduct an experiment due to ethical or practical concerns.
To Identify Patterns
Correlational research allows researchers to identify patterns and relationships between variables, which can inform future research and help to develop theories. However, it is important to note that correlational research does not prove that one variable causes changes in the other.
While correlational research has its limitations, it is still a valuable tool for researchers in many fields, including psychology, sociology, and education.
How to Collect Data in Correlational Research
Researchers can collect data for correlational research in a few different ways. To conduct correlational research, data can be collected using the following:
- Surveys : One method is through surveys, where participants are asked to self-report their behaviors or attitudes. This approach allows researchers to gather large amounts of data quickly and affordably.
- Naturalistic observation : Another method is through observation, where researchers observe and record behaviors in a natural or controlled setting. This method allows researchers to learn more about the behavior in question and better generalize the results to real-world settings.
- Archival, retrospective data : Additionally, researchers can collect data from archival sources, such as medical, school records, official records, or past polls.
The key is to collect data from a large and representative sample to measure the relationship between two variables accurately.

Pros and Cons of Correlational Research
There are some advantages of using correlational research, but there are also some downsides to consider.
- One of the strengths of correlational research is its ability to identify patterns and relationships between variables that may be difficult or unethical to manipulate in an experimental study.
- Correlational research can also be used to examine variables that are not under the control of the researcher , such as age, gender, or socioeconomic status.
- Correlational research can be used to make predictions about future behavior or outcomes, which can be valuable in a variety of fields.
- Correlational research can be conducted quickly and inexpensively , making it a practical option for researchers with limited resources.
- Correlational research is limited by its inability to establish causality between variables. Correlation does not imply causation, and it is possible that a third variable may be influencing both variables of interest, creating a spurious correlation. Therefore, it is important for researchers to use multiple methods of data collection and to be cautious when interpreting correlational findings.
- Correlational research relies heavily on self-reported data , which can be biased or inaccurate.
- Correlational research is limited in its ability to generalize findings to larger populations, as it only measures the relationship between two variables in a specific sample.
Frequently Asked Questions About Correlational Research
What are the main problems with correlational research.
Some of the main problems that can occur in correlational research include selection bias, confounding variables. and misclassification.
- Selecting participants based on their exposure to an event means that the sample might be biased since the selection was not randomized.
- Correlational studies may also be impacted by extraneous factors that researchers cannot control.
- Finally, there may be problems with how accurately data is recorded and classified, which can be particularly problematic in retrospective studies.
What are the variables in a correlational study?
In a correlational study, variables refer to any measurable factors being examined for their potential relationship or association with each other. These variables can be continuous (meaning they can take on a range of values) or categorical (meaning they fall into distinct categories or groups).
For example, in a study examining the correlation between exercise and mental health, the independent variable would be exercise frequency (measured in times per week), while the dependent variable would be mental health (measured using a standardized questionnaire).
What is the goal of correlational research?
The goal of correlational research is to examine the relationship between two or more variables. It involves analyzing data to determine if there is a statistically significant connection between the variables being studied.
Correlational research is useful for identifying patterns and making predictions but cannot establish causation. Instead, it helps researchers to better understand the nature of the relationship between variables and to generate hypotheses for further investigation.
How do you identify correlational research?
To identify correlational research, look for studies that measure two or more variables and analyze their relationship using statistical techniques. The results of correlational studies are typically presented in the form of correlation coefficients or scatterplots, which visually represent the degree of association between the variables being studied.
Correlational research can be useful for identifying potential causal relationships between variables but cannot establish causation on its own.
Curtis EA, Comiskey C, Dempsey O. Importance and use of correlational research . Nurse Researcher . 2016;23(6):20-25. doi10.7748/nr.2016.e1382
Lau F. Chapter 12 Methods for Correlational Studies . University of Victoria; 2017.
Mitchell TR. An evaluation of the validity of correlational research conducted in organizations . The Academy of Management Review . 1985;10(2):192. doi:10.5465/amr.1985.4277939
Seeram E. An overview of correlational research . Radiol Technol . 2019;91(2):176-179.
Have a language expert improve your writing
Run a free plagiarism check in 10 minutes, generate accurate citations for free.
- Knowledge Base
- Correlation Coefficient | Types, Formulas & Examples
Correlation Coefficient | Types, Formulas & Examples
Published on August 2, 2021 by Pritha Bhandari . Revised on June 22, 2023.
A correlation coefficient is a number between -1 and 1 that tells you the strength and direction of a relationship between variables .
In other words, it reflects how similar the measurements of two or more variables are across a dataset.
Correlation coefficient value | Correlation type | Meaning |
---|---|---|
1 | Perfect positive correlation | When one variable changes, the other variables change in the same direction. |
0 | Zero correlation | There is no relationship between the variables. |
-1 | Perfect negative correlation | When one variable changes, the other variables change in the opposite direction. |
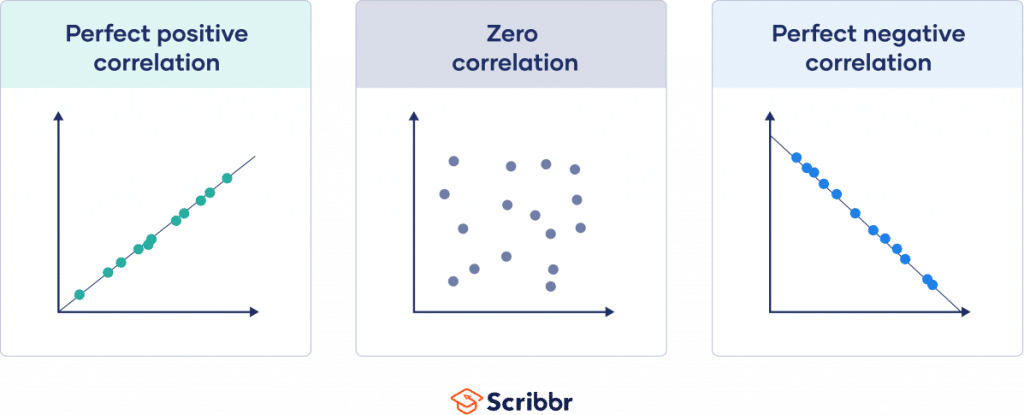
Table of contents
What does a correlation coefficient tell you, using a correlation coefficient, interpreting a correlation coefficient, visualizing linear correlations, types of correlation coefficients, pearson’s r, spearman’s rho, other coefficients, other interesting articles, frequently asked questions about correlation coefficients.
Correlation coefficients summarize data and help you compare results between studies.
Summarizing data
A correlation coefficient is a descriptive statistic . That means that it summarizes sample data without letting you infer anything about the population. A correlation coefficient is a bivariate statistic when it summarizes the relationship between two variables, and it’s a multivariate statistic when you have more than two variables.
If your correlation coefficient is based on sample data, you’ll need an inferential statistic if you want to generalize your results to the population. You can use an F test or a t test to calculate a test statistic that tells you the statistical significance of your finding.
Comparing studies
A correlation coefficient is also an effect size measure, which tells you the practical significance of a result.
Correlation coefficients are unit-free, which makes it possible to directly compare coefficients between studies.
Here's why students love Scribbr's proofreading services
Discover proofreading & editing
In correlational research , you investigate whether changes in one variable are associated with changes in other variables.
After data collection , you can visualize your data with a scatterplot by plotting one variable on the x-axis and the other on the y-axis. It doesn’t matter which variable you place on either axis.
Visually inspect your plot for a pattern and decide whether there is a linear or non-linear pattern between variables. A linear pattern means you can fit a straight line of best fit between the data points, while a non-linear or curvilinear pattern can take all sorts of different shapes, such as a U-shape or a line with a curve.
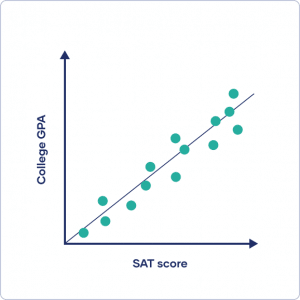
There are many different correlation coefficients that you can calculate. After removing any outliers , select a correlation coefficient that’s appropriate based on the general shape of the scatter plot pattern. Then you can perform a correlation analysis to find the correlation coefficient for your data.
You calculate a correlation coefficient to summarize the relationship between variables without drawing any conclusions about causation .
Both variables are quantitative and normally distributed with no outliers, so you calculate a Pearson’s r correlation coefficient .
The value of the correlation coefficient always ranges between 1 and -1, and you treat it as a general indicator of the strength of the relationship between variables.
The sign of the coefficient reflects whether the variables change in the same or opposite directions: a positive value means the variables change together in the same direction, while a negative value means they change together in opposite directions.
The absolute value of a number is equal to the number without its sign. The absolute value of a correlation coefficient tells you the magnitude of the correlation: the greater the absolute value, the stronger the correlation.
There are many different guidelines for interpreting the correlation coefficient because findings can vary a lot between study fields. You can use the table below as a general guideline for interpreting correlation strength from the value of the correlation coefficient.
While this guideline is helpful in a pinch, it’s much more important to take your research context and purpose into account when forming conclusions. For example, if most studies in your field have correlation coefficients nearing .9, a correlation coefficient of .58 may be low in that context.
Correlation coefficient | Correlation strength | Correlation type |
---|---|---|
-.7 to -1 | Very strong | Negative |
-.5 to -.7 | Strong | Negative |
-.3 to -.5 | Moderate | Negative |
0 to -.3 | Weak | Negative |
0 | None | Zero |
0 to .3 | Weak | Positive |
.3 to .5 | Moderate | Positive |
.5 to .7 | Strong | Positive |
.7 to 1 | Very strong | Positive |
The correlation coefficient tells you how closely your data fit on a line. If you have a linear relationship, you’ll draw a straight line of best fit that takes all of your data points into account on a scatter plot.
The closer your points are to this line, the higher the absolute value of the correlation coefficient and the stronger your linear correlation.
If all points are perfectly on this line, you have a perfect correlation.
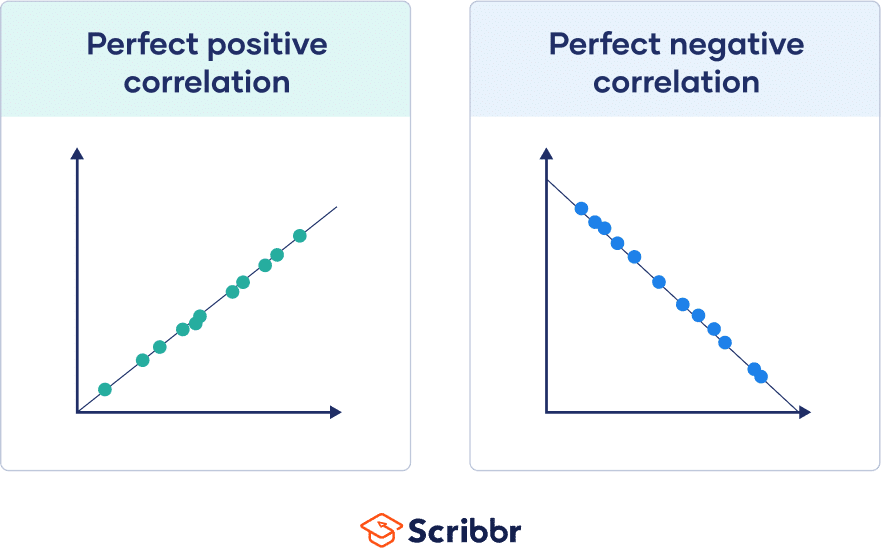
If all points are close to this line, the absolute value of your correlation coefficient is high .
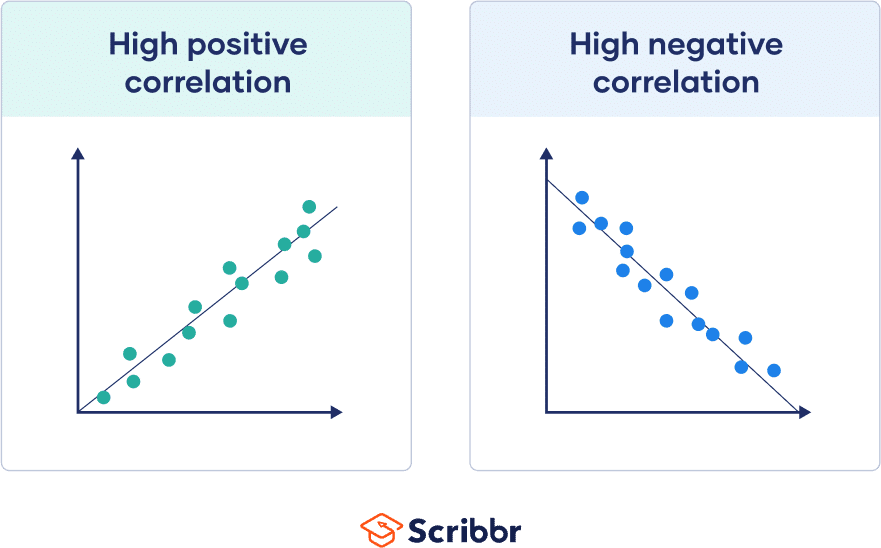
If these points are spread far from this line, the absolute value of your correlation coefficient is low .
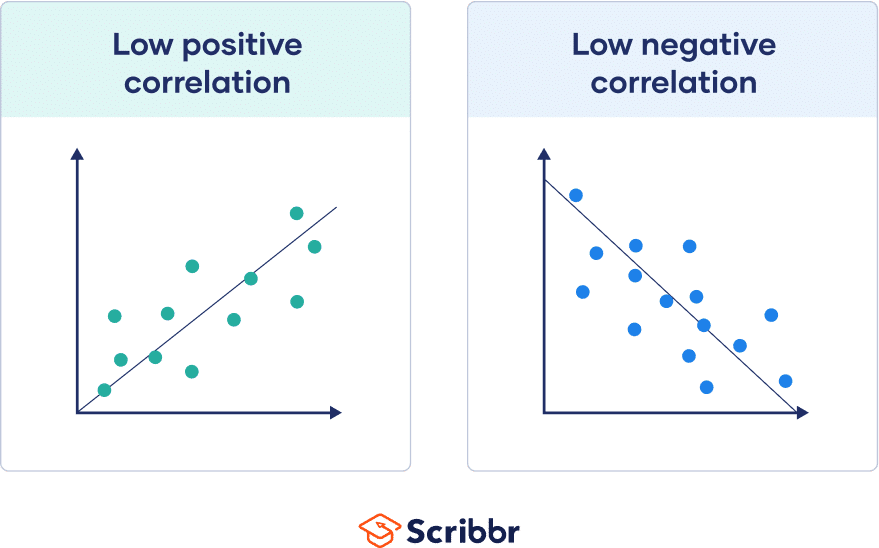
Note that the steepness or slope of the line isn’t related to the correlation coefficient value. The correlation coefficient doesn’t help you predict how much one variable will change based on a given change in the other, because two datasets with the same correlation coefficient value can have lines with very different slopes.
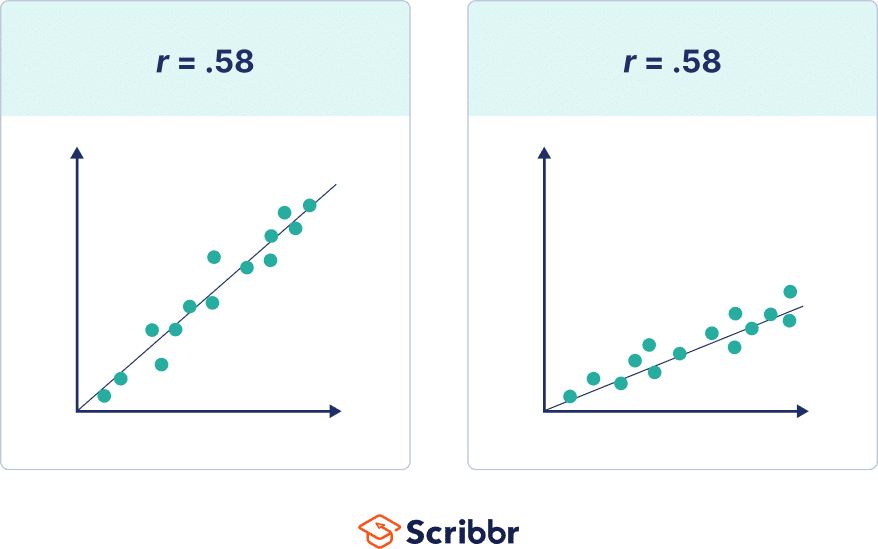
You can choose from many different correlation coefficients based on the linearity of the relationship, the level of measurement of your variables, and the distribution of your data.
For high statistical power and accuracy, it’s best to use the correlation coefficient that’s most appropriate for your data.
The most commonly used correlation coefficient is Pearson’s r because it allows for strong inferences. It’s parametric and measures linear relationships. But if your data do not meet all assumptions for this test, you’ll need to use a non-parametric test instead.
Non-parametric tests of rank correlation coefficients summarize non-linear relationships between variables. The Spearman’s rho and Kendall’s tau have the same conditions for use, but Kendall’s tau is generally preferred for smaller samples whereas Spearman’s rho is more widely used.
The table below is a selection of commonly used correlation coefficients, and we’ll cover the two most widely used coefficients in detail in this article.
Correlation coefficient | Type of relationship | Levels of measurement | Data distribution |
---|---|---|---|
Pearson’s r | Linear | Two quantitative (interval or ratio) variables | Normal distribution |
Spearman’s rho | Non-linear | Two , interval or ratio variables | Any distribution |
Point-biserial | Linear | One dichotomous (binary) variable and one quantitative ( or ratio) variable | Normal distribution |
Cramér’s V (Cramér’s φ) | Non-linear | Two | Any distribution |
Kendall’s tau | Non-linear | Two ordinal, interval or | Any distribution |
The Pearson’s product-moment correlation coefficient, also known as Pearson’s r, describes the linear relationship between two quantitative variables.
These are the assumptions your data must meet if you want to use Pearson’s r:
- Both variables are on an interval or ratio level of measurement
- Data from both variables follow normal distributions
- Your data have no outliers
- Your data is from a random or representative sample
- You expect a linear relationship between the two variables
The Pearson’s r is a parametric test, so it has high power. But it’s not a good measure of correlation if your variables have a nonlinear relationship, or if your data have outliers, skewed distributions, or come from categorical variables. If any of these assumptions are violated, you should consider a rank correlation measure.
The formula for the Pearson’s r is complicated, but most computer programs can quickly churn out the correlation coefficient from your data. In a simpler form, the formula divides the covariance between the variables by the product of their standard deviations .
Formula | Explanation |
---|---|
| = strength of the correlation between variables x and y = sample size = sum of what follows… = every x-variable value = every y-variable value = the product of each x-variable score and the corresponding y-variable score |
Pearson sample vs population correlation coefficient formula
When using the Pearson correlation coefficient formula, you’ll need to consider whether you’re dealing with data from a sample or the whole population.
The sample and population formulas differ in their symbols and inputs. A sample correlation coefficient is called r , while a population correlation coefficient is called rho, the Greek letter ρ.
The sample correlation coefficient uses the sample covariance between variables and their sample standard deviations.
Sample correlation coefficient formula | Explanation |
---|---|
| = strength of the correlation between variables x and y ( , ) = covariance of x and y = sample standard deviation of x = sample standard deviation of y |
The population correlation coefficient uses the population covariance between variables and their population standard deviations.
Population correlation coefficient formula | Explanation |
---|---|
| = strength of the correlation between variables X and Y ( , ) = covariance of X and Y = population standard deviation of X = population standard deviation of Y |
Spearman’s rho, or Spearman’s rank correlation coefficient, is the most common alternative to Pearson’s r . It’s a rank correlation coefficient because it uses the rankings of data from each variable (e.g., from lowest to highest) rather than the raw data itself.
You should use Spearman’s rho when your data fail to meet the assumptions of Pearson’s r . This happens when at least one of your variables is on an ordinal level of measurement or when the data from one or both variables do not follow normal distributions.
While the Pearson correlation coefficient measures the linearity of relationships, the Spearman correlation coefficient measures the monotonicity of relationships.
In a linear relationship, each variable changes in one direction at the same rate throughout the data range. In a monotonic relationship, each variable also always changes in only one direction but not necessarily at the same rate.
- Positive monotonic: when one variable increases, the other also increases.
- Negative monotonic: when one variable increases, the other decreases.
Monotonic relationships are less restrictive than linear relationships.
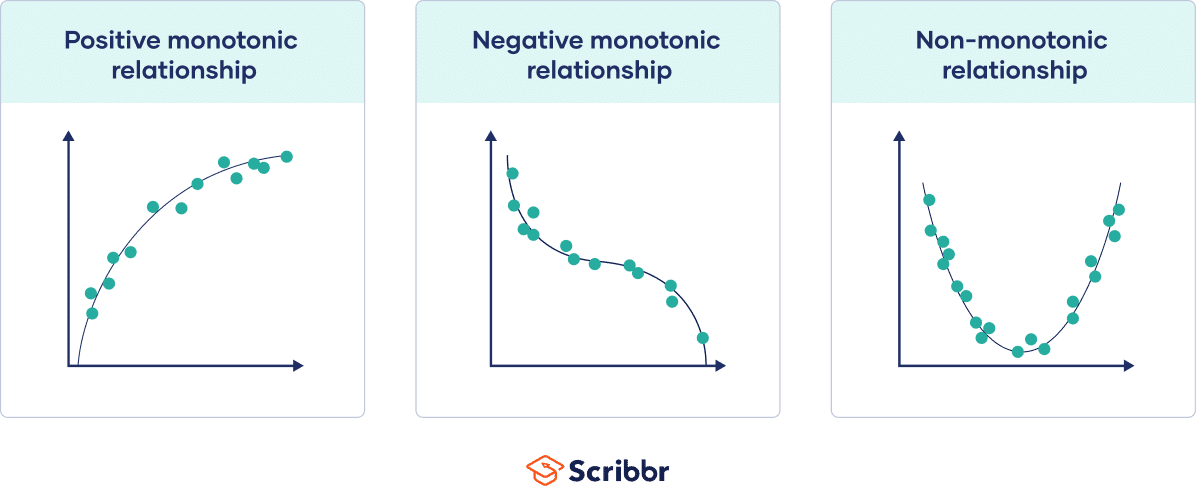
Spearman’s rank correlation coefficient formula
The symbols for Spearman’s rho are ρ for the population coefficient and r s for the sample coefficient. The formula calculates the Pearson’s r correlation coefficient between the rankings of the variable data.
To use this formula, you’ll first rank the data from each variable separately from low to high: every datapoint gets a rank from first, second, or third, etc.
Then, you’ll find the differences (d i ) between the ranks of your variables for each data pair and take that as the main input for the formula.
Spearman’s rank correlation coefficient formula | Explanation |
---|---|
| = strength of the rank correlation between variables = the difference between the x-variable rank and the y-variable rank for each pair of data = sum of the squared differences between x- and y-variable ranks = sample size |
If you have a correlation coefficient of 1, all of the rankings for each variable match up for every data pair. If you have a correlation coefficient of -1, the rankings for one variable are the exact opposite of the ranking of the other variable. A correlation coefficient near zero means that there’s no monotonic relationship between the variable rankings.
The correlation coefficient is related to two other coefficients, and these give you more information about the relationship between variables.
Coefficient of determination
When you square the correlation coefficient, you end up with the correlation of determination ( r 2 ). This is the proportion of common variance between the variables. The coefficient of determination is always between 0 and 1, and it’s often expressed as a percentage.
Coefficient of determination | Explanation |
---|---|
The correlation coefficient multiplied by itself |
The coefficient of determination is used in regression models to measure how much of the variance of one variable is explained by the variance of the other variable.
A regression analysis helps you find the equation for the line of best fit, and you can use it to predict the value of one variable given the value for the other variable.
A high r 2 means that a large amount of variability in one variable is determined by its relationship to the other variable. A low r 2 means that only a small portion of the variability of one variable is explained by its relationship to the other variable; relationships with other variables are more likely to account for the variance in the variable.
The correlation coefficient can often overestimate the relationship between variables, especially in small samples, so the coefficient of determination is often a better indicator of the relationship.
Coefficient of alienation
When you take away the coefficient of determination from unity (one), you’ll get the coefficient of alienation. This is the proportion of common variance not shared between the variables, the unexplained variance between the variables.
Coefficient of alienation | Explanation |
---|---|
1 – | One minus the coefficient of determination |
A high coefficient of alienation indicates that the two variables share very little variance in common. A low coefficient of alienation means that a large amount of variance is accounted for by the relationship between the variables.
If you want to know more about statistics , methodology , or research bias , make sure to check out some of our other articles with explanations and examples.
- Chi square test of independence
- Statistical power
- Descriptive statistics
- Degrees of freedom
- Pearson correlation
- Null hypothesis
Methodology
- Double-blind study
- Case-control study
- Research ethics
- Data collection
- Hypothesis testing
- Structured interviews
Research bias
- Hawthorne effect
- Unconscious bias
- Recall bias
- Halo effect
- Self-serving bias
- Information bias
A correlation reflects the strength and/or direction of the association between two or more variables.
- A positive correlation means that both variables change in the same direction.
- A negative correlation means that the variables change in opposite directions.
- A zero correlation means there’s no relationship between the variables.
A correlation is usually tested for two variables at a time, but you can test correlations between three or more variables.
A correlation coefficient is a single number that describes the strength and direction of the relationship between your variables.
Different types of correlation coefficients might be appropriate for your data based on their levels of measurement and distributions . The Pearson product-moment correlation coefficient (Pearson’s r ) is commonly used to assess a linear relationship between two quantitative variables.
These are the assumptions your data must meet if you want to use Pearson’s r :
Correlation coefficients always range between -1 and 1.
The sign of the coefficient tells you the direction of the relationship: a positive value means the variables change together in the same direction, while a negative value means they change together in opposite directions.
No, the steepness or slope of the line isn’t related to the correlation coefficient value. The correlation coefficient only tells you how closely your data fit on a line, so two datasets with the same correlation coefficient can have very different slopes.
To find the slope of the line, you’ll need to perform a regression analysis .
Cite this Scribbr article
If you want to cite this source, you can copy and paste the citation or click the “Cite this Scribbr article” button to automatically add the citation to our free Citation Generator.
Bhandari, P. (2023, June 22). Correlation Coefficient | Types, Formulas & Examples. Scribbr. Retrieved June 24, 2024, from https://www.scribbr.com/statistics/correlation-coefficient/
Is this article helpful?
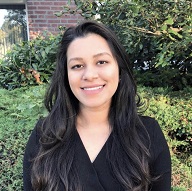
Pritha Bhandari
Other students also liked, correlational research | when & how to use, correlation vs. causation | difference, designs & examples, simple linear regression | an easy introduction & examples, what is your plagiarism score.
- Skip to primary navigation
- Skip to main content
- Skip to primary sidebar
- Skip to footer
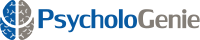
Psychologenie
Definition of Positive Correlation in Psychology With Examples
Positive correlation can be defined as the direct relationship between two variables, i.e., when the value of one variable increases, the value of the other increases too. This post explains this concept in psychology, with the help of some examples.

“The consumption of ice cream (pints per person) and the number of murders in New York are positively correlated. That is, as the amount of ice cream sold per person increases, the number of murders increases. Strange but true!” ― Deborah J. Rumsey, Statistics For Dummies®
Psychology uses various methods for its research, and one of them is studying the correlation between any two variables. Correlation is nothing but the measure of degree of relation between two variables. It can be plotted graphically to show the relationship between them.
Correlation studies the relationship between two variables, and its coefficient can range from -1 to 1. A positively inclining relationship is nothing but positive correlation. Its value can range from 0 to 1. Positive correlation implies there is a positive relationship between the two variables, i.e., when the value of one variable increases, the value of other variable also increases, and the opposite happens when the value of one variable decreases. Correlation is used in many fields, such as mathematics, statistics, economics, psychology, etc.
Let’s take a hypothetical example , where a researcher is trying to study the relationship between two variables, namely ‘x’ and ‘y’. The example will help you understand what is positive correlation.
Let ‘x’ be the number of hours that a student has studied, and ‘y’ be his score in a test (maximum marks: 120). The researcher picks up 20 students from a class, and records the number of hours they studied for the test. The researcher then records the marks scored by the students in the test. We try to compare the relationship between the number of hours the student has devoted in studying, and his corresponding score.
4 | 47 |
2 | 23 |
3 | 31 |
5 | 55 |
6 | 66 |
5.5 | 65 |
8 | 82 |
3.5 | 48 |
10 | 94 |
9.5 | 80 |
8.5 | 80 |
6.7 | 62 |
8.9 | 84 |
2.5 | 38 |
4.7 | 35 |
3.3 | 43 |
5.2 | 51 |
10.1 | 101 |
7.6 | 84 |
9.3 | 70 |
► The given data is of two variables ‘x’ and ‘y’. There are 20 observations recorded by the researcher. We will plot these points on a graph.
► After plotting the points on the graph, we get a scatter diagram. The scatter diagram indicates the trend, and displays whether the correlation is positive or negative.
► An upward trend usually indicates a positive correlation, and on the other hand, a downward trend usually indicates a negative correlation. The degree of relation will however differ every time. Thus, the scatter diagram helps us visualize the correlation.
► In psychology, correlation can be helpful in studying behavioral patterns. For example, if you want to study whether those students who are depressed fail in their examinations or score poorly, you can plot your observations and study the association between them. If there is a positive association, it implies that depressed students are more prone to fail in their examinations.
Graphical Representation of Data: Scatter Diagram

What Do We Observe?
► After plotting the points on the graph, we can notice the upward/rising trend of the scatter diagram. This indicates that as the value of variable ‘x’ increases, the value of ‘y’ also increases. Thus, this indicates that the students who have put in more hours of study have scored better in the test.
► However, this survey method has its own limitations. This data is based on the statistics of 20 students in a class with different IQ levels. Though the trend here observed is positive, there are high chances that the IQ level of that student can play an important contributing factor too. The inference that more the hours you study, better the score, might hold true, if it is assumed that the IQ level of all the students is similar, on an average. However, there are other variables that cannot be ruled out, such as the level of concentration of the students, which can influence the scores.
Examples of Positive Correlation in Real Life
► If I walk more, I will burn more calories. ► With the growth of the company, the market value of company stocks increase. ► When demand increases, price of the product increases (at same supply level). ► When you study more, you score high in the exams. ► When you pay more to your employees, they’re motivated to perform better. ► With increase in consumption of junk food, there is increase in obesity. ► When you meditate more, your concentration level increases. ► Couples who spend more time together have a healthier and long-lasting relationship.
It must be noted that correlation does not imply causation. A direct relationship or positive relationship does not imply that they are the cause and effect of each other. A correlation between two variables aids the researcher in determining the association between them. However, statistical data is based on a sample, and hence, can sometimes lead to misleading results. A strong positive correlation does not imply there is necessarily a relationship between them; it might be due to an unknown external variable. Hence, researchers have to be careful about the statistical data while drawing inferences.
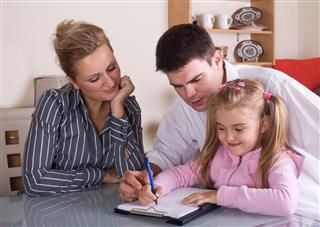
Like it? Share it!
Get Updates Right to Your Inbox
Further insights.
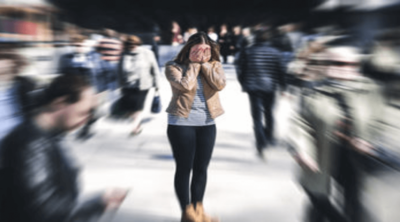
Privacy Overview

Want to create or adapt books like this? Learn more about how Pressbooks supports open publishing practices.
2.2 Psychologists Use Descriptive, Correlational, and Experimental Research Designs to Understand Behavior
Learning objectives.
- Differentiate the goals of descriptive, correlational, and experimental research designs and explain the advantages and disadvantages of each.
- Explain the goals of descriptive research and the statistical techniques used to interpret it.
- Summarize the uses of correlational research and describe why correlational research cannot be used to infer causality.
- Review the procedures of experimental research and explain how it can be used to draw causal inferences.
Psychologists agree that if their ideas and theories about human behavior are to be taken seriously, they must be backed up by data. However, the research of different psychologists is designed with different goals in mind, and the different goals require different approaches. These varying approaches, summarized in Table 2.2 “Characteristics of the Three Research Designs” , are known as research designs . A research design is the specific method a researcher uses to collect, analyze, and interpret data . Psychologists use three major types of research designs in their research, and each provides an essential avenue for scientific investigation. Descriptive research is research designed to provide a snapshot of the current state of affairs . Correlational research is research designed to discover relationships among variables and to allow the prediction of future events from present knowledge . Experimental research is research in which initial equivalence among research participants in more than one group is created, followed by a manipulation of a given experience for these groups and a measurement of the influence of the manipulation . Each of the three research designs varies according to its strengths and limitations, and it is important to understand how each differs.
Table 2.2 Characteristics of the Three Research Designs
Research design | Goal | Advantages | Disadvantages |
---|---|---|---|
Descriptive | To create a snapshot of the current state of affairs | Provides a relatively complete picture of what is occurring at a given time. Allows the development of questions for further study. | Does not assess relationships among variables. May be unethical if participants do not know they are being observed. |
Correlational | To assess the relationships between and among two or more variables | Allows testing of expected relationships between and among variables and the making of predictions. Can assess these relationships in everyday life events. | Cannot be used to draw inferences about the causal relationships between and among the variables. |
Experimental | To assess the causal impact of one or more experimental manipulations on a dependent variable | Allows drawing of conclusions about the causal relationships among variables. | Cannot experimentally manipulate many important variables. May be expensive and time consuming. |
There are three major research designs used by psychologists, and each has its own advantages and disadvantages. |
Stangor, C. (2011). Research methods for the behavioral sciences (4th ed.). Mountain View, CA: Cengage.
Descriptive Research: Assessing the Current State of Affairs
Descriptive research is designed to create a snapshot of the current thoughts, feelings, or behavior of individuals. This section reviews three types of descriptive research: case studies , surveys , and naturalistic observation .
Sometimes the data in a descriptive research project are based on only a small set of individuals, often only one person or a single small group. These research designs are known as case studies — descriptive records of one or more individual’s experiences and behavior . Sometimes case studies involve ordinary individuals, as when developmental psychologist Jean Piaget used his observation of his own children to develop his stage theory of cognitive development. More frequently, case studies are conducted on individuals who have unusual or abnormal experiences or characteristics or who find themselves in particularly difficult or stressful situations. The assumption is that by carefully studying individuals who are socially marginal, who are experiencing unusual situations, or who are going through a difficult phase in their lives, we can learn something about human nature.
Sigmund Freud was a master of using the psychological difficulties of individuals to draw conclusions about basic psychological processes. Freud wrote case studies of some of his most interesting patients and used these careful examinations to develop his important theories of personality. One classic example is Freud’s description of “Little Hans,” a child whose fear of horses the psychoanalyst interpreted in terms of repressed sexual impulses and the Oedipus complex (Freud (1909/1964).

Political polls reported in newspapers and on the Internet are descriptive research designs that provide snapshots of the likely voting behavior of a population.
Another well-known case study is Phineas Gage, a man whose thoughts and emotions were extensively studied by cognitive psychologists after a railroad spike was blasted through his skull in an accident. Although there is question about the interpretation of this case study (Kotowicz, 2007), it did provide early evidence that the brain’s frontal lobe is involved in emotion and morality (Damasio et al., 2005). An interesting example of a case study in clinical psychology is described by Rokeach (1964), who investigated in detail the beliefs and interactions among three patients with schizophrenia, all of whom were convinced they were Jesus Christ.
In other cases the data from descriptive research projects come in the form of a survey — a measure administered through either an interview or a written questionnaire to get a picture of the beliefs or behaviors of a sample of people of interest . The people chosen to participate in the research (known as the sample ) are selected to be representative of all the people that the researcher wishes to know about (the population ). In election polls, for instance, a sample is taken from the population of all “likely voters” in the upcoming elections.
The results of surveys may sometimes be rather mundane, such as “Nine out of ten doctors prefer Tymenocin,” or “The median income in Montgomery County is $36,712.” Yet other times (particularly in discussions of social behavior), the results can be shocking: “More than 40,000 people are killed by gunfire in the United States every year,” or “More than 60% of women between the ages of 50 and 60 suffer from depression.” Descriptive research is frequently used by psychologists to get an estimate of the prevalence (or incidence ) of psychological disorders.
A final type of descriptive research—known as naturalistic observation —is research based on the observation of everyday events . For instance, a developmental psychologist who watches children on a playground and describes what they say to each other while they play is conducting descriptive research, as is a biopsychologist who observes animals in their natural habitats. One example of observational research involves a systematic procedure known as the strange situation , used to get a picture of how adults and young children interact. The data that are collected in the strange situation are systematically coded in a coding sheet such as that shown in Table 2.3 “Sample Coding Form Used to Assess Child’s and Mother’s Behavior in the Strange Situation” .
Table 2.3 Sample Coding Form Used to Assess Child’s and Mother’s Behavior in the Strange Situation
Coder name: | ||||
---|---|---|---|---|
Mother and baby play alone | ||||
Mother puts baby down | ||||
Stranger enters room | ||||
Mother leaves room; stranger plays with baby | ||||
Mother reenters, greets and may comfort baby, then leaves again | ||||
Stranger tries to play with baby | ||||
Mother reenters and picks up baby | ||||
The baby moves toward, grasps, or climbs on the adult. | ||||
The baby resists being put down by the adult by crying or trying to climb back up. | ||||
The baby pushes, hits, or squirms to be put down from the adult’s arms. | ||||
The baby turns away or moves away from the adult. | ||||
This table represents a sample coding sheet from an episode of the “strange situation,” in which an infant (usually about 1 year old) is observed playing in a room with two adults—the child’s mother and a stranger. Each of the four coding categories is scored by the coder from 1 (the baby makes no effort to engage in the behavior) to 7 (the baby makes a significant effort to engage in the behavior). More information about the meaning of the coding can be found in Ainsworth, Blehar, Waters, and Wall (1978). |
The results of descriptive research projects are analyzed using descriptive statistics — numbers that summarize the distribution of scores on a measured variable . Most variables have distributions similar to that shown in Figure 2.5 “Height Distribution” , where most of the scores are located near the center of the distribution, and the distribution is symmetrical and bell-shaped. A data distribution that is shaped like a bell is known as a normal distribution .
Table 2.4 Height and Family Income for 25 Students
Student name | Height in inches | Family income in dollars |
---|---|---|
Lauren | 62 | 48,000 |
Courtnie | 62 | 57,000 |
Leslie | 63 | 93,000 |
Renee | 64 | 107,000 |
Katherine | 64 | 110,000 |
Jordan | 65 | 93,000 |
Rabiah | 66 | 46,000 |
Alina | 66 | 84,000 |
Young Su | 67 | 68,000 |
Martin | 67 | 49,000 |
Hanzhu | 67 | 73,000 |
Caitlin | 67 | 3,800,000 |
Steven | 67 | 107,000 |
Emily | 67 | 64,000 |
Amy | 68 | 67,000 |
Jonathan | 68 | 51,000 |
Julian | 68 | 48,000 |
Alissa | 68 | 93,000 |
Christine | 69 | 93,000 |
Candace | 69 | 111,000 |
Xiaohua | 69 | 56,000 |
Charlie | 70 | 94,000 |
Timothy | 71 | 73,000 |
Ariane | 72 | 70,000 |
Logan | 72 | 44,000 |
Figure 2.5 Height Distribution
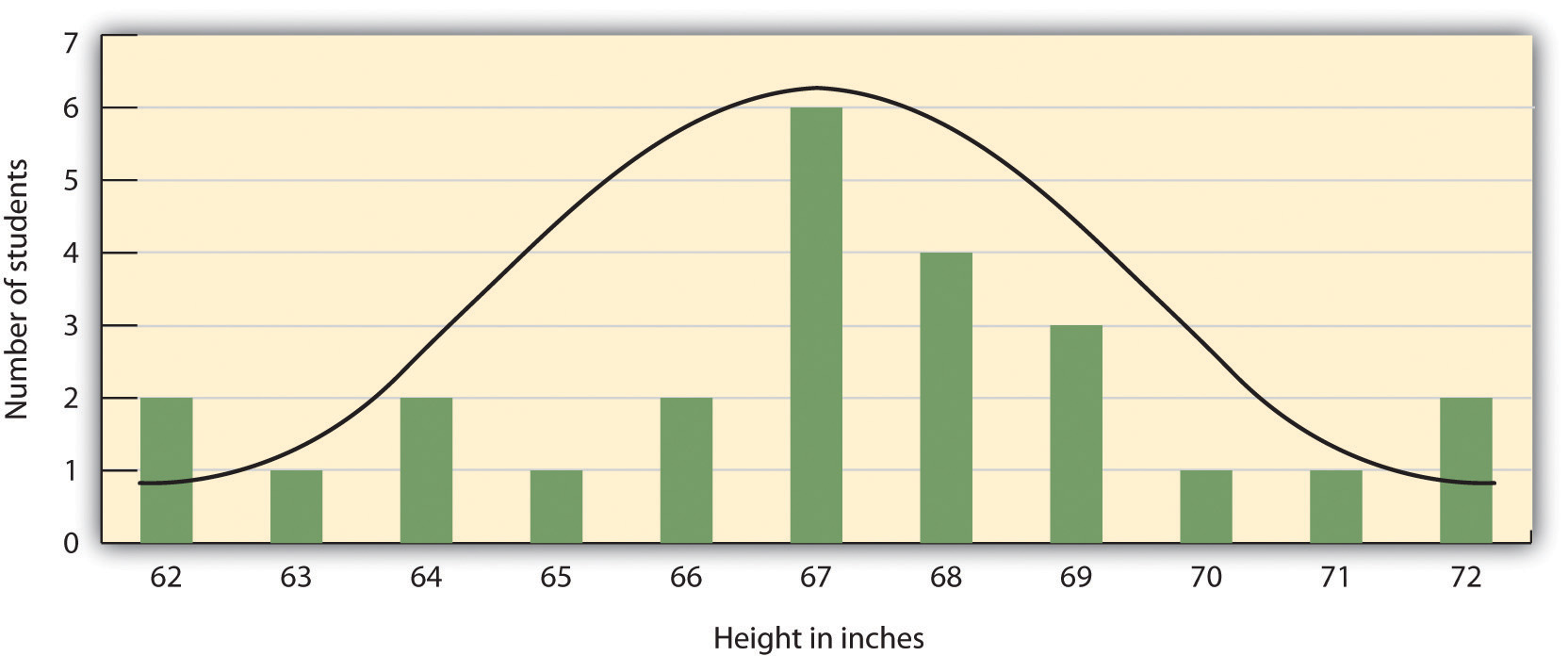
The distribution of the heights of the students in a class will form a normal distribution. In this sample the mean ( M ) = 67.12 and the standard deviation ( s ) = 2.74.
A distribution can be described in terms of its central tendency —that is, the point in the distribution around which the data are centered—and its dispersion , or spread. The arithmetic average, or arithmetic mean , is the most commonly used measure of central tendency . It is computed by calculating the sum of all the scores of the variable and dividing this sum by the number of participants in the distribution (denoted by the letter N ). In the data presented in Figure 2.5 “Height Distribution” , the mean height of the students is 67.12 inches. The sample mean is usually indicated by the letter M .
In some cases, however, the data distribution is not symmetrical. This occurs when there are one or more extreme scores (known as outliers ) at one end of the distribution. Consider, for instance, the variable of family income (see Figure 2.6 “Family Income Distribution” ), which includes an outlier (a value of $3,800,000). In this case the mean is not a good measure of central tendency. Although it appears from Figure 2.6 “Family Income Distribution” that the central tendency of the family income variable should be around $70,000, the mean family income is actually $223,960. The single very extreme income has a disproportionate impact on the mean, resulting in a value that does not well represent the central tendency.
The median is used as an alternative measure of central tendency when distributions are not symmetrical. The median is the score in the center of the distribution, meaning that 50% of the scores are greater than the median and 50% of the scores are less than the median . In our case, the median household income ($73,000) is a much better indication of central tendency than is the mean household income ($223,960).
Figure 2.6 Family Income Distribution
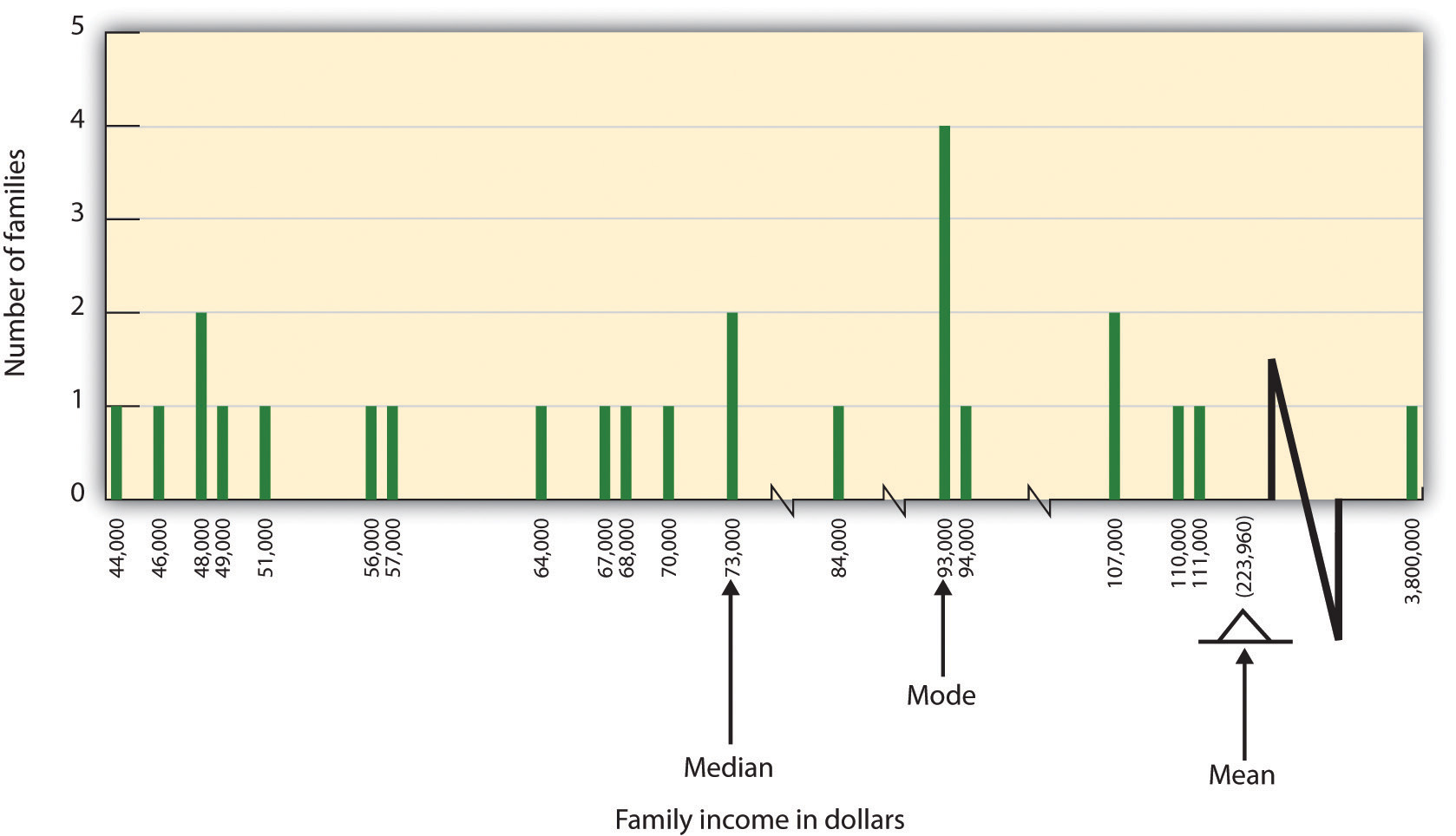
The distribution of family incomes is likely to be nonsymmetrical because some incomes can be very large in comparison to most incomes. In this case the median or the mode is a better indicator of central tendency than is the mean.
A final measure of central tendency, known as the mode , represents the value that occurs most frequently in the distribution . You can see from Figure 2.6 “Family Income Distribution” that the mode for the family income variable is $93,000 (it occurs four times).
In addition to summarizing the central tendency of a distribution, descriptive statistics convey information about how the scores of the variable are spread around the central tendency. Dispersion refers to the extent to which the scores are all tightly clustered around the central tendency, like this:
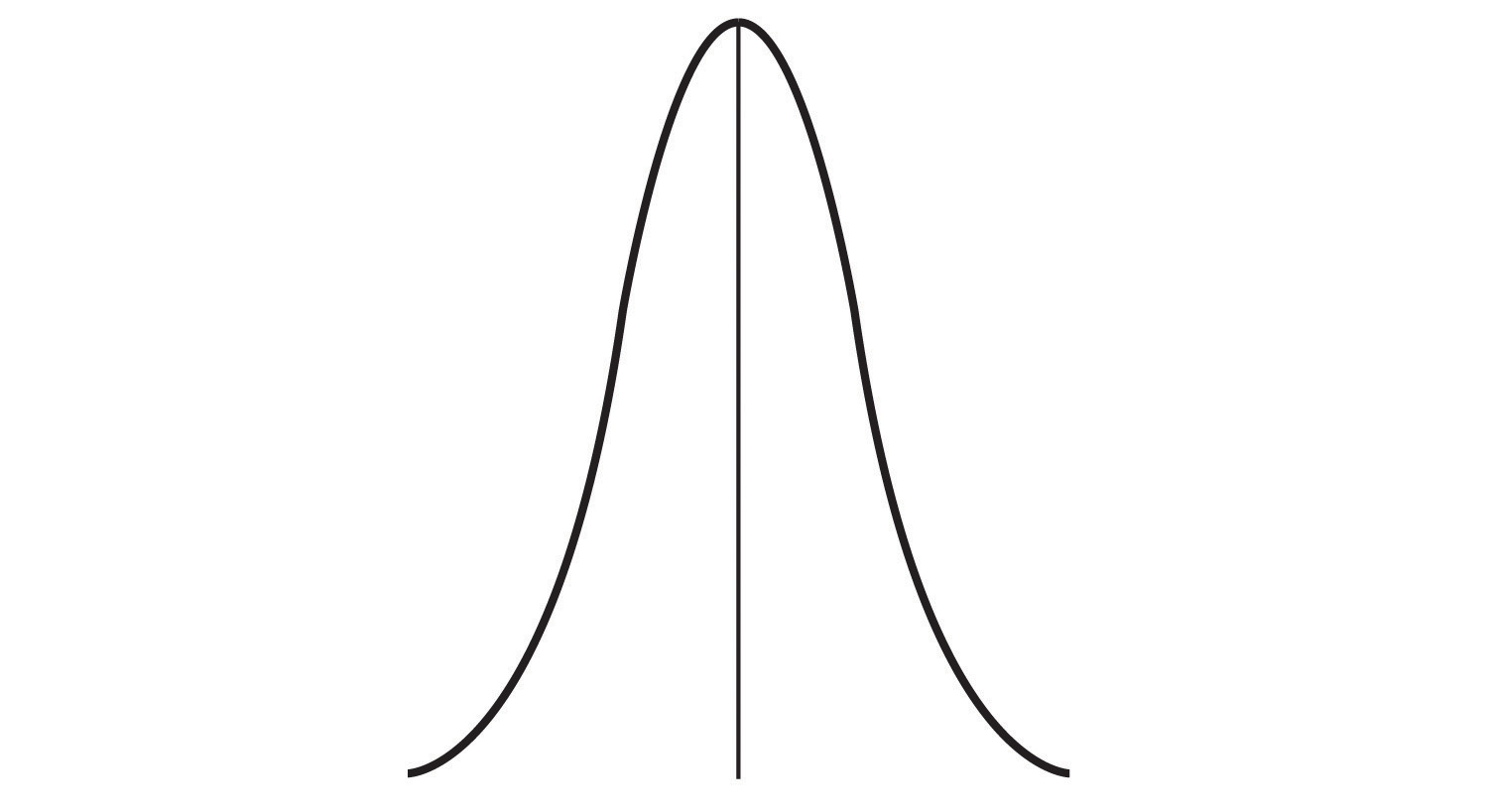
Or they may be more spread out away from it, like this:
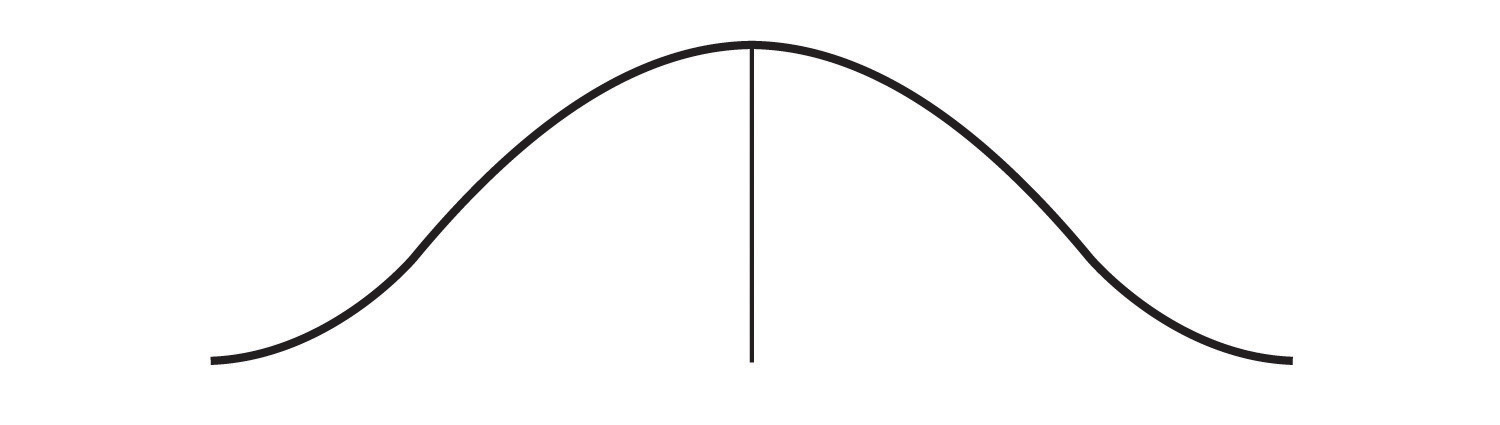
One simple measure of dispersion is to find the largest (the maximum ) and the smallest (the minimum ) observed values of the variable and to compute the range of the variable as the maximum observed score minus the minimum observed score. You can check that the range of the height variable in Figure 2.5 “Height Distribution” is 72 – 62 = 10. The standard deviation , symbolized as s , is the most commonly used measure of dispersion . Distributions with a larger standard deviation have more spread. The standard deviation of the height variable is s = 2.74, and the standard deviation of the family income variable is s = $745,337.
An advantage of descriptive research is that it attempts to capture the complexity of everyday behavior. Case studies provide detailed information about a single person or a small group of people, surveys capture the thoughts or reported behaviors of a large population of people, and naturalistic observation objectively records the behavior of people or animals as it occurs naturally. Thus descriptive research is used to provide a relatively complete understanding of what is currently happening.
Despite these advantages, descriptive research has a distinct disadvantage in that, although it allows us to get an idea of what is currently happening, it is usually limited to static pictures. Although descriptions of particular experiences may be interesting, they are not always transferable to other individuals in other situations, nor do they tell us exactly why specific behaviors or events occurred. For instance, descriptions of individuals who have suffered a stressful event, such as a war or an earthquake, can be used to understand the individuals’ reactions to the event but cannot tell us anything about the long-term effects of the stress. And because there is no comparison group that did not experience the stressful situation, we cannot know what these individuals would be like if they hadn’t had the stressful experience.
Correlational Research: Seeking Relationships Among Variables
In contrast to descriptive research, which is designed primarily to provide static pictures, correlational research involves the measurement of two or more relevant variables and an assessment of the relationship between or among those variables. For instance, the variables of height and weight are systematically related (correlated) because taller people generally weigh more than shorter people. In the same way, study time and memory errors are also related, because the more time a person is given to study a list of words, the fewer errors he or she will make. When there are two variables in the research design, one of them is called the predictor variable and the other the outcome variable . The research design can be visualized like this, where the curved arrow represents the expected correlation between the two variables:
Figure 2.2.2

One way of organizing the data from a correlational study with two variables is to graph the values of each of the measured variables using a scatter plot . As you can see in Figure 2.10 “Examples of Scatter Plots” , a scatter plot is a visual image of the relationship between two variables . A point is plotted for each individual at the intersection of his or her scores for the two variables. When the association between the variables on the scatter plot can be easily approximated with a straight line, as in parts (a) and (b) of Figure 2.10 “Examples of Scatter Plots” , the variables are said to have a linear relationship .
When the straight line indicates that individuals who have above-average values for one variable also tend to have above-average values for the other variable, as in part (a), the relationship is said to be positive linear . Examples of positive linear relationships include those between height and weight, between education and income, and between age and mathematical abilities in children. In each case people who score higher on one of the variables also tend to score higher on the other variable. Negative linear relationships , in contrast, as shown in part (b), occur when above-average values for one variable tend to be associated with below-average values for the other variable. Examples of negative linear relationships include those between the age of a child and the number of diapers the child uses, and between practice on and errors made on a learning task. In these cases people who score higher on one of the variables tend to score lower on the other variable.
Relationships between variables that cannot be described with a straight line are known as nonlinear relationships . Part (c) of Figure 2.10 “Examples of Scatter Plots” shows a common pattern in which the distribution of the points is essentially random. In this case there is no relationship at all between the two variables, and they are said to be independent . Parts (d) and (e) of Figure 2.10 “Examples of Scatter Plots” show patterns of association in which, although there is an association, the points are not well described by a single straight line. For instance, part (d) shows the type of relationship that frequently occurs between anxiety and performance. Increases in anxiety from low to moderate levels are associated with performance increases, whereas increases in anxiety from moderate to high levels are associated with decreases in performance. Relationships that change in direction and thus are not described by a single straight line are called curvilinear relationships .
Figure 2.10 Examples of Scatter Plots
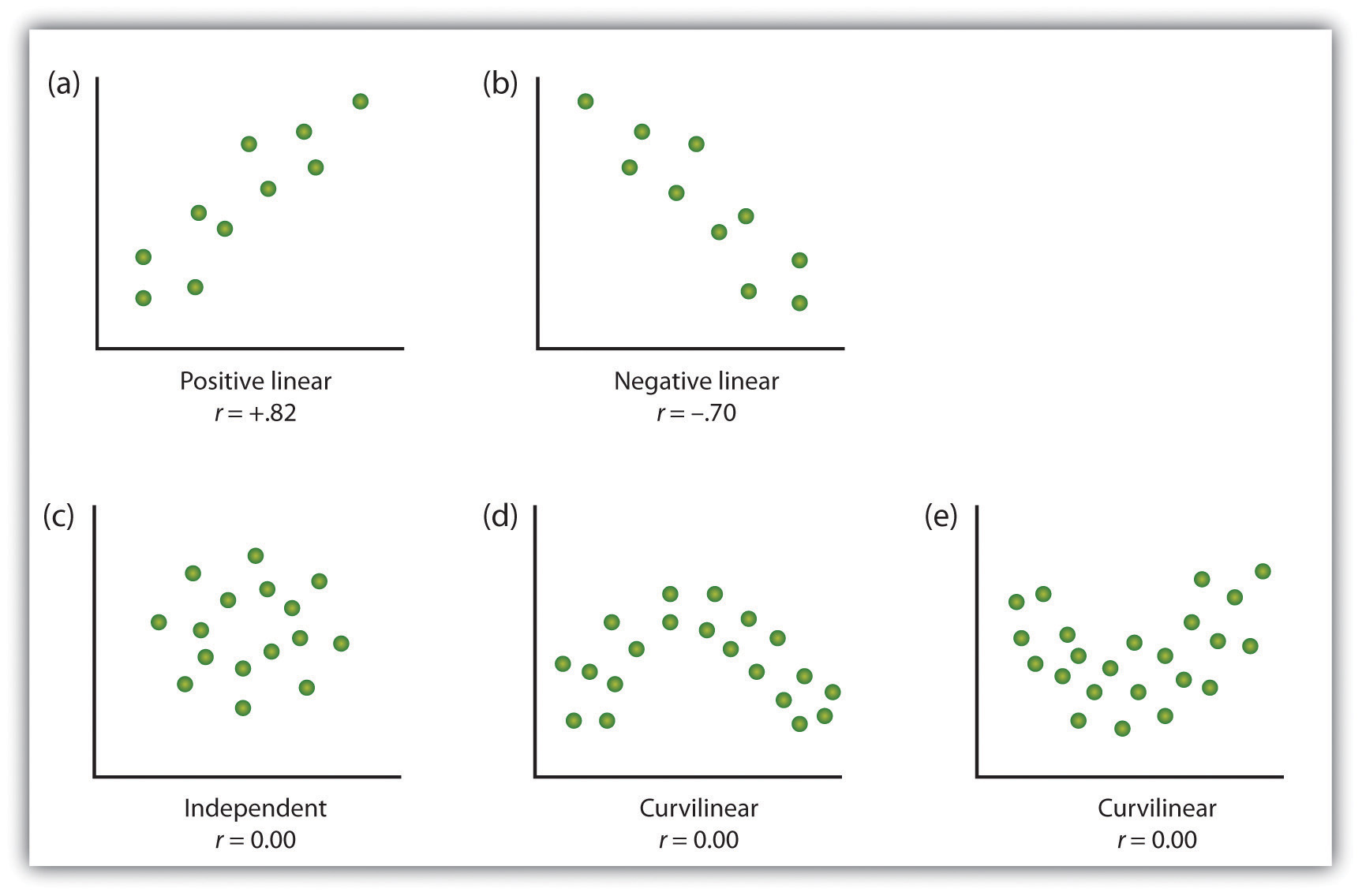
Some examples of relationships between two variables as shown in scatter plots. Note that the Pearson correlation coefficient ( r ) between variables that have curvilinear relationships will likely be close to zero.
Adapted from Stangor, C. (2011). Research methods for the behavioral sciences (4th ed.). Mountain View, CA: Cengage.
The most common statistical measure of the strength of linear relationships among variables is the Pearson correlation coefficient , which is symbolized by the letter r . The value of the correlation coefficient ranges from r = –1.00 to r = +1.00. The direction of the linear relationship is indicated by the sign of the correlation coefficient. Positive values of r (such as r = .54 or r = .67) indicate that the relationship is positive linear (i.e., the pattern of the dots on the scatter plot runs from the lower left to the upper right), whereas negative values of r (such as r = –.30 or r = –.72) indicate negative linear relationships (i.e., the dots run from the upper left to the lower right). The strength of the linear relationship is indexed by the distance of the correlation coefficient from zero (its absolute value). For instance, r = –.54 is a stronger relationship than r = .30, and r = .72 is a stronger relationship than r = –.57. Because the Pearson correlation coefficient only measures linear relationships, variables that have curvilinear relationships are not well described by r , and the observed correlation will be close to zero.
It is also possible to study relationships among more than two measures at the same time. A research design in which more than one predictor variable is used to predict a single outcome variable is analyzed through multiple regression (Aiken & West, 1991). Multiple regression is a statistical technique, based on correlation coefficients among variables, that allows predicting a single outcome variable from more than one predictor variable . For instance, Figure 2.11 “Prediction of Job Performance From Three Predictor Variables” shows a multiple regression analysis in which three predictor variables are used to predict a single outcome. The use of multiple regression analysis shows an important advantage of correlational research designs—they can be used to make predictions about a person’s likely score on an outcome variable (e.g., job performance) based on knowledge of other variables.
Figure 2.11 Prediction of Job Performance From Three Predictor Variables
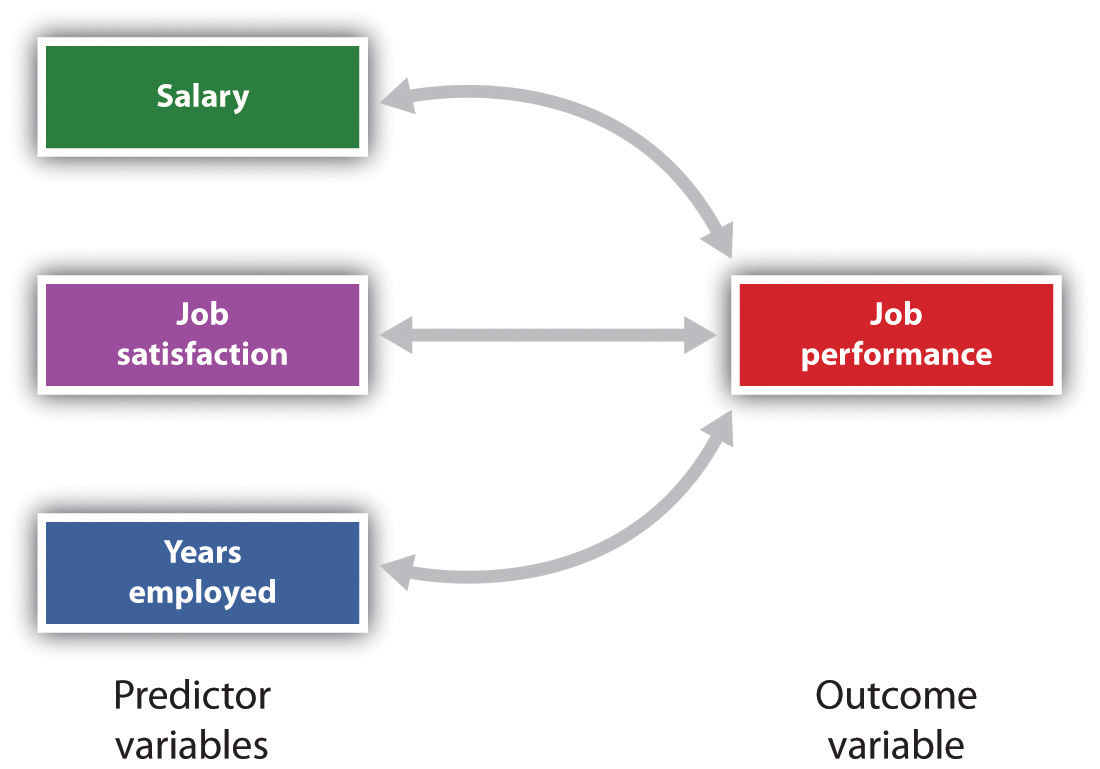
Multiple regression allows scientists to predict the scores on a single outcome variable using more than one predictor variable.
An important limitation of correlational research designs is that they cannot be used to draw conclusions about the causal relationships among the measured variables. Consider, for instance, a researcher who has hypothesized that viewing violent behavior will cause increased aggressive play in children. He has collected, from a sample of fourth-grade children, a measure of how many violent television shows each child views during the week, as well as a measure of how aggressively each child plays on the school playground. From his collected data, the researcher discovers a positive correlation between the two measured variables.
Although this positive correlation appears to support the researcher’s hypothesis, it cannot be taken to indicate that viewing violent television causes aggressive behavior. Although the researcher is tempted to assume that viewing violent television causes aggressive play,

there are other possibilities. One alternate possibility is that the causal direction is exactly opposite from what has been hypothesized. Perhaps children who have behaved aggressively at school develop residual excitement that leads them to want to watch violent television shows at home:

Although this possibility may seem less likely, there is no way to rule out the possibility of such reverse causation on the basis of this observed correlation. It is also possible that both causal directions are operating and that the two variables cause each other:

Still another possible explanation for the observed correlation is that it has been produced by the presence of a common-causal variable (also known as a third variable ). A common-causal variable is a variable that is not part of the research hypothesis but that causes both the predictor and the outcome variable and thus produces the observed correlation between them . In our example a potential common-causal variable is the discipline style of the children’s parents. Parents who use a harsh and punitive discipline style may produce children who both like to watch violent television and who behave aggressively in comparison to children whose parents use less harsh discipline:

In this case, television viewing and aggressive play would be positively correlated (as indicated by the curved arrow between them), even though neither one caused the other but they were both caused by the discipline style of the parents (the straight arrows). When the predictor and outcome variables are both caused by a common-causal variable, the observed relationship between them is said to be spurious . A spurious relationship is a relationship between two variables in which a common-causal variable produces and “explains away” the relationship . If effects of the common-causal variable were taken away, or controlled for, the relationship between the predictor and outcome variables would disappear. In the example the relationship between aggression and television viewing might be spurious because by controlling for the effect of the parents’ disciplining style, the relationship between television viewing and aggressive behavior might go away.
Common-causal variables in correlational research designs can be thought of as “mystery” variables because, as they have not been measured, their presence and identity are usually unknown to the researcher. Since it is not possible to measure every variable that could cause both the predictor and outcome variables, the existence of an unknown common-causal variable is always a possibility. For this reason, we are left with the basic limitation of correlational research: Correlation does not demonstrate causation. It is important that when you read about correlational research projects, you keep in mind the possibility of spurious relationships, and be sure to interpret the findings appropriately. Although correlational research is sometimes reported as demonstrating causality without any mention being made of the possibility of reverse causation or common-causal variables, informed consumers of research, like you, are aware of these interpretational problems.
In sum, correlational research designs have both strengths and limitations. One strength is that they can be used when experimental research is not possible because the predictor variables cannot be manipulated. Correlational designs also have the advantage of allowing the researcher to study behavior as it occurs in everyday life. And we can also use correlational designs to make predictions—for instance, to predict from the scores on their battery of tests the success of job trainees during a training session. But we cannot use such correlational information to determine whether the training caused better job performance. For that, researchers rely on experiments.
Experimental Research: Understanding the Causes of Behavior
The goal of experimental research design is to provide more definitive conclusions about the causal relationships among the variables in the research hypothesis than is available from correlational designs. In an experimental research design, the variables of interest are called the independent variable (or variables ) and the dependent variable . The independent variable in an experiment is the causing variable that is created (manipulated) by the experimenter . The dependent variable in an experiment is a measured variable that is expected to be influenced by the experimental manipulation . The research hypothesis suggests that the manipulated independent variable or variables will cause changes in the measured dependent variables. We can diagram the research hypothesis by using an arrow that points in one direction. This demonstrates the expected direction of causality:
Figure 2.2.3

Research Focus: Video Games and Aggression
Consider an experiment conducted by Anderson and Dill (2000). The study was designed to test the hypothesis that viewing violent video games would increase aggressive behavior. In this research, male and female undergraduates from Iowa State University were given a chance to play with either a violent video game (Wolfenstein 3D) or a nonviolent video game (Myst). During the experimental session, the participants played their assigned video games for 15 minutes. Then, after the play, each participant played a competitive game with an opponent in which the participant could deliver blasts of white noise through the earphones of the opponent. The operational definition of the dependent variable (aggressive behavior) was the level and duration of noise delivered to the opponent. The design of the experiment is shown in Figure 2.17 “An Experimental Research Design” .
Figure 2.17 An Experimental Research Design
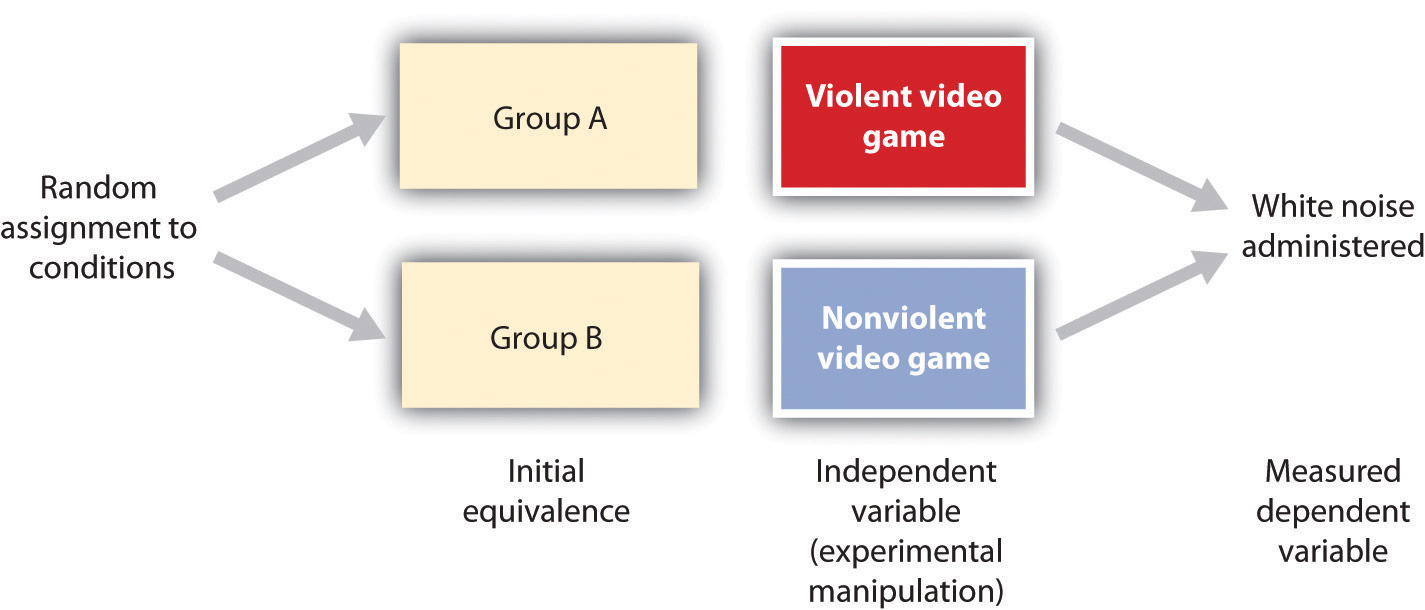
Two advantages of the experimental research design are (1) the assurance that the independent variable (also known as the experimental manipulation) occurs prior to the measured dependent variable, and (2) the creation of initial equivalence between the conditions of the experiment (in this case by using random assignment to conditions).
Experimental designs have two very nice features. For one, they guarantee that the independent variable occurs prior to the measurement of the dependent variable. This eliminates the possibility of reverse causation. Second, the influence of common-causal variables is controlled, and thus eliminated, by creating initial equivalence among the participants in each of the experimental conditions before the manipulation occurs.
The most common method of creating equivalence among the experimental conditions is through random assignment to conditions , a procedure in which the condition that each participant is assigned to is determined through a random process, such as drawing numbers out of an envelope or using a random number table . Anderson and Dill first randomly assigned about 100 participants to each of their two groups (Group A and Group B). Because they used random assignment to conditions, they could be confident that, before the experimental manipulation occurred, the students in Group A were, on average, equivalent to the students in Group B on every possible variable, including variables that are likely to be related to aggression, such as parental discipline style, peer relationships, hormone levels, diet—and in fact everything else.
Then, after they had created initial equivalence, Anderson and Dill created the experimental manipulation—they had the participants in Group A play the violent game and the participants in Group B play the nonviolent game. Then they compared the dependent variable (the white noise blasts) between the two groups, finding that the students who had viewed the violent video game gave significantly longer noise blasts than did the students who had played the nonviolent game.
Anderson and Dill had from the outset created initial equivalence between the groups. This initial equivalence allowed them to observe differences in the white noise levels between the two groups after the experimental manipulation, leading to the conclusion that it was the independent variable (and not some other variable) that caused these differences. The idea is that the only thing that was different between the students in the two groups was the video game they had played.
Despite the advantage of determining causation, experiments do have limitations. One is that they are often conducted in laboratory situations rather than in the everyday lives of people. Therefore, we do not know whether results that we find in a laboratory setting will necessarily hold up in everyday life. Second, and more important, is that some of the most interesting and key social variables cannot be experimentally manipulated. If we want to study the influence of the size of a mob on the destructiveness of its behavior, or to compare the personality characteristics of people who join suicide cults with those of people who do not join such cults, these relationships must be assessed using correlational designs, because it is simply not possible to experimentally manipulate these variables.
Key Takeaways
- Descriptive, correlational, and experimental research designs are used to collect and analyze data.
- Descriptive designs include case studies, surveys, and naturalistic observation. The goal of these designs is to get a picture of the current thoughts, feelings, or behaviors in a given group of people. Descriptive research is summarized using descriptive statistics.
- Correlational research designs measure two or more relevant variables and assess a relationship between or among them. The variables may be presented on a scatter plot to visually show the relationships. The Pearson Correlation Coefficient ( r ) is a measure of the strength of linear relationship between two variables.
- Common-causal variables may cause both the predictor and outcome variable in a correlational design, producing a spurious relationship. The possibility of common-causal variables makes it impossible to draw causal conclusions from correlational research designs.
- Experimental research involves the manipulation of an independent variable and the measurement of a dependent variable. Random assignment to conditions is normally used to create initial equivalence between the groups, allowing researchers to draw causal conclusions.
Exercises and Critical Thinking
- There is a negative correlation between the row that a student sits in in a large class (when the rows are numbered from front to back) and his or her final grade in the class. Do you think this represents a causal relationship or a spurious relationship, and why?
- Think of two variables (other than those mentioned in this book) that are likely to be correlated, but in which the correlation is probably spurious. What is the likely common-causal variable that is producing the relationship?
- Imagine a researcher wants to test the hypothesis that participating in psychotherapy will cause a decrease in reported anxiety. Describe the type of research design the investigator might use to draw this conclusion. What would be the independent and dependent variables in the research?
Aiken, L., & West, S. (1991). Multiple regression: Testing and interpreting interactions . Newbury Park, CA: Sage.
Ainsworth, M. S., Blehar, M. C., Waters, E., & Wall, S. (1978). Patterns of attachment: A psychological study of the strange situation . Hillsdale, NJ: Lawrence Erlbaum Associates.
Anderson, C. A., & Dill, K. E. (2000). Video games and aggressive thoughts, feelings, and behavior in the laboratory and in life. Journal of Personality and Social Psychology, 78 (4), 772–790.
Damasio, H., Grabowski, T., Frank, R., Galaburda, A. M., Damasio, A. R., Cacioppo, J. T., & Berntson, G. G. (2005). The return of Phineas Gage: Clues about the brain from the skull of a famous patient. In Social neuroscience: Key readings. (pp. 21–28). New York, NY: Psychology Press.
Freud, S. (1964). Analysis of phobia in a five-year-old boy. In E. A. Southwell & M. Merbaum (Eds.), Personality: Readings in theory and research (pp. 3–32). Belmont, CA: Wadsworth. (Original work published 1909)
Kotowicz, Z. (2007). The strange case of Phineas Gage. History of the Human Sciences, 20 (1), 115–131.
Rokeach, M. (1964). The three Christs of Ypsilanti: A psychological study . New York, NY: Knopf.
Introduction to Psychology Copyright © 2015 by University of Minnesota is licensed under a Creative Commons Attribution-NonCommercial-ShareAlike 4.0 International License , except where otherwise noted.
Live revision! Join us for our free exam revision livestreams Watch now →
Reference Library
Collections
- See what's new
- All Resources
- Student Resources
- Assessment Resources
- Teaching Resources
- CPD Courses
- Livestreams
Study notes, videos, interactive activities and more!
Psychology news, insights and enrichment
Currated collections of free resources
Browse resources by topic
- All Psychology Resources
Resource Selections
Currated lists of resources
Positive Correlation
A positive correlation occurs when two variables are related and as one variable increases/decreases the other also increases/decreases (i.e. they both move in the same direction). For example, you might expect to find a positive correlation between height and shoe size.
- Share on Facebook
- Share on Twitter
- Share by Email
Correlations
Study Notes
Research Methods - Correlations
Quizzes & Activities
Research Methods: MCQ Revision Test 1 for AQA A Level Psychology
Topic Videos
Example Answers for Research Methods: A Level Psychology, Paper 2, June 2018 (AQA)
Exam Support
A Level Psychology Topic Quiz - Research Methods
Our subjects.
- › Criminology
- › Economics
- › Geography
- › Health & Social Care
- › Psychology
- › Sociology
- › Teaching & learning resources
- › Student revision workshops
- › Online student courses
- › CPD for teachers
- › Livestreams
- › Teaching jobs
Boston House, 214 High Street, Boston Spa, West Yorkshire, LS23 6AD Tel: 01937 848885
- › Contact us
- › Terms of use
- › Privacy & cookies
© 2002-2024 Tutor2u Limited. Company Reg no: 04489574. VAT reg no 816865400.

Positive Correlation
Ai generator.
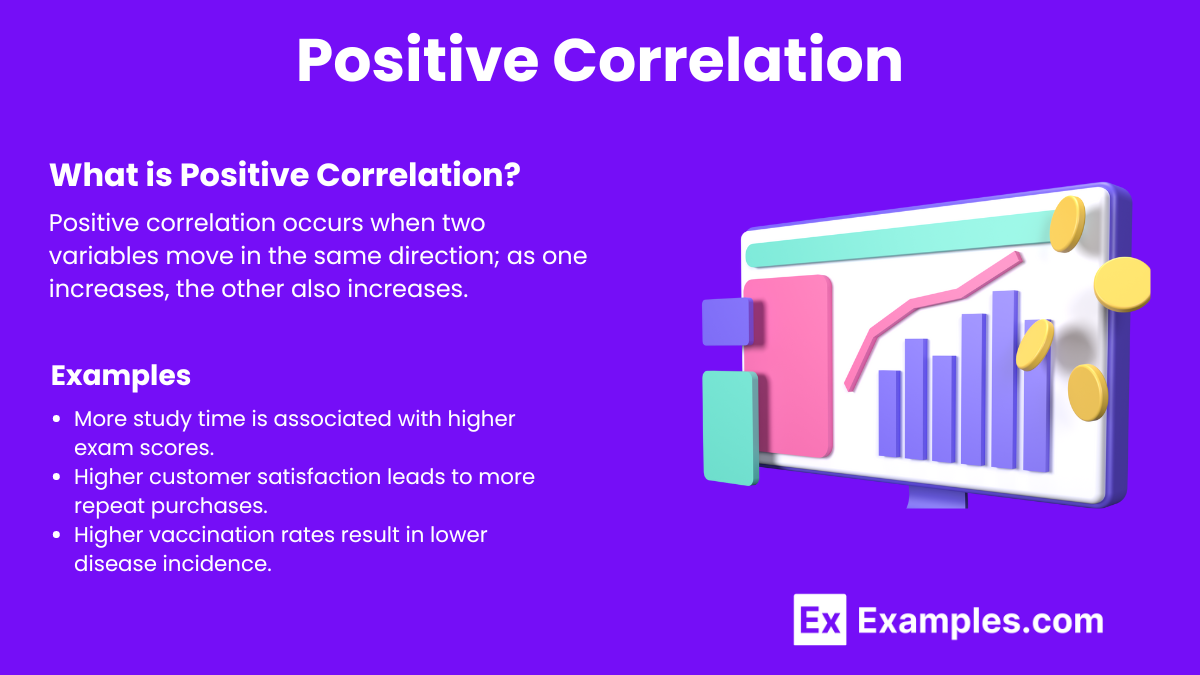
Understanding positive correlation is essential for interpreting relationships between variables in various fields, including economics, psychology, and natural sciences. A positive correlation indicates that as one variable increases, the other variable also increases. This relationship provides valuable insights into how interconnected factors behave and influence each other. Researchers and analysts use Scatter Plots , statistical methods to quantify the strength of these correlations, guiding decision-making and theoretical advancements. In this article, we will explore the concept of positive correlation, negative correlation its significance in data analysis, and its implications in real-world scenarios.
What is Positive Correlation?
A positive correlation exists when two variables move in the same direction, meaning that as one variable increases, the other variable also increases. This relationship is typically represented by a correlation coefficient that ranges from +0.01 to +1.00. A Correlation Hypothesis coefficient close to +1.00 indicates a strong positive correlation, where the variables show a direct and consistent relationship. This type of correlation is often visualized through a scatterplot where the points trend upwards as you move from left to right.
Positive Correlation Examples
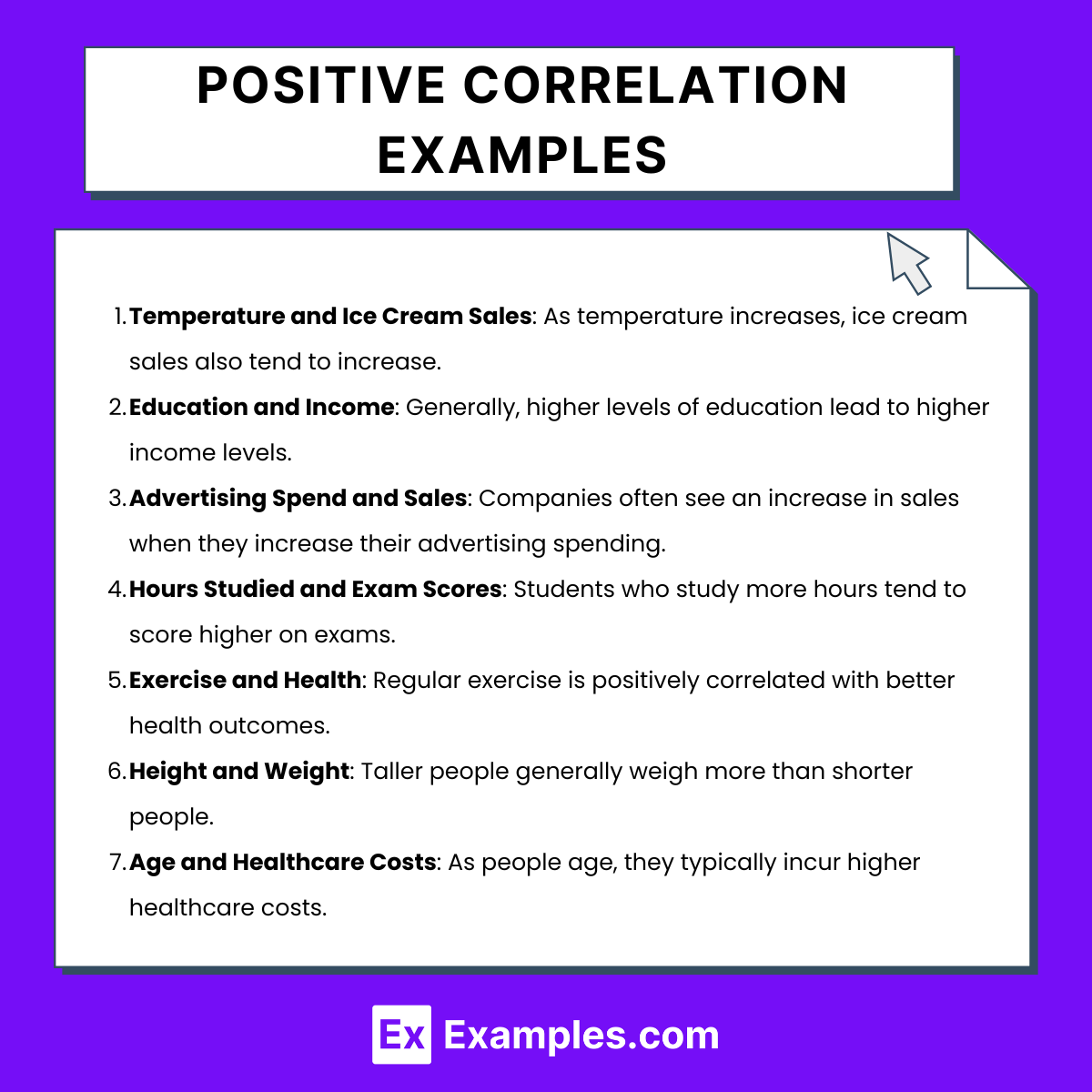
- Temperature and Ice Cream Sales : As temperature increases, ice cream sales also tend to increase.
- Education and Income : Generally, higher levels of education lead to higher income levels.
- Advertising Spend and Sales : Companies often see an increase in sales when they increase their advertising spending.
- Hours Studied and Exam Scores : Students who study more hours tend to score higher on exams.
- Exercise and Health : Regular exercise is positively correlated with better health outcomes.
- Height and Weight : Taller people generally weigh more than shorter people.
- Age and Healthcare Costs : As people age, they typically incur higher healthcare costs.
- Speed and Travel Time : Faster travel speeds usually result in shorter travel times.
- Population Density and Traffic : Areas with higher population density often experience more traffic congestion.
- Social Media Use and Connectivity : More frequent use of social media is associated with higher levels of connectivity with friends and family.
- Practice Time and Musical Ability : Musicians who practice more often tend to have better musical skills.
- Investment in Technology and Productivity : Companies that invest in new technology often see improvements in productivity.
- Savings and Financial Security : Higher savings rates are correlated with greater financial security.
- Rainfall and Agricultural Yield : In many regions, more rainfall leads to higher agricultural yields.
- Sunlight Exposure and Vitamin D Levels : More exposure to sunlight typically increases Vitamin D levels in the body.
- Number of Employees and Output : Larger companies with more employees can often produce more goods or services.
- Internet Speed and Download Times : Faster internet speeds reduce the time it takes to download files.
- Car Engine Size and Fuel Consumption : Larger engines generally consume more fuel.
- Age and Wisdom : Older individuals often have more life experience and wisdom, although this can be subjective.
- Customer Satisfaction and Loyalty : Higher customer satisfaction often leads to greater customer loyalty and repeat business.
Positive Correlation Examples In Table
Field | Variables | Example |
---|---|---|
Education | Study Time & Exam Scores | More study time is associated with higher exam scores. |
Business | Customer Satisfaction & Repeat Purchases | Higher customer satisfaction leads to more repeat purchases. |
Public Health | Vaccination Rates & Disease Incidence | Higher vaccination rates result in lower disease incidence. |
Manufacturing | Machine Maintenance & Production Efficiency | Regular maintenance leads to higher production efficiency. |
Finance | Stock Market Performance & Economic Growth | Better stock market performance correlates with economic growth. |
Marketing | Advertising Expenditure & Sales Revenue | Increased advertising expenditure boosts sales revenue. |
Education | Interactive Teaching & Student Engagement | Interactive teaching methods increase student engagement. |
Healthcare | Exercise & Blood Pressure | Regular exercise is linked to lower blood pressure. |
Environment | Industrial Emissions & Air Pollution | Higher industrial emissions are associated with increased air pollution. |
Measuring Positive Correlation
Pearson correlation coefficient.
The Pearson correlation coefficient, denoted as rrr, quantifies the degree to which two variables are linearly related. It ranges from -1 to +1, where +1 indicates a perfect positive linear relationship, 0 indicates no linear relationship, and -1 indicates a perfect negative linear relationship.
Steps to Compute
- Collect Data : Ensure you have paired data for the two variables.
- Calculate Means : Find the mean of each variable.
- Compute Deviations and Product : For each pair of values, calculate the difference from their respective means, multiply these deviations for pairs, and sum the results.
- Calculate Covariance : Divide the sum of the products by the number of observations minus one.
- Calculate Standard Deviations : Find the standard deviation for each variable.
- Compute Pearson’s rrr : Use the formula provided.
Spearman’s Rank Correlation Coefficient
If your data are ordinal or not normally distributed, you might use Spearman’s rank correlation coefficient. It assesses how well the relationship between two variables can be described using a monotonic function.
Using Software Tools
Most statistical software and spreadsheet programs can compute these correlation coefficients quickly. Common tools include Excel, SPSS, R, and Python, each offering functions or packages that facilitate the computation of Pearson and Spearman correlation coefficients.
Types of Positive Correlation
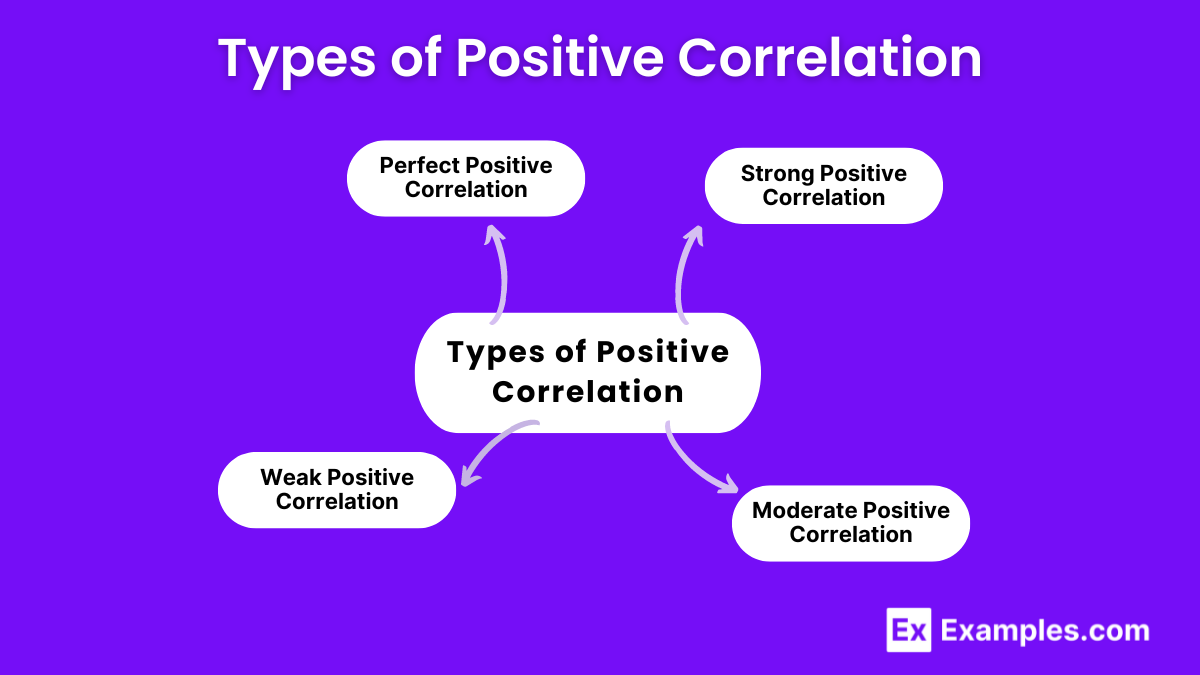
1. Perfect Positive Correlation
A perfect positive correlation occurs when two variables move in exactly the same proportion. If one variable increases by a certain percentage, the other variable also increases by the same percentage.
- Height and Weight: If a 10% increase in height leads to a 10% increase in weight, there is a perfect positive correlation between height and weight.
2. Strong Positive Correlation
A strong positive correlation means that the two variables have a high degree of association but are not perfectly correlated. Changes in one variable result in significant changes in the other, though not necessarily in the same proportion.
- Study Hours and Exam Scores: Students who spend more hours studying tend to score higher on exams, but the increase in scores may not be directly proportional to the increase in study hours.
3. Moderate Positive Correlation
In a moderate positive correlation, there is a noticeable, though not strong, relationship between two variables. While there is a positive trend, other factors may influence the variables.
- Exercise and Happiness Levels: People who exercise regularly tend to report higher levels of happiness, but other factors such as diet and sleep also play a role.
4. Weak Positive Correlation
A weak positive correlation indicates that there is a slight positive relationship between two variables. The variables tend to move in the same direction, but the relationship is not strong and can be easily influenced by other factors.
- Coffee Consumption and Productivity: Employees who drink coffee might show a slight increase in productivity, but the relationship is weak, In Simple Hypothesis factors can impact productivity.
How does positive correlation work?
Positive correlation occurs when two variables move in the same direction; as one variable increases, the other also increases, and as one decreases, the other decreases as well. This relationship is quantified by a correlation coefficient ranging from 0 to +1, where +1 indicates a perfect positive correlation. For example, in finance, if stock A and stock B have a positive correlation, an increase in the price of stock A is likely accompanied by an increase in the price of stock B. Positive correlation helps investors and analysts predict behavior, manage risk, and make more informed decisions by understanding how different assets or metrics are likely to interact.
Positive Correlation vs. Negative Correlation
Feature | Positive Correlation | Negative Correlation |
---|---|---|
As one variable increases, the other also increases. | As one variable increases, the other decreases. | |
The correlation coefficient (rrr) is positive (r>0r > 0r>0). | The correlation coefficient (rrr) is negative (r<0r < 0r<0). | |
The slope of the line of best fit is upward. | The slope of the line of best fit is downward. | |
Height and weight (typically, as height increases, weight increases). | Temperature and heating cost (as temperature increases, heating cost decreases). | |
Strength increases as rrr approaches +1. | Strength increases as rrr approaches -1. |
Limitations of Positive Correlation Analysis
1. Correlation Does Not Imply Causation : Correlation analysis can show that two variables move together, but it cannot determine whether one variable causes the other to change. This can lead to incorrect assumptions about cause-and-effect relationships.
2. Sensitivity to Outliers : Outliers, or extreme values, can skew correlation results, making the relationship appear stronger or weaker than it actually is. This can lead to misleading interpretations.
3. Assumes Linear Relationship : Correlation measures the strength of a linear relationship between two variables. If the relationship is non-linear, correlation analysis may not provide an accurate representation of the relationship.
4. Ignores the Influence of Other Variables : Correlation analysis looks at the relationship between only two variables, neglecting the potential impact of other factors. This can result in an incomplete understanding of the relationships within the data.
5. Spurious Correlation : Sometimes, two variables may show a correlation by chance or due to the influence of a third, unmeasured variable. This can create a false impression of a meaningful relationship.
6. Static Snapshot : Correlation analysis provides a static view of the relationship between variables, not accounting for changes over time. This limitation makes it less useful for understanding dynamic relationships.
Zero Correlation
In statistics, zero correlation describes a relationship between two variables where no association exists. When two variables have a zero correlation, changes in one variable do not predict changes in the other.
Zero correlation means that there is no linear relationship between two variables. The correlation coefficient, represented by rrr, is used to quantify this relationship. When r=0r = 0r=0, it indicates that there is no linear association between the variables.
Characteristics of Zero Correlation
- No Predictive Power : Changes in one variable provide no information about changes in the other.
- Scatter Plot : A scatter plot of two variables with zero correlation will show points randomly dispersed, with no discernible pattern.
- Linearity : Zero correlation specifically refers to the absence of a linear relationship. Non-linear relationships might still exist even if the linear correlation is zero.
Examples of Zero Correlation
To better understand zero correlation, consider the following examples:
- Height and Favorite Color : There is no relationship between a person’s height and their favorite color. Knowing someone’s height doesn’t help predict their favorite color.
- Shoe Size and Intelligence : A person’s shoe size does not predict their intelligence. There is no association between these two variables.
Why Zero Correlation Matters
- Research : Helps researchers understand whether variables influence each other.
- Business : Informs decisions by identifying unrelated factors.
- Education : Assists in developing teaching methods by identifying non-related factors.
Positive Correlations in Psychology Research
Positive correlations are a fundamental concept in psychology research, helping researchers understand the relationships between different variables. In Correlational Study , When two variables exhibit a positive correlation, it means that as one variable increases, the other also increases. This relationship is pivotal in various psychological studies and applications.
Importance of Positive Correlations in Psychology
- Identifying Relationships: Positive correlations help psychologists identify and understand the relationships between different psychological phenomena.
- Predicting Outcomes: By understanding these relationships, psychologists can predict outcomes based on the presence of certain variables.
- Formulating Hypotheses: Positive correlations provide a basis for forming hypotheses about how different factors influence behavior and mental processes.
When to Use Positive Correlations
Positive correlations are used in various fields to identify and understand the relationship between two variables. Here are some key instances when positive correlations are beneficial:
1. Predicting Outcomes
Positive correlations help predict the outcome of one variable based on another. For example, in education, if there’s a positive correlation between study time and exam scores, educators can predict that students who study more tend to score higher.
2. Identifying Trends
Businesses and researchers use positive correlations to identify trends and patterns. For instance, companies might find a positive correlation between customer satisfaction and repeat purchases, indicating that happier customers are more likely to return.
3. Making Data-Driven Decisions
Positive correlations provide evidence for making informed decisions. For example, public health officials might observe a positive correlation between vaccination rates and reduced disease incidence, guiding them to promote vaccinations more vigorously.
4. Improving Processes
In manufacturing, positive correlations between machine maintenance and production efficiency can help identify practices that enhance productivity. Regular maintenance can be linked to fewer breakdowns and higher output.
5. Understanding Relationships
Researchers in social sciences use positive correlations to understand relationships between variables. For example, a positive correlation between physical activity and mental health suggests that increased exercise is associated with better mental well-being.
6. Financial Analysis
In finance, positive correlations are crucial for portfolio management. For instance, a positive correlation between stock market performance and economic growth helps investors make strategic investment choices.
7. Marketing Strategies
Marketers analyze positive correlations to refine their strategies. For example, a positive correlation between advertising expenditure and sales revenue can justify increased spending on advertising campaigns.
8. Educational Research
Educational researchers use positive correlations to study the impact of various teaching methods on student performance. For example, a positive correlation between interactive teaching techniques and student engagement can lead to the adoption of more interactive methods in classrooms.
9. Healthcare Studies
In healthcare, positive correlations between lifestyle factors and health outcomes guide interventions. For instance, a positive correlation between regular exercise and lower blood pressure can promote exercise programs for hypertension patients.
10. Environmental Studies
Environmental scientists use positive correlations to study the impact of human activities on the environment. For example, a positive correlation between industrial emissions and air pollution levels can drive policies to reduce emissions.
Applications of Positive Correlation
1. economics and finance.
Positive correlation plays a significant role in economics and finance by helping analysts and investors understand the relationships between different economic indicators and financial assets.
- Stock Prices and Economic Growth: When the economy grows, stock prices generally increase. Investors use this correlation to make investment decisions.
- Consumer Spending and Income: As household income rises, consumer spending typically increases. This correlation helps businesses forecast sales and manage inventory.
2. Education
In education, positive correlation can be used to understand the relationship between different educational variables and outcomes.
- Study Time and Academic Performance: Students who spend more time studying tend to have higher academic performance. Educators can use this information to encourage effective study habits.
- Teacher Experience and Student Achievement: More experienced teachers often lead to better student achievement. This correlation can guide hiring practices and professional development programs.
3. Healthcare
Healthcare professionals use positive correlation to identify relationships between lifestyle factors and health outcomes, which aids in preventive measures and treatment planning.
- Exercise and Physical Health: Regular physical activity is positively correlated with better physical health. Public health campaigns often promote exercise to improve community health.
- Diet and Chronic Diseases: Healthy diets are associated with lower rates of chronic diseases such as diabetes and heart disease. Nutritionists use this correlation to advise patients on dietary choices.
4. Marketing
Marketers utilize positive correlation to understand consumer behavior and optimize marketing strategies.
- Advertising and Sales: Increased advertising expenditure is often correlated with higher sales. Companies allocate budgets to advertising campaigns based on this relationship.
- Customer Satisfaction and Loyalty: Higher customer satisfaction is positively correlated with customer loyalty. Businesses focus on improving satisfaction to retain customers.
5. Environmental Studies
In environmental science, positive correlation helps in understanding the impact of human activities on the environment and developing sustainable practices.
- Carbon Emissions and Global Warming: Higher carbon emissions are correlated with global warming. Policymakers use this correlation to implement regulations aimed at reducing emissions.
- Deforestation and Biodiversity Loss: Increased deforestation leads to a loss of biodiversity. Conservation efforts are guided by this relationship to protect endangered species.
6. Social Sciences
Social scientists use positive correlation to study human behavior and social phenomena.
- Education Level and Income: Higher levels of education are generally associated with higher income levels. This correlation informs policies aimed at improving educational access and reducing poverty.
- Social Media Usage and Social Connectivity: Increased use of social media is correlated with higher levels of social connectivity. Researchers study this relationship to understand the impact of social media on society.
What is positive correlation?
Positive correlation occurs when two variables move in the same direction; as one increases, the other also increases.
How is positive correlation measured?
Positive correlation is measured using the correlation coefficient, ranging from 0 to +1, where +1 indicates a perfect positive correlation.
What is an example of positive correlation?
An example of positive correlation is the relationship between hours studied and test scores; more study hours usually lead to higher scores.
Why is positive correlation important?
Positive correlation helps identify relationships between variables, aiding in predictions and decision-making processes in various fields.
What does a correlation coefficient of +0.8 indicate?
A correlation coefficient of +0.8 indicates a strong positive correlation between two variables.
Can positive correlation be causal?
Positive correlation does not imply causation; it only indicates a relationship between variables, not that one causes the other.
What are some fields that use positive correlation?
Fields such as finance, economics, psychology, and medicine use positive correlation to analyze data and predict trends.
How does positive correlation differ from negative correlation?
Positive correlation means variables move in the same direction, while negative correlation means they move in opposite directions.
What is a weak positive correlation?
A weak positive correlation is when the correlation coefficient is close to 0, indicating a slight relationship between variables.
How can you visualize positive correlation?
Positive correlation can be visualized using scatter plots, where points form an upward sloping pattern from left to right.
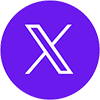
Text prompt
- Instructive
- Professional
10 Examples of Public speaking
20 Examples of Gas lighting
2. Psychological Research
Analyzing findings, learning objectives.
By the end of this section, you will be able to:
- Explain what a correlation coefficient tells us about the relationship between variables
- Recognize that correlation does not indicate a cause-and-effect relationship between variables
- Discuss our tendency to look for relationships between variables that do not really exist
- Explain random sampling and assignment of participants into experimental and control groups
- Discuss how experimenter or participant bias could affect the results of an experiment
- Identify independent and dependent variables
Did you know that as sales in ice cream increase, so does the overall rate of crime? Is it possible that indulging in your favorite flavor of ice cream could send you on a crime spree? Or, after committing crime do you think you might decide to treat yourself to a cone? There is no question that a relationship exists between ice cream and crime (e.g., Harper, 2013), but it would be pretty foolish to decide that one thing actually caused the other to occur.
It is much more likely that both ice cream sales and crime rates are related to the temperature outside. When the temperature is warm, there are lots of people out of their houses, interacting with each other, getting annoyed with one another, and sometimes committing crimes. Also, when it is warm outside, we are more likely to seek a cool treat like ice cream. How do we determine if there is indeed a relationship between two things? And when there is a relationship, how can we discern whether it is attributable to coincidence or causation?
CORRELATIONAL RESEARCH
Correlation means that there is a relationship between two or more variables (such as ice cream consumption and crime), but this relationship does not necessarily imply cause and effect. When two variables are correlated, it simply means that as one variable changes, so does the other. We can measure correlation by calculating a statistic known as a correlation coefficient. A correlation coefficient is a number from -1 to +1 that indicates the strength and direction of the relationship between variables. The correlation coefficient is usually represented by the letter r .
The number portion of the correlation coefficient indicates the strength of the relationship. The closer the number is to 1 (be it negative or positive), the more strongly related the variables are, and the more predictable changes in one variable will be as the other variable changes. The closer the number is to zero, the weaker the relationship, and the less predictable the relationships between the variables becomes. For instance, a correlation coefficient of 0.9 indicates a far stronger relationship than a correlation coefficient of 0.3. If the variables are not related to one another at all, the correlation coefficient is 0. The example above about ice cream and crime is an example of two variables that we might expect to have no relationship to each other.
The sign—positive or negative—of the correlation coefficient indicates the direction of the relationship ( [link] ). A positive correlation means that the variables move in the same direction. Put another way, it means that as one variable increases so does the other, and conversely, when one variable decreases so does the other. A negative correlation means that the variables move in opposite directions. If two variables are negatively correlated, a decrease in one variable is associated with an increase in the other and vice versa.
The example of ice cream and crime rates is a positive correlation because both variables increase when temperatures are warmer. Other examples of positive correlations are the relationship between an individual’s height and weight or the relationship between a person’s age and number of wrinkles. One might expect a negative correlation to exist between someone’s tiredness during the day and the number of hours they slept the previous night: the amount of sleep decreases as the feelings of tiredness increase. In a real-world example of negative correlation, student researchers at the University of Minnesota found a weak negative correlation ( r = -0.29) between the average number of days per week that students got fewer than 5 hours of sleep and their GPA (Lowry, Dean, & Manders, 2010). Keep in mind that a negative correlation is not the same as no correlation. For example, we would probably find no correlation between hours of sleep and shoe size.
As mentioned earlier, correlations have predictive value. Imagine that you are on the admissions committee of a major university. You are faced with a huge number of applications, but you are able to accommodate only a small percentage of the applicant pool. How might you decide who should be admitted? You might try to correlate your current students’ college GPA with their scores on standardized tests like the SAT or ACT. By observing which correlations were strongest for your current students, you could use this information to predict relative success of those students who have applied for admission into the university.
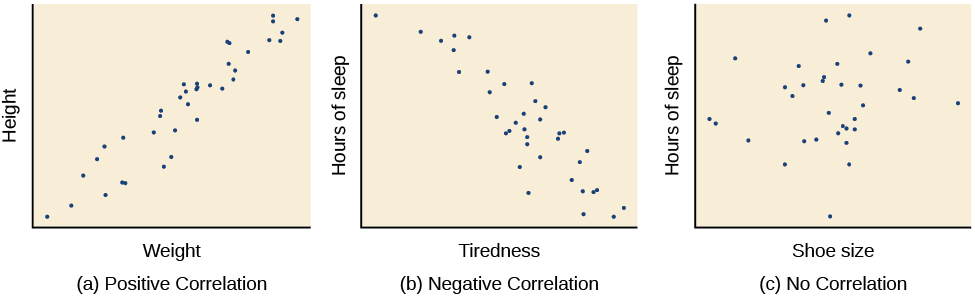
Scatterplots are a graphical view of the strength and direction of correlations. The stronger the correlation, the closer the data points are to a straight line. In these examples, we see that there is (a) a positive correlation between weight and height, (b) a negative correlation between tiredness and hours of sleep, and (c) no correlation between shoe size and hours of sleep.
Link to Learning
Manipulate this interactive scatterplot to practice your understanding of positive and negative correlation.
Correlation Does Not Indicate Causation
Correlational research is useful because it allows us to discover the strength and direction of relationships that exist between two variables. However, correlation is limited because establishing the existence of a relationship tells us little about cause and effect . While variables are sometimes correlated because one does cause the other, it could also be that some other factor, a confounding variable , is actually causing the systematic movement in our variables of interest. In the ice cream/crime rate example mentioned earlier, temperature is a confounding variable that could account for the relationship between the two variables.
Even when we cannot point to clear confounding variables, we should not assume that a correlation between two variables implies that one variable causes changes in another. This can be frustrating when a cause-and-effect relationship seems clear and intuitive. Think back to our discussion of the research done by the American Cancer Society and how their research projects were some of the first demonstrations of the link between smoking and cancer. It seems reasonable to assume that smoking causes cancer, but if we were limited to correlational research , we would be overstepping our bounds by making this assumption.
Unfortunately, people mistakenly make claims of causation as a function of correlations all the time. Such claims are especially common in advertisements and news stories. For example, recent research found that people who eat cereal on a regular basis achieve healthier weights than those who rarely eat cereal (Frantzen, Treviño, Echon, Garcia-Dominic, & DiMarco, 2013; Barton et al., 2005). Guess how the cereal companies report this finding. Does eating cereal really cause an individual to maintain a healthy weight, or are there other possible explanations, such as, someone at a healthy weight is more likely to regularly eat a healthy breakfast than someone who is obese or someone who avoids meals in an attempt to diet ( [link] )? While correlational research is invaluable in identifying relationships among variables, a major limitation is the inability to establish causality. Psychologists want to make statements about cause and effect, but the only way to do that is to conduct an experiment to answer a research question. The next section describes how scientific experiments incorporate methods that eliminate, or control for, alternative explanations, which allow researchers to explore how changes in one variable cause changes in another variable.
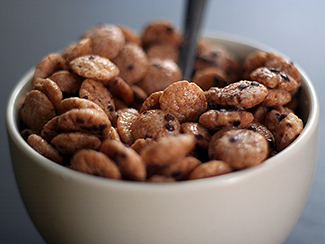
Does eating cereal really cause someone to be a healthy weight? (credit: Tim Skillern)
Illusory Correlations
The temptation to make erroneous cause-and-effect statements based on correlational research is not the only way we tend to misinterpret data. We also tend to make the mistake of illusory correlations, especially with unsystematic observations. Illusory correlations , or false correlations, occur when people believe that relationships exist between two things when no such relationship exists. One well-known illusory correlation is the supposed effect that the moon’s phases have on human behavior. Many people passionately assert that human behavior is affected by the phase of the moon, and specifically, that people act strangely when the moon is full ( [link] ).
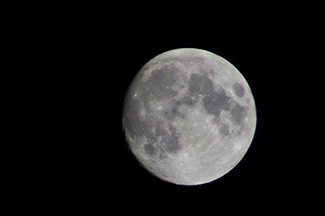
Many people believe that a full moon makes people behave oddly. (credit: Cory Zanker)
There is no denying that the moon exerts a powerful influence on our planet. The ebb and flow of the ocean’s tides are tightly tied to the gravitational forces of the moon. Many people believe, therefore, that it is logical that we are affected by the moon as well. After all, our bodies are largely made up of water. A meta-analysis of nearly 40 studies consistently demonstrated, however, that the relationship between the moon and our behavior does not exist (Rotton & Kelly, 1985). While we may pay more attention to odd behavior during the full phase of the moon, the rates of odd behavior remain constant throughout the lunar cycle.
Why are we so apt to believe in illusory correlations like this? Often we read or hear about them and simply accept the information as valid. Or, we have a hunch about how something works and then look for evidence to support that hunch, ignoring evidence that would tell us our hunch is false; this is known as confirmation bias . Other times, we find illusory correlations based on the information that comes most easily to mind, even if that information is severely limited. And while we may feel confident that we can use these relationships to better understand and predict the world around us, illusory correlations can have significant drawbacks. For example, research suggests that illusory correlations—in which certain behaviors are inaccurately attributed to certain groups—are involved in the formation of prejudicial attitudes that can ultimately lead to discriminatory behavior (Fiedler, 2004).
CAUSALITY: CONDUCTING EXPERIMENTS AND USING THE DATA
As you’ve learned, the only way to establish that there is a cause-and-effect relationship between two variables is to conduct a scientific experiment . Experiment has a different meaning in the scientific context than in everyday life. In everyday conversation, we often use it to describe trying something for the first time, such as experimenting with a new hair style or a new food. However, in the scientific context, an experiment has precise requirements for design and implementation.
The Experimental Hypothesis
In order to conduct an experiment, a researcher must have a specific hypothesis to be tested. As you’ve learned, hypotheses can be formulated either through direct observation of the real world or after careful review of previous research. For example, if you think that children should not be allowed to watch violent programming on television because doing so would cause them to behave more violently, then you have basically formulated a hypothesis—namely, that watching violent television programs causes children to behave more violently. How might you have arrived at this particular hypothesis? You may have younger relatives who watch cartoons featuring characters using martial arts to save the world from evildoers, with an impressive array of punching, kicking, and defensive postures. You notice that after watching these programs for a while, your young relatives mimic the fighting behavior of the characters portrayed in the cartoon ( [link] ).
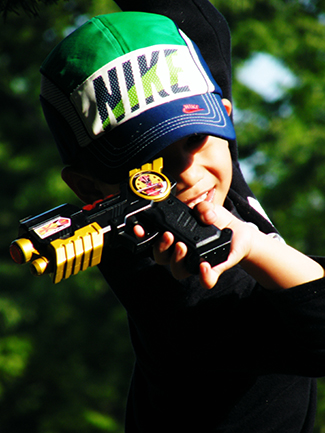
Seeing behavior like this right after a child watches violent television programming might lead you to hypothesize that viewing violent television programming leads to an increase in the display of violent behaviors. (credit: Emran Kassim)
These sorts of personal observations are what often lead us to formulate a specific hypothesis, but we cannot use limited personal observations and anecdotal evidence to rigorously test our hypothesis. Instead, to find out if real-world data supports our hypothesis, we have to conduct an experiment.
Designing an Experiment
The most basic experimental design involves two groups: the experimental group and the control group. The two groups are designed to be the same except for one difference— experimental manipulation. The experimental group gets the experimental manipulation—that is, the treatment or variable being tested (in this case, violent TV images)—and the control group does not. Since experimental manipulation is the only difference between the experimental and control groups, we can be sure that any differences between the two are due to experimental manipulation rather than chance.
In our example of how violent television programming might affect violent behavior in children, we have the experimental group view violent television programming for a specified time and then measure their violent behavior. We measure the violent behavior in our control group after they watch nonviolent television programming for the same amount of time. It is important for the control group to be treated similarly to the experimental group, with the exception that the control group does not receive the experimental manipulation. Therefore, we have the control group watch non-violent television programming for the same amount of time as the experimental group.
We also need to precisely define, or operationalize, what is considered violent and nonviolent. An operational definition is a description of how we will measure our variables, and it is important in allowing others understand exactly how and what a researcher measures in a particular experiment. In operationalizing violent behavior, we might choose to count only physical acts like kicking or punching as instances of this behavior, or we also may choose to include angry verbal exchanges. Whatever we determine, it is important that we operationalize violent behavior in such a way that anyone who hears about our study for the first time knows exactly what we mean by violence. This aids peoples’ ability to interpret our data as well as their capacity to repeat our experiment should they choose to do so.
Once we have operationalized what is considered violent television programming and what is considered violent behavior from our experiment participants, we need to establish how we will run our experiment. In this case, we might have participants watch a 30-minute television program (either violent or nonviolent, depending on their group membership) before sending them out to a playground for an hour where their behavior is observed and the number and type of violent acts is recorded.
Ideally, the people who observe and record the children’s behavior are unaware of who was assigned to the experimental or control group, in order to control for experimenter bias. Experimenter bias refers to the possibility that a researcher’s expectations might skew the results of the study. Remember, conducting an experiment requires a lot of planning, and the people involved in the research project have a vested interest in supporting their hypotheses. If the observers knew which child was in which group, it might influence how much attention they paid to each child’s behavior as well as how they interpreted that behavior. By being blind to which child is in which group, we protect against those biases. This situation is a single-blind study , meaning that one of the groups (participants) are unaware as to which group they are in (experiment or control group) while the researcher who developed the experiment knows which participants are in each group.
In a double-blind study , both the researchers and the participants are blind to group assignments. Why would a researcher want to run a study where no one knows who is in which group? Because by doing so, we can control for both experimenter and participant expectations. If you are familiar with the phrase placebo effect , you already have some idea as to why this is an important consideration. The placebo effect occurs when people’s expectations or beliefs influence or determine their experience in a given situation. In other words, simply expecting something to happen can actually make it happen.
The placebo effect is commonly described in terms of testing the effectiveness of a new medication. Imagine that you work in a pharmaceutical company, and you think you have a new drug that is effective in treating depression. To demonstrate that your medication is effective, you run an experiment with two groups: The experimental group receives the medication, and the control group does not. But you don’t want participants to know whether they received the drug or not.
Why is that? Imagine that you are a participant in this study, and you have just taken a pill that you think will improve your mood. Because you expect the pill to have an effect, you might feel better simply because you took the pill and not because of any drug actually contained in the pill—this is the placebo effect.
To make sure that any effects on mood are due to the drug and not due to expectations, the control group receives a placebo (in this case a sugar pill). Now everyone gets a pill, and once again neither the researcher nor the experimental participants know who got the drug and who got the sugar pill. Any differences in mood between the experimental and control groups can now be attributed to the drug itself rather than to experimenter bias or participant expectations ( [link] ).
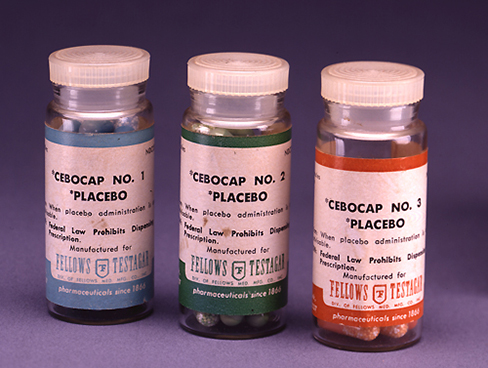
Providing the control group with a placebo treatment protects against bias caused by expectancy. (credit: Elaine and Arthur Shapiro)
Independent and Dependent Variables
In a research experiment, we strive to study whether changes in one thing cause changes in another. To achieve this, we must pay attention to two important variables, or things that can be changed, in any experimental study: the independent variable and the dependent variable. An independent variable is manipulated or controlled by the experimenter. In a well-designed experimental study, the independent variable is the only important difference between the experimental and control groups. In our example of how violent television programs affect children’s display of violent behavior, the independent variable is the type of program—violent or nonviolent—viewed by participants in the study ( [link] ). A dependent variable is what the researcher measures to see how much effect the independent variable had. In our example, the dependent variable is the number of violent acts displayed by the experimental participants.
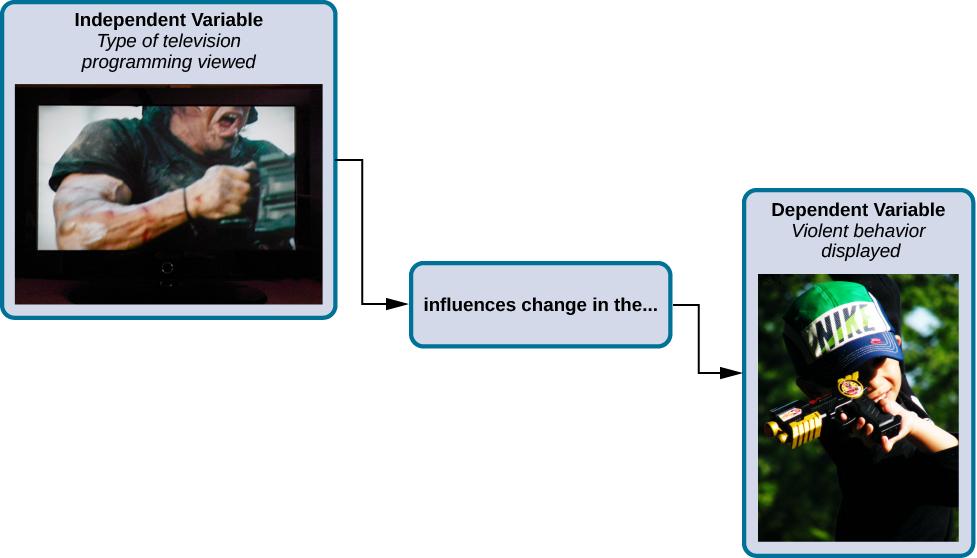
In an experiment, manipulations of the independent variable are expected to result in changes in the dependent variable. (credit “automatic weapon”: modification of work by Daniel Oines; credit “toy gun”: modification of work by Emran Kassim)
We expect that the dependent variable will change as a function of the independent variable. In other words, the dependent variable depends on the independent variable. A good way to think about the relationship between the independent and dependent variables is with this question: What effect does the independent variable have on the dependent variable? Returning to our example, what effect does watching a half hour of violent television programming or nonviolent television programming have on the number of incidents of physical aggression displayed on the playground?
Selecting and Assigning Experimental Participants
Now that our study is designed, we need to obtain a sample of individuals to include in our experiment. Our study involves human participants so we need to determine who to include. Participants are the subjects of psychological research, and as the name implies, individuals who are involved in psychological research actively participate in the process. Often, psychological research projects rely on college students to serve as participants. In fact, the vast majority of research in psychology subfields has historically involved students as research participants (Sears, 1986; Arnett, 2008). But are college students truly representative of the general population? College students tend to be younger, more educated, more liberal, and less diverse than the general population. Although using students as test subjects is an accepted practice, relying on such a limited pool of research participants can be problematic because it is difficult to generalize findings to the larger population.
Our hypothetical experiment involves children, and we must first generate a sample of child participants. Samples are used because populations are usually too large to reasonably involve every member in our particular experiment ( [link] ). If possible, we should use a random sample (there are other types of samples, but for the purposes of this chapter, we will focus on random samples). A random sample is a subset of a larger population in which every member of the population has an equal chance of being selected. Random samples are preferred because if the sample is large enough we can be reasonably sure that the participating individuals are representative of the larger population. This means that the percentages of characteristics in the sample—sex, ethnicity, socioeconomic level, and any other characteristics that might affect the results—are close to those percentages in the larger population.
In our example, let’s say we decide our population of interest is fourth graders. But all fourth graders is a very large population, so we need to be more specific; instead we might say our population of interest is all fourth graders in a particular city. We should include students from various income brackets, family situations, races, ethnicities, religions, and geographic areas of town. With this more manageable population, we can work with the local schools in selecting a random sample of around 200 fourth graders who we want to participate in our experiment.
In summary, because we cannot test all of the fourth graders in a city, we want to find a group of about 200 that reflects the composition of that city. With a representative group, we can generalize our findings to the larger population without fear of our sample being biased in some way.
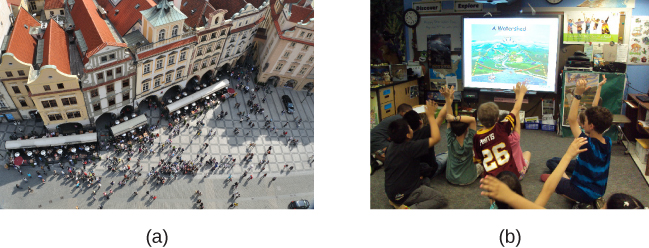
Researchers may work with (a) a large population or (b) a sample group that is a subset of the larger population. (credit “crowd”: modification of work by James Cridland; credit “students”: modification of work by Laurie Sullivan)
Now that we have a sample, the next step of the experimental process is to split the participants into experimental and control groups through random assignment. With random assignment , all participants have an equal chance of being assigned to either group. There is statistical software that will randomly assign each of the fourth graders in the sample to either the experimental or the control group.
Random assignment is critical for sound experimental design . With sufficiently large samples, random assignment makes it unlikely that there are systematic differences between the groups. So, for instance, it would be very unlikely that we would get one group composed entirely of males, a given ethnic identity, or a given religious ideology. This is important because if the groups were systematically different before the experiment began, we would not know the origin of any differences we find between the groups: Were the differences preexisting, or were they caused by manipulation of the independent variable? Random assignment allows us to assume that any differences observed between experimental and control groups result from the manipulation of the independent variable.
Use this online tool to instantly generate randomized numbers and to learn more about random sampling and assignments.
Issues to Consider
While experiments allow scientists to make cause-and-effect claims, they are not without problems. True experiments require the experimenter to manipulate an independent variable, and that can complicate many questions that psychologists might want to address. For instance, imagine that you want to know what effect sex (the independent variable) has on spatial memory (the dependent variable). Although you can certainly look for differences between males and females on a task that taps into spatial memory, you cannot directly control a person’s sex. We categorize this type of research approach as quasi-experimental and recognize that we cannot make cause-and-effect claims in these circumstances.
Experimenters are also limited by ethical constraints. For instance, you would not be able to conduct an experiment designed to determine if experiencing abuse as a child leads to lower levels of self-esteem among adults. To conduct such an experiment, you would need to randomly assign some experimental participants to a group that receives abuse, and that experiment would be unethical.
Interpreting Experimental Findings
Once data is collected from both the experimental and the control groups, a statistical analysis is conducted to find out if there are meaningful differences between the two groups. A statistical analysis determines how likely any difference found is due to chance (and thus not meaningful). In psychology, group differences are considered meaningful, or significant, if the odds that these differences occurred by chance alone are 5 percent or less. Stated another way, if we repeated this experiment 100 times, we would expect to find the same results at least 95 times out of 100.
The greatest strength of experiments is the ability to assert that any significant differences in the findings are caused by the independent variable. This occurs because random selection, random assignment, and a design that limits the effects of both experimenter bias and participant expectancy should create groups that are similar in composition and treatment. Therefore, any difference between the groups is attributable to the independent variable, and now we can finally make a causal statement. If we find that watching a violent television program results in more violent behavior than watching a nonviolent program, we can safely say that watching violent television programs causes an increase in the display of violent behavior.
Reporting Research
When psychologists complete a research project, they generally want to share their findings with other scientists. The American Psychological Association (APA) publishes a manual detailing how to write a paper for submission to scientific journals. Unlike an article that might be published in a magazine like Psychology Today, which targets a general audience with an interest in psychology, scientific journals generally publish peer-reviewed journal articles aimed at an audience of professionals and scholars who are actively involved in research themselves.
The Online Writing Lab (OWL) at Purdue University can walk you through the APA writing guidelines.
A peer-reviewed journal article is read by several other scientists (generally anonymously) with expertise in the subject matter. These peer reviewers provide feedback—to both the author and the journal editor—regarding the quality of the draft. Peer reviewers look for a strong rationale for the research being described, a clear description of how the research was conducted, and evidence that the research was conducted in an ethical manner. They also look for flaws in the study’s design, methods, and statistical analyses. They check that the conclusions drawn by the authors seem reasonable given the observations made during the research. Peer reviewers also comment on how valuable the research is in advancing the discipline’s knowledge. This helps prevent unnecessary duplication of research findings in the scientific literature and, to some extent, ensures that each research article provides new information. Ultimately, the journal editor will compile all of the peer reviewer feedback and determine whether the article will be published in its current state (a rare occurrence), published with revisions, or not accepted for publication.
Peer review provides some degree of quality control for psychological research. Poorly conceived or executed studies can be weeded out, and even well-designed research can be improved by the revisions suggested. Peer review also ensures that the research is described clearly enough to allow other scientists to replicate it, meaning they can repeat the experiment using different samples to determine reliability. Sometimes replications involve additional measures that expand on the original finding. In any case, each replication serves to provide more evidence to support the original research findings. Successful replications of published research make scientists more apt to adopt those findings, while repeated failures tend to cast doubt on the legitimacy of the original article and lead scientists to look elsewhere. For example, it would be a major advancement in the medical field if a published study indicated that taking a new drug helped individuals achieve a healthy weight without changing their diet. But if other scientists could not replicate the results, the original study’s claims would be questioned.
Dig Deeper: The Vaccine-Autism Myth and the Retraction of Published Studies
Some scientists have claimed that routine childhood vaccines cause some children to develop autism, and, in fact, several peer-reviewed publications published research making these claims. Since the initial reports, large-scale epidemiological research has suggested that vaccinations are not responsible for causing autism and that it is much safer to have your child vaccinated than not. Furthermore, several of the original studies making this claim have since been retracted.
A published piece of work can be rescinded when data is called into question because of falsification, fabrication, or serious research design problems. Once rescinded, the scientific community is informed that there are serious problems with the original publication. Retractions can be initiated by the researcher who led the study, by research collaborators, by the institution that employed the researcher, or by the editorial board of the journal in which the article was originally published. In the vaccine-autism case, the retraction was made because of a significant conflict of interest in which the leading researcher had a financial interest in establishing a link between childhood vaccines and autism (Offit, 2008). Unfortunately, the initial studies received so much media attention that many parents around the world became hesitant to have their children vaccinated ( [link] ). For more information about how the vaccine/autism story unfolded, as well as the repercussions of this story, take a look at Paul Offit’s book, Autism’s False Prophets: Bad Science, Risky Medicine, and the Search for a Cure.
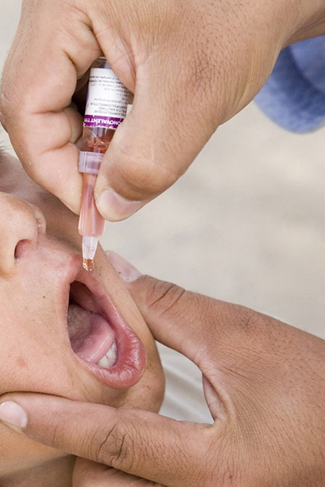
Some people still think vaccinations cause autism. (credit: modification of work by UNICEF Sverige)
RELIABILITY AND VALIDITY
Reliability and validity are two important considerations that must be made with any type of data collection. Reliability refers to the ability to consistently produce a given result. In the context of psychological research, this would mean that any instruments or tools used to collect data do so in consistent, reproducible ways.
Unfortunately, being consistent in measurement does not necessarily mean that you have measured something correctly. To illustrate this concept, consider a kitchen scale that would be used to measure the weight of cereal that you eat in the morning. If the scale is not properly calibrated, it may consistently under- or overestimate the amount of cereal that’s being measured. While the scale is highly reliable in producing consistent results (e.g., the same amount of cereal poured onto the scale produces the same reading each time), those results are incorrect. This is where validity comes into play. Validity refers to the extent to which a given instrument or tool accurately measures what it’s supposed to measure. While any valid measure is by necessity reliable, the reverse is not necessarily true. Researchers strive to use instruments that are both highly reliable and valid.
Standardized tests like the SAT are supposed to measure an individual’s aptitude for a college education, but how reliable and valid are such tests? Research conducted by the College Board suggests that scores on the SAT have high predictive validity for first-year college students’ GPA (Kobrin, Patterson, Shaw, Mattern, & Barbuti, 2008). In this context, predictive validity refers to the test’s ability to effectively predict the GPA of college freshmen. Given that many institutions of higher education require the SAT for admission, this high degree of predictive validity might be comforting.
However, the emphasis placed on SAT scores in college admissions has generated some controversy on a number of fronts. For one, some researchers assert that the SAT is a biased test that places minority students at a disadvantage and unfairly reduces the likelihood of being admitted into a college (Santelices & Wilson, 2010). Additionally, some research has suggested that the predictive validity of the SAT is grossly exaggerated in how well it is able to predict the GPA of first-year college students. In fact, it has been suggested that the SAT’s predictive validity may be overestimated by as much as 150% (Rothstein, 2004). Many institutions of higher education are beginning to consider de-emphasizing the significance of SAT scores in making admission decisions (Rimer, 2008).
In 2014, College Board president David Coleman expressed his awareness of these problems, recognizing that college success is more accurately predicted by high school grades than by SAT scores. To address these concerns, he has called for significant changes to the SAT exam (Lewin, 2014).
A correlation is described with a correlation coefficient, r , which ranges from -1 to 1. The correlation coefficient tells us about the nature (positive or negative) and the strength of the relationship between two or more variables. Correlations do not tell us anything about causation—regardless of how strong the relationship is between variables. In fact, the only way to demonstrate causation is by conducting an experiment. People often make the mistake of claiming that correlations exist when they really do not.
Researchers can test cause-and-effect hypotheses by conducting experiments. Ideally, experimental participants are randomly selected from the population of interest. Then, the participants are randomly assigned to their respective groups. Sometimes, the researcher and the participants are blind to group membership to prevent their expectations from influencing the results.
In ideal experimental design, the only difference between the experimental and control groups is whether participants are exposed to the experimental manipulation. Each group goes through all phases of the experiment, but each group will experience a different level of the independent variable: the experimental group is exposed to the experimental manipulation, and the control group is not exposed to the experimental manipulation. The researcher then measures the changes that are produced in the dependent variable in each group. Once data is collected from both groups, it is analyzed statistically to determine if there are meaningful differences between the groups.
Psychologists report their research findings in peer-reviewed journal articles. Research published in this format is checked by several other psychologists who serve as a filter separating ideas that are supported by evidence from ideas that are not. Replication has an important role in ensuring the legitimacy of published research. In the long run, only those findings that are capable of being replicated consistently will achieve consensus in the scientific community.
Self Check Questions
Critical thinking questions.
1. Earlier in this section, we read about research suggesting that there is a correlation between eating cereal and weight. Cereal companies that present this information in their advertisements could lead someone to believe that eating more cereal causes healthy weight. Why would they make such a claim and what arguments could you make to counter this cause-and-effect claim?
2. Recently a study was published in the journal, Nutrition and Cancer , which established a negative correlation between coffee consumption and breast cancer. Specifically, it was found that women consuming more than 5 cups of coffee a day were less likely to develop breast cancer than women who never consumed coffee (Lowcock, Cotterchio, Anderson, Boucher, & El-Sohemy, 2013). Imagine you see a newspaper story about this research that says, “Coffee Protects Against Cancer.” Why is this headline misleading and why would a more accurate headline draw less interest?
3. Sometimes, true random sampling can be very difficult to obtain. Many researchers make use of convenience samples as an alternative. For example, one popular convenience sample would involve students enrolled in Introduction to Psychology courses. What are the implications of using this sampling technique?
4. Peer review is an important part of publishing research findings in many scientific disciplines. This process is normally conducted anonymously; in other words, the author of the article being reviewed does not know who is reviewing the article, and the reviewers are unaware of the author’s identity. Why would this be an important part of this process?
Personal Application Questions
5. We all have a tendency to make illusory correlations from time to time. Try to think of an illusory correlation that is held by you, a family member, or a close friend. How do you think this illusory correlation came about and what can be done in the future to combat them?
6. Are there any questions about human or animal behavior that you would really like to answer? Generate a hypothesis and briefly describe how you would conduct an experiment to answer your question.
1. The cereal companies are trying to make a profit, so framing the research findings in this way would improve their bottom line. However, it could be that people who forgo more fatty options for breakfast are health conscious and engage in a variety of other behaviors that help them maintain a healthy weight.
2. Using the word protects seems to suggest causation as a function of correlation. If the headline were more accurate, it would be less interesting because indicating that two things are associated is less powerful than indicating that doing one thing causes a change in the other.
3. If research is limited to students enrolled in Introduction to Psychology courses, then our ability to generalize to the larger population would be dramatically reduced. One could also argue that students enrolled in Introduction to Psychology courses may not be representative of the larger population of college students at their school, much less the larger general population.
4. Anonymity protects against personal biases interfering with the reviewer’s opinion of the research. Allowing the reviewer to remain anonymous would mean that they can be honest in their appraisal of the manuscript without fear of reprisal.
- Psychology. Authored by : OpenStax College. Located at : http://cnx.org/contents/[email protected]:1/Psychology . License : CC BY: Attribution . License Terms : Download for free at http://cnx.org/content/col11629/latest/.
- Tools and Resources
- Customer Services
- Affective Science
- Biological Foundations of Psychology
- Clinical Psychology: Disorders and Therapies
- Cognitive Psychology/Neuroscience
- Developmental Psychology
- Educational/School Psychology
- Forensic Psychology
- Health Psychology
- History and Systems of Psychology
- Individual Differences
- Methods and Approaches in Psychology
- Neuropsychology
- Organizational and Institutional Psychology
- Personality
- Psychology and Other Disciplines
- Social Psychology
- Sports Psychology
- Back to results
- Share This Facebook LinkedIn Twitter
Article contents
The concept of crisis in the history of western psychology.
- Martin Wieser Martin Wieser Sigmund Freud Privat Universität Berlin, Department of Psychology
- https://doi.org/10.1093/acrefore/9780190236557.013.470
- Published online: 28 February 2020
With roots that range from medicine to politics, to jurisdiction and historiography in ancient Greece, the concept of “crisis” played an eminent role in the founding years of Western academic psychology and continued to be relevant during its development in the 19th and 20th century. “Crisis” conveys the idea of an imminent danger of disintegration and breakdown, as well as a pivotal turning point with the chance of a new beginning. To this day, both levels of meaning are present in psychological discourses. Early diagnoses of a state of “crisis” of psychology date back to the end of the 19th century and focused on the question of the correct metaphysical foundation of psychology. During the interwar period, warnings of a disintegration of the discipline reached their first climax in German academia, when many eminent psychologists expressed their worries about the increasing fragmentation of the discipline. The rise of totalitarian systems in the 1930s brought an end to these debates, silencing the theoretical polyphony with physical violence. The 1960s saw a resurgence of “crisis literature” and the emergence of a more positive connotation of the concept in U.S.-American experimental psychology, when it was connected with Thomas Kuhn’s ideas of scientific “revolutions” and “paradigm shifts.” Since that time, psychological crisis literature has revolved around the question of unity, disunity, and the scientific status of the discipline. Although psychological crisis literature showed little success in solving the fundamental problems it addressed, it still provides one of the most theoretically rich and thought-provoking bodies of knowledge for theoretical and historical analyses of the discipline.
- history of psychology
- history of science
Introduction
The etymological root of the concept of crisis in ancient Greek, κρίσις (“decision or choice,” from κρινω : “part, separate, disconnect”) referred to a short (“critical”) period of time when an ultimate decision had to be made that required a choice between irreversible consequences, or a climax which could either result in the total destruction or a new beginning. In Hippocratic medicine, κρίσις described the turning point of a disease, from which either recovery or death would follow. In military contexts, the concept referred to the tipping point of a battle. Aristotle used the term to describe political judgments concerning important public affairs (Koselleck, 1995 ). This meaning of “crisis” as a moment that determines all subsequent events is still prevalent in modern academic psychology, for example, in the works of Erik Erikson ( 1959 ), who described the development of the human personality as a series of eight “psychosocial crises” that every individual is supposed to master during the course of life. Since the 1970s, the popular concept of “midlife crisis” is used somewhat similarly (Schmidt, 2017 ) in the sense that it refers to nothing intrinsically extraordinary or pathological, but a necessary (or at least common) stage of development that involves a chance for further growth, but also a risk of mental deterioration and social relegation. In clinical-psychological contexts (e.g., in psychological crisis intervention), the concept of crisis usually has a more negative connotation, as it is traditionally used in this context to describe extraordinary and often life-threatening experiences that can pose a serious threat for mental health and cause various symptoms of traumatization (e.g., mood and sleep disorders, flashbacks, etc.).
Within the broad spectrum of all different (and sometimes contradictory) meanings of “crisis,” this work focuses on the genesis and development of this concept as it was used and discussed in Western academic psychology. A state of “crisis” of the discipline was called out almost on a regular basis through the history of psychology (beginning even before psychology was established as an independent academic discipline), but the denotation and connotation of what this “crisis” means has shifted repeatedly. It is not in the scope of this article to cover all variants of psychological crisis literature that came up in all the different psychological schools, currents, or subdisciplines. Instead, it provides the reader with a broad overview and critical understanding of the historical development and rhetorical function of psychological crisis literature as far as it was concerned with the state of psychology as a whole.
Early Diagnoses of a “Crisis” in Academic Psychology
One of the first academics who saw the young and rapidly growing field of experimental psychology in a state of crisis was the Swiss philosopher Rudolf Willy, a student and follower of Richard Avenarius. Almost 20 years had gone by since Wilhelm Wundt established the first institute of experimental psychology in Leipzig, yet Willy saw psychology still “lying deeply in the fetters of speculation even today” (Willy, 1897 , p. 79). For Willy, the main obstacle of contemporary psychology was the fact that psychological research was still laden with metaphysical presuppositions and ideas. Because “experience and metaphysics . . . do not just exclude, but even negate each other,” as Willy argued, the prevalence of metaphysics in psychological research would inflict “infinite damage” (Willy, 1897 , p. 80) to psychology. Willy did not just address Wilhelm Wundt, but also attacked other influential psychologists such as Carl Stumpf, Johannes Rehmke, Theodor Lipps, and Franz Brentano. Two years later, Willy published the first book about the “crisis in psychology” (Willy, 1899 ), wherein he proposed the solution of the “metaphysical-methodological crisis” of the discipline by turning toward the empiriocriticism of Ernst Mach and Richard Avenarius, a position that, according to Willy, would enable psychologists to overcome the pitfalls of dualism and materialism by only accepting “pure experience” as psychology’s foundation (cf. Mülberger, 2012 ).
As was mentioned in the introduction, the Greek word Κρίσις referred to an objective process heading toward a deciding turning point as well as a subjective judgment or decision (as it is performed by a judge, a politician, or a military leader). Although the latter has been commonly described as a “critique” since the 17th century (Röttgers, 1995 ), a close relationship between both concepts still lingers on. In the preface of his eminent work “Critique of Pure Experience,” Willy’s teacher Avenarius declared that it was Kant’s understanding of critique that led him toward the recognition of a crisis in philosophy: “It was critique that became crisis for me.” However, Avenarius’s “crisis” conveyed a much more positive outlook than that of his student: “Perhaps it also helps somebody else to a pleasant [wohltätig] crisis, or helps him out of one that is unpleasant” (Avenarius, 1888 , p. XIII). In the case of Avenarius, it was critique that led him to a crisis, whereas Willy’s critique was supposed to reveal and heal a crisis that already existed before. The “critical” revelation was supposed to lead the way out of the crisis: “By acknowledging the crisis, the crisis will disappear” (Willy, 1899 , p. 5).
Wilhelm Wundt, however, played the ball right back to Willy and Avenarius, as he accused them of following a “peculiar metaphysical method” of their own, a method that, in Wundt’s perspective, is blinding out its own premises. He described empiriocriticism as “primarily a metaphysical system in which criticism plays a rather small role” (Wundt, 1898 , p. 2). Meanwhile, the philosopher and theologian Constantin Gutberlet expressed his amusement as he observed “the truly delightful spectacle when various representatives of empiricism all accuse each other of metaphysics.” Despite the sarcastic remarks, Gutberlet bemoaned the fact that “philosophers use metaphysics, the most sublime, most necessary and most certain of all sciences, as an insult with which one believes to be able to attach a flaw to one’s opponent” (Gutberlet, 1898 , p. 121). In this way, the first debate on psychology’s crisis shifted from the question of whether or not there is metaphysics within psychology toward the problem of which kind of metaphysics should be acceptable, a question that—unsurprisingly—did not reach any consent.
Willy’s first diagnosis of a metaphysical and methodological crisis of academic psychology fell into an era of increasing disassociation between philosophy and psychology, a separation that was not always carried out in mutual consent (cf. Kusch, 1995 ). Although Wilhelm Wundt vehemently argued that psychology was better off under the umbrella of philosophy (Wundt, 1913 ), Neo-Kantian philosophers such as Wilhelm Windelband publicly polemicized against experimental psychologists who would “keep their distance from the big problems of life, the political, religious and social questions,” while focusing on small-scale methodological problems to “prove that some people need more time to remember something than others” (Windelband, 1909 , pp. 92–93). This dispute between psychologists and philosophers culminated in the publication of a manifesto in the famous philosophical journal Kant-Studien in 1913 . More than one hundred eminent philosophers from Germany, Austria, and Switzerland demanded the establishment of distinct chairs and faculties for empirically oriented psychologists to prevent them from taking away those from “real” philosophers (“Eine Kundgebung,” 1913 ). Although many, if not most, psychologists certainly would have also welcomed the creation of independent psychological chairs and institutes, it did take another 30 years until academic psychology gained independence on the level of the university: In 1941 , in the midst of World War II, a National Study Program for Psychologists was established for the first time in German history (Geuter, 1988 ). Between that time and Willy’s first declaration of psychology’s crisis, the institutional status of the discipline was far from secure, and the recurring emergence of psychological crisis literature can be interpreted as a clear sign of this disciplinary insecurity.
In the meantime, psychological crisis literature began to emerge in France (Binet, 1911 ; Chazottes, 1902 ; Kostyleff, 1911 ; Rageot, 1908 ) and Italy (De Sanctis, 1912 ; De Sarlo, 1914 ). Kostyleff’s book La crise de la psychologie expérimentale treated the topic most extensively. He was less worried about metaphysical problems than the fact that experimental psychology began to branch out into an increasing number of rivaling schools. The solution, in Kostyleff’s view, was to be found in the Russian “objective psychology” of Bechterev, a branch of experimental psychology that heavily drew on the reflex as the basic unit of psychological investigation. Neither Kostyleff’s suggestion nor the other contributions provoked much of a debate, as the diversity of psychological schools was rather welcomed than worried about in most parts of French and Italian academia (cf. Carson, 2012 ; Proietto & Lombardo, 2015 ). Yet, Kostyleff’s book was the first one that explicitly identified the undirected growth of the discipline as the most urgent problem of modern psychology.
The Rise of “Crisis Literature” in the Interwar Era
After the loss of World War I, a new type of crisis literature became particularly popular in interwar Germany. As the academic elite of the once so prestigious German universities feared to lose their public reputation and moral high ground between the rising working class and influential corporate leaders (cf. Ringer, 1969 ), the cause for moral “degradation” was found in modern-day technology and their spiritual equivalents, mechanicism and materialism. In 1913 , the industrialist, politician, and later foreign minister Walther Rathenau published Zur Kritik der Zeit [On the critique of time] (Rathenau, 1913 ), and five years later Zur Mechanik des Geistes [On the mechanics of the mind] (Rathenau, 1918 ). Both works presented a radical critique of the disintegration of modern capitalist mass societies. Although Rathenau still saw a possible escape from moral deterioration and materialism in the neo-romantic utopia of an “empire of the soul,” other authors showed a much more pessimistic attitude in regards to the future of the industrialized Western societies: Oswald Spengler’s Der Untergang des Abendlandes [The decline of the West] (Spengler, 1918 ) became immensely popular after World War I, and Max Weber’s famous lecture “Wissenschaft als Beruf” [Science as a Profession] from 1917 (Weber, 1922 ), wherein Weber saw the potential of science to provide any moral guidelines for living, were widely discussed in Germany. Edmund Husserl’s unfinished last work from 1936 , “The crisis of European Sciences and Transcendental Phenomenology” (Husserl, 1970 ) also lamented that philosophy had been replaced by specialized small-scale disciplines and the philosophy of positivism, which resulted in a disconnection between science and the pre-theoretical, subjective “lifeworld” of everyday life. Popular writers such as Ludwig Klages ( 1929 ) criticized the “disintegration” of the personality and the loss of traditional values in modernity. This critique was based on his contrasting of the primal “soul” and “character” on the one side and the modern “mind” or “intellect” on the other, a position which fervently condemned cold-blooded scientific rationalization and mechanization of all areas of modern life. Psychology and psychological concepts played a central role in this collective “search for wholeness” (Harrington, 1996 ), although it often remained unclear how it was supposed to do so.
While many cultural critics turned to psychology in search of a resort from the dark sides of modernity, psychology struggled more and more to find itself. Even before the turn of the century, Wilhelm Dilthey ( 1894 ) had attacked experimental psychology as a misguided endeavor that “disintegrated” the “structured wholeness” of the person. Herrmann Ebbinghaus ( 1896 ) countered Dilthey’s attack and defended the natural-scientific, “explanatory” approach within psychology, as he saw no need for Dilthey’s proposal of a second, “descriptive” psychology based on the arts and humanities. Thirty years later, the philosopher and pedagogue Eduard Spranger repeated Dilthey’s argument and claimed that “psychology has again entered a stage of the most severe upheavals of its foundations; indeed, it almost seems as if a division into two psychologies should result from it” (Spranger, 1974 , p. 1). When psychoanalysis entered the arena and new psychological currents or schools emerged in North America (functionalism and behaviorism), Germany (Gestalt psychology in Frankfurt and Berlin, holistic psychology in Leipzig, “thought psychology” in Würzburg), and the Soviet Union (reactology, reflexology, and cultural-historical psychology), a common and integrating conceptual and methodological foundation of psychology seemed to be further away than ever before.
It was against this historical background of a rising distrust against modernity, as well as the proliferation of different psychological schools, theories, and methods, that Karl Bühler published his eminent paper “The Crisis of Psychology” (Bühler, 1926 ), which was extended into a book one year later (Bühler, 1927 ). Bühler’s book starts out with the famous words: “Never before have there been so many psychologies, so many approaches on their own, together at the same time. One is sometimes reminded of the story of the tower of Babel” (Bühler, 1927 , p. 1). In contrast to Willy, Bühler did not find the root of psychology’s crisis in misguided metaphysical presuppositions, but in the multitude (and contradiction) of psychological theories and methods. However, psychology’s crisis, in Bühler’s perspective, was not necessarily a sign of its demise:
A quickly acquired and still unresolved wealth of new ideas, new approaches and research opportunities have provoked the critical [krisenartig] state of psychology. It is . . . not a crisis of decay, but of construction, an embarras de richesse . (Bühler, 1927 , p. 1)
After the downfall of association psychology at the end of the 19th century , Bühler argued, psychology lost its common foundation while it was also growing rapidly. Bühler’s answer to the “unresolved wealth of new ideas” was his theory of the “three aspects of one object.” In Bühler’s framework, “experience” (which is investigated via experimentally controlled introspection), “conduct” (as it is observed by the behaviorist) and the “objective mind” (the material results of collective interaction that are reconstructed by interpretative psychology) are interpreted as three “aspects” of one object, that is, the human psyche. According to Bühler, the three “perspectives” (experiential, behavioral, and interpretational) should not be understood as approaches that exclude one another, but rather as complementary: “Experience, behaviour and cultural products are largely independent variables, and yet somehow they belong together and constitute a higher unity” (Bühler, 1927 , p. 64). In Bühler’s view, psychology’s crisis should be interpreted as an inducement to find a common language for these three perspectives. However, as open and conciliatory as Bühler’s attempt to integrate different perspectives might appear, it did not accept all psychological currents alike, as he rejected and excluded the psychoanalytic perspective in the same book.
Reactions to Bühler’s analyses differed considerably. Wilhelm Wirth, a former assistant of Wundt in Leipzig, contested Bühler’s diagnosis of a crisis and emphasized the “steadiness of the development of our science” (Wirth, 1926 , p. 110). The pedagogue and psychoanalyst Siegfried Bernfeld agreed with Bühler’s diagnosis but located psychoanalysis in the center of—and not apart from—competing psychological schools and currents: “They all have to distance themselves from psychoanalysis . . . but the truth . . . is that psychology is unthinkable without Freud” (Bernfeld, 1931 , p. 177). Franz Scola from Prague praised Bühler’s scholarly and thoughtful analysis of contemporary psychology but refuted the idea that psychology should integrate different “aspects,” as he considered only one psychological perspective as necessary. Psychology, as Scola argued “is constituted solely by the aspect of internal perception; and in view of the object ‘psyche’ appearing under this aspect, the question of a unity of our science behind the three ‘aspects’ is unnecessary” (Scola, 1931 , p. 174).
Published in the same year as Bühler’s monograph, Hans Driesch’s book Basic Problems of Psychology: Its Crisis in the Present started out with the assertion that “no science is as ‘problematic’ today as psychology” (Driesch, 1926 , p. 1). The most important unresolved “basic problems” were, in Driesch’s view, the problem of the relationship between body and mind, the problem of the unconscious, the question of parapsychology, and the problem of general laws of the mind. Driesch strongly advocated for the integration of vitalism and “holistic biology” to resolve these “basic problems.” In his view, these problems were caused by the prevalence of mechanistic presuppositions in academic psychology. Mechanicism—a legacy of association psychology, as Driesch emphasized—could not explain the existence of activity, structure, sense, and meaning in the human psyche. Although the eminent Gestalt psychologists Kurt Koffka agreed with Driesch that there is a crisis in psychology, and shared his critique of mechanicism and association psychology, he strongly rejected Driesch’s proposed neo-vitalist solution. Koffka emphasized that “Driesch does not speak for psychology. Psychology’s crisis . . . can only be overcome through research, like the natural scientist does it” (Koffka, 1926 , p. 586). Koffka’s concept of “natural science,” however, differed from a traditional view insofar as it was supposed to include “wholeness” and “structure” in its vocabulary (cf. Ash, 1995 ).
In the meantime, unbeknownst to and independently from Bühler and Driesch, a young Russian psychologist had formulated his own perspective on the problematic state of psychology: Lev Vygotsky’s essay “The Historical meaning of the Crisis of Psychology” (Vygotsky, 1997 ) was also written in 1927 . To surmount the fragmented state of the discipline and the confusing variety of its methodologies, Vygotsky argued, psychology needed to leave “idealist” philosophy behind and rest its foundations on dialectics and historical materialism. Just as Bühler, he interpreted the crisis as a chance for the discipline to mature and grow. In contrast to Bühler, however, he rejected the idea of synthesizing existing approaches and schools, as he emphasized their incompatible conceptual and epistemological foundations. In Vygotsky’s view, only a completely new, systematic, materialist, and practically oriented foundation could help psychology become a proper science. Although Vygotsky’s essay remained unfinished and was first translated to English only 70 years after it was written (Vygotsky, 1997 ), it still stands as a key text on the theoretical foundation of cultural-historical psychology (Slunecko & Wieser, 2014 ) and its critique of Western academic psychology (Hyman, 2012 ).
It seems remarkable that so many authors agreed that psychology was in a problematic state during the interwar era, yet absolutely no consent could be reached as to what caused the crisis or how it could be solved. One outstanding aspect of the crisis literature of the interwar era was that all authors used the diagnosis of a crisis to push their own agenda on how it could be overcome, while accusing competing schools or currents as incapable of identifying the “real” causes or the proper solution of the crisis. Ten years after Bühler published his book on the topic, his assessment was much more pessimistic than before:
If you look at contemporary theoretical psychology, you get a picture like I drew it 10 years ago in the “crisis.” Not one, but many psychologies stand side by side and against each other. And whoever wants to unite them grasps with horror that it is not possible, because there is a discrepancy between concepts . . . (Bühler, 1969 , p. 180)
The first heyday of psychological “crisis literature” did not put forth any consent on how the crisis could be overcome, but it certainly did motivate and bring together a large group of psychologists and philosophers from different schools and currents to critically reflect on the methodological and epistemological presuppositions of their discipline. Even representatives from early applied psychology (“psychotechnics,” as it was called then) joined the discussion and reflected on the consequences of psychology’s crisis for the profession (Juhàsz, 1929 ).
Finally, not arguments, but violence brought an end to the first phase of crisis literature in Europe: After the rise of National Socialism, about a third of psychologists were killed, detained, or expelled from German and Austrian universities, and those who stayed either adapted their theories and practices to the new political and ideological agenda (Ash, 2002 ) or remained silent. After racist and political persecution and ideological Gleichschaltung eradicated most of the blooming intellectual landscape, the Austrian psychologist Peter Hofstätter added the last comment to the first chapter of psychology’s “crisis.” While the war raged over the continent, Hofstätter saw psychology’s only chance for survival in the adaption to the new “spiritual attitude [Seelenhaltung] of the nation” and the readjustment toward the “primate of the practice” (Hofstätter, 1941 , p. 573; cf. Gundlach, 2012 ). Although debates on psychology’s foundations were silenced and theoretical work was mostly confined to racial psychology, characterology, and holistic psychology [ Ganzheitspsychologie ], applied psychology flourished. In the Wehrmacht, psychologists offered their services to select high-ranked officers and specialists (Geuter, 1988 ). In the war industry, industrial psychologists helped to select forced laborers, women, and prisoners; and in clinical contexts, the first “counselling psychologists” were trained during the war (Cocks, 1997 ). In the face of omnipresent violence, as it seems, the question of how to deal with the plurality and contradictoriness of psychological knowledge had solved itself.
“Crises” and “Revolutions” in the 1960s and 1970s
After the end of World War II, it did not take long until a new generation of psychologists saw the need for a structural renewal, if not a revolution, of their discipline. The starting point for the next wave of psychological crisis literature was in North America, where critical evaluations on the methodological, theoretical, and (lack of) practical dimensions of psychology had been debated since the founding years of the “New Psychology” at the end of the 19th century . As early as 1892 , William James had expressed his doubt that “psychology as it stands to-day, is a natural science, or in an exact way a science at all.” Instead, he “wished, by treating Psychology like a natural science, to help her to become one” (James, 1892 , p. 146). However, in North America, the concept of crisis did not play such an important role in the debates between structuralism, functionalism and behaviorism as it did in Europa at the same time. Even John Watson’s “behaviorist manifesto,” which presented one of the fiercest attacks on the goals and methods of experimental psychology ever to be published (Watson, 1913 ), saw many shortcomings of the discipline—but not a crisis. During the “Golden Age” of behaviorism and operationalism in U.S.-American psychology, which lasted approximately from the 1920s to the 1950s, most experimental psychologists found their day-to-day task in the observation and control of animal behavior, and little concerns about the foundations of the discipline were raised (although behaviorism was not as homogenous as it is often presented in retrospective, cf. Leahey, 2001 ).
The 1960s saw a rapid change of the intellectual and psychological landscape, a turnover which was mirrored by a new wave of crisis literature in psychology. Several reasons for this resurgence can be named: First off, the 1960s saw a rise of diverse protest movements in North America, such as the anti-Vietnam War movement, the civil rights movement, second-wave feminism, the antipsychiatry movement, hippie culture, and many other variants of counterculture. As divergent as these groups and their goals might have been, there was one thing they had in common: they were strongly opposed to the social norm of “adjustment” as the ideal for mental health and social well-being. Many of these movements, such as the movement for “human potential” (which was centered at the Esalen-Institute in California) showed a strong interest in psychological topics and practices. However, the dominating branches of experimental psychology (classical and neo-behaviorism as proposed by Clark Hull and B. F. Skinner) and psychoanalysis (dominated by ego psychology, which focused on defense mechanisms and the strengthening of the ego against the id) had little to offer for these movements. Well-established psychologists and psychotherapists who worked for the government and big corporations were identified as a part of the oppressive establishment. As “architects of adjustment” (Napoli, 1981 ) who worked in powerful institutions such as psychiatric wards, prisons, or schools, they were seen as psychological assistants of an oppressive system. Calls for a “revolution” of societal order and thinking were taken up by psychology students who were dissatisfied with the ideals of control and adjustment. They searched for a different kind of “revolutionary” psychological knowledge, a knowledge that was not designed around the problems of social control of adjustment.
Secondly, besides cultural uprisings, technological progress at the dawn of the cold war made behaviorism and its “slot-machine” model of the human organism look helplessly outdated. Concepts of cybernetics (Wiener, 1948 ) and information theory (Shannon, 1948 ) such as “input,” “output,” “feedback,” “storage,” and “filter” became immensely popular in the natural and social sciences. The interdisciplinary “Macy Conferences,” which were held in New York from 1946 to 1953 , played an important part in this context, as they brought together proponents from many different disciplines, with the aim of formulating a universal science of the structure and functions of the human mind (Bowker, 1993 ). As cybernetics used the same language to analyze and describe technological, biological, and social “feedback systems,” it transgressed traditional disciplinary boundaries and fueled the hope for an objective and computable language that described mental processes. Calls for a cognitive “revolution” within psychology would not have been possible without the rise of cybernetics, information theory, and computing machines, and the hopes that were connected with these technological innovations in the 1960s.
Thirdly, the new outlook of psychology’s crisis was strongly influenced by the physicist and historian Thomas Kuhn, whose study The Structure of Scientific Revolutions was widely discussed immediately after its publication in 1962 . Based on his historical analysis of the development of physics, chemistry, astronomy, and biology, Kuhn interpreted scientific progress as a process that passes through different stages: In a pre-paradigmatic state, different currents and schools of thought are competing with each other, aiming to expand their scope while trying to usurp their competitors. After one school manages to dominate the field and reach a “paradigmatic” state, the stage of “normal science” commences. In this stage, most proponents of a discipline follow (and teach their students) a coherent set of concepts and methods while working on commonly recognized problems (or “puzzles,” as Kuhn calls them) that have not been solved yet. At a certain stage, however, unexpected results or “anomalies” are discovered through this graduate process of “puzzle solving.” If the anomalies prove to be reproducible and incompatible with the existing paradigm, alternative explanations are sought. As the search for a solution to the persisting unsolved “puzzles” advances, some scientists come up with radically new solutions and completely different perspectives. These new solutions often put doubt on the first principles, the established methods, or even the definition of relevant “problems” of the existing paradigm. In this way, the discovery of anomalies can lead to a struggle between different explanatory frameworks—the discipline now has entered the short, but ground-breaking stage of “crisis.” As the dominance of the old paradigm is broken, the “crisis loosens the rules of normal puzzle-solving in ways that ultimately permit a new paradigm to emerge” (Kuhn, 1996 , p. 80). Ultimately, the crisis leads to a scientific “revolution,” Kuhn argues, a radical change in the scientific worldview. This process is also described in terms of a “Gestalt switch” by Kuhn: “At times of revolution, when the normal-scientific tradition changes, the scientist’s perception of his environment must be re-educated—in some familiar situations he must learn to see a new gestalt” (Kuhn, 1996 , p. 112). In contrast to Karl Popper’s theory of falsification, Kuhn proposed a model of scientific progress that does not gradually accumulate knowledge over time by testing one hypothesis after the other, but repeatedly shifts through phases of “normal science,” “crises,” and “revolutions.” Following Kuhn, an accumulation of knowledge in a traditional sense is only possible within a paradigm. As soon as this paradigm is left behind, however, all the “puzzles” that were once regarded as “solved” might need to be looked at again. The “incommensurability” of different paradigms prevents a simple transfer or accumulation of knowledge between different paradigms.
Kuhn’s notions of “crises” and scientific “revolutions” not only resonated with the broader cultural climate of the 1960s in the United States but also coincided with the rise of a new generation of American and British psychologists (such as Donald Broadbent, Ulric Neisser, George A. Miller, Jerome Bruner) who aimed to transgress the conceptual and methodological boundaries of behaviorism by drawing on cybernetics concepts and imagery (Wieser & Slunecko, 2013 ). To justify their breaking with the discipline’s past, some proponents of cognitive psychology adopted Kuhn’s terms and declared that a “cognitive revolution” was taking place in psychology (Palermo, 1971 ; Segal & Lachman, 1972 ; Weimer & Palermo, 1973 ; Buss, 1978 ; Dember, 1978 ). Although Kuhn was skeptical whether contemporary psychology could be defined as a “paradigmatic” science (which would be a necessary ingredient for a revolution taking place), and although there were no widely accepted unsolved “puzzles” or “anomalies”—at least in the eyes of behaviorists, who dominated the field in the United States until then—Kuhn’s positive notions of “crisis” and “revolution” (which were pictured as the true motors of scientific progress) seemed to perfectly match with the needs and goals of cognitive psychologists at that point in time:
Participating in a scientific revolution at the same time as a political one unified personal and professional lives, heightened the romantic sense of making epochal change, and made the changing times that much more exciting. Surely, it was satisfying to attack tenured old fogies, supported by a scholarly reference to “Kuhn, 1962 .” (Leahey, 1992 , p. 315; see also Green, 2004 )
Although Thomas Kuhn’s work still stands as a classic of its own, it was also strongly criticized for its unclear use of the concept of “paradigm” and challenged by historians and philosophers for other reasons as well (cf. Lakatos & Musgrawe, 1970 ). Cognitive psychologists mostly ignored the debates about the weaknesses of Kuhn’s analysis (and his kinship to logical positivism, cf. Friedman, 1999 ), as they preferred to interpret his description of scientific progress as an instruction on how to turn psychology into a proper science (Driver-Linn, 2003 ). It still remains somewhat paradoxical that psychology’s textbooks speak of the “cognitive revolution” of the 1960s (as if it was unanimously clear that there had been a “revolution”) while skipping the question whether there had been any “crisis” in a Kuhnian sense beforehand. A classic example of this narrative can be found in the 2014 reprint of Ulric Neisser’s Cognitive Psychology from 1967 . In it, the author does not mention the concept of “crisis,” but heavily draws on the idea of the historical necessity of a revolution in the 1960s: “The cognitive movement was a scientific revolution and Cognitive Psychology became the rallying cry for the cognitive revolution. In the 1950s and 60s, Psychology needed a scientific revolution. In Kuhnian terms, the field was ready” (Hyman, in Neisser, 2014 , p. xv).
Not all psychologists agreed with the rhetoric of the “revolution” of the 1960s and the uncritical reproduction of this narrative in the following decades. As early as in 1972 , Neil Warren lamented the “idle chatter in loose Kuhnian terms about psychology these days” and pointed out that the “vague, varied, and cavalier usage of the concept of paradigm bears little relation to Kuhn’s original conception” (Warren, 1972 , p. 1196). Jerome Bruner ( 1990 ) and Jean-Pierre Dupuy ( 2009 ) argued that the revolution did happen, but it had lost its scope and former critical orientation. Worst of all, the cognitive turn failed to integrate subjectivity and the key category of “meaning” into psychology. As Bruner argued, it “had been diverted from its originating impulse by the computational metaphor” (Bruner, 1990 , p. 33). Despite these critical voices, the narrative of the “cognitive revolution” seems to have taken a life on its own since the 1970s, disconnected from Kuhn’s later work as well as all other debates about his work in philosophical and historical departments. Spreading into almost every textbook of experimental psychology, the idea of a scientific “revolution” of the 1960s seems to have become a matter of identity for mainstream Western psychology. However, the concept of “crisis” sensu Kuhn , defined as a necessary precondition for a “legitimate” scientific revolution, was soon forgotten in psychological circles. But if there were no unexpected anomalies, no behaviorist “puzzles” waiting to be “solved” by cognitive psychologists, if there was no crisis—why would there have been a “revolution” in the first place? One answer to this question was suggested by Thomas Leahey ( 1992 ), who argued that the recurring narrative of the cognitive “revolution” merely served a rhetorical purpose, a narrative that provided cognitive psychology with a legitimizing “origin myth.” In Leahey’s eyes, there never was a real shift of paradigms, but just the continuation of a social technology of prediction and control that was just dressed up in a new cybernetic guise.
The Infinite Quest for Unification
Although Kuhn’s concept of crisis received less attention in psychological discourses than his ideas of a scientific revolution, this does not mean that psychological crisis literature completely diminished after World War II. In fact, the psychological textbooks and journals from the second half of the 20th century are overfilled with diagnoses of a discipline in crisis and conflict. One particularly outstanding strain of the theme is an heir of C. P. Snow’s famous Rede lecture on “The Two Cultures and the Scientific Revolution,” which was held at the University of Cambridge in 1959 . Trained as a physicist and working as a novelist, Snow bemoaned and criticized a widening cultural gap between the two “cultures” he knew very well: science and technology on the one side, and the arts and humanities on the other. The natural sciences not only decided the outcome of both world wars, Snow argued, they also determine the structure and development of all aspects of modern living. Most “literary intellectuals,” however, do not seem to be interested at all to get to know even the most basic principles of physics, biology, chemistry, or engineering. Between the scientist and the literate, Snow saw an increasing “gulf of mutual incomprehension—sometimes (particularly among the young) hostility and dislike, but most of all lack of understanding” (Snow, 2012 , p. 4). Snow’s warning that “when those two senses have grown apart, then no society is going to be able to think with wisdom” (Snow, 2012 , p. 50) was primarily addressed toward the British education system. He proposed a reform of the training of policymakers, as their education should include elements of both fields of knowledge. Snow’s critique of the “cultural” divide in education and academia was also recognized in psychological circles. “In psychology,” as Kimble noted, “these conflicting cultures exist within a single field, and those who hold opposing values are currently engaged in a bitter family feud” (Kimble, 1984 , p. 834). Based on his own studies, Kimble found a strong divide between believers of determinism and indeterminism, objectivism and intuitionism, elementarism, and holistic thinking. These and other dualisms supposedly separated humanistic and scientific psychologists. They demarcated an epistemic and moral division that Kimble also saw in connection with a widening gap between experimental researchers and clinical practitioners.
Snow and Kimble were not the only ones who were worried about the divide between different fractions in Western academia. In his address as president of the American Psychological Association of 1957 , Lee Cronbach discussed a methodological schism in psychology: “Two historic streams of method, thought, and affiliation which run through the last century of our science” (Cronbach, 1957 , p. 671)—experimental psychology, which studies isolated variables in an artificial and controlled environment, and correlational psychology, which analyses “data from Nature’s experiments” (Cronbach, 1957 , p. 672). For Cronbach, the methodological divide between experimenters and “correlators” presented a threat to psychology’s progress, for as long as these two methods could not be combined, psychology would be unable to ask “the question we really want to put to Nature, and she will never answer until our two disciplines ask it in a single voice” (Cronbach, 1957 , p. 683). As the inventor of “Cronbach’s alpha,” he was not just a pioneer in developing methods for evaluating the reliability and “construct validity” of psychological tests. Cronbach’s quest for the systematization of research instruments and the evaluation of quantitative methods was a common interest of all psychologists who were part of the movement of logical positivism and operationalism. This movement had its roots in the “Vienna Circle” which formed in the middle of the 1920s and became particularly influential in the English-speaking world after most of its members (e.g., Rudolph Carnap, Herbert Feigl, Otto Neurath, and Edgar Zilsel, among many others) emigrated to the United States in the 1930s (Stadler, 2004 ). Many experimental psychologists who were seeking admission into the Olympus of the natural sciences were drawn to the logical positivist idea of an integration of their discipline into one grand scheme of a “unified science,” a universal body of knowledge that was supposed to be built solely on empirical data and logic. Obviously, a multitude of psychological currents, schools, theories, and methods obviously therefore represented an obstacle if psychology wanted to be part of a “unified science.” In 1932 , Carnap expressed the hope that “every sentence of psychology may be formulated in physical language” (Carnap, 1932–1933 , p. 107). For a short period in the history of U.S.-American psychology, during the golden age of behaviorism, it seemed like experimental psychology was actually coming closer to this ideal. The works of Egon Brunswik, who presented the idea of psychology as a science of “objective relations” (Brunswik, 1937 ; cf. Wieser, 2014 ) or Clark Hull’s Principles of Behavior (Hull, 1943 ) represent two of the most ambitious attempts of an alliance between logical positivism and psychology, searching for one grand theoretical scheme that would encompass all of experimental psychology and connect it to the other natural sciences (cf. Smith, 1986 ).
Serious attempts to unify psychology under the umbrella of one grand neo-behaviorist theory were mostly abandoned after the death of Hull in 1952 and the rise of cybernetics in the years to follow. Although physicalism lost most of its traction for psychological theorizing, the wish for unification of psychology still lives on, especially (but not solely) in communities where logical positivism still has a strong influence. Arthur Staats, for instance, repeatedly bemoaned the “crisis of disunity,” a crisis that he described as an overload of “unrelated methods, findings, problems, theoretical languages, schismatic issues, and philosophical positions” and which, if unresolved, would “continue to grow” (Staats, 1991 , p. 899). Staats interpreted the “chaos” of psychological knowledge as a typical characteristic of a premature science. What was direly needed, in Staats’ view, was a theoretical framework to help psychology grow together and transition to a state of unity, a model which he found in his philosophy of “unified positivism.” Neither empirical data nor methodological innovations could resolve the crisis of disunity: what was needed was a concerted effort to formulate a cohesive “interlevel framework” between different fields of study, a collective theoretical effort that required its own infrastructure, organization, and—last but not least—recognition in academic psychology (cf. Staats, 1983 , 1999 ). In a similar vein, Kimble proposed his own attempt to formulate a universal “framework that aims to bring the incoherent discipline of psychology together.” This theoretical framework was built on the principle that psychology must be a “science of behaviour,” which he understood as the “expression of three latent potentials—cognition, affect, and reaction tendencies” (Kimble, 1994 , p. 510). Following Kimble’s analysis, Jason Goertzen ( 2008 ) declared that “there is a crisis in psychology, and it should be understood in terms of . . . philosophical problems which generate the fragmentation of the discipline and its knowledge” (Goertzen, 2008 , p. 834). For Goertzen, the disunity of the discipline is only a “symptom” (not the disease itself) that is caused by a long list of unresolved philosophical and epistemological problems (e.g., the relation between body and mind, the nature of subjectivity and its relation to the ideal of objective knowledge, etc.).To address these problems, Goertzen suggested bringing together “psychologists of diverse persuasions in order to jointly tackle the fundamental tensions” Goertzen, 2008 , p. 846).
Staats’, Kimble’s, and Goertzen’s analyses represent only three selected examples out of an ever-growing list of suggestions on how to approach psychology’s supposed crisis of disunity. An outstanding characteristic of the late- 20th-century variant of crisis literature is that its rhetoric is often even more dramatic than works of the interwar era, in many cases drawing a devastating image of the “inconsistent, nonconsensual, faddish, disorganized, unrelated, redundant” (Staats, 1991 , p. 910) state of psychological knowledge, “an array of bits and pieces without an organizing theme” (Kimble, 1994 , p. 510) that is unable to face its “fundamental, serious points of tension” (Goertzen, 2008 , p. 832). As alarming as these diagnoses might appear, the reactions to them varied considerably. Baars ( 1984 ), in answer to Staats’ analysis, argued that psychology has overcome its disunity after the cognitive turn, and therefore the crisis has been resolved. Stam ( 2004 ) argued that, although “profound metaphysical problems” remain unsolved, two common features have kept most of psychology’s subdisciplines stable, at least on an institutional level: a common methodology (psychometrics and statistics) and the vocabulary of functionalism (defining mental and behavioral processes as “variables”). Although these authors saw unity (at least on some levels) already at hand, others embraced the plurality of the discipline and saw it as strength, not a weakness, of psychology. Dixon ( 1983 ) grasped disunity as an “important precondition of scientific progress” (p. 337), and Bower ( 1993 ) emphasized the “inevitable consequence of increasing specialization of knowledge as our science matures” (p. 905). Others did see the growing fragmentation of psychology as problematic but considered it to be unresolvable on an academic level. Howard and Simon Gruber ( 1996 ) did acknowledge that psychology is in a crisis, but interpreted this state as an inevitable symptom of much more fundamental and bigger problems (e.g., pollution, global warming, and nuclear armament) that humankind faces at the dawn of the 21st century . Sigmund Koch, one of the most consistent writers on the topic (e.g., Koch, 1969 , 1971 , 1981 , 1993 , 1999 ), argued that all attempts of unification are doomed to failure because of philosophical and epistemological problems that ultimately cannot be resolved. Psychology, as Koch argued, should therefore rather be named “a collectivity of studies of varied cast” (Koch, 1981 , p. 268) or simply “psychological studies” (Koch, 1993 ). David Bakan ( 1996 ) argued that psychology did fall into a state of crisis for political reasons: it lost its subject matter, its method, and its mission, and forgot its humanistic mission. Although it was formerly designed to “to enlighten and liberate” (p. 335) through education and empowerment, it became a social technology of control and adjustment.
Our list of examples from psychology’s crisis literature is far from complete (cf. Goertzen, 2008 , and Green, 2015 , for an overview of recent discussions) and, for lack of space, does not elaborate on diagnoses of crises of individual subdisciplines (e.g., the crisis of U.S.-American social psychology in the 1970s, cf. Israel & Tajfel, 1972 ; Elms, 1975 ; Faye, 2012 ) or recent debates about the quality of psychological data and experimental methods (e.g., the “replication crisis,” cf. Maxwell, Lau, & Howard, 2015 ). For our context, it suffices to point out that, from the very beginning, debates on psychology’s crisis have been as fragmented and polyphonic as the discipline itself. Many (but not all!) discussants agree that the diversity of psychological currents represents an obstacle for the progress, coherence, and scientific status of the discipline, but there is very little consent on the suggested causes of the crisis (be they epistemological, methodological, institutional, or social and political) or how the crisis is supposed to be overcome. This disagreement seems to increase the tensions that psychology’s crisis literature aims to solve, and thereby reinforces the seemingly eternal circle of diagnoses, proposed solutions, critique, and disagreement.
Psychology—Forever in Crisis?
It is probably not by accident that it was during the interwar era when the growth of the discipline became increasingly problematic in the eyes of many of its proponents. Academic psychology began to institutionally detach itself from philosophy, develop several different methodological approaches, and spread out into the sphere of applied sciences. Although practitioners usually seem to be less worried about the diversity of psychological currents, their colleagues in, academia tend to see an imminent collapse of the discipline approaching, a collapse that could only be avoided if its representatives finally managed to seriously address the “real” causes of the crisis. However, despite over one hundred years of crisis literature, the variety of psychological currents continuously increased, both in the academic and the applied sphere.
Although skeptics may argue that institutional growth is not a sign of scientific success per se, and truth does not necessarily come closer when more scientists try to find it (especially if they do not have the right means to do so), it can hardly be denied that psychology’s crisis literature did not achieve much of a success to stop the branching out of different psychological schools and currents. One reason for this failure might be found in the fact that, since the very first calls of crisis, discussants often followed their own agenda when attempting to “solve” the crisis, promoting their theory or model as an all-in-one “solution” to all of psychology’s problems (for a recent example, see Henriques, 2011 ; for a critique of this attempt, see Goertzen, 2013 ). Unsurprisingly, these “partisan” (Green, 2015 ) efforts are often perceived as a threat by members of competing schools or currents and are therefore usually either silently ignored or openly rejected. Therefore, psychological crisis literature often aggravates the problems it tries to solve (Wieser, 2016 ). The persisting diversity of psychological schools and currents and the absence of any agreement on the very basic concepts, methods, and problems of psychological research seem to prevent the establishment of any kind of “overarching” or “independent” standpoint that would facilitate a description of psychological problems from a “neutral” perspective. For instance, Staats lamented that his plea for “unified positivism” was labeled by one “partisan” reviewer as “behavioristic,” whereas in his own view it represented “a general philosophy of science with no theoretical position” (Staats, 1999 , p. 9). Another example would be Karl Bühler’s attempt to unify psychology with his theory of “three aspects of one object” (Bühler, 1927 )—which was constructed to unify three grand theoretical perspectives into one scheme, but explicitly excluded psychoanalysis. A cynical commentator might say that the only moment when the crisis was “solved” and unification was achieved was after the rise of National Socialism in Germany or under Stalin in the Union of Soviet Socialist Republics (USSR) (cf. Joravsky, 1989 )—but obviously, it wasn’t arguments, but violence, that silenced all voices that did not agree with the enforced conformation of the discipline. From a historical point of view, it seems like it was ideological, political, and/or economic pressure—and not the exchange of arguments—that succeeded in the unification of the discipline—but only for as long as the pressure was strong enough to prevent the theoretical proliferation.
The seemingly eternally recurring topic of psychology’s crisis has led some authors to use the expression of a “perennial” (Giorgi, 1992 , p. 48) or “chronic” crisis, or declare that crisis had become “endemic” (Koch, 1999 , p. 92) to psychology. As was mentioned in the beginning of this paper, the concept of “crisis” was primordially used to describe a short, decisive turning point (e.g., of a disease or during a battle), so talking about a “chronic” crisis might appear somewhat paradoxical. However, this expression may not only tell us something about the defeatist attitude that has spread within the debate; it could also help us to critically reflect on the different (and often contradictory) expectations toward psychology, both from inside the discipline as well as from the outside (cf. Sturm & Mülberger, 2012 ). From this perspective, the concept of crisis may be regarded as a fruitful historical category that has proven to be quite productive through the history of psychology: when entering the arena, contributors to psychology’s crisis literature are often forced to explicate and clarify methodological and epistemological assumptions that usually remain hidden or implicit in day-to-day empirical research. Therefore, the enormous and ever-growing body of crisis literature represents a unique treasure of critical debate within academic psychology—it may be the only problem that the majority of all psychologists have ever agreed on. Psychologists who follow the positivist idea that the discipline does not deserve to be called a “proper science” unless it has achieved conceptual and methodological unification might not be happy with the prospect of a never-ending crisis. But, as was mentioned in the beginning, the Greek word “ κρίσις ” did not just include the risk of an end that is nearing—it also described a chance that was opening up for further development. For historiographers and theoreticians of psychology, the chance of psychology’s crisis lies in its rich body of theoretical debates, methodological reflections, and epistemological critique, a body that still awaits to be fully reconstructed.
- Ash, M. (1995). Gestalt psychology in German culture 1890–1967 . Cambridge, UK: Cambridge University Press.
- Ash, M. (2002). Psychologie [Psychology]. In F. Hausmann & E. Müller-Luckner (Eds.), Die Rolle der Geisteswissenschaften im Dritten Reich 1933–1945 [ The role of the humanities in the Third Reich 1933–1945 ] (pp. 229–264). München, Germany: Ouldenburg.
- Avenarius, R. (1888). Kritik der reinen Erfahrung [ Critique of pure experience ]. Leipzig, Germany: Pues.
- Baars, B. J. (1984). View from a road not taken. Contemporary Psychology , 29 (10), 804–805.
- Bakan, D. (1996). The crisis in psychology. Journal of Social Distress and the Homeless , 5 (4), 335–342.
- Bernfeld, S. (1931). Die Krise der Psychologie und die Psychoanalyse [The crisis of psychology and psychoanalysis]. Internationale Zeitschrift für Psychoanalyse , 17 (2), 176–211.
- Binet, A. (1911). Qu’est-ce qu’une emotion? Qu’est-ce qu’un acte intellectuel? L’Année psychologique , 17 , 1–47.
- Bower, G. H. (1993). The fragmentation of psychology? American Psychologist , 48 (8), 905–907.
- Bowker, G. (1993). How to be universal: Some cybernetic strategies, 1943–70. Social Studies of Science , 23 (1), 107–127.
- Bruner, J. (1990). Acts of meaning . Cambridge, MA: Harvard University Press.
- Brunswik, E. (1937). Psychology as a science of objective relations. Philosophy of Science , 4 (2), 227–260.
- Bühler, K. (1926). Die Krise der Psychologie [The crisis of psychology]. Kant-Studien , 31 (1–3), 455–526.
- Bühler, K. (1927). Die Krise der Psychologie [ The crisis of psychology ]. Jena, Germany: Fischer.
- Bühler, K. (1969) Die Uhren der Lebewesen und Fragmente aus dem Nachlass [ The clocks of the living and fragments from the estate ]. Wien, Austria: VÖAW.
- Buss, A. R. (1978). The structure of psychological revolutions. Journal of the History of the Behavioral Sciences , 14 (1), 57–64.
- Carnap, R. (1932–1933). Psychologie in physikalischer Sprache [Psychology in physical language]. Erkenntnis , 3 , 107–142.
- Carson, J. (2012). Has psychology “found its true path”? Methods, objectivity, and cries of “crisis” in early twentieth-century French psychology. Studies in History and Philosophy of Biological and Biomedical Sciences , 43 (2), 445–454.
- Chazottes, J. (1902). Le conflit actuel de la science et de la philosophie dans la psychologie. Revue philosophique , 54 , 249–259.
- Cocks, G. (1997). Psychotherapy in the Third Reich. The Göring Institute . New Brunswick, NJ: Transaction.
- Cronbach, L. (1957). The two disciplines of scientific psychology. American Psychologist , 12 (11), 671–684.
- Dember, W. N. (1978). Motivation and the cognitive revolution. American Psychologist , 29 (3), 161–168.
- De Sanctis, S. (1912). I metodi della psicologia moderna [The methods of modern psychology]. Rivista di psicologia , 7 (1), 10–26.
- De Sarlo, F. (1914). La crisi della psicologia [The crisis of psychology]. Psiche , 3 (1), 105–120.
- Dilthey, W. (1894). Ideen über eine beschreibende und zergliedernde Psychologie [Ideas concerning a descriptive and analytical psychology]. Sitzungsberichte der königlich-preußischen Akademie der Wissenschaften , 24–26 , 1309–1407.
- Dixon, R. A. (1983). Theoretical proliferation in psychology: A plea for sustained disunity. The Psychological Record , 33 (3), 337–340.
- Driesch, H. (1926). Grundprobleme der Psychologie. Ihre Krisis in der Gegenwart [ Basic problems of psychology. Its crisis in the present ]. Leipzig, Germany: Reinicke.
- Driver-Linn, E. (2003). Where is psychology going? American Psychologist , 58 (4), 269–278.
- Dupuy, J. (2009). On the origins of cognitive science. The mechanization of the mind . Cambridge, MA: MIT Press.
- Ebbinghaus, H. (1896). Über erklärende und beschreibende Psychology [On analytical and descriptive psychology]. Zeitschrift für Psychologie und Physiologie der Sinnesorgane , 9 , 161–205.
- Eine Kundgebung zu Gunsten der Erhaltung philosophischer Lehrstühle [A public call in favour of the retention of philosophical chairs]. (1913). Kant-Studien , 18 , 306–307.
- Elms, A. C. (1975). The crisis of confidence in social psychology. American Psychologist , 30 (10), 967–976.
- Erikson, E. H. (1959). Identity and the life cycle . New York, NY: International Universities Press.
- Faye, C. (2012). American social psychology: Examining the contours of the 1970s crisis. Studies in History and Philosophy of Biological and Biomedical Sciences , 43 , 514–521.
- Friedman, M. (1999). Reconsidering logical positivism: A collection of essays . Cambridge, UK: Cambridge University Press.
- Geuter, U. (1988). The professionalization of psychology in Nazi Germany . Cambridge, UK: Cambridge University Press.
- Giorgi, A. (1992). Toward the articulation of psychology as a coherent discipline. In S. Koch & D. Leary (Eds.), A century of psychology as a science (pp. 46–59). Washington, DC: American Psychological Association.
- Goertzen, J. R. (2008). On the possibility of unification. The reality and nature of the crisis in psychology. Theory & Psychology , 18 (6), 829–852.
- Goertzen, J. R. (2013). The blind leading the blind? Theory & Psychology , 23 (5), 693–694.
- Green, C. (2004). Where is Kuhn going? American Psychologist , 59 (4), 271–272.
- Green, C. (2015). Why psychology isn’t unified, and probably never will be. Review of General Psychology , 19 (3), 207–214.
- Gruber, H. , & Gruber, S. (1996). Where is the crisis in psychology? Journal of Social Distress and the Homeless , 5 (4), 347–352.
- Gundlach, H. (2012). Bühler revisited in times of war—Peter R. Hofstätter’s The crisis of psychology (1941). Studies in History and Philosophy of Biological and Biomedical Sciences , 43 (2), 504–513.
- Gutberlet, C. (1898). Die “Krisis in der Psychologie” [The “crisis in psychology”]. Philosophisches Jahrbuch , 11 , 1–19, 121–146.
- Harrington, A. (1996). Reenchanted science. Holism in German culture from Wilhelm II to Hitler . Princeton, NJ: Princeton University Press.
- Henriques, G. (2011). A new unified theory of psychology . New York, NY: Springer.
- Hofstätter, P. (1941). Die Krise der Psychologie. Betrachtungen über den Standort einer Wissenschaft im Volksganzen [The crisis of psychologie. Considerations on the position of a science within the whole of the nation]. Deutschlands Erneuerung , 25 , 561–578.
- Hull, C. (1943). Principles of behavior. An introduction to behavior theory . New York, NY: Appleton-Century-Crofts.
- Husserl, E. (1970). The crisis of European sciences and transcendental phenomenology . Evanston, IL: Northwestern University Press.
- Hyman, L. (2012). Vygotsky’s crisis: Argument, context, relevance. Studies in History and Philosophy of Biological and Biomedical Sciences , 43 (2), 473–482.
- Israel, J. , & Tajfel, H. (Eds.). 1972). The context of social psychology: A critical assessment . London, UK: Academic Press.
- James, W. (1892). A plea for psychology as a “natural science.” Philosophical Review , 1 (2), 146–153.
- Joravsky, D. (1989). Russian psychology. A critical history . Oxford, UK: Basil Blackwell.
- Juhász, A. (1929). Die “Krise” der Psychotechnik [The “crisis” of Psychotechnics]. Zeitschrift für angewandte Psychologie , 33 , 456–464.
- Kimble, G. (1984). Psychology’s two cultures. American Psychologist , 39 (8), 833–839.
- Kimble, G. (1994). A frame of reference for psychology. American Psychologist , 49 (6), 510–519.
- Klages, L. (1929). Der Geist als Widersacher der Seele [ The mind as antagonist of the soul ]. Bonn, Germany: Bouvier.
- Koch, S. (1969). Psychology cannot be a coherent science. Psychology Today , 3 (4), 64–68.
- Koch, S. (1971). Reflections on the state of psychology. Social Research , 38 , 669–709.
- Koch, S. (1981). The nature and limits of psychological knowledge: Lessons of a century qua “science.” American Psychologist , 36 (3), 257–269.
- Koch, S. (1993). “Psychology” or “the psychological studies”? American Psychologist , 48 , 902–904.
- Koch, S. (1999). The age of the “paradigm.” In D. Finkelman & F. Kessel (Eds.), Psychology in human context (pp. 91–114). Chicago, IL: University of Chicago Press.
- Koffka, K. (1926). Zur Krisis in der Psychologie [On the crisis of psychology]. Die Naturwissenschaften , 14 (25), 581–586.
- Koselleck, R. (1995). Krise [Crisis]. In O. Brunner , W. Conze & R. Koselleck (Eds.), Geschichtliche Grundbegriffe [ Basic concepts in history ] (Vol. 3, pp. 617–650). Stuttgart, Germany: Klett-Cotta.
- Kostyleff, N. (1911). La crise de la psychologie expérimetale [ The crisis of experimental psychology ]. Paris, France: Alcan.
- Kuhn, T. (1996). The structure of scientific revolutions . Chicago, IL: University of Chicago Press. (First published in 1962)
- Kusch, M. (1995). Psychologism. A case study in the sociology of philosophical knowledge . London, UK: Routledge.
- Lakatos, I. , & Musgrawe, A. (1970). Criticism and the growth of knowledge . Cambridge, UK: Cambridge University Press.
- Leahey, T. H. (1992). The mythical revolutions of American psychology. American Psychologist , 47 (2), 308–318.
- Leahey, T. H. (2001). A history of modern psychology . Upper Saddle River, NJ: Prentice Hall.
- Maxwell, S. E. , Lau, M. Y. , & Howard, G. S. (2015). Is psychology suffering from a replication crisis? What does “failure to replicate” really mean? American Psychologist , 70 (6), 487–498.
- Mülberger, A. (2012). Wundt contested: The first crisis declaration in psychology. Studies in History and Philosophy of Biological and Biomedical Sciences , 43 (2), 434–444.
- Napoli, D. (1981). Architects of adjustment . The history of the psychological profession in the United States . Port Washington, NY: Kennikat Press.
- Neisser, U. (2014). Cognitive psychology . New York, NY: Psychology Press.
- Palermo, D. (1971). Is a scientific revolution taking place in psychology? Science Studies , 1 , 135–155.
- Proietto, M. , & Lombardo, G. P. (2015). The “crisis” of psychology between fragmentation and integration: The Italian case. Theory & Psychology , 25 (3), 313–327.
- Rageot, G. (1908). Les savants et la philosophie . Paris, France: Alcan.
- Rathenau, W. (1913). Zur Kritik der Zeit [ On the critique of time ]. Berlin, Germany: Fischer.
- Rathenau, W. (1918). Zur Mechanik des Geistes [ On the mechanics of the mind ]. Berlin, Germany: Fischer.
- Ringer, F. (1969). The decline of the German mandarins. The German Academic Community, 1890–1933 . Cambridge, MA: Harvard University Press
- Röttgers, K. (1995). Kritik [Critique]. In O. Brunner , W. Conze & R. Koselleck (Eds.), Geschichtliche Grundbegriffe [ Basic concepts in history ] (Vol. 3, pp. 651–675). Stuttgart, Germany: Klett-Cotta.
- Schmidt, S. (2017). The feminist origins of the midlife crisis. The Historical Journal , 61 (2), 503–523.
- Scola, F. (1931). Literaturbericht über Karl Bühlers “Krise der Psychologie” [Literature review on Karl Bühler’s “Crisis of psychology”]. Zeitschrift für Psychologie , 123 , 172–189.
- Shannon, C. E. (1948). A mathematical theory of communication. The Bell System Technical Journal , 27 , 379–423, 623–656.
- Segal, E. M. , & Lachman, R. (1972). Complex behavior or higher mental process: Is there a paradigm shift? American Psychologist , 27 (1), 46–55.
- Slunecko, T. , & Wieser, M. (2014). Cultural-historical psychology. In T. Teo (Ed.), Encyclopedia of critical psychology (pp. 352–356). New York, NY: Springer.
- Smith, L. D. (1986). Behaviorism and logical positivism: A reassessment of the alliance . Palo Alto, CA: Stanford University Press.
- Snow, C. P. (2012). The two cultures . Cambridge, UK: Cambridge University Press.
- Spengler, O. (1918). Der Untergang des Abendlandes [ The decline of the West ]. München, Germany: Beck.
- Spranger, E. (1974). Die Frage nach der Einheit der Psychologie [The question of the unity of psychology]. In W. Eisermann (Ed.), Psychologie und Menschenbildung [ Psychology and human education ] (pp. 1–36). Tübingen, Germany: Niemeyer.
- Staats, A. W. (1983). Psychology’s crisis of disunity: Philosophy and method for a unified science . New York, NY: Praeger.
- Staats, A. W. (1991). Unified positivism and unification psychology: Fad or new field? American Psychologist , 46 (9), 899–912.
- Staats, A. W. (1999). Unifying psychology requires new infrastructure, theory, method, and a research agenda. Review of General Psychology , 3 (1), 3–13.
- Stadler, F. (Ed.). (2004). Vertriebene Vernunft I. Emigration und Exil österreichischer Wissenschaft 1930–1940 [ Expelled reason. Emigration and exile of Austrian science 1930–1940 ]. Berlin, Germany: Lit.
- Stam, H. (2004). Unifying psychology: Epistemological act or disciplinary maneuver? Journal of Clinical Psychology , 60 (12), 1259–1262.
- Sturm, T. , & Mülberger, A. (2012). Crisis discussions in psychology—New historical and philosophical perspectives. Studies in History and Philosophy of Biological and Biomedical Sciences , 43 (2), 425–433.
- Vygotsky, L. (1997). The historical meaning of the crisis in psychology: A methodological investigation. In R. W. Rieber & J. Wollock (Eds.), The collected works of L. S. Vygotsky (pp. 233–343). New York, NY: Springer.
- Warren, N. (1972). On Segal and Lachman. American Psychologist , 27 , 1196–1197.
- Watson, J. B. (1913). Psychology as the behaviorist views it. Psychological Review , 20 (2), 158–177.
- Weber, M. (1922). Wissenschaft als Beruf [Science as a profession]. In M. Weber , (Ed.), Gesammelte Aufsätze zur Wissenschaftslehre [ Collected works on the theory of science ] (pp. 524–555).Tübingen, Germany: Mohr.
- Weimer, W. B. , & Palermo, D. S. (1973). Paradigms and normal science in psychology. Science Studies , 3 (3), 211–244.
- Wiener, N. (1948). Cybernetics or control and communication in the animal and the machine . Cambridge, MA: MIT Press.
- Wieser, M. , & Slunecko, T. (2013). Images of the invisible. An account of iconic media in the history of psychology. Theory & Psychology , 23 (4), 435–457.
- Wieser, M. (2014). Remembering the “lens”: Visual transformations of a concept from Heider to Brunswik. History of Psychology , 17 (2), 83–104.
- Wieser, M. (2016). Psychology’s “crisis” and the need for reflection. A plea for modesty in psychological theorizing. Integrative Psychological and Behavioral Science , 50 (3), 359–367.
- Willy, R. (1897). Die Krisis in der Psychologie [The crisis in psychology]. Vierteljahresschrift für wissenschaftliche Philosophie , 21 , 79–96, 227–249, 332–353.
- Willy, R (1899). Die Krisis in der Psychologie [The crisis in psychology]. Leipzig, Germany: Reisland.
- Windelband, W. (1909). Die Philosophie im deutschen Geistesleben des 19. Jahrhunderts [ Philosophy in 19th-century German intellectual life ]. Tübingen, Germany: Siebeck.
- Wirth, W. (1926). Zur Widerlegung von Krisenbehauptungen in der modernen Psychologie [On the refutation of assertions of a crisis in modern psychology]. Psychologie und Medizin , 2 (1), 100–131.
- Wundt, W. (1898). Über naiven und kritischen Realismus, Zweiter Artikel. Philosophische Studien , 13 , 1–105.
- Wundt, W. (1913). Die Psychologie im Kampf ums Dasein [Psychology in the fight for its existence]. Leipzig, Germany: Kröner.
Related Articles
- Social Psychology and Language
- A Historical Overview of Psychological Inquiry as a Contested Method
Printed from Oxford Research Encyclopedias, Psychology. Under the terms of the licence agreement, an individual user may print out a single article for personal use (for details see Privacy Policy and Legal Notice).
date: 01 July 2024
- Cookie Policy
- Privacy Policy
- Legal Notice
- Accessibility
- [195.158.225.230]
- 195.158.225.230
Character limit 500 /500

IMAGES
VIDEO
COMMENTS
A positive correlation is a relationship between two variables in which both variables move in the same direction. Therefore, one variable increases as the other variable increases, or one variable decreases while the other decreases. An example of a positive correlation would be height and weight. Taller people tend to be heavier.
A correlational study is a type of research design that looks at the relationships between two or more variables. Correlational studies are non-experimental, which means that the experimenter does not manipulate or control any of the variables. A correlation refers to a relationship between two variables. Correlations can be strong or weak and ...
A correlation coefficient, often expressed as r, indicates a measure of the direction and strength of a relationship between two variables. When the r value is closer to +1 or -1, it indicates that there is a stronger linear relationship between the two variables. Correlational studies are quite common in psychology, particularly because some ...
Positive Correlation in Research. Experiment design is like crafting a blueprint for discovery. Scientists and researchers use it to test hypotheses, unearth relationships between variables, and find answers to their burning questions. ... Psychology. Delving into the human mind, psychologists use correlation to unravel the mysteries of ...
The sign—positive or negative—of the correlation coefficient indicates the direction of the relationship (Figure 1). A positive correlation means that the variables move in the same direction. Put another way, it means that as one variable increases so does the other, and conversely, when one variable decreases so does the other.
Correlational research is a type of nonexperimental research in which the researcher measures two variables and assesses the statistical relationship (i.e., the correlation) between them with little or no effort to control extraneous variables. There are essentially two reasons that researchers interested in statistical relationships between ...
The sign—positive or negative—of the correlation coefficient indicates the direction of the relationship (Figure 1). A positive correlation means that the variables move in the same direction. Put another way, it means that as one variable increases so does the other, and conversely, when one variable decreases so does the other.
The strength of a correlation between quantitative variables is typically measured using a statistic called Pearson's Correlation Coefficient (or Pearson's r).As Figure 6.4 shows, Pearson's r ranges from −1.00 (the strongest possible negative relationship) to +1.00 (the strongest possible positive relationship).
The sign—positive or negative—of the correlation coefficient indicates the direction of the relationship (Figure 1). A positive correlation means that the variables move in the same direction. Put another way, it means that as one variable increases so does the other, and conversely, when one variable decreases so does the other.
Correlational research is a type of non-experimental research in which the researcher measures two variables (binary or continuous) and assesses the statistical relationship (i.e., the correlation) between them with little or no effort to control extraneous variables. There are many reasons that researchers interested in statistical ...
In a positive correlation, the correlation coefficient value ranges from 0 to +1. A value close to +1 indicates a strong positive correlation, while a value close to 0 suggests a weak positive correlation. 4. Causation: A positive correlation does not imply causation. It only indicates that the variables tend to move together.
Correlational Research. Correlation means that there is a relationship between two or more variables (such as ice cream consumption and crime), but this relationship does not necessarily imply cause and effect. When two variables are correlated, it simply means that as one variable changes, so does the other.
What Is Positive Correlation? Positive correlation refers to a relationship between two variables where they move in the same direction, meaning an increase in one variable corresponds to an increase in the other variable.. For example, a classic illustration of positive correlation is the relationship between ice cream consumption and crime rate.
The sign—positive or negative—of the correlation coefficient indicates the direction of the relationship (Figure 2.12). A positive correlation means that the variables move in the same direction. Put another way, it means that as one variable increases so does the other, and conversely, when one variable decreases so does the other.
In 2001, Bushman and Huesmann conducted a survey-type correlational research study that found a positive correlation between watching violent television shows or movies and aggressive behavior.
Research Methods. Correlational research is a type of scientific investigation in which a researcher looks at the relationships between variables but does not vary, manipulate, or control them. It can be a useful research method for evaluating the direction and strength of the relationship between two or more different variables.
Using a correlation coefficient. In correlational research, you investigate whether changes in one variable are associated with changes in other variables.. Correlational research example You investigate whether standardized scores from high school are related to academic grades in college. You predict that there's a positive correlation: higher SAT scores are associated with higher college ...
Positive correlation implies there is a positive relationship between the two variables, i.e., when the value of one variable increases, the value of other variable also increases, and the opposite happens when the value of one variable decreases. Correlation is used in many fields, such as mathematics, statistics, economics, psychology, etc.
Although this positive correlation appears to support the researcher's hypothesis, it cannot be taken to indicate that viewing violent television causes aggressive behavior. ... Although correlational research is sometimes reported as demonstrating causality without any mention being made of the possibility of reverse causation or common ...
A correlation checks to see if two sets of numbers are related; in other words, are the two sets of numbers corresponding in some way. In the case of psychology, the numbers being analysed relate to behaviours (or variables that could affect behaviour) but actually any two variables producing quantitative data could be checked to establish ...
A Level Psychology Topic Quiz - Research Methods. A positive correlation occurs when two variables are related and as one variable increases/decreases the other also increases/decreases (i.e. they both move in the same direction). For example, you might expect to find a positive correlation between height and shoe size.
Positive Correlations in Psychology Research. Positive correlations are a fundamental concept in psychology research, helping researchers understand the relationships between different variables. In Correlational Study, When two variables exhibit a positive correlation, it means that as one variable increases, the other also increases. This ...
A correlation coefficient is a number from -1 to +1 that indicates the strength and direction of the relationship between variables. The correlation coefficient is usually represented by the letter r. The number portion of the correlation coefficient indicates the strength of the relationship. The closer the number is to 1 (be it negative or ...
The 1960s saw a resurgence of "crisis literature" and the emergence of a more positive connotation of the concept in U.S.-American experimental psychology, when it was connected with Thomas Kuhn's ideas of scientific "revolutions" and "paradigm shifts." ... p. 671)—experimental psychology, which studies isolated variables in an ...