Fixed- and Random-Effects Models
- First Online: 23 September 2021

Cite this protocol
- Steve Kanters 4 , 5
Part of the book series: Methods in Molecular Biology ((MIMB,volume 2345))
3832 Accesses
45 Citations
Deciding whether to use a fixed-effect model or a random-effects model is a primary decision an analyst must make when combining the results from multiple studies through meta-analysis. Both modeling approaches estimate a single effect size of interest. The fixed-effect meta-analysis assumes that all studies share a single common effect and, as a result, all of the variance in observed effect sizes is attributable to sampling error. The random-effects meta-analysis estimates the mean of a distribution of effects, thus assuming that study effect sizes vary from one study to the next. Under this model, variance in observed effect sizes is attributable to both sampling error (within-study variance) and statistical heterogeneity (between-study variance).
The most popular meta-analyses involve using a weighted average to combine the study-level effect sizes. Both fixed- and random-effects models use an inverse-variance weight (variance of the observed effect size). However, given the shared between-study variance used in the random-effects model, it leads to a more balanced distribution of weights than under the fixed-effect model (i.e., small studies are given more relative weight and large studies less). The standard error for these estimators also relates to the inverse-variance weights. As such, the standard errors and confidence intervals for the random-effects model are larger and wider than in the fixed-effect analysis. Indeed, in the presence of statistical heterogeneity, fixed-effect models can lead to overly narrow intervals.
In addition to commonly used, generalizable models, there are additional fixed-effect models and random-effect models that can be considered. Additional fixed-effect models that are specific to dichotomous data are more robust to issues that arise from sparse data. Furthermore, random-effects models can be expanded upon using generalized linear mixed models so that different covariance structures are used to distribute statistical heterogeneity across multiple parameters. Finally, both fixed- and random-effects modeling can be conducted using a Bayesian framework.
This is a preview of subscription content, log in via an institution to check access.
Access this chapter
- Available as PDF
- Read on any device
- Instant download
- Own it forever
- Available as EPUB and PDF
- Compact, lightweight edition
- Dispatched in 3 to 5 business days
- Free shipping worldwide - see info
- Durable hardcover edition
Tax calculation will be finalised at checkout
Purchases are for personal use only
Institutional subscriptions
Similar content being viewed by others
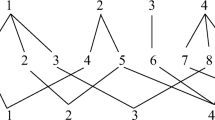
A demonstration and evaluation of the use of cross-classified random-effects models for meta-analysis
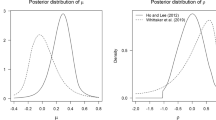
Bayesian hypothesis testing and estimation under the marginalized random-effects meta-analysis model
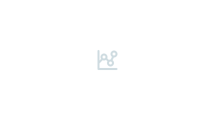
Methods for calculating confidence and credible intervals for the residual between-study variance in random effects meta-regression models
Glass G (1976) Primary, secondary, and meta-analysis of research. Educ Res 5(10):3–8
Article Google Scholar
Feinstein AR (1995) Meta-analysis: statistical alchemy for the 21st century. J Clin Epidemiol 48(1):71–79. https://doi.org/10.1016/0895-4356(94)00110-c
Article CAS PubMed Google Scholar
DerSimonian R, Laird N (2015) Meta-analysis in clinical trials revisited. Contemp Clin Trials 45(Pt A):139–145. https://doi.org/10.1016/j.cct.2015.09.002
Article PubMed PubMed Central Google Scholar
Hoaglin DC (2016) Misunderstandings about Q and ‘Cochran’s Q test’ in meta-analysis. Stat Med 35(4):485–495. https://doi.org/10.1002/sim.6632
Article PubMed Google Scholar
Mantel N, Haenszel W (1959) Statistical aspects of the analysis of data from retrospective studies of disease. J Natl Cancer Inst 22(4):719–748
CAS PubMed Google Scholar
Davey J, Turner RM, Clarke MJ, Higgins JP (2011) Characteristics of meta-analyses and their component studies in the Cochrane Database of Systematic Reviews: a cross-sectional, descriptive analysis. BMC Med Res Methodol 11:160. https://doi.org/10.1186/1471-2288-11-160
Higgins J, Thomas J, Chandler J, Cumpston M, Li T, Page M, Welch V (2019) Cochrane handbook for systematic reviews of interventions version 6, 2nd edn. Wiley, Chichester
Book Google Scholar
Bradburn MJ, Deeks JJ, Berlin JA, Russell Localio A (2007) Much ado about nothing: a comparison of the performance of meta-analytical methods with rare events. Stat Med 26(1):53–77. https://doi.org/10.1002/sim.2528
Robins J, Breslow N, Greenland S (1986) Estimators of the Mantel-Haenszel variance consistent in both sparse data and large-strata limiting models. Biometrics 42(2):311–323
Greenland S, Robins JM (1985) Estimation of a common effect parameter from sparse follow-up data. Biometrics 41(1):55–68
Simmonds MC, Higgins JP (2016) A general framework for the use of logistic regression models in meta-analysis. Stat Methods Med Res 25(6):2858–2877. https://doi.org/10.1177/0962280214534409
Van Houwelingen HC, Zwinderman KH, Stijnen T (1993) A bivariate approach to meta-analysis. Stat Med 12(24):2273–2284. https://doi.org/10.1002/sim.4780122405
Jackson D, Law M, Stijnen T, Viechtbauer W, White IR (2018) A comparison of seven random-effects models for meta-analyses that estimate the summary odds ratio. Stat Med 37(7):1059–1085. https://doi.org/10.1002/sim.7588
Higgins JP, Thompson SG, Spiegelhalter DJ (2009) A re-evaluation of random-effects meta-analysis. J R Stat Soc Ser A Stat Soc 172(1):137–159. https://doi.org/10.1111/j.1467-985X.2008.00552.x
Luce BR, Claxton K (1999) Redefining the analytical approach to pharmacoeconomics. Health Econ 8(3):187–189. https://doi.org/10.1002/(SICI)1099-1050(199905)8:3<187::AID-HEC434>3.3.CO;2-D
Spiegelhalter DJ, Abrams KR, Myles JP (2004) Bayesian approaches to clinical trials and health-care evaluation. Wiley, Chichester. vol. Book, Whole
Google Scholar
Sutton AJ, Abrams KR (2001) Bayesian methods in meta-analysis and evidence synthesis. Stat Methods Med Res 10(4):277–303. https://doi.org/10.1177/096228020101000404
Goodman S (1999) Toward evidence-based medical statistics. 1: The P value fallacy. Ann Intern Med 130(12):995
Turner RM, Jackson D, Wei Y, Thompson SG, Higgins JP (2015) Predictive distributions for between-study heterogeneity and simple methods for their application in Bayesian meta-analysis. Stat Med 34(6):984–998. https://doi.org/10.1002/sim.6381
Download references
Author information
Authors and affiliations.
School of Population and Public Health, University of British Columbia, Vancouver, BC, Canada
Steve Kanters
RainCity Analytics, Vancouver, BC, Canada
You can also search for this author in PubMed Google Scholar
Corresponding author
Correspondence to Steve Kanters .
Editor information
Editors and affiliations.
Department of Hygiene and Epidemiology University of Ioannina Medical School, Department of Epidemiology and Biostatistics Imperial College London London, UK, Ioannina, Greece
Evangelos Evangelou
Knowledge Translation Program Li Ka Shing Knowledge Institute, St. Michael’s Hospital, Department of Surgery and Cancer Faculty of Medicine Institute of Reproductive and Developmental Biology Imperial College London, UK ISSN, Toronto, ON, Canada
Areti Angeliki Veroniki
Rights and permissions
Reprints and permissions
Copyright information
© 2022 Springer Science+Business Media, LLC, part of Springer Nature
About this protocol
Kanters, S. (2022). Fixed- and Random-Effects Models. In: Evangelou, E., Veroniki, A.A. (eds) Meta-Research. Methods in Molecular Biology, vol 2345. Humana, New York, NY. https://doi.org/10.1007/978-1-0716-1566-9_3
Download citation
DOI : https://doi.org/10.1007/978-1-0716-1566-9_3
Published : 23 September 2021
Publisher Name : Humana, New York, NY
Print ISBN : 978-1-0716-1565-2
Online ISBN : 978-1-0716-1566-9
eBook Packages : Springer Protocols
- Publish with us
Policies and ethics
- Find a journal
- Track your research
- Topics Computer Setup Software Installation Developing Coding Skills Research Skills & Theses Writing an academic paper Templates and Dynamic Documents Navigating Scopus for Effective Literature Research Collect & Store Data Collection Data Storage Manage and Manipulate Data Loading and Importing Data Manipulating and Cleaning Data Analyze Data Regression Analysis Tests Causal Inference Machine Learning and Predictive Modeling Visualizing and Reporting Data Visualization Reporting Tables Automations and Workflows Workflows Automation Tools Replicability and Environment Management Version Control and Repository Management Using Artificial Intelligence Collaborate and Share Project Management and Team Sciences Share your Work
- Examples A Reproducible Research Workflow with AirBnB Data A Simple Reproducible Research Workflow A Simple Reproducible Web Scraping Example An Interactive Shiny App of Google's COVID-19 Data Exploring Trends in the Cars Dataset with Regression Analysis A Reproducible Workflow Using Snakemake and R Find Keywords and Sentences from Multiple PDF Files A Primer on Bayesian Inference for Accounting Research
- About About Tilburg Science Hub Meet our contributors Visit our blog
The Random Effects Model
The Random Effects (RE) model is the last method for panel data analysis discussed in this series of topics. Unlike the Fixed Effects (FE) model, which focuses on within-group variations, the RE model treats the unobserved entity-specific effects as random and uncorrelated with the explanatory variables. After delving into the RE model first, we address probably the most critical choice to make when working with panel data: deciding between an FE or RE model.
This is an overview of the content:
- Error term structure
Estimation in R
- Two-way RE model
- Choice between a FE and RE model
The RE model
- $invest_{it}$ is the gross investment of firm i in year t
- $value_{it}$ is the market value of assets of firm i in year t
- $capital_{it}$ is the stock value of plant and equipment of firm i in year t
- $\alpha_i$ is the fixed effect for firm i
- $\epsilon_{it}$ is the error term, which includes all other unobserved factors that affect investment but are not accounted for by the independent variables or the fixed effects.
The fixed effects $\alpha_i$ represent the time-invariant unobserved heterogeneity that differs across firms. The RE model assumes that $\alpha_i$ is uncorrelated with the explanatory variables, allowing for the inclusion of time-invariant variables such as a person’s gender or education level,
Error term in the RE model
The error term (capturing everything unobserved in the model) consists of two components:
- The individual-specific error component: $\alpha_i$.
This captures the unobserved heterogeneity varying across individuals but constant over time. It is assumed to be uncorrelated with the explanatory variables.
- The time-varying error component: $\epsilon_{it}$.
This component accounts for the within-firm variation in gross investment over time. It captures the fluctuations and changes that occur within each firm over different periods. While these time-varying effects can be correlated within the same firm, they are assumed to be uncorrelated across different firms. Note that this correlation of the error term across time is allowed in a FE model as well.
To estimate the RE model in R, use the plm() function and specify the model type as "random" .
The estimated coefficients capture the average effect of the independent variable (X) on the dependent variable (Y) while accounting for both within-entity and between-entity effects. This means that the coefficients represent the average effect of X on Y when X changes within each entity (e.g., firm) over time and when X varies between different entities.
Two-way Random Effects model
Extend the RE model to a twoway model by including time-specific effects. These time-specific effects are also unobservable and assumed to be uncorrelated with the independent variables, just like the entity-specific effects.
Include effect = "twoways" within the plm() function in R:
Choice between Fixed or Random Effects
When deciding between an FE and RE model for panel data analysis, considering the structure of your data is the most important thing to do.
FE model preference
If there is a correlation between unobserved effects and the independent variables, the FE model is preferred as it controls for time-invariant heterogeneity. This is particularly valuable when dealing with observational data where inherent differences among entities (e.g., universities or companies) could affect the outcome.
RE model preference
Opt for the RE model if you are not worried about invariant unobserved effects in the error term. This is often the case in experimental settings where you have control over treatment assignments.
One important reason to use the RE model over the FE model is when you have a specific interest in the coefficients of time-invariant variables. Unlike the FE model, the RE model allows for leaving these time-invariant variables in and estimating their impact on the outcome variable while still accounting for unobserved entity-specific differences.
Practical example: University performance analysis
Imagine you want to understand if the availability of research grants at universities affects student performance. You have panel data from universities per year, with variables describing their students' performance and research grants. You believe that universities have unobserved university-specific effects, such as its reputation, that could influence both the research grant and student performance but are not included as variables in the data.
Use the FE model
- …if you believe unobserved effects are correlated with grants availability and student performance. You want to control for university-specific factors and isolate grants impact within each university over time.
- For example, you suspect that universities with stronger reputations are more likely to secure research grants.
Use the RE model
…if you believe that unobserved university-specific effects are random and not directly tied to grant availability.
RE allows you to add multi-level fixed effects. For example, university-level FE (capturing differences across universities) and student-level FE (capturing differences across students within universities) can be added simultaneously.
For example, grant decisions are made by an external committee and largely independent of university-specific characteristics. Moreover, you want to control for both university-level and student-level variations.
Hausman test
To determine the appropriate model, a Hausman test can be conducted to test the endogeneity of the entity-specific effects.
- The null hypothesis states no correlation between the independent variables and the entity-specific effects $\alpha_i$. If $H_{0}$ is true, the RE model is preferred.
- The alternative hypothesis states a correlation between the independent variables and the entity-specific effects($\alpha_i$). If $H_{0}$ is rejected, the FE model is preferred.
The Hausman test can be performed in R with the phtest() function from the package plm . Specify the FE and RE model as arguments in this function. Note that the models included as arguments should be estimated with plm . Therefore, the Within model is also estimated with plm() first (instead of with feols() from the fixest package like in the Fixed Effects model article ).
The p-value is 0.0013, which is lower than 0.05. Thus the $H_{0}$ is rejected and an FE model is preferred according to the Hausman test.
Recommended reading
A recommended paper for delving deeper into Random Effects Models is Wooldridge (2019) , which introduces strategies for allowing unobserved heterogeneity to be correlated with observed covariates in unbalanced panels.
The Random Effects (RE) model is a method for panel data analysis that treats unobserved entity-specific effects as random and uncorrelated with the explanatory variables.
One distinct advantage of the RE model is its flexibility in allowing the inclusion of time-invariant variables, a feature not available in the FE model. Additionally, the RE model allows you to include multi-level fixed effects.
The key difference between the RE and FE model is:
- In a FE model, the unobserved effects are assumed to be correlated with the independent variables
- In a RE model, the unobserved effects are assumed to be uncorrelated with the independent variables
Related Posts
The between estimator.
A topic about the Between estimator
The Fixed Effects Model (Within Estimator)
A topic about the FE model (Within estimator)
The Pooled OLS Model
A topic about the pooled OLS model, a model used to analyze panel data
Google Analytics (functional)
This code gives us insight into the number of people that visit our website, where they are from and what they are clicking on.
Google Tag Manager (functional)
This code manages the deployment of various marketing and analytics tags on the website.
Want to know more? Check our Disclaimer .

Why Log In?
- All collections
You are here
Small Area Estimation with Random Effects Selection
- Description
Lee, Jiwon (author) She, Yiyuan (professor directing dissertation) Ökten, Giray (university representative) McGee, Daniel (committee member) Sinha, Debajyoti (committee member) Florida State University (degree granting institution) College of Arts and Sciences (degree granting college) Department of Statistics (degree granting department)
text doctoral thesis
In this study, we propose a robust method holding a selective shrinkage power for small area estimation with automatic random effects selection referred to as SARS. In our proposed model, both fixed effects and random effects are treated as joint target. In this case, maximizing joint likelihood of fixed effects and random effects makes more sense than maximizing marginal likelihood. In practice, variance of sampling error and variance of modeling error (random effects) are unknown. SARS does not require any prior information of both variance components and dimensionality of data. Furthermore, area-specific random effects, accounting for additional area variation, are not always necessary in small area estimation model. From this observation, we can impose sparsity on random effects by assigning zero for the large area. This sparsity brings heavy tails, which means that the normality assumption of random effects is not retained any longer. The SARS holding selective and predictive power employs penalized regression using a non-convex penalty. For solving the non-convex problem of SARS, we employ iterative algorithms via a quantile thresholding procedure. The algorithms make use of the iterative selection-estimation paradigm with a variety of techniques such as progressive screening when tuning parameters, muti-start strategy with subsampling method and feature subset method to generate more efficient initial points for enhancing computation efficiency and efficacy. To achieve optimal prediction error under the dimensional relaxation, we propose a new theoretical predictive information criterion for SARS (SARS-PIC) which is derived based upon non-asymptotic oracle inequalities using minimax rate of ideal predictive risk. Experiments with simulation and real poverty data of school-age(5-17) children demonstrate the efficiency of SARS.
February 6, 2017.
A Dissertation submitted to the Department of Statistics in partial fulfillment of the requirements for the degree of Doctor of Philosophy.
Includes bibliographical references.
Yiyuan She, Professor Directing Dissertation; Giray Okten, University Representative; Danniel McGee, Committee Member; Debajyoti Sinha, Committee Member.
Florida State University
FSU_2017SP_Lee_fsu_0071E_13675
This Item is protected by copyright and/or related rights. You are free to use this Item in any way that is permitted by the copyright and related rights legislation that applies to your use. For other uses you need to obtain permission from the rights-holder(s). The copyright in theses and dissertations completed at Florida State University is held by the students who author them.
http://rightsstatements.org/vocab/InC/1.0/
In Collections
- Theses and Dissertations
- Skip to search box
- Skip to main content
Princeton University Library
Panel data using stata: fixed effects and random effects, fixed effects and random effects, table of contents, 1. what is panel data , 2. panel data properties, 3. setting data as panel in stata, 4. estimating panel data models in stata , 4.1. estimating the fixed effects model in stata , 4.2 estimating the random effects model in stata , 5. fixed effects or random effects , 6. references, 1. what is panel data.
Panel data (also known as longitudinal or cross-sectional time-series data) is a dataset in which the behavior of each individual or entity (e.g., country, state, company, industry) is observed at multiple points in time.
Example:
entity time y x1 x2 x3
Angola 2018 13 6 0.5 26, angola 2019 17 4 0.3 15, angola 2020 12 7 0.9 18, brazil 2018 16 5 0.4 16, brazil 2019 11 3 0.5 19, brazil 2020 14 4 0.7 21, china 2018 11 8 0.8 14, china 2019 18 2 0.6 17, china 2020 10 5 0.2 21.
In the above Panel dataset, we have data for variables y, x1, x2, and x3 for each entity (i.e., countries - Angola, Brazil, and China) at multiple points in time (i.e., years - 2018, 2019, and 2020).
When all entities are observed across all times, we call it a balanced panel.
When some entities are not observed in some years, we call it an unbalanced panel .
The increasing availability of data observed on cross-sections of units (like households, firms, countries etc.) and over time has given rise to a number of estimation approaches exploiting this double dimensionality to cope with some of the typical problems associated with economic data.
Panel data enables us to control for individual heterogeneity. That means, Panel data allows us to control for variables you cannot observe or measure like cultural factors or difference in business practices across companies; or variables that change over time but not across entities (i.e. national policies, federal regulations, international agreements, etc.)
With panel data you can include variables at different levels of analysis (i.e. students, schools, districts, states) suitable for multilevel or hierarchical modeling.
Some drawbacks are data collection issues (i.e. sampling design, coverage), non-response in the case of micro panels or cross-country dependency in the case of macro panels (i.e. correlation between countries)
Note: For a comprehensive list of advantages and disadvantages of panel data and examples explaining this, see Baltagi, Econometric Analysis of Panel Data (chapter 1).
3. Setting Data as Panel in Stata
When we work with panel data in Stata, we need to declare that we have a panel dataset.
To get the data in Stata, type the following codes in Stata command window:
use https://dss.princeton.edu/training/Panel101_new.dta
For setting the data as Panel, type:
xtset country year
Stata will give us the following message:
. xtset country year
Panel variable: country (strongly balanced) time variable: year, 2011 to 2020 delta: 1 unit .
The term “( strongly balanced )” refers to the fact that all countries have data for all years. If, for example, a country does not have data for any year, then the data is unbalanced. Ideally, you would want to have a balanced dataset, but this is not always the case. Nevertheless, you can still run the model.
NOTE : If you get the following error after using xtset :
string variables not allowed in varlist; country is a string variable
You need to convert ‘country’ to numeric. To do this, type:
encode country, gen(country1)
Now you have to use ‘ country1 ’ instead of ‘ country ’ for xtset declaration. That means you have to type:
xtset country1 year
4. Estimating Panel Data Models in Stata
This guide discusses two basic methods we commonly use to analyze panel data:
- Fixed Effects Method
- Random Effects Method
4.1. Estimating the Fixed Effects Model in Stata
When using FE, we assume that the characteristics of an individual may impact or bias the predictor or outcome variables, and we need to control for this. This is the rationale behind the assumption of the correlation between an entity’s error term and predictor variables. FE removes the effect of those time-invariant characteristics, and therefore, we can assess the net effect of the predictors on the outcome variable.
When using FE, we assume that something within the individual may impact or bias the predictor or outcome variables, and we need to control for this. FE model removes the effects of individual or entity's time-invariant characteristics so we can assess the net effect of the predictors on the outcome variable.
The FE regression model has n different intercepts, one for each entity. These intercepts can be represented by a set of binary variables, and these binary variables absorb the influences of all omitted variables that differ from one entity to the next but are constant over time.
This guide discusses two different ways to estimate fixed effects models: (i) within estimator, (ii) dummy variable estimator .
(i) Within Estimator
This is the more commonly used estimator for fixed effects models. This estimator is called the "within estimator", as it uses time variation within each cross-section.
- Use the following dataset (ignore this step if you have already opened the dataset in the previous section)
use https://dss.princeton.edu/training/Panel101_new.dta, clear
- Declare the dataset as a panel using xtset (ignore this step if you have already declared the dataset as a panel)
- Use the following command to estimate your fixed effects model
xtreg y x1 x2, fe
Note : using the fe option indicates we estimate a fixed effects model.
Stata will give us the following results:
. xtreg y x1 x2, fe
Fixed-effects (within) regression number of obs = 70 group variable: country number of groups = 7, r-squared: obs per group: within = 0.0903 min = 10 between = 0.0546 avg = 10.0 overall = 0.0000 max = 10, f(2,61) = 3.03 corr(u_i, xb) = -0.8561 prob > f = 0.0557, ------------------------------------------------------------------------------ y | coefficient std. err. t p>|t| [95% conf. interval] -------------+---------------------------------------------------------------- x1 | 2.23e+09 1.13e+09 1.97 0.053 -2.86e+07 4.50e+09 x2 | 2.05e+09 2.00e+09 1.02 0.310 -1.95e+09 6.06e+09 _cons | 1.23e+08 7.99e+08 0.15 0.878 -1.48e+09 1.72e+09 -------------+---------------------------------------------------------------- sigma_u | 3.070e+09 sigma_e | 2.794e+09 rho | .54680874 (fraction of variance due to u_i) ------------------------------------------------------------------------------ f test that all u_i=0: f(6, 61) = 3.14 prob > f = 0.0095.
The coefficient of x1 indicates how much of Y changes over time, on average per country, when x1 increases by one unit, holding all other variables constant.
The first highlighted p-value suggests whether x1 significantly affects the dependent variable (y). As the p value is < 0.10, the coefficient for x1 is significant at 10% level.
The second highlighted p-value suggests whether the estimated model is statistically significant. As the p value is < 0.01, the model is statistically significant at 1% level.
(ii) Dummy Variable Regression
When there are a small number of fixed effects to be estimated, it is convenient to just run dummy variable regression for a FE model.
- Use the following dataset (ignore this step if you have already opened the dataset for the previous section)
reg y x1 x2 i.country
. reg y x1 x2 i.country
Source | SS df MS Number of obs = 70 -------------+---------------------------------- F(8, 61) = 2.42 Model | 1.5096e+20 8 1.8870e+19 Prob > F = 0.0245 Residual | 4.7634e+20 61 7.8088e+18 R-squared = 0.2406 -------------+---------------------------------- Adj R-squared = 0.1411 Total | 6.2729e+20 69 9.0912e+18 Root MSE = 2.8e+09
------------------------------------------------------------------------------ y | coefficient std. err. t p>|t| [95% conf. interval] -------------+---------------------------------------------------------------- x1 | 2.23e+09 1.13e+09 1.97 0.053 -2.86e+07 4.50e+09 x2 | 2.05e+09 2.00e+09 1.02 0.310 -1.95e+09 6.06e+09 | country | b | -6.77e+09 4.88e+09 -1.39 0.171 -1.65e+10 2.99e+09 c | -1.44e+09 1.96e+09 -0.74 0.464 -5.36e+09 2.47e+09 d | -2.93e+09 5.24e+09 -0.56 0.578 -1.34e+10 7.55e+09 e | -6.54e+09 5.10e+09 -1.28 0.204 -1.67e+10 3.65e+09 f | 6.14e+08 1.38e+09 0.44 0.659 -2.15e+09 3.38e+09 g | -3.32e+08 2.12e+09 -0.16 0.876 -4.56e+09 3.90e+09 | _cons | 2.61e+09 1.94e+09 1.34 0.184 -1.27e+09 6.49e+09 ------------------------------------------------------------------------------ .
Notice that the estimated coefficients for x1 and x2 are the same for both the "Within Estimator" method and the "Dummy Variable Regression" method.
- Including a lagged dependent variable as a regressor in a fixed effects model can introduce bias, a problem often referred to as the "Nickell bias" or "dynamic panel bias." This bias arises because the lagged dependent variable is correlated with the individual-specific effects, violating the assumption of strict exogeneity required for consistent estimation of fixed effects models. In this case, using dynamic panel data models such as the Arellano-Bond or the generalized method of moments (GMM) can provide consistent estimates.
4.2. Estimating the Random Effects Model in Stata
If individual or entity-specific effects are strictly uncorrelated with the regressors, it may be appropriate to model the individual or entity-specific constant terms as randomly distributed across cross-sectional units. This view would be appropriate if we believe that sampled cross-sectional units were drawn from a large population.
An advantage of using the random effects method is that you can include time-invariant variables (e.g., geographical contiguity, distance between states) in your model. In the fixed effects model, these variables are absorbed by the intercept.
- Use the following dataset
- Declare the dataset as a panel using xtset
- Use the following command to estimate your random effects model
xtreg y x1 x2, re
Note : the use of re option indicates that we are estimating a random effects model.

. xtreg y x1 x2, re
Random-effects gls regression number of obs = 70 group variable: country number of groups = 7, r-squared: obs per group: within = 0.0803 min = 10 between = 0.2333 avg = 10.0 overall = 0.0055 max = 10, wald chi2(2) = 2.24 corr(u_i, x) = 0 (assumed) prob > chi2 = 0.3261, ------------------------------------------------------------------------------ y | coefficient std. err. z p>|z| [95% conf. interval] -------------+---------------------------------------------------------------- x1 | 1.46e+09 9.78e+08 1.50 0.134 -4.53e+08 3.38e+09 x2 | 2.44e+08 4.20e+08 0.58 0.562 -5.80e+08 1.07e+09 _cons | 8.64e+08 8.48e+08 1.02 0.308 -7.98e+08 2.53e+09 -------------+---------------------------------------------------------------- sigma_u | 1.070e+09 sigma_e | 2.794e+09 rho | .12789303 (fraction of variance due to u_i) ------------------------------------------------------------------------------.
The highlighted p-value suggests whether x1 significantly affects the dependent variable (y). As the p value is not < 0.10, the coefficient for x1 is not significant at 10% level.
5. Fixed Effects or Random Effects?
Hausman Test
- Use the Hausman test to decide whether to use a fixed effects or random effects model.
- Procedures:
- Run a fixed effects model and save the estimates
- Run a random effects model and save the estimates
- Perform the Hausman test
- Use the following Stata commands
xtreg y x1 x2, fe estimates store fixed xtreg y x1 x2, re estimates store random hausman fixed random
Stata will give us the following results:
. hausman fixed random
---- coefficients ---- | (b) (b) (b-b) sqrt(diag(v_b-v_b)) | fixed random difference std. err. -------------+---------------------------------------------------------------- x1 | 2.23e+09 1.46e+09 7.69e+08 5.68e+08 x2 | 2.05e+09 2.44e+08 1.81e+09 1.96e+09 ------------------------------------------------------------------------------ b = consistent under h0 and ha; obtained from xtreg. b = inconsistent under ha, efficient under h0; obtained from xtreg., test of h0: difference in coefficients not systematic, chi2(2) = (b-b)'[(v_b-v_b)^(-1)](b-b) = 5.99 prob > chi2 = 0.0500 .
Decision rule : if the Prob > chi2 (p value) value is < 0.05, use a fixed effects model. In this case, we should use a random effect model as the p-value is = 0.05.
6. References
DSS Data Analysis Guides https://library.princeton.edu/dss/training
Princeton DSS Libguides https://libguides.princeton.edu/dss
Stata Resources https://www.stata.com/features/overview/linear-fixed-and-random-effects-models/
Angrist, J. D., & Pischke, J. S. (2009). Mostly harmless econometrics: An empiricist's companion . Princeton University Press.
Baltagi, B. (2021). Econometric analysis of panel data (6 th ed). Springer.
Bartels, B. (2008). "Beyond fixed versus random effects": a framework for improving substantive and statistical analysis of panel, time-series cross-sectional, and multilevel data. The Society for Political Methodology , 9 , 1-43. Available at: https://home.gwu.edu/~bartels/cluster.pdf
Baum, C. F. (2006). An introduction to modern econometrics using Stata . Stata Press.
Gelman, A., & Hill, J. (2007). Data analysis using regression and multilevel/hierarchical models . Cambridge University Press.
Greene, W. H. (2018). Econometric analysis (8th ed.). Pearson.
Hamilton, L. C. (2012). Statistics with Stata: version 12 . Cengage Learning.
Hoechle, D. (2007). Robust standard errors for panel regressions with cross-sectional dependence. The stata journal , 7 (3), 281-312. Available at: https://journals.sagepub.com/doi/pdf/10.1177/1536867X0700700301
Kohler, U., & Kreuter, F. (2012). Data analysis using Stata (3rd ed.). Stata Press.
Stock, J. H., & Watson, M. W. (2019). Introduction to econometrics (4th ed.). Pearson.
Wooldridge, J. M. (2010). Econometric analysis of cross section and panel data . MIT Press.
Wooldridge, J. M. (2020). Introductory econometrics: a modern approach ( 7th ed). Cengage Learning.
Data Consultant
Comments or Questions?
If you have questions or comments about this guide or method, please email [email protected] .
- Last Updated: Mar 11, 2024 8:08 PM
- URL: https://libguides.princeton.edu/stata-panel-fe-re
Help | Advanced Search
High Energy Physics - Theory
Title: quantum field theory in curved spacetime approach to the backreaction of dynamical casimir effect.
Abstract: In this thesis, we investigate the dynamical Casimir effect, the creation of particles from vacuum by dynamical boundary conditions or dynamical background, and its backreaction to the motion of the boundary. The backreaction of particle creation to the boundary motion is studied using quantum field theory in curved spacetime technique, in 1+1 dimension and 3+1 dimension. The relevant quantities in these quantum field processes are carefully analyzed, including regularization of the UV and IR divergent of vacuum energy, and estimation of classical backreaction effects like radiation pressure. We recovered the qualitative result of backreaction in 1+1 dimensions. In the 3+1 dimension, we find that the backreaction tends to slow down the system to suppress the further particle creation, similar to the case of cosmological particle creation.
Submission history
Access paper:.
- HTML (experimental)
- Other Formats
References & Citations
- INSPIRE HEP
- Google Scholar
- Semantic Scholar
BibTeX formatted citation

Bibliographic and Citation Tools
Code, data and media associated with this article, recommenders and search tools.
- Institution
arXivLabs: experimental projects with community collaborators
arXivLabs is a framework that allows collaborators to develop and share new arXiv features directly on our website.
Both individuals and organizations that work with arXivLabs have embraced and accepted our values of openness, community, excellence, and user data privacy. arXiv is committed to these values and only works with partners that adhere to them.
Have an idea for a project that will add value for arXiv's community? Learn more about arXivLabs .
Congratulations to Sean Kim on a Successful Thesis Defense!

Congratulations to Sean Kim who successfully defended his thesis, entitled “Genetic and Environmental Factors Shaping Cannabis Phenotypes: A Study on Temperature Effects and Genetic Regulation of Anthocyanin Accumulation in Cannabis sativa. ”

Sean is an aspiring cannabis breeder with hopes to create natural medicine for people around the world. Sean also p lans on starting a small cannabis operation here in Wisconsin with hopes to breed new varieties and produce high quality cannabis products.
Sean will be sticking around for a TE position through the summer as a key contributor the lab’s many research projects!
Moving forward, Sean can be contacted at 608-698-4105 or [email protected]
Thank you so much, Sean!
Log in using your username and password
- Search More Search for this keyword Advanced search
- Latest content
- Current issue
- BMJ Journals More You are viewing from: Google Indexer
You are here
- Online First
- Clinical trial design and treatment effects: a meta-analysis of randomised controlled and single-arm trials supporting 437 FDA approvals of cancer drugs and indications
- Article Text
- Article info
- Citation Tools
- Rapid Responses
- Article metrics

- http://orcid.org/0000-0003-2913-1867 Daniel Tobias Michaeli 1 ,
- http://orcid.org/0000-0003-0293-9401 Thomas Michaeli 2 , 3 , 4 ,
- http://orcid.org/0000-0002-6688-764X Sebastian Albers 5 ,
- http://orcid.org/0000-0001-6484-7161 Julia Caroline Michaeli 6
- 1 Department of Medical Oncology, National Center for Tumor Diseases , Heidelberg University Hospital , Heidelberg , Germany
- 2 Department of Personalized Oncology, University Hospital Mannheim , Heidelberg University , Mannheim , Germany
- 3 German Cancer Research Center–Hector Cancer Institute , University Medical Center Mannheim , Mannheim , Germany
- 4 Division of Personalized Medical Oncology , German Cancer Research Center , Heidelberg , Germany
- 5 Department of Trauma Surgery, Klinikum Rechts Der Isar , Technical University of Munich , Munich , Germany
- 6 Department of Obstetrics and Gynaecology, LMU University Hospital , LMU Munich , Munich , Germany
- Correspondence to Daniel Tobias Michaeli, Department of Medical Oncology, National Center for Tumor Diseases, Heidelberg University Hospital, Heidelberg, Germany; danielmichaeli{at}yahoo.com
Objectives This study aims to analyse the association between clinical trial design and treatment effects for cancer drugs with US Food and Drug Administration (FDA) approval.
Design Cross-sectional study and meta-analysis.
Setting Data from Drugs@FDA, FDA labels, ClincialTrials.gov and the Global Burden of Disease study.
Participants Pivotal trials for 170 drugs with FDA approval across 437 cancer indications between 2000 and 2022.
Main outcome measures Treatment effects were measured in HRs for overall survival (OS) and progression-free survival (PFS), and in relative risk for tumour response. Random-effects meta-analyses and meta-regressions explored the association between treatment effect estimates and clinical trial design for randomised controlled trials (RCTs) and single-arm trials.
Results Across RCTs, greater effect estimates were observed in smaller trials for OS (ß=0.06, p<0.001), PFS (ß=0.15, p<0.001) and tumour response (ß=−3.61, p<0.001). Effect estimates were larger in shorter trials for OS (ß=0.08, p<0.001) and PFS (ß=0.09, p=0.002). OS (ß=0.04, p=0.006), PFS (ß=0.10, p<0.001) and tumour response (ß=−2.91, p=0.004) outcomes were greater in trials with fewer centres. HRs for PFS (0.54 vs 0.62, p=0.011) were lower in trials testing the new drug to an inactive (placebo/no treatment) rather than an active comparator. The analysed efficacy population (intention-to-treat, per-protocol, or as-treated) was not consistently associated with treatment effects. Results were consistent for single-arm trials and in multivariable analyses.
Conclusions Pivotal trial design is significantly associated with measured treatment effects. Particularly small, short, single-centre trials testing a new drug compared with an inactive rather than an active comparator could overstate treatment outcomes. Future studies should verify results in unsuccessful trials, adjust for further confounders and examine other therapeutic areas. The FDA, manufacturers and trialists must strive to conduct robust clinical trials with a low risk of bias.
- Medical Oncology
- Drug Development
Data availability statement
All data relevant to the study are included in the article or uploaded as online supplemental information. All data used in this study were in the public domain. All data relevant to the study are included in the article or uploaded as online supplemental information.
https://doi.org/10.1136/bmjebm-2023-112544
Statistics from Altmetric.com
Request permissions.
If you wish to reuse any or all of this article please use the link below which will take you to the Copyright Clearance Center’s RightsLink service. You will be able to get a quick price and instant permission to reuse the content in many different ways.
WHAT IS ALREADY KNOWN ON THIS TOPIC
Clinical trials demonstrating a new drug’s safety and efficacy for their US Food and Drug Administration (FDA) approval ought to provide unbiased treatment effect estimates.
Prior meta-epidemiological studies found trial design can bias reported treatment outcomes.
This is the first study to analyse the association between sources of bias arising from clinical trial design features and treatment effects in trials supporting the FDA approval of new cancer drugs.
WHAT THIS STUDY ADDS
Larger treatment effects were reported for smaller (small study effect), shorter (short study effect) and single-centre trials.
Greater treatment effects were observed in trials comparing a new cancer drug to an inactive comparator (placebo/no treatment) rather than an active comparator (anticancer drug), particularly for surrogate endpoints.
HOW THIS STUDY MIGHT AFFECT RESEARCH, PRACTICE OR POLICY
Regulators, pharmaceutical companies and trialists must strive to conduct large, multicentre trials that test clinically relevant hypotheses.
Patients and physicians should critically review clinical trial design for the interpretation of overall survival, progression-free survival and tumour response outcomes.
Introduction
Randomised controlled trials (RCTs) and systematic reviews with meta-analysis of these RCTs provide the best evidence to assess a new drug’s efficacy. However, the design of RCTs may bias measured effect estimates. Previous meta-analyses and meta-epidemiological studies identified inadequate allocation concealment of randomisation, lack of blinding and a small sample may overestimate treatment effects. 1–7
Clinical trials demonstrating a new drug’s safety and efficacy for their US Food and Drug Administration (FDA) approval ought to provide unbiased effect estimates. However, concerns were raised that the FDA’s expedited review programmes, for example, accelerated approval, incentivise sponsors to conduct biased clinical trials that could overstate efficacy outcomes. 8–12 Specifically, small, short, single-centre, open-label, industry-sponsored trials comparing the new drug to an inadequate comparator were criticised to test trivial hypotheses and lead to exaggerated effect estimates. 13–21 Especially, trials measuring a subjective surrogate parameter rather than an objective clinical endpoint could be at greater risk of biased trial designs. 1 22 This could be particularly concerning for drugs whose accelerated approval relies on these potentially biased surrogate endpoints which are rarely an adequate measure for the clinical endpoint they aim to predict. 23–29 Further, trials that exclude patients postrandomisation, thereby deviating from the intention-to-treat (ITT) analysis, were found to report greater treatment effects. 30–34 Yet, to date, there has been no formal analysis that identifies and quantifies sources of bias in FDA approval trials.
In this study, we analyse the association between clinical trial design and treatment effects for cancer drugs with FDA approval. Particularly, we evaluated the association between clinical trial phase, comparator, backbone treatment, blinding, sample size, length, centres and analysed efficacy population on treatment effect estimates in trials supporting the FDA approval of 170 drugs across 437 cancer indications. We assessed overall survival (OS), progression-free survival (PFS) and tumour response treatment outcomes across potential sources of bias in single-arm trials and RCTs.
Data and methods
Sample identification.
We accessed the Drugs@FDA database to identify all new drugs and their supplemental indications, including New Drug Applications and Biologic License Applications, with FDA approval between 1 January 2000 and 1 January 2022 ( figure 1 ). The sample was then restricted to include only anticancer drugs, excluding non-oncology, supportive care and diagnostic agents while including gene therapies. For each drug, we identified all original and supplementary indications with FDA approval until 1 January 2022, excluding approvals for non-oncology indications. The sample of anticancer indications was then restricted to concurrent RCTs and single-arm trials, excluding dose-comparison trials and non-randomised trials.
- Download figure
- Open in new tab
- Download powerpoint
Flow diagram of cancer drugs and indications with FDA approval included in the analysis, 2000–2022. All drugs that received FDA approval between 1 January 2000 and 1 January 2022 were identified in the Drugs@FDA database. We then limited the sample to anticancer drugs by excluding non-oncology drugs and oncology drugs indicated for diagnostic, supportive care or antiemetic treatments while including cell and gene therapies. For each drug, we identified all original and supplementary indications with FDA approval until 1 January 2022, excluding approvals for non-oncology indications. The sample of anticancer indications was then restricted to concurrent randomised controlled trials and single-arm trials, excluding dose-comparison trials and non-randomised trials. FDA, US Food and Drug Administration.
Data collection
FDA labels for each cancer drug and indication were reviewed by two independent medical doctors to collect data on clinical trial design. The first reviewer (DTM) independently retrieved data from FDA labels, which was then cross-checked by the second reviewer (TM) with data found on clinicaltrials.gov and associated peer-reviewed publications. Disagreements were resolved in consensus or by consulting an experienced oncologist. Full details of the data extraction method have been described elsewhere. 10 35
Clinical trial design
From these data sources, we collected the following trial design features. First, we noted the trial’s phase, for example, phase 1, phase 2 or phase 3. Trials with combined phase 1 and phase 2 designs were categorised as phase 2. Accordingly, combined phase 2 and phase 3 trials were categorised as phase 3. Second, trials were classified by participant and investigator blinding into open-label/single-blind and double-blind. Third, the new drug’s direct comparator was noted. We differentiated between active and inactive, e.g. placebo/no treatment, comparators. Fourth, we differentiated between RCTs and single-arm trials. Fifth, we noted if patients in both trial arms received an active backbone anticancer drug or supportive care in addition to the intervention or comparator. Sixth, we collected the number of patients enrolled in each pivotal trial. Seventh, we approximated the trial length based on the study start and primary completion date posted on ClinicalTrials.gov. Then, the number of trial sites was noted. Finally, each trial’s efficacy population was classified as ITT, modified ITT (mITT), per-protocol (PP) or as-treated (AT).
Treatment outcomes
For RCTs, we extracted HRs for OS and/or PFS and/or the relative risk (RR) of tumour response with 95% CIs. The number of subjects and events were noted for the control and intervention arms. Median improvements in OS, PFS and duration of tumour response were calculated with IQRs. For single-arm trials, we calculated objective response rates (ORRs) based on the number of tumour responders and subjects.
Data synthesis and analysis
The association of trial design on treatment effect estimates was assessed in random-effects meta-analyses using DerSimonian and Laird method. In RCTs, HRs for OS and PFS as well as RRs for tumour response outcomes were compared by phase (phase 1/2 vs phase 3), blinding (open-label/single-blind vs double-blind), comparator (active vs inactive), backbone treatment (supportive care vs anticancer drug), the number of enrolled patients, length, sites and efficacy population. For time-to-event meta-analyses, we used HRs for OS and PFS. In single-arm trials, ORRs were compared by trial phase, size, length, sites and efficacy population. Subgroup differences in trial design characteristics were compared with Cochran’s Q-test. We further compared median improvements in OS, PFS and tumour response durations across these subgroups using Kruskal-Wallis tests. Then, the association between trial size/length/centre and treatment effects was analysed in meta-regressions. Within these meta-regressions trial sample size, length and the number of centres were transformed using the natural logarithm due to their skewed distributions.
Data were stored in Microsoft Excel (Microsoft) and analysed with Stata software, V.14.2 (StataCorp). Two-tailed p values below 0.05 were considered significant. This cross-sectional study and meta-analysis followed the Strengthening the Reporting of Observational Studies in Epidemiology and Preferred Reporting Items for Systematic Reviews and Meta-Analyses guidelines where applicable. 36 37 Given that this study was not preregistered, interpretation of results may be subject to confirmation and hindsight bias.
Sensitivity analyses
The association between clinical trial design and treatment effects was reassessed in multivariable meta-regressions. All previously examined variables, for example, trial phase, blinding, comparator, backbone treatment, size, length, sites and efficacy population, were included in the regression. We further included the treated disease’s prevalence rate (per 100 000), year of FDA approval, biomarker status and expedited FDA approval (eg, accelerated approval, fast-track review and breakthrough therapy designation) to account for the greater treatment effects observed among orphan and special-designated indications. 38 , 11 12 39 40 Additionally, we re-conducted the multivariable meta-regression analysis when limiting the sample to include only phase 3 trials.
Based on a total of 720 new drug approvals by the FDA from 2000 to 2022, we identified 170 new anticancer drugs ( figure 1 ). For these 170 anticancer drugs, we identified a total of 455 indications. Of these, 274 (60%) were supported by RCTs and 163 (36%) by single-arm trials ( table 1 ). Among these 274 RCTs, 182 (66%) reported OS, 199 (73%) PFS and 218 (80%) tumour response outcomes ( figures 2 and 3 and online supplemental figure e1 ). These pivotal clinical trials enrolled a median of 304 patients (IQR 108–615) for 3.6 years (IQR 2.5–5.2) at 99 centres (IQR 39–154) with the majority being phase 3 trials (265 (61%)) and reporting ITT analyses (311 (86%)). Among RCTs, the majority was of open-label/single-blind (160 (58%)) design comparing the new drug to no treatment or placebo (169 (62%)) without any anticancer backbone treatment (108 (39%)).
Supplemental material
- View inline
Baseline characteristics for the sample of clinical trials supporting the FDA approval of new cancer drugs
Meta-analysis comparing overall survival by pivotal clinical trial design in RCTs supporting the FDA approval of new cancer drugs. In this figure, we compared OS outcomes from randomised controlled trials across clinical trial design features. OS HRs were compared across subgroups with random-effects meta-analysis using Cochran’s Q test for subgroup differences. Median improvements in OS, defined as the OS difference between the treatment and control arm, were compared across subgroups using Kruskal-Wallis tests. a P values calculated based on Cochran’s Q test for subgroup differences. b P values calculated based on Kruskal-Wallis tests. AT, as-treated; FDA, US Food and Drug Administration; ITT, intention-to-treat; mITT, modified intention-to-treat; OS, overall survival; PP, per-protocol; RCT, randomised controlled trial.
Meta-analysis comparing progression-free survival by pivotal clinical trial design in RCTs supporting the FDA approval of new cancer drugs. In this figure, we compared PFS outcomes from RCTs across clinical trial design features. PFS HRs were compared across subgroups with random-effects meta-analysis using Cochran’s Q test for subgroup differences. Median improvements in PFS, defined as the PFS difference between the treatment and control arm, were compared across subgroups using Kruskal-Wallis tests. a P values calculated based on Cochran’s Q test for subgroup differences. b P values calculated based on Kruskal-Wallis tests. AT, as-treated; US FDA, Food and Drug Administration; ITT, intention-to-treat; mITT, modified intention-to-treat; PFS, progression-free survival; PP, per-protocol; RCT, randomised controlled trial.
Trial phase
Greater treatment effects were observed in phase 1/2 compared with phase 3 RCTs for OS (HR 0.61 vs 0.74, p=0.002) and tumour response (RR 1.78 vs 1.38, p=0.032), yet not PFS (HR 0.47 vs 0.57, p=0.065). Accordingly, median improvements in OS (4.95 months vs 2.80, p=0.002), yet not PFS (4.40 months vs 3.25, p=0.338), were greater for phase 1/2 trials. This result was not observed for single-arm trials ( online supplemental figure e2 ).
Trial blinding
There was no significant difference in OS outcomes for open-label/single-blind compared with double-blinded trials. However, greater PFS estimates were observed in double-blinded compared with open-label/single-blind trials (HR 0.51 vs 0.61, p=0.001; median 4.50 vs 2.70, p=0.008).
Trial comparator
Larger treatment effects were reported in trials comparing the new drug to an inactive (placebo/no treatment) rather than an active comparator for PFS (HR 0.54 vs 0.62, p=0.011) and tumour response (RR 1.45 vs 1.34, p=0.014), yet not OS (HR 0.74 vs 0.73, p=0.590). Median improvements in PFS were 1.70 months longer for trials with an inactive comparator (4.20 vs 2.50, p<0.001).
Trial backbone treatment
Effect estimates were greater for trials testing the new drug in combination with only supportive care rather than another anticancer backbone treatment for OS (HR 0.72 vs 0.75, p=0.038) and tumour response (RR 1.56 vs 1.28, p<0.001), yet not PFS (HR: 0.55 vs 0.59, p=0.213). Median improvements in the duration of response were 4.1 months greater for trials without an anticancer backbone treatment (6.80 vs 2.70 months, p<0.001).
Trial sample size
Treatment effect estimates decreased with a larger sample size of <250, 250–499, 500–749 and ≥750 patients in RCTs for OS (HR 0.62 vs 0.75 vs 0.74 vs 0.75, p<0.001) and PFS (HR 0.39 vs 0.51 vs 0.60 vs 0.68, p<0.001), yet not tumour response (RR 1.56 vs 1.35 vs 1.40 vs 1.42, p=0.261). A larger tumour response was observed in smaller single-arm trials (ORR 0.58 vs 0.45 vs 0.45 vs 0.40, p=0.005). Median improvements in PFS were significantly greater for smaller trials (4.10 vs 4.40 vs 2.70 vs 1.70 months, p<0.001), yet not OS (4.15 vs 2.80 vs 2.80 vs 2.20 months, p=0.157).
Results were confirmed in meta-regressions ( figure 4 ) and weighted-average regressions ( online supplemental figure e3 ). Treatment effects were significantly greater in smaller trials for OS (ß=0.06, 95% CI 0.04 to 0.09, p<0.001), PFS (ß=0.15, 95% CI 0.11 to 0.20, p<0.001) and tumour response (ß=−3.61, 95% CI −5.54 to −1.68, p<0.001).
Meta-regressions of clinical trial sample size on treatment outcomes in RCTs supporting the FDA approval of new cancer drugs. Each indication’s treatment outcome (y-axis) is mapped against the number of patients enrolled in each clinical trial (x-axis). Treatment outcomes are the HR for OS (A), the HR for PFS (B), and the relative risk of tumour response (C) reported by randomised controlled trials supporting the FDA approval of new cancer indications from 2000 to 2022. The graphs show that treatment outcomes were significantly associated with trial size for OS (ß=0.06, 95% CI 0.04 to 0.09, p<0.001), PFS (ß=0.15, 95% CI 0.11 to 0.20, p<0.001) and tumour response (ß=−3.61, 95% CI −5.54 to −1.68, p<0.001). FDA, US Food and Drug Administration; OS, overall survival; PFS, progression-free survival; RCTs, randomised controlled trials; RR, relative risk.
Trial length
Treatment effect estimates decreased with a longer trial duration of <2.0, 2.0–2.9, 3.0–3.9 and ≥4.0 years in RCTs for OS (HR 0.66 vs 0.72 vs 0.76 vs 0.77, p=0.001), PFS (HR 0.48 vs 0.56 vs 0.61 vs 0.60, p=0.028) and tumour response (RR 1.50 vs 1.54 vs 1.27 vs 1.36, p<0.001). There was no significant association between trial length and tumour response in single-arm trials. Coherently, in the meta-regression, treatment effects were significantly greater in shorter trials for OS (ß=0.08, 95% CI 0.04 to 0.11, p<0.001) and PFS (ß=0.09, 95% CI 0.03 to 0.14, p=0.002), yet not tumour response (ß=−2.42, 95% CI −5.24 to 0.41, p=0.093) ( online supplemental figure e4 ).
Study centres
In single-arm trials, larger ORRs were observed for single-centre relative to multicentre trials (71% vs 47%, p=0.005). Consistently, in RCTs, HRs and improvements in median OS and PFS were significantly associated with the number of trial centres in subgroup meta-analyses. In the conducted meta-regressions ( online supplemental figure e5 ), treatment effects were significantly greater in trials with fewer centres for OS (ß=0.04, 95% CI 0.01 to 0.07, p=0.006), PFS (ß=0.10, 95% CI 0.05 to 0.15, p<0.001) and tumour response (ß=−2.91, 95% CI −4.88 to −0.95, p=0.004).
Efficacy population
There was no consistent association between the analysed efficacy population and treatment effects across OS, PFS and tumour response outcomes.
In the multivariable meta-regressions ( online supplemental table e1 ), treatment effects were consistently larger for smaller trials across OS, PFS and tumour response outcomes. OS, yet not PFS and tumour response, was significantly associated with trial length. Tumour response rates were significantly higher for RCTs with an inactive comparator and without an anticancer backbone treatment. Overall, the multivariable regression models explained 71% of the variance between RCTs for OS, 36% for PFS and 18% for tumour response. Results were consistent when limiting the sample to include only phase 3 trials ( online supplemental table e2 ).
This study evaluated the association between clinical trial design and treatment effect estimates in a sample of 170 new drugs supporting the FDA approval of 437 cancer indications. We observed significantly larger treatment effects for smaller, shorter, single-centre trials. Greater treatment effects were observed in phase 1 or 2 relative to phase 3 trials. Further, treatment effects for OS and tumour response could be overstated among trials comparing the new drug to an inactive rather than an active comparator. Lower HRs for OS and greater tumour response rates were observed for clinical trials without an anticancer background treatment administered to both arms.
Strengths and weaknesses of this study
The results of this study are based on a large sample of clinical trials supporting the FDA approval of 170 anticancer drugs across a total of 437 indications from 2000 to 2022. The implications of our analyses are not only highly relevant for regulators, trialists and manufacturers who design clinical trials to test new drugs’ safety and efficacy but also for patients and physicians who interpret their results.
Our study is limited to clinical trials supporting the approval of new cancer drugs. Other clinical trials testing new cancer drugs’ efficacy may exist but were not included in this analysis. This study is further limited to efficacy data disclosed in FDA labels at the time of approval. Although long-term efficacy estimates are infrequently reported, 5-year or 10-year survival outcomes could increase the precision of our analyses. Coherent with previous meta-analyses of anticancer drugs, 10 29 41 42 we pooled OS, PFS and tumour response outcomes across cancer entities with medium to high heterogeneity. ‘However, it is reasonable to pool outcome data, even in the case of high heterogeneity, with previous meta-analyses demonstrating coherent interpretation of treatment outcomes across tumour types’. 10 43 Further, HRs reported in clinical trials could be influenced by the underlying statistical methods and assumptions of the survival analysis, for example, proportional versus non-proportional models. Therefore, we also evaluated median OS, PFS and DoR outcomes. Future meta-epidemiological studies could quantify the influence of the underlying statistical survival method on continuous treatment outcomes. Moreover, our study focuses on treatment effects. The association between clinical trial design and side effects should be evaluated in future studies. Further, this study was not preregistered. Therefore, interpretation of results may be subject to confirmation and hindsight bias. Additionally, this study could be subject to confounding. Although we collected data on multiple variable that could be associated with and influence treatment outcomes, non-observed variables not included in our analysis could moderate and mediate treatment outcomes. Moreover, in this study, we did not assess clinical trials’ risk of bias. However, half of pivotal clinical trials leading to the marketing authorisation of new cancer drugs were found to be at high risk of bias in the EU 22 . An analysis quantifying the association between cancer drug trials’ risk of bias and treatment effects is subject to future research. Finally, this study is limited to clinical trials for anticancer drugs. Future studies should validate our results for other therapeutic areas.
Possible mechanisms, context and policy implications
Coherent with previous meta-epidemiological studies, 2 6 7 44 we found evidence for the small study effect in our sample of FDA-approved cancer drugs. This small study effect could be explained by the following mechanisms. First, previous studies explained the small study effect by higher publication rates among larger clinical trials, regardless of the trial’s outcome (publication bias). However, in our sample, all clinical trials that received FDA approval must exhibit clinical significance in their examined endpoint. Therefore, the small study effect in our sample could rather be explained by other factors. The FDA may be prone to approve anticancer drugs exhibiting preliminary efficacy evidence in a single, small trial. However, by the sheer probability of statistical error and normality distribution, there is a greater chance of observing an above-average treatment effect in multiple small trials rather than in a single large trial. Second, large trials could display more heterogeneity in recruited patients, treatment settings and study conduct. 45 The heterogeneity between patients is particularly relevant for anticancer drugs which target biomarker-defined subgroups of a certain tumour entity. The example of PD-1/PD-L1 inhibitors highlights that targeted drugs are extremely effective for patients with a high biomarker expression. Yet, pooling the treatment effect across a large number of biomarker high, low and negative patients reduces the overall efficacy estimate. 10 Third, it is possible that anticancer drugs for rare diseases with few treatment alternatives and high unmet needs, which are more frequently supported by smaller, open-label trials, are more effective than those for common diseases. 39 Nonetheless, our results remained significant after adjusting for biomarker status and disease incidence. Finally, smaller studies are often of lower methodological quality than larger studies, resulting in greater treatment effect estimates. 2 44
In this study, shorter trials were associated with greater effect estimates. This short study effect could be explained by several mechanisms. First, shorter trials typically involve fewer patients; therefore, the association may be partially explained by the previously described mechanisms of the small study effect. Second, cancer trials for diseases with a poor prognosis, for example, small cell lung cancer, pancreatic cancer or brain cancer, are of shorter duration than those for diseases with a good prognosis, for example, breast or prostate cancer. For new drugs, it is comparatively easier to achieve a substantial survival benefit for cancers with a poor prognosis with few treatment options than those with a good prognosis. Third, anticancer drugs are frequently only administered until disease progression. In longer trials, patients have a greater chance to experience disease progression for which they may receive additional advanced-line treatments. These additional postprogression treatments may underestimate a new drug’s reported effect. Finally, there could be a long-term decrease in any new drug’s treatment effect. 46
Prior meta-epidemiological studies found larger reported treatment effects in single-centre relative to multicentre trials. 19–21 Coherently, our meta-analyses and meta-regressions showed greater reported OS, PFS and tumour response outcomesin cancer trials with fewer centres. This association could be mediated/moderated by the small study effect, short study effect, publication bias, 47 a lower methodological quality leading to a higher risk of bias 20 21 and more homogeneous/selected patient populations 48 of single-centre than multicentre trials.
Prior studies reported greater treatment effects in trials reporting outcomes based on postrandomisation deviations, for example, trials deviating from the ITT analysis. 30–34 Although there are valid reasons for excluding patients after randomisation from the efficacy analysis, scholars criticised that the inadequate exclusion of patients could bias treatment effects. 49 In contrast, in this study, there was no coherent association between the efficacy population and treatment effects. These results could be subject to the small sample of trials reporting mITT, PP or AT results. In our study, 95% of RCTs reported efficacy results based on the ITT population.
In the multivariable meta-regression analysis, greater tumour response rates were observed for clinical trials with an inactive rather than an active comparator. Naturally, comparing a new drug to no treatment or solely a placebo results in greater observed efficacy than comparing the same drug to an active comparator with any sort of anticancer potential. This observation is particularly apparent for the surrogate endpoint tumour response. Accordingly, greater treatment effects were observed in RCTs that tested the efficacy of a new drug with a backbone treatment that consisted of supportive care compared with another anticancer drug.
There is an ongoing scientific and ethical debate on the design of control arms in clinical trials supporting the FDA approval of new cancer drugs. 13–18 Industry-sponsored trials were criticised for systematically comparing their product to an inadequate comparator yielding favourable results. 13–18 Between 2013 and 2018, 16% of RCTs supporting the FDA approval of new anticancer drugs used a suboptimal control arm. 13 In particular, comparing a new drug to placebo or no treatment at all raises substantial ethical concerns. While placebo control arms may be deemed ethical for advanced-line treatment in patients who have exhausted the standard of care, they rarely represent clinical practice for early-line treatment. A previous meta-analysis found HRs of 0.82 for OS and 0.58 for PFS in trials comparing an anticancer drug to a placebo or no treatment. 50 Our analysis shows that particularly the surrogate measures of PFS and tumour response could be exaggerated by the use of an inactive rather than active comparator.
RCTs supporting the approval of new cancer drugs should test hypotheses relevant to clinical practice, rather than ‘trivialities’. 14 Of course, comparing a drug with any sort of anticancer potential to simply no treatment will yield a statistically significant effect. More clinically relevant would be to test the new drug with another anticancer agent. After a drug has received regulatory approval, payers in Europe often demand these additional head-to-head comparison trials to adequately assess and decide on the new drug’s value, price and reimbursement relative to the standard of care. 51 In the meantime, insurers often employ managed entry agreements to reimburse drugs with an uncertain safety and efficacy profile, yet high price. 35 52–54 The additional resources wasted in these head-to-head trials could perhaps be better spent on an adequate design of the initial approval trial. To ensure RCTs test clinically relevant questions and use an adequate control arm, the US Congress could grant the FDA the legal status of a comparative effectiveness authority. 11 As this is unlikely to happen anytime soon, a separate independent institution composed of clinical experts could oversee the design and conduct of clinical trials. Furthermore, future research should assess the suboptimal use of crossovers from the control to the treatment arm, which has been discussed to bias treatment effect estimates. 15 55 56
Most cancer drugs receive FDA approval based on RCTs or single-arm studies. The design of these pivotal trials is significantly associated with measured treatment effect estimates. Particularly small, short, phase 1/2 trials testing a new drug compared to an inactive rather than an active comparator and measuring surrogate endpoints could overstate treatment outcomes. The FDA, manufacturers and trialists should strive to conduct robust and unbiased clinical trials. Patients and physicians must consider the potential influence of clinical trial design on treatment outcomes when evaluating new drugs’ clinical benefits.
Ethics statements
Patient consent for publication.
Not applicable.
- Gluud LL , et al
- Kjaergard LL ,
- Villumsen J ,
- Bonis PAL ,
- Moskowitz H , et al
- Jones A , et al
- Schulz KF ,
- Chalmers I ,
- Hayes RJ , et al
- Dechartres A ,
- Trinquart L ,
- Boutron I , et al
- Reichenbach S , et al
- Darrow JJ ,
- Kesselheim AS
- Ribeiro TB ,
- Colunga-Lozano LE ,
- Araujo APV , et al
- Michaeli DT ,
- Michaeli T ,
- Albers S , et al
- Sonbol MB ,
- Gonzalez-Velez M ,
- Lathyris DN ,
- Patsopoulos NA ,
- Salanti G , et al
- Ioannidis JPA
- Flacco ME ,
- Manzoli L ,
- Boccia S , et al
- Boutron I ,
- Trinquart L , et al
- Unverzagt S ,
- Prondzinsky R ,
- Peinemann F
- Savović J , et al
- Burotto M , et al
- Gill J , et al
- Kennedy SA , et al
- Kwong R , et al
- Gurpinar E , et al
- Tierney JF ,
- Tripepi G ,
- Chesnaye NC ,
- Dekker FW , et al
- Melander H ,
- Ahlqvist-Rastad J ,
- Meijer G , et al
- McKenzie JE ,
- Bossuyt PM , et al
- Elm E von ,
- Altman DG ,
- Egger M , et al
- Michaeli DT
- Ladanie A ,
- Schmitt AM ,
- Speich B , et al
- Salas-Vega S ,
- Iliopoulos O ,
- Mossialos E
- Ioannidis JPA ,
- Rothstein HR
- Teo KK , et al
- Cécilia-Joseph E ,
- Broët P , et al
- von Elm E ,
- Blümle A , et al
- Montedori A ,
- Bonacini MI ,
- Casazza G , et al
- Moreau Bachelard C ,
- du Rusquec P , et al
- Ferrario A ,
- Michaeli T , et al
- Olivier T ,
Supplementary materials
Supplementary data.
This web only file has been produced by the BMJ Publishing Group from an electronic file supplied by the author(s) and has not been edited for content.
- Data supplement 1
X @DTMichaeli
Contributors DTM and TM had full access to all the data in the study and took responsibility for the integrity of the data and the accuracy of the data analyses. Concept and design: all authors. Acquisition, analysis or interpretation of data: all authors. Drafting of the manuscript: DTM Critical revision of the manuscript for important intellectual content: all authors. Statistical analysis: DTM. Administrative, technical or material support: all authors. Study supervision: all authors. DTM is the guarantor. The corresponding author attests that all listed authors meet authorship criteria and that no others meeting the criteria have been omitted.
Funding The authors have not declared a specific grant for this research from any funding agency in the public, commercial or not-for-profit sectors.
Competing interests None declared.
Patient and public involvement Patients and/or the public were not involved in the design, or conduct, or reporting, or dissemination plans of this research.
Provenance and peer review Not commissioned; externally peer reviewed.
Supplemental material This content has been supplied by the author(s). It has not been vetted by BMJ Publishing Group Limited (BMJ) and may not have been peer-reviewed. Any opinions or recommendations discussed are solely those of the author(s) and are not endorsed by BMJ. BMJ disclaims all liability and responsibility arising from any reliance placed on the content. Where the content includes any translated material, BMJ does not warrant the accuracy and reliability of the translations (including but not limited to local regulations, clinical guidelines, terminology, drug names and drug dosages), and is not responsible for any error and/or omissions arising from translation and adaptation or otherwise.
Read the full text or download the PDF:
- Investigates
- Houston Life
- Newsletters
WEATHER ALERT
11 warnings and an advisory in effect for 22 regions in the area
Entertainment, man charged in random assault on actor steve buscemi in new york.
Associated Press
NEW YORK – A man wanted in connection with the random attack on actor Steve Buscemi on a New York City street earlier this month was arrested on an assault charge Friday, police said.
The 66-year-old star of “Boardwalk Empire” and “Fargo” was walking in midtown Manhattan on May 8 when a stranger punched him in the face, city police said. He was taken to a hospital with bruising, swelling and bleeding to his left eye, but was otherwise OK, his publicist said at the time.
Recommended Videos
Police charged a 50-year-old homeless man with second-degree assault on Friday afternoon in the same precinct where Buscemi was attacked. Authorities announced on Tuesday that they had identified the man as the suspect and were looking for him.
It was not immediately clear if the man had an attorney who could respond to the allegations. A phone message was left at the local public defenders' office.
Buscemi's publicist did not immediately return a message. In previous comments, they said the actor was “another victim of a random act of violence in the city” and that he was OK.
In March, Buscemi’s “Boardwalk Empire” co-star Michael Stuhlbarg was hit in the back of the neck with a rock while walking in Manhattan’s Central Park. Stuhlbarg chased his attacker, who was taken into custody outside the park.
Copyright 2024 The Associated Press. All rights reserved. This material may not be published, broadcast, rewritten or redistributed without permission.

IMAGES
VIDEO
COMMENTS
fixed effects, random effects, linear model, multilevel analysis, mixed model, population, dummy variables. Fixed and random effects In the specification of multilevel models, as discussed in [1] and [3], an important question is, which explanatory variables (also called independent variables or covariates) to give random effects.
However, random effects (RE) models—also called multilevel models, hierarchical linear models and mixed models—have gained increasing prominence in political science (Beck and Katz Reference Beck and Katz 2007) and are used regularly in education (O'Connell and McCoach Reference O'Connell and McCoach 2008), epidemiology (Duncan, Jones and ...
Statistics 514: Random Effects in CRD Spring 2019 Random Effects vs Fixed Effects • Consider factor with numerous possible levels • Want to draw inference on population of levels • Not just concerned with levels in experiment • Example of differences - Fixed: Compare reading ability of 10 2nd grade classes in NY ∗ Select a =10specific classes of interest.
Abstract and Figures. This paper assesses modelling choices available to researchers using multilevel (including longitudinal) data. We present key features, capabilities, and limitations of fixed ...
varies across a grouping variable is called a random effect (Field et al., 2012, p. 862-865). Mixed effects models can have fixed and random effects on variables of interest and are flexible to the design of a study and the goals of an analyst. For example, a fixed effect term can be the conditions or the time of a sample.
In the fixed-effect model, we concluded the observed effect size was the sum of the true effect size and a random sampling error: Ti = θ + εi where \ ( {\varepsilon}_i\sim N\left (0, {\sigma}_i^2\right) \). We can use Fig. 4 to derive the new equations describing the relationship between observed and true effects.
It thereby builds on the "within-between Random-Effects" (REWB) framework for analyzing nested data that combines the advantages of fixed and random effects models (Bartels et al., 2008; Bell ...
The Random Effects (RE) model is the last method for panel data analysis discussed in this series of topics. Unlike the Fixed Effects (FE) model, which focuses on within-group variations, the RE model treats the unobserved entity-specific effects as random and uncorrelated with the explanatory variables. After delving into the RE model first ...
ABSTRACT. In statistical studies of correlated data, there is often a debate over whether to use fixed-effects or random-effects models. We perform two simulation studies to empirically compare four different models of clustered longitudinal binary data. The goal of these studies is twofold: (1) to compare the four models in terms of estimation ...
The ELS@H data shows a similar divergence, even though it exhibits a low ICC. We also find other general trends in how the fixed-effects and mixed-effects LASSO perform in various types of data. At the end of the paper, we are able to more strongly conclude that random effects are not contributing to the low predictive signal in the data.
This thesis is an account of research undertaken between September 2015 and December 2019 at the Mathematical Sciences Institute, College of Physical and Mathematical Sciences, The Aus- ... random-effects. 1.1Linear Mixed Models Suppose that from a set of gindependent clusters, we observe clustered pairs (y i;X i), where y i represents a m
The second chapter of the thesis proposes several random effects model specifi-cations. The chapter first assumes that the strict exogeneity assumption holds for the regressors, and derives optimal (F)GLS estimators for all models accordingly, discussing the estimation processes in depth. This is utterly important, as with the
2.9 Mean bias reduction in random effects meta-analysis and meta-regression 47 ... This thesis is dedicated to my beloved uncle Athinagoras who has lost the battle with cancer in April 2016. Declaration I, Sophia Kyriakou, confirm that the work presented in this thesis is my own. Where
In this study, we propose a robust method holding a selective shrinkage power for small area estimation with automatic random effects selection referred to as SARS. In our proposed model, both fixed effects and random effects are treated as joint target. In this case, maximizing joint likelihood of fixed effects and random effects makes more ...
a separate batch of effects for each row of the ANOVA table. We connect to classical ANOVA by working with finite-sample variance components: fixed and random effects models are characterized by inferences about existing levels of a factor and new levels, respectively. We also introduce a new graphical display showing inferences about the ...
We consider mainly three types of panel data analytic models: (1) constant coefficients (pooled regression) models, (2) fixed effects models, and (3) random effects models. The fixed effects model ...
The Hausman test merely tests whether the fixed-effect model provides results that are statistically different from those of the random-effect model. I would keep in mind that FE models are always more flexible, because you don't impose any assumption on the distribution of the unobservables. Conversely, if you were sure that the distribution ...
- Use the Hausman test to decide whether to use a fixed effects or random effects model. - Procedures: - Run a fixed effects model and save the estimates - Run a random effects model and save the estimates - Perform the Hausman test - Use the following Stata commands. xtreg y x1 x2, fe estimates store fixed xtreg y x1 x2, re estimates store random
Fixed effects vs Random effects is a common question and not limited to negative binomial model. Let check the fixed effect only generalized linear model. This model has long history in statistics and is used widely at present. These models are used to describe the relation between covariates and conditional mean of the response variable.
The Importance of Random Effects in Variable Selection: A Case Study of Early Childhood Education Citation Pham, Thu Minh. 2023. The Importance of Random Effects in Variable Selection: A Case Study of
In statistics, a random effects model, also called a variance components model, is a statistical model where the model parameters are random variables.It is a kind of hierarchical linear model, which assumes that the data being analysed are drawn from a hierarchy of different populations whose differences relate to that hierarchy.A random effects model is a special case of a mixed model.
A good thesis statement acknowledges that there is always another side to the argument. So, include an opposing viewpoint (a counterargument) to your opinion. Basically, write down what a person who disagrees with your position might say about your topic. television can be educational. GENERATE YOUR THESIS.
Title: Bounds on the Distribution of a Sum of Two Random Variables: Revisiting a problem of Kolmogorov with application to Individual Treatment Effects Authors: Zhehao Zhang , Thomas S. Richardson View a PDF of the paper titled Bounds on the Distribution of a Sum of Two Random Variables: Revisiting a problem of Kolmogorov with application to ...
Amonette, Jacqueline, "Examining the effect of random student drug-testing in a high school setting" (2007). Theses and Dissertations. 771. https://rdw.rowan.edu/etd/771 This Thesis is brought to you for free and open access by Rowan Digital Works. It has been accepted for inclusion
In this thesis, we investigate the dynamical Casimir effect, the creation of particles from vacuum by dynamical boundary conditions or dynamical background, and its backreaction to the motion of the boundary. The backreaction of particle creation to the boundary motion is studied using quantum field theory in curved spacetime technique, in 1+1 dimension and 3+1 dimension. The relevant ...
Congratulations to Sean Kim who successfully defended his thesis, entitled "Genetic and Environmental Factors Shaping Cannabis Phenotypes: A Study on Temperature Effects and Genetic Regulation of Anthocyanin Accumulation in Cannabis sativa.. Prior to his time in the Ellison Lab, Sean graduated from the University of Wisconsin-Stevens Point with a B.S. in Biology.
Objectives This study aims to analyse the association between clinical trial design and treatment effects for cancer drugs with US Food and Drug Administration (FDA) approval. Design Cross-sectional study and meta-analysis. Setting Data from Drugs@FDA, FDA labels, ClincialTrials.gov and the Global Burden of Disease study. Participants Pivotal trials for 170 drugs with FDA approval across 437 ...
NEW YORK - A person wanted in connection with the random assault on actor Steve Buscemi on a New York City street earlier this month was taken into custody Friday, police said. The 66-year-old ...