Mobile Systems
Mobile devices are the prevalent computing device in many parts of the world, and over the coming years it is expected that mobile Internet usage will outpace desktop usage worldwide. Google is committed to realizing the potential of the mobile web to transform how people interact with computing technology. Google engineers and researchers work on a wide range of problems in mobile computing and networking, including new operating systems and programming platforms (such as Android and ChromeOS); new interaction paradigms between people and devices; advanced wireless communications; and optimizing the web for mobile settings. In addition, many of Google’s core product teams, such as Search, Gmail, and Maps, have groups focused on optimizing the mobile experience, making it faster and more seamless. We take a cross-layer approach to research in mobile systems and networking, cutting across applications, networks, operating systems, and hardware. The tremendous scale of Google’s products and the Android and Chrome platforms make this a very exciting place to work on these problems.
Some representative projects include mobile web performance optimization, new features in Android to greatly reduce network data usage and energy consumption; new platforms for developing high performance web applications on mobile devices; wireless communication protocols that will yield vastly greater performance over today’s standards; and multi-device interaction based on Android, which is now available on a wide variety of consumer electronics.

Recent Publications
Some of our teams.
We're always looking for more talented, passionate people.
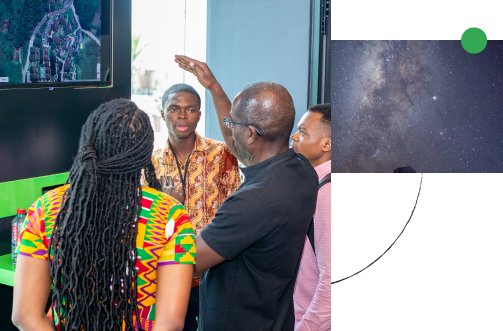
- Search Input Search Submit
- Media Center
- MobiCom 2020 Conference on Mobile Computing and Networking
MobiCom, Leading Venue for Mobile Computing and Wireless Networking Research, Goes Virtual
New York, NY, September 17, 2020 — The Association for Computing Machinery’s Special Interest Group on Mobility of Systems, Users, Data and Computing (SIGMOBILE) will hold its International Conference on Mobile Computing and Networking (MobiCom 2020) September 21-25 in a virtual setting for the first time ever.
MobiCom is a highly selective, premier international forum for addressing networks, systems, algorithms and applications that support the combination of mobile computers and mobile and wireless networks.
As in past years, the conference will feature research on the hottest innovations transforming our connected world, including computational health, machine learning for wireless networks, the impact of 5G technologies, and wireless sensing, among others.
“The world seems like a vastly different place than when we held MobiCom 2019, but the importance of mobile computing has remained the same, and may be even more vital now,” said MobiCom 2020 General Co-chair Jon Crowcroft, University of Cambridge. “As workforces, governments and other institutions around the world are operating remotely for the most part, connectivity is as important as ever. MobiCom has been and will continue to be a place where research on the solutions to today’s and tomorrow’s challenges are shared.”
“As the conference moves to a virtual platform, we have rethought the format of the conference in order to ensure that participants have opportunities to participate and interact with the presenters as well as other participants,” said General Co-chair Hamed Haddadi, Imperial College London. “In order to allow the audience to get enough technical details of the works before the sessions, a video about each paper will be made available on the MobiCom YouTube channel, and the full papers will be published in the ACM Digital Library.”
The MobiCom 2020 conference program will feature six keynote addresses, a roster of top-line research papers, workshops, panels, a Student Research Competition, and the N2Women Event, among other facets.
2020 ACM MOBICOM HIGHLIGHTS
Keynote Speakers (Partial List)
“Electronics on the Brain” George Malliaras, University of Cambridge One of the most important scientific and technological frontiers of our time is the interfacing of electronics with the human brain. Malliaras will present and discuss examples of novel devices for recording and stimulation of neurons within the brain, and show that organic electronic materials offer tremendous opportunities to study the brain and treat its pathologies. This endeavor promises to help understand how the brain works and deliver new tools for diagnosis and treatment of pathologies including epilepsy and Parkinson’s disease.
“What's next in 5G infrastructure?” Durga Malladi , Qualcomm Technologies 5G networks are poised to become the unifying connectivity fabric – across spectrum, services and deployment models. Venues, enterprises, industrial operations, healthcare, and city infrastructure will all become smart connected spaces, big or small, and in public or private networking domains. Learn how network architectures will evolve to situate network functions where they most efficiently and securely cater to the unique requirements of each use case, and scale easily with demand. Join this keynote to get perspective on some of the exciting milestones along this 5G network evolution journey such as disaggregated network functions, open interfaces, virtualization, with the opportunistic use of commercial off-the-shelf (COTS) white boxes and inline accelerators, high performance radios, and lower latency with intelligence at the wireless edge.
“From Research to Product: Stories from the Frontlines of R+D” Alex Kauffmann, Google Preparing research for production—so-called technology transfer—is, beyond the lab, a fraught and often thankless exercise in organizational diplomacy, problem solving, and improvisation. After nearly a decade of bridging the research and product worlds at Google, Kauffmann has many stories of tech transfer that highlight lessons he has learned—lessons he hopes can help others transform research into cutting-edge tech that ships quickly and at scale.
“Disaggregating the Cellular Radio Access Network with O-RAN” Sachin Katti,Stanford University and Vmware Since cellular networks have been deployed, the radio access network (RAN) has been a vertically integrated and closed part of the network. This has limited network agility, increased cost, hindered innovation and has led to vendor consolidation. Over the past few years, for the first time ever, this has begun to change. Katti and colleagues founded the xRAN forum out of our research on cellular network virtualization in 2016 which has now evolved to the O-RAN alliance that is defining the open RAN architecture. Katti will discuss the genesis of open RAN architecture, initial successes with the deployment of the Rakuten and Dish networks in Japan and the US, and the road ahead. Accepted Research Papers (Partial List) More than 62 research papers will be presented throughout nine different sessions. Below we highlight a sampling of papers to be presented. For a full list of papers, visit the MobiCom 2020 Accepted Papers page.
" Airdropping Sensor Networks from Drones and Insects " Vikram Iyer, Maruchi Kim, Qiuyue Xue, Anran Wang, Shyamnath Gollakota, University of WashingtoN The authors present the first system that can airdrop wireless sensors from small drones and live insects. In addition to the challenges of achieving low-power consumption and long-range communication, airdropping wireless sensors is difficult because it requires the sensor to survive the impact when dropped in mid-air. Their design takes inspiration from nature: small insects like ants can fall from tall buildings and survive because of their tiny mass and size. Inspired by this, they design insect-scale wireless sensors that come fully integrated with an onboard power supply and a lightweight mechanical actuator to detach from the aerial platform.
“Ghost Calls from Operational 4G Call Systems: IMS Vulnerability, Call DoS Attack, and Countermeasure” Yu-Han Lu, Chi-Yu Li, Yao-Yu Li, Sandy Hsin-Yu Hsiao, Wei-Xun Chen, National Chiao Tung University; Tan Xie, Guan-Hua Tu, Michigan State University IMS (IP Multimedia Subsystem) is an essential framework for pro-viding 4G/5G multimedia services. It has been deployed worldwide to support two call services: VoLTE (Voice over LTE) and VoWi-Fi (Voice over Wi-Fi). VoWi-Fi enables telephony calls over the Wi-Fi network to complement VoLTE. In this work, the authors uncover that the VoWi-Fi signaling session can be hijacked to maliciously manipulate the IMS call operation. The authors also propose and evaluate recommended solutions.
“Challenge: COSMOS: A City-Scale Programmable Testbed for Experimentation with Advanced Wireless” Dipankar Raychaudhuri, Ivan Seskar, Jakub Kolodziejski, Michael Sherman, Sumit Maheshwari, Rutgers University; Gil Zussman, Tingjun Chen, Zoran Kostic, Harish Krishnaswamy, Craig Gutterman, Columbia University; Thanasis Korakis, Panagiotis Skrimponis, New York University; Dan Kilper, University of Arizona; Xiaoxiong Gu, IBM Research This paper focuses on COSMOS (Cloud-enhanced Open Software-defined Mobile wireless testbed for city-scale deployment). The COSMOS testbed is being deployed in West Harlem (New York City) as part of the NSF Platforms for Advanced Wireless Research (PAWR) program. It will enable researchers to explore the technology "sweet spot" of ultra-high bandwidth and ultra-low latency in the most demanding real-world environment. The authors describe the testbed’s architecture, the design and deployment challenges, and the experience gained during the design and pilot deployment.
“EarSense: Earphones as a Teeth Activity Sensor” Jay Prakash, Singapore University of Technology and Design, University of Illinois at Urbana Champaign; Zhijian Yang, Yi-Lin Wei, Haitham Hassanieh, Romit Roy Choudhury, University of Illinois at Urbana-Champaign This paper finds that actions of the teeth, namely tapping and sliding, produce vibrations in the jaw and skull. These vibrations are strong enough to propagate to the edge of the face and produce vibratory signals at an earphone. By re-tasking the earphone speaker as an input transducer – a software modification in the sound card– the authors demonstrate that are able to sense teeth-related gestures across various models of ear/headphones.
“Self-Reconfigurable Micro-Implants for Cross-Tissue Wireless and Batteryless Connectivity” Mohamed R. Abdelhamid, Ruicong Chen, Joonhyuk Cho,Anantha P. Chandrakasan, Fadel Adib, Massachusetts Institute of Technology The paper introduces self-reconfigurable micro-implants— which are batteryless and wireless micro-implants that can change and adapt to different in-body environments while maintaining connectivity. Such sensors could be used for continuous monitoring of biomarkers and tumors, ultra-long lasting drug delivery systems (e.g., for patients with Alzheimer’s or Osteoporosis), and closed-loop control systems with real-time feedback (e.g., artificial pancreas for Diabetes’ patients.
- Workshop on Mobility in the Evolving Internet Architecture (MobiArch 2020)
- 4th International Workshop on Deep Learning for Mobile Systems and Applications (EMDL)
- 14th ACM Workshop on Wireless Network Testbeds, Experimental evaluation & CHaracterization (ACM WiNTECH 2020)
- Drone-Assisted Wireless Communications for 5G and Beyond (DroneCom)
- Cryptocurrencies and Blockchains for Distributed Systems (CryBlock)
- The 3rd Workshop on Benchmarking Cyber-Physical Systems and Internet of Things (CPS_IoTBench)
- Millimeter-Wave Networks and Sensing Systems (mmNets 2020)
- 1st Workshop on Experiences with the Design and Implementation of Frugal Smart Objects (FRUGALTHINGS 2020)
- Light up the IoT (LIoT 2020)
- COSMOS (“Cloud enhanced Open Software defined MObile wireless testbed for city-Scale deployment”) Testbed for Advanced Wireless and Edge Cloud Research
- Multipath Transport Protocols
About SIGMOBILE
SIGMOBILE, the ACM Special Interest Group on Mobility of Systems, Users, Data and Computing , is the international professional organization for scientists, engineers, executives, educators, and students dedicated to all things mobile. SIGMOBILE members work in academia, industry, and government. They are students, teachers, practitioners, policymakers, and scientists.
ACM, the Association for Computing Machinery , is the world's largest educational and scientific computing society, uniting educators, researchers, and professionals to inspire dialogue, share resources, and address the field's challenges. ACM strengthens the computing profession's collective voice through strong leadership, promotion of the highest standards, and recognition of technical excellence. ACM supports the professional growth of its members by providing opportunities for life-long learning, career development, and professional networking.
Contact: Jim Ormond ACM 212-626-0505 [email protected]
Printable PDF File

An official website of the United States government
The .gov means it’s official. Federal government websites often end in .gov or .mil. Before sharing sensitive information, make sure you’re on a federal government site.
The site is secure. The https:// ensures that you are connecting to the official website and that any information you provide is encrypted and transmitted securely.
- Publications
- Account settings
Preview improvements coming to the PMC website in October 2024. Learn More or Try it out now .
- Advanced Search
- Journal List
- Springer Nature - PMC COVID-19 Collection

Mobile Data Science and Intelligent Apps: Concepts, AI-Based Modeling and Research Directions
Iqbal h. sarker.
1 Swinburne University of Technology, Melbourne, VIC 3122 Australia
2 Chittagong University of Engineering and Technology, Chittagong, 4349 Bangladesh
Mohammed Moshiul Hoque
Md. kafil uddin, tawfeeq alsanoosy.
3 RMIT University, Melbourne, VIC 3000 Australia
Artificial intelligence (AI) techniques have grown rapidly in recent years in the context of computing with smart mobile phones that typically allows the devices to function in an intelligent manner. Popular AI techniques include machine learning and deep learning methods, natural language processing, as well as knowledge representation and expert systems, can be used to make the target mobile applications intelligent and more effective. In this paper, we present a comprehensive view on “ mobile data science and intelligent apps” in terms of concepts and AI-based modeling that can be used to design and develop intelligent mobile applications for the betterment of human life in their diverse day-to-day situation. This study also includes the concepts and insights of various AI-powered intelligent apps in several application domains, ranging from personalized recommendation to healthcare services, including COVID-19 pandemic management in recent days. Finally, we highlight several research issues and future directions relevant to our analysis in the area of mobile data science and intelligent apps. Overall, this paper aims to serve as a reference point and guidelines for the mobile application developers as well as the researchers in this domain, particularly from the technical point of view.
Introduction
Due to the recent development of science and technology in the world, the smartphone industry has made exponential growth in the mobile phone application market [ 1 ]. These devices are well known as one of the most important Internet-of-Things (IoT) devices as well, according to their diverse capabilities including data storage and processing [ 2 ]. Today’s smartphone is also considered as “a next-generation, multi-functional cell phone that facilitates data processing as well as enhanced wireless connectivity”, i.e., a combination of “a powerful cell phone” and a “wireless-enabled PDA” [ 3 ]. In our earlier paper [ 4 ], we have shown that users’ interest on “Mobile Phones” is more and more than other platforms like “Desktop Computer” , “Laptop Computer” or “Tablet Computer” for the last five years from 2014 to 2019 according to Google Trends data [ 5 ], shown in Fig. 1 .

Users’ interest trends over time where x-axis represents the timestamp information and y-axis represents the popularity score in a range of 0 (min) to 100 (max)
In the real world, people use smartphones not only for voice communication between individuals but also for various activities with different mobile apps like e-mailing, instant messaging, online shopping, Internet browsing, entertainment, social media like Facebook, Linkedin, Twitter, or various IoT services like smart cities, health or transport services, etc. [ 2 , 6 ]. Smartphone applications differ from desktop applications due to their execution environment [ 7 ]. A desktop computer application is typically designed for a static execution environment, either in-office or home, or other static locations. However, this static precondition is generally not applicable to mobile services or systems. The reason is that the world around an application is changing frequently and computing is moving toward pervasive and ubiquitous environments [ 7 ]. Thus, mobile applications should adapt to the changing environment according to the contexts and behave accordingly, which is known as context-awareness [ 8 ].
Artificial intelligence (AI) techniques have grown rapidly in recent years in the context of computing with smart mobile phones that typically allows the devices to function in an intelligent manner. AI can be applied to various types of mobile data such as structured, semi-structured, and unstructured [ 9 ]. Popular AI techniques include machine learning (ML) and deep learning (DL) methods, natural language processing (NLP), as well as knowledge representation and expert systems (ES), can be used according to their data characteristics, in order to make the target mobile applications intelligent. AI-based models and their usage in practice can be seen in many intelligent mobile applications, such as personalized recommendation, virtual assistant, mobile business, healthcare services, and even the corona-virus COVID-19 pandemic management in recent days. A brief discussion of these apps and their relation with AI techniques within the area of mobile data science has been conducted in Section 6. This made a paradigm shift to context-aware intelligent computing , powered by the increasing availability of contextual smartphone data and the rapid progress of data analytics techniques. The intelligent smartphone applications and corresponding services are considered as “context-aware” because smartphones are able to know their users’ current contexts and situations, “adaptive” because of their dynamic changing capability depending on the users’ needs, and “intelligent” because of building the model based on data-driven artificial intelligence, which makes them able to assist the end-users intelligently according to their needs in their different day-to-day situations. Thus AI-based modeling for intelligent decision making, is the key to achieve our goal in this paper.
Based on the importance of AI in mobile apps, mentioned above, in this paper, we study on mobile data science and intelligent apps that covers how the artificial intelligence methods can be used to design and develop data-driven intelligent mobile applications for the betterment of human life in different application scenarios. Thus, the purpose of this paper is to provide a base reference for those academia and industry people who want to study and develop various AI-powered intelligent mobile apps considering these characteristics rather than traditional apps, in which we are interested.
The main contributions of this paper are listed as follows:
- To provide a brief overview and concept of the mobile data science paradigm for the purpose of building data-driven intelligent apps. For this, we first briefly review the relevant methods and systems, to motivate our study in this area.
- To present AI-based modeling for intelligent mobile apps where various machine learning and deep learning algorithms, the concept of natural language processing, as well as knowledge representation and rule-based expert systems, are used.
- To discuss the usefulness of various AI-powered intelligent apps in several application domains, and the role of AI-based modeling in practice for the betterment of human life.
- To highlight and summarize the potential research directions relevant to our study and analysis in the area of mobile data science and intelligent apps.
The rest of the paper is organized as follows. Section 2 motivates and defines the scope of our study. In Section 3, we provide a background of our study including traditional data science and context-aware mobile computing, and review the works related to data-driven mobile systems and services. We define and discuss briefly about mobile data science paradigm in Section 4. In Section 5, we present our AI-based modeling within the scope of our study. Various AI-powered intelligent apps are discussed and summarized in Section 6. In section 7, we highlight and summarize a number of research issues and potential future directions. In Section 8, we highlight some key points regarding our studies, and finally, Section 9 concludes this paper.
The motivation and scope of the study
In this section, our goal is to motivate the study of exploring mobile data analytics and artificial intelligence methods that work well together in data-driven intelligent modeling and mobile applications in the interconnected world, especially in the environment of today’s smartphones and Internet-of-Things (IoT), where these devices are well known as one of the most important IoT devices. Hence, we also present the scope of our study.
We are currently living in the era of Data Science (DS), Machine Learning (ML), Artificial Intelligence (AI), Internet-of-Things (IoT), and Cybersecurity, which are commonly known as the most popular latest technologies in the fourth industrial revolution (4IR) [ 10 , 11 ]. The computing devices like smartphones and corresponding applications are now used beyond the desktop, in diverse environments, and this trend toward ubiquitous and context-aware smart computing is accelerating. One key challenge that remains in this emerging research domain is the ability to effectively process mobile data and enhance the behavior of any application by informing it of the surrounding contextual information such as temporal context, spatial context, social context, environmental or device-related context, etc. Typically, by context, we refer to any information that characterizes a situation related to the interaction between humans, applications, and the surrounding environment [ 4 , 12 ].
For AI-based modeling, several machine learning and deep learning algorithms, the concept of natural language processing, as well as knowledge representation and rule-based expert systems, can be used according to their data characteristics, in order to make the target mobile applications intelligent. For instance, machine learning (ML) algorithms typically find the insights or natural patterns in mobile phone data to make better predictions and decisions in an intelligent systems [ 13 , 14 ]. Deep learning is a part of machine learning that allows us to solve complex problems even when using a diverse data set. Natural language processing (NLP) is also an important part of AI that derives intelligence from unstructured mobile content expressed in a natural language, such as English or Bengali [ 15 ]. Another important part of AI is knowledge representation and a rule-based expert system that is also considered in our analysis. Expert system (ES) typically emulates the decision-making ability of a human expert in an intelligent system that is designed to solve complex problems by reasoning through knowledge, represented mainly as IF-THEN rules rather than conventional procedural code.
Thus, the overall performance of the AI-based mobile applications depends on the nature of the contextual data, and artificial intelligence tasks that can play a significant role to build an effective model, in which we are interested in this paper. Overall, the reasons for AI-tasks in mobile applications and systems can be summarized as below -
- to empower the evolution of the mobile industry by making smartphone apps as intelligent pieces of software that can predict future outcomes and make decisions according to users’ needs.
- to learn from data including user-centric, and device-centric contexts, by analyzing the data patterns.
- to deliver an enhanced personalized experience while adapting quickly to changing innovations and environments.
- to better utilization of available resources with higher effectiveness and efficiency.
- to understand the real-world problems and to provide intelligent and automated services accordingly as well as complex problems in this mobile domain.
- to enable the smartphones more secured through predictive analytics by taking into account possible threats in real-time.
To achieve our goal, in this study, we mainly explore mobile data science and intelligent apps that aims at providing an overview of how AI-based modeling by taking into account various techniques’ that can be used to design and develop intelligent mobile apps for the betterment of human life in various application domains, briefly discussed in Section 5, and Section 6.
Background and related work
In this section, we give an overview of the related technologies of mobile data science that include the traditional data science, as well as the computing device and Internet, and context-aware mobile computing in the scope of our study.
Data science
We are living in the age of data [ 16 ]. Thus, relevant data-oriented technologies such as data science, machine learning, artificial intelligence, advanced analytics, etc. are related to data-driven intelligent decision making in the applications. Nowadays, many researchers use the term “data science” to describe the interdisciplinary field of data collection, pre-processing, inferring, or making decisions by analyzing the data. To understand and analyze the actual phenomena with data, various scientific methods, machine learning techniques, processes, and systems are used, which is commonly known as data science. According to Cao et al. [ 16 ] “data science is a new interdisciplinary field that synthesizes and builds on statistics, informatics, computing, communication, management, and sociology to study data and its environments, to transform data to insights and decisions by following a data-to-knowledge-to-wisdom thinking and methodology”. As a high-level statement, it is the study of data to provide data-driven solutions for the given problems, as known as “the science of data”.
Computing devices and internet
The advancement of mobile computing and the Internet have played a central role in the development of the current digital age. The use of the Internet with mobile devices makes it the most popular computing device, for the people in the real world.
Mobile devices have become one of the primary ways, in which people around the globe communicate with each other for various purposes. While mobile phones may come in various forms in the real world, in this paper, they refer to smartphones or mobile devices with the capability of computing and Internet access. These devices have incorporated a variety of significant and interesting features to facilitate better information access through smart computing and the proper utilization of the devices for the benefit of the users. In recent times, the smartphones are becoming more and more powerful in both computing and the data storage capacity. As such, in addition to being used as a communication device, these smart mobile phones are capable of doing a variety of things relevant to users’ daily life such as instant messaging, Internet or web browsing, e-mail, social network systems, online shopping, or various IoT services like smart cities, health or transport services [ 2 , 6 ]. The future smartphones will be more powerful than current devices, communicate more quickly, store more data, and integrate new interaction technologies.
Context-aware Mobile computing
The notion of context has been used in numerous areas, including mobile and pervasive computing, human-centered computing, and ambient intelligence [ 17 ]. In the area of mobile and pervasive computing, several early works on context-aware computing, or context-awareness referred context as the location of people and objects [ 18 ]. Moreover, locational context, or user activities [ 17 , 18 ], temporal information [ 4 , 19 ], environmental information [ 20 ], user’s identity [ 21 ], or social context [ 22 , 23 ] are taken into account as contexts for different purposes. The state of the surrounding information of the applications are also considered as contexts in [ 24 , 25 ]. In [ 26 ], Schilit et al. claim that the important aspects of context are: (i) where you are, (ii) whom you are with, and (iii) what resources are nearby. Dey et al. [ 12 ] define context, which is perhaps now the most widely accepted. According to Dey et al. [ 12 ] “Context is any information that can be used to characterize the situation of an entity. An entity is person, place, or object that is considered relevant to the interaction between a user and an application, including the user and the application themselves”. We can also define context äs a specific type of knowledge to adapt application behavior.”
Based on the contextual information defined above, context-awareness can be the spirit of pervasive computing [ 27 ]. In general, context-awareness has adapting capability in the applications with the movement of mobile phone users, and thecontext-aware computing refers to sense the surrounding physical environment, and able to adapt application behavior. Therefore, context-awareness simply represents the dynamic nature of the applications. The use of contextual information in mobile applications is thus able to reduce the amount of human effort and attention that is needed for an application to provide the services according to user’s needs or preferences, in a pervasive computing environment [ 28 ]. Different types of contexts might have a different impact on the applications that are discussed briefly in our earlier paper, Sarker et al. [ 4 , 29 ].
Mobile systems and services
Research that relies on mobile data collected from diverse sources is mostly application-specific, which differs from application-to-application. A number of research has been done on mobile systems and services considering diverse sources of data. For instance, phone call logs [ 30 , 31 ] that contain context data related to a user’s phone call activities. In addition to call-related metadata, other types of contextual information such as user location, thesocial relationship between the caller a callee identified by the individual’s unique phone contact number are also recorded by the smart mobile phones [ 31 ]. Mobile SMS Log contains all the message including the spam and non-spam text messages [ 32 ] or good content and bad content [ 33 ] with their related contextual information such as user identifier, date, time, and other SMS related metadata, which can be used in the task of automatic filtering SMS spam for different individuals in different contexts [ 31 , 32 ], or predicting good time or bad time to deliver such messages [ 33 ]. App usages log contains various contextual information such as date, time-of-the-day, battery level, profile type such as general, silent, meeting, outdoor, offline, charging state such as charging, complete, or not connected, location such as home, workplace, on the way, etc. and other apps relatedmetadata with various kinds of mobile apps [ 34 – 38 ]. The notification log contains the contextual information such as notification type, user’s various physical activity (still, walking, running, biking and in-vehicle), user location such as home, work, or other, date, time-of-the-day, user’s response with such notifications (dismiss or accept) and other notification related metadata [ 39 ]. Weblog contains the information about user mobile web navigation, web searching, e-mail, entertainment, chat, misc., news, TV, netting, travel, sport, banking, and related contextual information such as date, time-of-the-day, weekdays, weekends [ 40 – 42 ]. Game log contains the information about playing various types such games of individual mobile phone users, and related contextual information such as date, time-of-the-day, weekdays, weekends etc. [ 43 ].
The ubiquity of smart mobile phones and their computing capabilities for vairous real life purposes provide an opportunity of using these devices as a life-logging device, i.g., personal e-memories [ 44 ]. In a more technical sense, life-logs sense and store individual’s contextual information from their surrounding environment through a variety of sensors available in their smart mobile phones, which are the core components of life-logs such as user phone calls, SMS headers (no content), App use (e.g., Skype, Whatsapp, Youtube etc.), physical activities form Google play API, and related contextual information such as WiFi and Bluetooth devices in user’s proximity, geographical location, temporal information [ 44 ]. Several applications such as smart context-aware mobile communication, intelligent mobile notification management, context-aware mobile recommendation etc. are popular in the area of mobile analytics and applications. Smart context-aware mobile communication (e.g., intelligent phone call interruption management) is one of the most compelling and widely studied applications [ 45 – 55 ]. For mobile notification management, several research [ 39 , 56 – 59 ] has been done. Similarly, a number of research [ 34 , 60 – 65 ] has been done on recommendation system.
Various techniques are used in various applications, such as interruption management, activity recognition, recommendation system, mobile commerce, etc. in the area of mobile analytics. For instance, Seo et al. [ 66 ] design a context-aware configuration manager for smartphones PYP. An intelligent interruption management system is proposed in [ 48 ], use decision tree for making decisions. Bozanta et al. [ 67 ], Lee et al. [ 68 ] use classification technique to build a personalized hybrid recommender system. Turner et al. [ 59 , 69 ], Fogarty et al. [ 70 ] use classification technique in their interruptibility predictionand management system. In the area of transportation, Bedogni et al. [ 71 ] use classification techniques in their context-aware mobile applications. To adopt mobile learning, Tan et al. [ 72 ] investigates using a multi-layer perceptron model. In [ 43 ], Paireekreng et al. have proposed a personalization mobile game recommendation system. Moreover, regression techniques such as Linear regression [ 9 ], support vector regression [ 73 ], and ensemble learning techniques, such as Random Forest learning [ 74 ] are popular in the area of supervised learning.
Beside the above mentioned approaches, several researchers [ 34 , 35 , 39 ] use association rules that are used to build various context-aware mobile service according to users needs. A number of research [ 40 , 98 – 102 ] have been done based on clustering approach for different purposes in their study. Moreover, a significant amount of research [ 72 , 94 – 97 ] have been done on deep learning for various purposes in the area of mobile analytics. Moreover, context engineering including principal component analysis, or context correlation analysis [ 77 , 78 ] is another important issue to work in this area. In Table Table1, 1 , we have summarized this research based on the most popular approaches and data-driven tasks within the scope of our analysis.
A summary of data-driven tasks and approaches used for various context-aware mobile services and systems
Tasks and Approaches | Purposes | References |
---|---|---|
Clustering | Time-based segmentation, Time-series modeling | Halvey et al. [ ], Sarker et al. [ ] |
Association | Notification management, Usage modeling, Interruption management, Recency analysis | Mehrotra et al. [ ], Srinivasan et al. [ ], Sarker et al. [ ], Sarker et al. [ ] |
Context Engineering | Apps usage, principal component analysis, Context correlation analysis, Context Pre-modeling | Sarker et al. [ ], Sarker et al. [ ] |
Support Vector Machine | Instant messaging, transportation system, activity recognition, notification management, interruptibility prediction | Pielot et al. [ ], Bedogni et al. [ ], Bayat et al. [ ], Ayu et al. [ ], Fetter et al. [ ], Turner et al. [ , ], Fogarty et al. [ ], Fisher et al. [ ] Turner et al. [ ] |
K-Nearest Neighbor | Mobile search, recommender system, location prediction, activity recognition, interruptibility prediction | Swati et al. [ ], Middleton et al. [ ], Bozanta et al. [ ], Anagnostopoulos et al. [ ], Ayu et al. [ ], Fisher et al. [ ], Turner et al. [ ] |
Logistic Regression | Activity recognition, user modeling, recommendation system, health analytics, interruptibility prediction | Riboni et al. [ ], Zhong et al. [ ], Wang et al. [ ], Ernsting et al. [ ], Turner et al. [ , ] |
Naive Bayes | Phone call prediction, location prediction, interruption management | Sarker et al. [ ], Pejovic et al. [ ], Ayu et al. [ ], Fogarty et al. [ ], Fisher et al. [ ] |
Decision Tree | Context-aware system, mobile service, interruption management, interruptibility prediction, phone call prediction | Hong et al. [ ], Lee et al. [ ], Zulkernain et. al. [ ], Turner et al. [ , ], Sarker et al. [ , ], Fogarty et al. [ ], Fisher et al. [ ] |
AdaBoost | Interruption management, interruptibility prediction, location prediction, recommeder system | Pejovic et al. [ ], Turner et al. [ , ], Anagnostopoulos et al. [ ], Fogarty et al. [ ], Middleton et al. [ ] |
Random Forest | Call availability prediction, instant messaging, transportation system, activity recognition, interruptibility prediction | Pielot et al. [ , ], Bedogni et al. [ ], Bayat et al. [ ], Ayu et al. [ ], Turner et al. [ , ] |
Neural Network | Smartphone power modeling, mobile credit card payment, mobile commerce, mobile learning, smartphone characterization | Alawnah et al. [ ], Leong et al. [ ], Chong et al. [ ], Tan et al. [ ], Rajashekar et al. [ ] |
Although various types of mobile phone data and techniques discussed above are used in the area of mobile analytics and systems for different purposes, a comprehensive AI-based modeling for building intelligent apps is being interested, according to the needs of the current in the community. Thus, in this paper, we focus on mobile data science and corresponding intelligent apps, where the most popular AI techniques including machine learning and deep learning methods, the concept of natural language processing, as well as knowledge representation and expert systems, can be used to build intelligent mobile apps in various application domains.
Mobile data science paradigm
In this section, we provide a brief overview of mobile data science and its related components within the scope of our study.
Understanding Mobile data
Mobile data science and data-driven intelligent apps are largely driven by the availability of data. Mobile datasets typically represent a collection of information records that consist of several attributes or contextual features and related facts. Thus,it’s important to understand the nature of mobile data containing various types of features and contexts. The reason is that raw data collected from relevant sources for a particular application can be used to analyze the various patterns or insight, to build a data-driven model to achieve our goal. Several datasets exist in the area of mobile analytics, such as phone call logs [ 30 ], apps logs [ 34 , 35 ], weblogs [ 40 ] etc. These context-rich historical mobile phone data are the collection of the past contextual information and users’ diverse activities [ 92 , 103 ]. Moreover, IoT data, smart cities data, business data, health data, mobile security data, or various sensors data associated with the mobile devices and target application can also be used as data sources. Intelligent apps are based on the extracted insight from such kinds of relevant datasets depending on apps characteristics. In the next, we summarize several characteristics of intelligent apps.
Intelligent apps characteristics
Intelligent apps offer personalized and adaptive user experiences, where artificial intelligence, the Internet-of-Things, and data analytics are the core components. Based on this, we have summarized the characteristics of intelligent apps to assist smartphone users in their daily life activities.
- Action-Oriented: The foremost characteristic of intelligent apps is that these applications do not wait for users to make decisions in various situations. Rather, the apps can study user behavior and deliver personalized and actionable results using the power of predictive analytics.
- Adaptive in Nature: The apps should be adaptive in nature. Every user is different in their use, the adaptability of the app plays a very crucial role. Meaning, they can easily upgrade their knowledge as per their surroundings to produce a highly-satisfying user experience.
- Suggestive and Decision-Oriented: Generating suggestions and making decisions according to users’ needs and interests, could be an interesting characteristic of an intelligent app. Such suggestions may vary from user-to-user according to their interests and helps the users to decide what suits best for them.
- Data-driven: Delivering a data-driven output is also one of the key features of intelligent apps. The intelligent apps gather data from a variety of sources, such as online, user interaction, sensors, etc. relevant to the target application and extracting data patterns, thus providing better user experience.
- Context-awareness: Context awareness is the ability of an application to gather information about its surrounding environment at any given time and adapt behaviors accordingly. It makes the apps much smarter use by taking into account users contexts as well as the device’s contexts to proactively deliver highly relevant information and suggestions.
- Cross-Platform Operation: The app also should have the ability to understand and process the desired output in a way that the users feel the same experience while working on cross platforms.
In this study, we take into account the above-discussed characteristics of mobile apps that could be able to intelligently assist the users in their diverse daily life activities. Based on these characteristics, in the next, we briefly discuss the concept of mobile data science and AI that can help to achieve the goal.
Mobile data science and AI
Data science is transforming the world’s industries. It is critically important for the future of intelligent mobile apps and services because of “apps intelligence is all about mobile data”. Traditionally, mobile application developers didn’t use data science techniques to make the apps intelligent considering the above characteristics. Although, a number of recent research [ 4 , 29 , 34 , 38 , 48 ] has been done based on machine learning techniques to model and build mobile applications, most of existing mobile applications are static or used custom-written rules like signatures, or manually defined heuristics for their different applications [ 47 , 66 ]. The main drawback of these custom-written rules-based approaches is that the knowledge or rules used by the applications are not automatically discovered; users need to define and maintain the rules manually. In general, users may not have the time, inclination, expertise, or interest to maintain rules manually. Although these rule-based approaches have their own merits in several cases, it needs too much manual work to keep up with the changing of userscontext landscape. On the contrary, data science can make a massive shift in technology and its operations, where AI techniques including machine learning and deep learning methods, natural language processing, as well as knowledge representation and expert systems, can be used to learn and making intelligent decisions. Thus, data science is considered as a practical application of machine learning, a major part of AI, with a complete focus on solving real-world problems. Overall, data science is a comprehensive process that involves data collection, pre-processing, data analysis, visualization, and decision making [ 16 ], whereas AI makes use of computer algorithms that can show human intelligence.
The concept of mobile data science incorporates the methods and techniques of machine learning and AI and data science as well as the context-aware computing to build intelligent mobile apps. The combination of these technologies has given birth to the term “mobile data science”, which refers to collect a large amount of mobile data from different sources and analyze it using machine learning techniques through the discovery of useful insights or the data-driven patterns, which is primarily defined in our earlier paper [ 104 ]. It is, however, worth remembering that mobile data science is not just about a collection of AI techniques. Mobile data science is a process that can help mobile application developers or analysts to scale and automate the target apps in a smart way and in a timely manner. Thus in a broader sense, we can say that “Mobile data science is research or working area existing at the intersection of context-aware mobile computing, data science, and artificial intelligence, which is mainly data-focused associated with target mobile apps, applies AI techniques for modeling, and eventually making intelligent decisions in applications. Thus it aims to seek for optimizing solutions to build automated and intelligent mobile applications to intelligently assist the users in their various daily activities.”. Several key modules, such as data collection, data processing, context and usage analysis, and building models, are involved in mobile data science, which are discussed briefly in our earlier paper [ 104 ]. In this paper, we mainly explore on AI-based modeling and its role in mobile apps in various application domains ranging from personalized services to healthcare services, which includes machine learning (ML) and deep learning (DL) methods, the concept of natural language processing (NLP), as well as knowledge representation, and rule-based expert systems (ES).
Overall, the outputs of mobile data science are typically mobile data products, which can be a data-driven AI-based model, potential mobile service and recommendation, or the corresponding intelligent mobile apps. In Section 6, we have discussed about AI-powered intelligent mobile apps in several application domains within the area of mobile data science.
Mobile security and privacy
Although we focus on intelligent apps from the perspective of artificial intelligence within the scope of our study discussed above, mobile security and privacy could be another part related to mobile data science in terms of data-driven security solutions. In the real world, most of the people including business people use smartphones not only to communicate but also to plan and organize their various kinds of daily works and also in their private life with family and friends. In most cases, both the business or personal information are stored on smartphones and people use such information when needed [ 105 , 106 ]. Thus, in addition to intelligent apps, mobile security and privacy is also important. Smartphones collect and analyze the sensitive information to which access must be controlled to protect the privacy of the user and the intellectual property of the organization or the company. Besides, there are several threats to mobile devices, including mobile malware, botnet, denial-of-service (DoS), eavesdropping, phishing, data breaches, etc. [ 106 – 108 ]. In terms of security analytics, in our earlier paper, Sarker et al. [ 10 ], we have discussed various types of security data and the effectiveness of the data-driven cybersecurity modeling based on artificial intelligence, particularly using machine learning techniques. Thus data-driven intelligent solutions through finding security insight could be effective to detect and mitigate such kind of mobile security threats.
AI-based modeling for Mobile services
As discussed earlier, mobile data science is data-focused, applies various artificial intelligence methods that eventually seek for intelligent decision making in mobile applications or services. In our analysis, we divide the artificial intelligence methods into several categories, such as basic machine learning and deep learning algorithms, natural language processing, knowledge representation and expert systems, within the scope of our study. These AI-based methods potentially can be used to make intelligent decisions in apps, which are discussed briefly in the following.
Machine learning modeling with Mobile data
Machine Learning (ML) including deep neural network learning is an important part of Artificial Intelligence (AI) which can empower mobile devices to learn, explore, and envisage outcomes automatically without user interference. For instance, machine learning algorithms can do the analysis of targeted user behavior patterns utilizing phone log data to make personalized suggestions as well as recommendations for mobile phone users. Typically, a machine learning model for building intelligent apps is a collection of target app-related data from relevant diverse sources, such as phone logs, sensors, or external sources, etc. and the chosen algorithms that work on that data in order to deduce the output.
To build a model utilizing collected data, supervised learning is performed when specific target classes are defined to reach from a certain set of inputs [ 13 ]. For instance, to classify or predict the future outcome, several popular algorithms such as Navies Bayes [ 109 ], Decision Trees [ 93 , 110 , 111 ], K-nearest neighbors [ 112 ], Support vector machines [ 73 ], Adaptive boosting [ 113 ], Logistic regression [ 114 ] etc. can be used. Such classification techniques are capable to build a prediction model ranging from predicting next usage to smartphone security, e.g., predicting mobile malware attack. Several feature engineering tasks, such as feature selection, extraction, etc., or context pre-modeling [ 78 ] can make the resultant predictive model more effective. On the other hand, in unsupervised learning, data is not labeled or classified, and it investigates similarity among unlabeled data [ 9 ]. Several clustering algorithms such as K-means [ 115 ], K-medoids [ 116 ], Single linkage [ 117 ], Complete linkage [ 118 ], BOTS [ 75 ] can be used for such modeling by taking into account certain similarity measures depending on the data characteristics. For instance, considering certain similarity in users’ preferences or behavioral activities, and to generate suggestions and recommendations accordingly, these algorithms can play a role to achieve the goal. Moreover, association rule learning techniques such as AIS [ 119 ], Apriori [ 120 ], FP-Tree [ 121 ], RARM [ 122 ], Eclat [ 123 ], ABC-RuleMiner [ 29 ] can be used for building rule-based machine learning model for the mobile phone users. In addition to these basic machine learning techniques, several deep neural learning methods such as recurrent neural network, long-short term memory, convolutional neural network, multilayer perceptron, etc. that are originated from an Artificial Neural Network (ANN) can be used in the learning process [ 9 , 13 ]. In these deep learning models, several hidden layers can be included to complete the overall process.
To understand and analyze the actual phenomena with mobile data, the above-discussed machine learning and deep learning techniques are useful to build AI-based modeling, depending on the target application and corresponding data characteristics. Thus the machine learning models and corresponding mobile apps that are close to the reality, are able to make data-driven intelligent decisions in apps and can behave according to users’ needs. Overall, the machine learning models can change the future of mobile applications and industry because of its learning capability from data. Therefore, machine learning methods including deep neural networks, on a global scale, is able to make mobile platforms more user-friendly, improve users’ experiences, and aid in building intelligent applications.
Natural language processing for Mobile content
Natural Language Processing (NLP) is an important branch of artificial intelligence that typically deals with the interaction between computers and humans using the natural language. One of the ultimate goals of NLP is to derive intelligence from unstructured data or content expressed in a natural language, such as English or Bengali. As each language has a unique set of grammar and syntax, and convention, NLP techniques can make it possible for computers to read text, hear speech, interpret it, measure sentiment or to mine opinions, and eventually determine which parts are important in an intelligent system [ 124 ]. For instance, to extract sentiments associated with positive, neutral, or negative polarities for specific subjects from a text document, an NLP-based methodology can be used. Thus, NLP can play a significant role to build intelligent apps when unstructured mobile content is available, and to be an important part within the scope of our study.
In recent days, a large amount of content read on mobile devices is text-based, such as emails, web pages, comments, blogs, or documents [ 15 ]. NLP techniques particularly, text mining extracts patterns and structured information from textual content that could make the apps smarter and intelligent, in which we are interested. For instance, browsing through large amounts of textual content on a small-screen mobile device may be tedious or time-consuming. In some cases, the important information might be easily overlooked due to the small screen of the devices. Thus, document summarization based on NLP might be the potential solution to provide a summary with high quality and minimal time.
Information extraction from mobile content could be another example of NLP based modeling. It typically identifies instances of a particular class of events, entities, or relationships in a natural language text and creates a structured representation of the discovered information [ 15 ]. For instance, this can be used to automatically find all the occurrences of a specific type of entity, such as ‘business’, and gather complementary information in the form of metadata around them. In addition to information extraction, NLP techniques can also be used when needed to develop the new mobile content. For instance, response generation while replying to an email, question answering, e.g., a company might need a mobile app that can answer questions about various products or services. Similarly, medical information extraction, personalized recommendation system through comments or text mining, context-aware chatbot, etc. are also included within the area. Thus, NLP techniques can play a significant role to build AI-based modeling depending on the target application and corresponding data type and characteristics.
Domain knowledge representation and Mobile expert system modeling
Due to the diversity of mobile users, contexts, increasing information, and variations in mobile computing platforms, mobile applications today are facing the challenges to provide the expected services. In artificial intelligence (AI), knowledge representation and expert system modeling is considered as another important part to minimize this issue, and to build knowledge-base intelligent systems.
Knowledge representation
In the real world, knowledge is considered as the information about a particular domain. It is typically a study of how the beliefs, intentions, and judgments of an intelligent agent can be expressed suitably for automated reasoning to solve problems. Thus, the main purpose of knowledge representation is modeling the intelligent behavior of an agent. It allows a machine to learn from that knowledge and behave intelligently like a human being. Instead of trying to understand from the bottom-up learning, its main goal is to understand the problems from the top-down, and to focus on what an associated agent needs to know in order to behave intelligently. Knowledge can be of several types:
- Declarative Knowledge: known as descriptive knowledge that represents to know about something, which includes concepts, facts, and objects, and expressed in a declarative sentence.
- Structural Knowledge: represents the basic knowledge to solve problems which describes the relationship between concepts and objects.
- Procedural Knowledge: known as imperative knowledge that is responsible for knowing how to do something which includes rules, strategies, procedures, etc.
- Meta Knowledge: represents knowledge about other types of knowledge.
- Heuristic Knowledge: represents knowledge of some experts in a field or subject that could be based on previous experiences.
To represent knowledge in Artificial Intelligence (AI), “Ontology” in general has become popular as a paradigm by providing a methodology for easier development of interoperable and reusable knowledge bases (KB). Ontologies can be used to capture, represent knowledge and describe concepts and the relationship that holds between those concepts. In general, ontology is an explicit specification of conceptualization and a formal way to define the semantics of knowledge and data. According to [ 125 ], formally, an ontology is represented as “{ O = C , R , I , H , A }, where { C = C 1 , C 2 , …, C n } represents a set of concepts, and { R = R 1 , R 2 , …, R m } represents a set of relations defined over the concepts. I represents a set of instances of concepts, and H represents a Directed AcyclicGraph (DAG) defined by the subsumption relation between concepts, and A represents a set of axioms bringing additional constraints on the ontology”. Let’s consider an inference rules in ontologies for deductive reasoning. A rule may exist which states “If a mobile user accepts phone calls from family at work and a phone call is from his mother, then the call has been answered.” Then a program could deduce from a social relationship ontology that the user answers her mother’s incoming call at work. Thus, particular domain ontologies can help for building an effective semantic mobile application. Moreover, ontologies capturing complex dependencies between concepts for a particular problem domain provide a flexible and expressive tool for modeling high-level concepts and relations among the given attributes, which allows both the system and the user to operate the same taxonomy and play an important role to build an expert system [ 125 ].
Mobile expert system modeling
A mobile expert system is an example of a knowledge-based system, which is broadly divided into two subsystems, such as the inference engine and the knowledge base, shown in Fig. 2 . The knowledge base typically represents facts and rules, while the inference engine applies the rules to the known facts to deduce new facts. The knowledge-base module is the core of this expert system as it consists of knowledge of the target mobile application domain as well as operational knowledge of apps’ decision rules. The user interface accepts the original facts and invokes the inference engine to activate the decision rules in the knowledge base. The system uses expert knowledge represented mainly as IF-THEN rules, to offer recommendations or making decisions in relevant application areas. For instance, by using the expert system model, the process of selecting the semantic outcome for mobile users becomes more appropriate according to expert recommendations. A rule consists of two parts: the IF part, called the antecedent (premise or condition) and the THEN part called the consequent (conclusion or action).

A structure of a mobile expert system modeling
The basic syntax of a rule is:
IF < antecedent > THEN < consequent > .
Such an IF-THEN rule-based expert system model can have the decision-making ability of a human expert in an intelligent system that is designed to solve complex problems as well through knowledge reasoning. To develop the knowledge base module, an ontology-based knowledge representation platform discussed earlier can play a major role to generate the conceptual rules. To provide a continuous supply of knowledge to a rule-based expert system, data mining, and machine learning techniques can be used. For instance, in our earlier approach “ABC-RuleMiner”, Sarker et al. [ 29 ], we have discovered a set of useful contextual rules for mobile phone users considering their behavioral patterns in the data. Domain experts having knowledge of business rules can then update and manage the rules according to the needs. Thus, the mobile expert systems can be used to make intelligent decisions in corresponding mobile applications.
AI-powered intelligent Mobile apps
An intelligent system typically tells what to do or what to conclude in different situations [ 126 ] and can act as an intelligent software agent. Thus, intelligent mobile apps are those applications that use AI-based modeling discussed above, in order to make intelligent decisions and to provide useful suggestions and recommendations. Based on this, the target mobile applications for various daily life services are outlined in the following subsections ranging from personalized to community services.
Personalized Mobile user experience
In the real world, people want their experience to be absolutely personalized these days. Thus, most of the mobile apps heavily rely on personalization to keep users engaged and interested. Users also now expect the applications to deliver unique experiences that may vary from user-to-user according to their own preferences. Thus understanding “user persona” is the key to creating personalized mobile applications that are based on users’ past experiences represented by users’ historical data. ML-based models can effectively discover useful insight from individuals’ phone data by taking into account users own behavioral activities, interactions, or preferences, and can be used to perform individual personalized services in various applications. For instance, an intelligent phone call interruption management system can be a real-life application based on the discovered rules, which handles the incoming phone calls automatically according to the behavior of an individual user [ 29 ]. Moreover, mobile notification management [ 58 , 59 ], apps usage prediction and management, etc. can be the real-life examples of personalized services for the end mobile phone users. Thus, the extracted insight from relevant contextual historical and real-time interaction data using ML-based models can be used to deliver rich and personalized experiences to the users in various day-to-day situations in their daily life activities. Similarly, a knowledge-based mobile expert system considering a set of context-aware IF-THEN rules, can also help to provide personalized services for individual users.
Mobile recommendation
Recommender systems are typically developed to overcome the problem of information overload by aiding users in the search for relevant information and helping them identify which items (e.g., media, product, or service) are worth viewing in detail. This task is also known as information filtering. According to [ 127 ], the most important feature of a recommender system is its ability to “guess” a user’s preferences and interests by analyzing the behavior of the user and/or the behavior of other users to generate personalized recommendations. In general, the traditional recommender systems mainly focus on recommending the most relevant items to users among a huge number of items [ 128 ]. However, mobile recommendation systems based on users’ contextual information such as temporal, spatial, or social etc. could be more interesting for the users [ 62 – 64 ]. The advanced mobile apps powered by predictive intelligent capabilities using ML-based models make recommend engines smart enough to analyze the user content preferences and cater to the appropriate content that the user is looking for. For instance, a mobile system generating shopping recommendations helps the user to find the most satisfying product by reducing search effort and information overload. Similarly, tourist guides [ 129 ], food or restaurant services [ 130 ], finding cheaper flights, accommodation, attractions, or leisure dissemination, etc. can be other real-life examples for the mobile phone users. Moreover, an NLP-based methodology can be a way to retrieve the best recommendation service based on public comments.
Mobile virtual assistance
An intelligent virtual assistant is also known as an intelligent personal assistant that is typically a software agent to perform tasks or services for an individual based on queries like commands or questions. The chatbot is sometimes used to refer to virtual assistants, which is a software application used to conduct an online chat conversation via text or text-to-speech. Several key advantages make the chatbots beneficial these days as they are able to provide 24*7 automated support, able to provide instant answers, good in handling customers or users, avoiding repetitive work, as well as save time and service cost. Intelligent mobile apps powered by AI are able to provide such services with higher accuracy. AI-based models including NLP and ML can be used to build such applications. Moreover, people are now typically spending more time on different messaging apps that are the platforms of communication and bots will be how their users access all sorts of services. Thus, chatbots can engage by answering basic questions in various services. For instance, online ordering, product suggestions, customer support, personal finance assistance, searching, and flight tracking, finding a restaurant, etc. A knowledge-based mobile expert system considering a set of IF-THEN rules, can also be applied to provide such service. Thus, different virtual assistant apps like voice assistants or chatbots offer interactive experiences to users, who are able to retrieve the necessary information effectively and efficiently according to their needs.
Internet of things (IoT) and smart cities
The Internet of Things (IoT) is typically a network of physical devices, and objects which utilize sensors, software, etc. for sending and receiving data. Smart cities use IoT devices as well to collect and analyze data, and become the most extensive application domain these days. In general, the smart city development is considered as a new way of thinking among cities, businesses, citizens, academia, industry people or others, who are the key stakeholders. As today’s smartphones are considered as one of the most important IoT devices [ 2 ], integrating mobile apps with IoT developments can dramatically improve the quality of human life. AI-based modeling in apps can provide relevant intelligent services in this domain, as well as can bring technology, government, and different layers of society together for the betterment of human life. For instance, machine learning-based modeling utilizing sensor data collected from parking places, or traffic signals, can be used for a better city planning for the governments. Similarly, a knowledge-based mobile expert system considering a set of IF-THEN rules, can help to make context-aware and timely decisions. Overall, AI-based modeling can assist the users in our most common daily life issues, such as questions, suggestions, general feedback, and reporting in various smart city services including smart governance, smart home, education, communication, transportation, retail, agriculture, health care, enterprise and many more.
Mobile business
Smart mobile apps have the potential to increase the operational excellence in the business-to-business as well as business-to-customer sectors. The new availability and advancement of AI and machine learning are causing a revolutionary shift in business and is considered as the new digital frontier for enterprises. Since, almost every organization deal with customer service, the businesses people think about intelligent interactions within mobile applications these days according to consumer demands. Businesses can leverage the data that are collected from various sources such as point-of-sale machines, online traffic, mobile devices, etc. to analyze and strategically improve the user experience. AI techniques can find trends from data and adjust the apps themselves to create more meaningful and context-rich opportunities to engage users. For instance, machine learning algorithms are capable to understand the customer behavior, interests, and provide them with more relevant product recommendations based on purchase history, fraud identification with credit cards, and visual search. By taking into account context-awareness, it can also empower businesses with prominent features, such as delivering precise location-based suggestions. Moreover, an NLP-based methodology of sentiment evaluation such as positive, neutral, or negetive sentiment (also known as opinion mining) on business data, e.g., review comments, can retrieve the best and perfect suggestions and product recommendations in terms of quality and quantity for the customers. AI positively impacts customer behavior by incorporating the chatbots as well in a mobile application, which may reduce the repetitive tasks and optimize manpower utilization. Similarly, knowledge-based mobile expert system considering a set of business IF-THEN rules, can make intelligent decisions. Overall, AI mobile applications in the business domain help in expanding businesses, introducing new products or services, identifying customer interests, and maintaining a prominent position in the global market.
Mobile healthcare and medicine
Intelligent mobile healthcare applications are bringing better opportunities for both the patients, medical practitioners, or related organizations through simplifying their physical interactions. These apps can provide opportunities to several health-related services such as medical diagnosis, medicine recommendation including e-prescription, suggesting primary precautions, remote health monitoring, or effectively patient management in the hospital. For assessing and strengthening health facilities, or building health management information systems (HMIS), various kinds of health data can be collecting from multiple sources on a wide variety of health topics to analyze [ 131 ]. With the help of AI methods including ML-based models, intelligent health services can be provided. Thus it may reduce the expense and time of the patients and clinics, as they offer customized medicines and drugs as well as give preventive measures through continuous information accumulation. Moreover, AI-powered mobile applications could also be applicable to find the best nearest doctor, to book a consultation, to keep reminders of medication, getting a basic knowledge of each medication, and more. Mobile healthcare app is also able to help doctors with remaining updates with real-time status of consultations, assigning duties to staff, ensuring the availability of equipment, maintaining a proper temperature for medicines, and more. In addition, the healthcare virtual assistant services like chatbots can be used to provide basic healthcare service as well, as these online programs can assist patients in many ways, such as scheduling appointments, answering common questions, aiding in the payment process, and even providing basic virtual diagnostics. Overall, AI-modeling based mobile healthcare services may create a new endeavor for all citizens in a country including the rural people of low-income countries.
The novel coronavirus COVID-19
Coronavirus disease (COVID-19) is an infectious disease caused by a newly discovered coronavirus [ 131 ]. COVID-19 apps typically are known as the mobile software applications that use digital contact tracing in response to the COVID-19 pandemic, i.e. the process of identifying persons (“contacts”) who may have been in contact with an infected person. According to the World Health Organization (WHO) [ 131 ], most people infected with the COVID-19 virus will experience mild to moderate respiratory illness and recover without requiring special treatment. Thus, in order to keep this infectious disease in control, “contact tracing” is an important factor. Smartphone apps are playing a big role in the response to the COVID-19 pandemic. These apps are being used to track infected people, social distancing, detecting COVID-19 symptoms, self-quarantine guidelines, the latest communication to the citizens, and ease the burden on healthcare staff. Thus, mobile apps are considered as an effective control strategy against the spread of COVID-19 or similar future pandemics, considering the patient and social sensing data. An intelligent framework and mobile application design will not only strengthen the fight against ongoing COVID-19 challenges based on the collected data by mobile phones, but also against similar disasters in a post-COVID world.
In addition to these application areas, AI-based models in mobile applications can also be applicable to several other domains, such as financial, manufacturing, smart robotics, security and privacy, and many more. Thus, the impact of AI-models in mobile app development and user experience is significant in these days and can be considered as next-generation mobile learning.
Research issues and future directions
With the rapid development of smartphones, Internet-of-Things (IoT), and AI technologies, the most fundamental challenge is to explore the relevant data collected from diverse sources and to extract useful insights for future actions. Thus, in this section, we highlight and analyze the main challenges and research issues in the scope of our study. In the following, the issues that we identified and corresponding future directions are discussed briefly.
- According to our study in this paper, source datasets are the primary component to work in the area of mobile data science. Thus, collecting real-world data such as categorical, numerical, or textual relevant to a particular application is the first step for building an intelligent smartphone apps, which may vary from service to service. For instance, to manage mobile interruptions, the relevant contextual information and an individual’s behavioral data is needed to be analyzed [ 4 ]. Similarly, for smart healthcare services, patient data and corresponding contextual information might be useful. Thus, to facilitate the extraction of reliable insight from the data using AI techniques and to use the knowledge in context-aware applications, integrating and effective management of mobile data is important. The reason is that AI methods particularly machine learning techniques highly impact on data [ 9 ]. Therefore, establishing a large number of recent datasets from diverse sources and to integrate and manage such information for effective data analysis is needed, which could be one of the major challenges to work in the area of mobile data science and data-driven intelligent applications.
- The next challenge is an effective modeling of mobile users and their activities from the relevant data. The main goal of mobile user modeling is the customization and adaptation of systems to the user’s specific needs. The system needs to output the ‘right’ outcome at the ‘right’ time or contexts in the ‘right’ way [ 4 ]. Thus, several aspects such as context-dependency, individual user behavior, and their preferences in different contexts are needed to take into account for an effective user modeling and to build corresponding intelligent apps. The reason is that usage patterns of mobile phones vary greatly between individuals behaving differently in different contexts. Thus considering various contexts, such as temporal, spatial, social, etc. and their effective modeling based on these contexts are important to build an intelligent app [ 93 ]. For this purpose, data preparation, discretization of contexts, and discovery of useful insights are the key issues [ 4 ]. Moreover, the concept of RecencyMiner [ 76 ] can be more effective because of considering the recent pattern-based insights. Therefore, effectively modeling mobile users considering these aspects, could be another research issue in the area of mobile data science and intelligent applications.
- The context-sensitive features in mobile data and their patterns are of high interest to be discovered and analyzed to make context-aware intelligent decisions for a particular application in a pervasive computing environment. The traditional analytical techniques including data science and machine learning may not be applicable to make real-time decisions for analyzing smartphone data, because of a large number of data processing that may reduce the performance of mobile phones. For instance, the association rule mining technique [ 120 ] may discover a large number of redundant rules that become useless and make the decision-making process complex and ineffective [ 29 ]. Such traditional techniques may not be applicable for analyzing smartphone data. Thus, a deeper understanding is necessary on the strengths and weaknesses of state-of-the-art big data processing and analytics systems to realize large-scale context-awareness and to build a smart context-aware model. Therefore effectively building a data-driven context-aware model for intelligent decision-making on smartphones, could be another research issue in the area of mobile data science and intelligent applications.
- Real-life mobile phone datasets may contain many features or high-dimensions of contexts, which may cause several issues such as increases model complexity, may arise over-fitting problem, and consequently decreases the outcome of the resultant AI-based model [ 77 ]. The reason is that the performance of AI methods particularly machine learning algorithms heavily depends on the choice of features or data representation. Having irrelevant features or contextual information in the data makes the model learn based on irrelevant features that consequently decrease the accuracy of the models [ 132 ]. Thus the challenge is to effectively select the relevant and important features or extracting new features that are known as feature optimization. In the area of AI, particularly data science and machine learning, feature optimization problem is considered as an important pre-processing step that helps to build an effective and simplified model and consequently improves the performance of the learning algorithms by removing the redundant and irrelevant features [ 111 ]. Therefore, feature optimization could be a significant research issue in the area of mobile data science and intelligent applications.
- The next challenge is the extraction of the relevant and accurate information from the unstructured or semi-structured data on mobile phones. A large amount of content such as emails, web pages, or documents is read on these devices frequently that is text-based [ 15 ]. Thus the problem of information overload arises due to the small screen of the devices rather than the desktop computer. Therefore effectively mining the contents or texts considering these aspects, could be another research issue in the area of mobile data science and intelligent applications. Natural language processing (NLP) techniques can help to make such text-based apps smarter, by automatically analyzing the meaning of content and taking appropriate actions on behalf of their users. Due to the devices’ limited input and processing capabilities rather than desktop computers, it is then needed to develop novel approaches that can bring NLP power to smartphones. Several NLP tasks such as automatic summarization, information extraction, or new content development, etc. could be useful to minimize the issue.
- Mobile expert system uses expert knowledge represented mainly as IF-THEN rules, to offer recommendations or making decisions in relevant application areas. However, the development of large-scale rule-based systems may face numerous challenges. For instance, the reasoning process can be very complex, and designing of such systems becomes hard to manage [ 133 ]. There is still a lack of lightweight rule-based inference engines that will allow for reasoning on mobile devices [ 133 ]. Thus a set of concise and effective rules will be beneficial in terms of outcome and simplicity for such a rule-based expert system for mobile devices. Moreover, ontologies [ 125 ] capturing complex dependencies between concepts for a particular problem domain provides a flexible and expressive tool for modeling high-level concepts and relations among the given attributes, which allows both the system and the user to operate the same taxonomy and play an important role to build an expert system. This is where the ontological modeling and reasoning is useful. Thus, an effective design of ontology, or knowledge representation model for the respective problem domain could be another research issue.
- The mobility of computing devices, e.g., smartphones, applications, and users leads to highly dynamic computing environments. Unlike desktop applications, which rely on a carefully configured, and largely static set of resources, pervasive computing applications are subjected to changes in available resources such as network connectivity, user contexts, etc. Moreover, they are frequently required to cooperate spontaneously and opportunistically with previous unknown software services to accomplish tasks on behalf of users. Thus, pervasive computing software must be highly adaptive and flexible. As an example, an application may need to modify it’s style of output following a transition from an office environment to a moving vehicle, to be less intrusive [ 4 ]. Thus to effectively adapt to the changing environment according to users’ needs is important, which is important in the area of mobile data science and intelligent applications. Context-awareness represents the ability of mobile devices to sense their physical environment and adapt their behavior accordingly, incorporating this property in the applications could be a potential solution to overcome this issue.
Although several research efforts have been directed towards intelligent mobile apps, discussed throughout the paper, this paper presents a comprehensive view of mobile data science and intelligent apps in terms of concepts and AI-based modeling. For this, we have conducted a literature review to understand the contexts, mobile data, context-aware computing, data science, intelligent apps characteristics, and different types of mobile systems and services, as well as the used techniques, related to mobile applications. Based on our discussion on existing work, several research issues related to mobile datasets, user modeling, intelligent decision making, feature optimization, mobile text mining based on NLP, mobile expert system, and context-aware adaptation, etc. are identified that require further research attention in the domain of mobile data science and intelligent apps.
The scope of mobile data science is broad. Several data-driven tasks, such as personalized user experience, mobile recommendations, virtual assistant, mobile business, and even mobile healthcare system including the COVID-19 smartphone app, etc. can be considered as the scope of mobile data science. Traditionally mobile app development mostly focused on knowledge that is not automatically discovered [ 47 , 66 ]. Taking the advantage of large amounts of data with rich information, AI is expected to help with studying much more complicated yet much closer to real-life applications, which then leads to better decision making in relevant applications. Considering the volume of collected data and the features, one can decide whether the standalone or cloud-based application is more suitable to provide the target service. Thus, the output of AI-based modeling can be used in many application areas such as mobile analytics, context-aware computing, pervasive computing, health analytics, smart cities, as well as the Internet of things (IoT). Moreover, intelligent data-driven solutions could also be effective in AI-based mobile security and privacy, where AI works with huge volumes of security event data to extract the useful insights using machine learning techniques [ 10 ].
Although the intelligent apps discussed in this paper can play a significant role in the betterment of human life in different directions, several dependencies may pose additional challenges, such as the availability of network and the data transfer speeds or the battery life of mobile devices. Moreover, privacy and security issues may become another challenge while considering the data collection and processing over the cloud or within the device. Taking the advantages of these issues considering the application type and target goal, we believe this analysis and guidelines will be helpful for both the researchers and application developers to work in the area of mobile data science and intelligent apps.
In this paper, we have studied on mobile data science and reviewed the motivation of using AI in mobile apps to make it intelligent. We aimed to provide an overview of how artificial intelligence can be used to design and develop data-driven intelligent mobile applications for the betterment of human life. For this, we have presented an AI-based modeling that includes machine learning and deep learning methods, the concept of natural language processing, as well as knowledge representation and expert systems. Such AI-based modeling can be used to build intelligent mobile applications ranging from personalized recommendations to healthcare services including COVID-19 pandemic management, that are discussed briefly in this paper. A successful intelligent mobile system must possess the relevant AI-based modeling depending on the data characteristics. The sophisticated algorithms then need to be trained through collected data and knowledge related to the target application before the system can assist the users with suggestions and decision making. We have concluded with a discussion about various research issues and future directions relevant to our analysis in the area of mobile data science and intelligent apps, that can help the researchers to do future research in the identified directions.
Biographies
received his PhD under the department of Computer Science and Software Engineering from Swinburne University of Technology, Melbourne, Australia in 2018. Before that, he completed his Master of Science in Computer Science and Engineering, and Bachelor of Science in Computer Science and Engineering from Chittagong University of Engineering and Technology, Bangladesh. His research interests include Data Science, Machine Learning and AI, IoT Analytics, Context-Aware Smart Computing, Cybersecurity Analytics and Intelligence. He has published a number of peer-reviewed journals and conferences in top venues, such as Journals (The Computer Journal - Oxford, Journal of Network and Computer Applications - Elsevier, Internet of Things - Elsevier, Journal of Big Data –Springer, Mobile Networks and Applications – Springer, Sensors - MDPI, and soon) and Conferences (IEEE DSAA, IEEE Percom, ACM Ubicomp, ACM Mobiquitous, Springer PAKDD and so on). He is also a member of ACM and IEEE.

Mohammed M. Hoque
is a Distinguish Professor of the Department of Computer Science & Engineering (CSE). He received Ph. D from the Dept. of Information and Computer Sciences, Saitama University, Japan in 2012. He servedas a TPC Chair (IEEE r10 HTC 2017, ECCE 2019), TPC Co-chair (ICISET 2018, IEEETenSymp 2020), Publication chair (IEEE WIECON-ECE 2018/2019, IEEE TenSymp 2020) and TPC members in several international conferences. Dr. Hoque was the Award Coordinator (2016-17), Conference Coordinator (2017-18) of IEEE BangladeshSection and now he is serving as Vice-chair (Technical) (2018-20) in Bangladesh section. Moreover, he is serving as Vice-chair (Activity) (2018-19), Award Coordinator (2017-18) of IEEE Computer Society Bangladesh Chapter and Educational Activity Coordinator (2017-18), IEEE RAS, Bangladesh Chapter respectively. He published more than 115 publications in several International Journals, Book Chapters and Conferences. His research interests include humanrobot/computer interaction, computer vision, and natural language processing.Dr. Hoque is a senior member of IEEE, IEEE Computer Society, IEEE Robotics and Automation Society, IEEE Women in Engineering, IEEE Signal Processing Society and Fellow of Institute of Engineers, Bangladesh.

Md Kafil Uddin
received the M. Sc.degree in Computer Engineering from the Pusan National University, Pusan, Korea, in 2009. Then he served as a lecturer in Najran University, Najran, Saudi Arabia for more than 7 years. Currently, he is a Ph.D. student at the Swinburne University of Technology, VIC, Australia. His current research interests include mobile app repository mining, review mining, feature processing and feature recommendation.

received the M.S. degree in Information Technology from Monash University, in 2016. Currently, he is a Ph.D. candidate in Software Engineering at RMIT University. In 2012, he joined Taibah University as a teacher assistant. Healso was granted a scholarship from Taibah University to complete his M.S. and Ph.D. degrees. His research interests include activities related to requirements engineering, software development, context-awareness, and human factors.

Publisher’s Note
Springer Nature remains neutral with regard to jurisdictional claims in published maps and institutional affiliations.
Grab your spot at the free arXiv Accessibility Forum
Help | Advanced Search
Computer Science > Information Theory
Title: a survey on mobile edge computing: the communication perspective.
Abstract: Driven by the visions of Internet of Things and 5G communications, recent years have seen a paradigm shift in mobile computing, from the centralized Mobile Cloud Computing towards Mobile Edge Computing (MEC). The main feature of MEC is to push mobile computing, network control and storage to the network edges (e.g., base stations and access points) so as to enable computation-intensive and latency-critical applications at the resource-limited mobile devices. MEC promises dramatic reduction in latency and mobile energy consumption, tackling the key challenges for materializing 5G vision. The promised gains of MEC have motivated extensive efforts in both academia and industry on developing the technology. A main thrust of MEC research is to seamlessly merge the two disciplines of wireless communications and mobile computing, resulting in a wide-range of new designs ranging from techniques for computation offloading to network architectures. This paper provides a comprehensive survey of the state-of-the-art MEC research with a focus on joint radio-and-computational resource management. We also present a research outlook consisting of a set of promising directions for MEC research, including MEC system deployment, cache-enabled MEC, mobility management for MEC, green MEC, as well as privacy-aware MEC. Advancements in these directions will facilitate the transformation of MEC from theory to practice. Finally, we introduce recent standardization efforts on MEC as well as some typical MEC application scenarios.
Comments: | Updated version with a new title of the paper "Mobile Edge Computing: Survey and Research Outlook", submitted to IEEE Commun. Surveys Tuts |
Subjects: | Information Theory (cs.IT) |
Cite as: | [cs.IT] |
(or [cs.IT] for this version) | |
Focus to learn more arXiv-issued DOI via DataCite |
Submission history
Access paper:.
- Other Formats
References & Citations
- Google Scholar
- Semantic Scholar
DBLP - CS Bibliography
Bibtex formatted citation.

Bibliographic and Citation Tools
Code, data and media associated with this article, recommenders and search tools.
- Institution
arXivLabs: experimental projects with community collaborators
arXivLabs is a framework that allows collaborators to develop and share new arXiv features directly on our website.
Both individuals and organizations that work with arXivLabs have embraced and accepted our values of openness, community, excellence, and user data privacy. arXiv is committed to these values and only works with partners that adhere to them.
Have an idea for a project that will add value for arXiv's community? Learn more about arXivLabs .
Information
- Author Services
Initiatives
You are accessing a machine-readable page. In order to be human-readable, please install an RSS reader.
All articles published by MDPI are made immediately available worldwide under an open access license. No special permission is required to reuse all or part of the article published by MDPI, including figures and tables. For articles published under an open access Creative Common CC BY license, any part of the article may be reused without permission provided that the original article is clearly cited. For more information, please refer to https://www.mdpi.com/openaccess .
Feature papers represent the most advanced research with significant potential for high impact in the field. A Feature Paper should be a substantial original Article that involves several techniques or approaches, provides an outlook for future research directions and describes possible research applications.
Feature papers are submitted upon individual invitation or recommendation by the scientific editors and must receive positive feedback from the reviewers.
Editor’s Choice articles are based on recommendations by the scientific editors of MDPI journals from around the world. Editors select a small number of articles recently published in the journal that they believe will be particularly interesting to readers, or important in the respective research area. The aim is to provide a snapshot of some of the most exciting work published in the various research areas of the journal.
Original Submission Date Received: .
- Active Journals
- Find a Journal
- Proceedings Series
- For Authors
- For Reviewers
- For Editors
- For Librarians
- For Publishers
- For Societies
- For Conference Organizers
- Open Access Policy
- Institutional Open Access Program
- Special Issues Guidelines
- Editorial Process
- Research and Publication Ethics
- Article Processing Charges
- Testimonials
- Preprints.org
- SciProfiles
- Encyclopedia
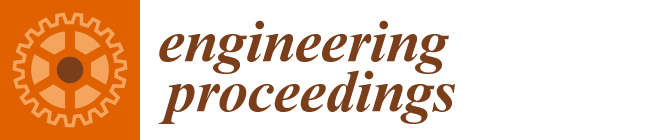
Article Menu
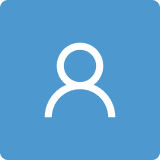
- Subscribe SciFeed
- Google Scholar
- on Google Scholar
- Table of Contents
Find support for a specific problem in the support section of our website.
Please let us know what you think of our products and services.
Visit our dedicated information section to learn more about MDPI.
JSmol Viewer
Mobile cloud computing: a survey on current security trends and future directions †.
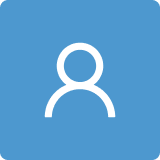
1. Introduction
2. literature review, 3. methodology, 3.1. mobile cloud computing (mcc), 3.2. the architecture of mcc.
- Access point: with the help of WI-FI devices
- Mobile network service: with the help of mobile communication networks like 3G, 4G, and 5G.
3.3. Advantages of MCC
3.4. limitations of mcc, 3.5. challenges of mcc, 4. discussion, 4.1. work on mcc after covid-19, 4.2. 5g impact on mcc, 6. future work and conclusions, author contributions, institutional review board statement, informed consent statement, data availability statement, conflicts of interest.
- Jegadeesan, S.; Azees, M.; Kumar, P.M.; Manogaran, G.; Chilamkurti, N.; Varatharajan, R.; Hsu, C.H. An efficient anonymous mutual authentication technique for providing secure communication in mobile cloud computing for smart city applications. Sustain. Cities Soc. 2019 , 49 , 101522. [ Google Scholar ] [ CrossRef ]
- Almusaylim, Z.A.; Jhanjhi, N.Z. Comprehensive review: Privacy protection of user in location-aware services of mobile cloud computing. Wirel. Pers. Commun. 2020 , 111 , 541–564. [ Google Scholar ] [ CrossRef ]
- Shabbir, M.; Shabbir, A.; Iwendi, C.; Javed, A.R.; Rizwan, M.; Herencsar, N.; Lin, J.C.W. Enhancing security of health information using modular encryption standard in mobile cloud computing. IEEE Access 2021 , 9 , 8820–8834. [ Google Scholar ] [ CrossRef ]
- Oh, E.N.; Baharon, M.R.; Yassin, S.M.W.M.S.M.M.; Idris, A.; MacDermott, A. Preserving Data Privacy in Mobile Cloud Computing using Enhanced Homomorphic Encryption Scheme. J. Phys. Conf. Ser. 2022 , 2319 , 012024. [ Google Scholar ] [ CrossRef ]
- Shamshirband, S.; Fathi, M.; Chronopoulos, A.T.; Montieri, A.; Palumbo, F.; Pescapè, A. Computational intelligence intrusion detection techniques in mobile cloud computing environments: Review, taxonomy, and open research issues. J. Inf. Secur. Appl. 2020 , 55 , 102582. [ Google Scholar ] [ CrossRef ]
- Akbar, A.; Lewis, P.R.; Wanner, E. A self-aware and scalable solution for efficient mobile-cloud hybrid robotics. Front. Robot. AI 2020 , 7 , 102. [ Google Scholar ] [ CrossRef ] [ PubMed ]
- AlAhmad, A.S.; Kahtan, H.; Alzoubi, Y.I.; Ali, O.; Jaradat, A. Mobile cloud computing models security issues: A systematic review. J. Netw. Comput. Appl. 2021 , 190 , 103152. [ Google Scholar ] [ CrossRef ]
- Maray, M.; Shuja, J. Computation offloading in mobile cloud computing and mobile edge computing: Survey, taxonomy, and open issues. Mob. Inf. Syst. 2022 , 2022 , 1121822. [ Google Scholar ] [ CrossRef ]
- Abidin, S.; Swami, A.; Ramirez-Asís, E.; Alvarado-Tolentino, J.; Maurya, R.K.; Hussain, N. Quantum cryptography technique: A way to improve security challenges in mobile cloud computing (MCC). Mater. Today Proc. 2022 , 51 , 508–514. [ Google Scholar ] [ CrossRef ]
- Ali, A.; Iqbal, M.M. A cost and energy efficient task scheduling technique to offload microservices based applications in mobile cloud computing. IEEE Access 2022 , 10 , 46633–46651. [ Google Scholar ] [ CrossRef ]
- Sheth, H.S.K.; Tyagi, A.K. Mobile cloud computing: Issues, applications and scope in COVID-19. In Intelligent Systems Design and Applications ; Springer International Publishing: Cham, Switzerland, 2022. [ Google Scholar ]
- Irshad, A.; Chaudhry, S.A.; Shafiq, M.; Usman, M.; Asif, M.; Ghani, A. A provable and secure mobile user authentication scheme for mobile cloud computing services. Int. J. Commun. Syst. 2019 , 32 , e3980. [ Google Scholar ] [ CrossRef ]
- Elmeadawy, S.; Shubair, R.M. 6G wireless communications: Future technologies and research challenges. In Proceedings of the 2019 International Conference on Electrical and Computing Technologies and Applications (ICECTA), Ras Al Khaimah, United Arab Emirates, 19–21 November 2019. [ Google Scholar ]
Click here to enlarge figure
Ref. | Technique | Security | Application | Architecture | Algorithms | Data Storage | Methodology Type |
---|---|---|---|---|---|---|---|
[ ] | ✓ | ✓ | ✓ | n/a | ✓ | n/a | Trusted third party (TTP) |
[ ] | ✓ | ✓ | ✓ | ✓ | ✓ | n/a | Homomorphic encryption technique |
[ ] | ✓ | ✓ | ✓ | ✓ | ✓ | ✓ | Enciphering scheme, Modular Encryption Standard |
[ ] | ✓ | ✓ | n/a | ✓ | ✓ | ✓ | Homomorphic cryptosystem technique. |
[ ] | ✓ | ✓ | ✓ | ✓ | n/a | ✓ | CI-based IDs |
[ ] | ✓ | n/a | ✓ | n/a | ✓ | n/a | Multi-objective optimization (MOO) algorithm |
[ ] | ✓ | ✓ | ✓ | n/a | n/a | n/a | Client-to-client authentication, RQ models |
[ ] | ✓ | ✓ | ✓ | ✓ | ✓ | n/a | Computation offloading, Mobile edge computing (MEC) |
[ ] | ✓ | ✓ | ✓ | ✓ | ✓ | n/a | Quantum cryptography application |
[ ] | ✓ | n/a | ✓ | ✓ | ✓ | ✓ | Task scheduling and microservice-based computational offloading (TSMCO) |
The statements, opinions and data contained in all publications are solely those of the individual author(s) and contributor(s) and not of MDPI and/or the editor(s). MDPI and/or the editor(s) disclaim responsibility for any injury to people or property resulting from any ideas, methods, instructions or products referred to in the content. |
Share and Cite
Sheikh, B.; Butt, A.; Hanif, J. Mobile Cloud Computing: A Survey on Current Security Trends and Future Directions. Eng. Proc. 2023 , 32 , 22. https://doi.org/10.3390/engproc2023032022
Sheikh B, Butt A, Hanif J. Mobile Cloud Computing: A Survey on Current Security Trends and Future Directions. Engineering Proceedings . 2023; 32(1):22. https://doi.org/10.3390/engproc2023032022
Sheikh, Bisma, Ayesha Butt, and Javeria Hanif. 2023. "Mobile Cloud Computing: A Survey on Current Security Trends and Future Directions" Engineering Proceedings 32, no. 1: 22. https://doi.org/10.3390/engproc2023032022

Article Metrics
Article access statistics, further information, mdpi initiatives, follow mdpi.
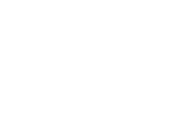
Subscribe to receive issue release notifications and newsletters from MDPI journals
Academia.edu no longer supports Internet Explorer.
To browse Academia.edu and the wider internet faster and more securely, please take a few seconds to upgrade your browser .
Enter the email address you signed up with and we'll email you a reset link.
- We're Hiring!
- Help Center
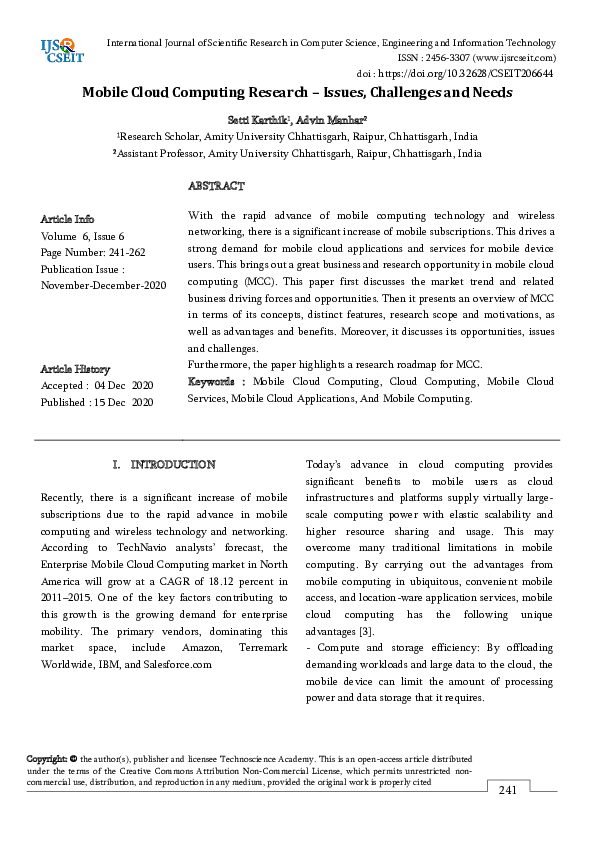
Mobile Cloud Computing Research – Issues, Challenges and Needs

2020, International Journal of Scientific Research in Computer Science, Engineering and Information Technology
With the rapid advance of mobile computing technology and wireless networking, there is a significant increase of mobile subscriptions. This drives a strong demand for mobile cloud applications and services for mobile device users. This brings out a great business and research opportunity in mobile cloud computing (MCC). This paper first discusses the market trend and related business driving forces and opportunities. Then it presents an overview of MCC in terms of its concepts, distinct features, research scope and motivations, as well as advantages and benefits. Moreover, it discusses its opportunities, issues and challenges. Furthermore, the paper highlights a research roadmap for MCC.
RELATED PAPERS
HOANG DINH THAI , Dusit Niyato
International Journal of Information Systems and Computer Technologies
Rizwan Amin
Dipayan Dev
Dr. Amit Sinhal
Esam Aloufi
Ibrahim H AlShourbaji
Journal of Computer Networks and Communications
Ahmed Aliyu
2013 9th International Wireless Communications and Mobile Computing Conference (IWCMC)
Samson Ooko
Mobile Networks and Applications
Nalini Venkatasubramanian
Daniela Villafañe
IAEME PUBLICATION
IAEME Publication
M.Rajendra Prasad , R. Lakshman Naik , Dr. Manjula Bairam
International Journal of Computer Applications
Ali Newaz Bahar
IEEE COMSOC Multimedia Communications …
Rerohit Kumar
International Journal of Engineering Research and Technology (IJERT)
IJERT Journal
Academic journal of Nawroz University
Amira Bibo Sallow
INFORMATION TECHNOLOGY IN INDUSTRY
Prasanthi Gottumukkala
International Journal of Scientific Research
Satish Kumar
RELATED TOPICS
- We're Hiring!
- Help Center
- Find new research papers in:
- Health Sciences
- Earth Sciences
- Cognitive Science
- Mathematics
- Computer Science
- Academia ©2024
IEEE Account
- Change Username/Password
- Update Address
Purchase Details
- Payment Options
- Order History
- View Purchased Documents
Profile Information
- Communications Preferences
- Profession and Education
- Technical Interests
- US & Canada: +1 800 678 4333
- Worldwide: +1 732 981 0060
- Contact & Support
- About IEEE Xplore
- Accessibility
- Terms of Use
- Nondiscrimination Policy
- Privacy & Opting Out of Cookies
A not-for-profit organization, IEEE is the world's largest technical professional organization dedicated to advancing technology for the benefit of humanity. © Copyright 2024 IEEE - All rights reserved. Use of this web site signifies your agreement to the terms and conditions.
Biostatistics Graduate Program
Siwei zhang is first author of jamia paper.
Posted by duthip1 on Tuesday, August 13, 2024 in News .
Congratulations to PhD candidate Siwei Zhang , alumnus Nicholas Strayer (PhD 2020; now at Posit), senior biostatistician Yajing Li , and assistant professor Yaomin Xu on the publication of “ PheMIME: an interactive web app and knowledge base for phenome-wide, multi-institutional multimorbidity analysis ” in the Journal of the American Medical Informatics Association on August 10. As stated in the abstract, “PheMIME provides an extensive multimorbidity knowledge base that consolidates data from three EHR systems, and it is a novel interactive tool designed to analyze and visualize multimorbidities across multiple EHR datasets. It stands out as the first of its kind to offer extensive multimorbidity knowledge integration with substantial support for efficient online analysis and interactive visualization.” Collaborators on the paper include members of Vanderbilt’s Division of Genetic Medicine, Department of Biomedical Informatics, Department of Urology, Department of Obstetrics and Gynecology, Division of Hematology and Oncology, VICTR , Department of Pharmacology, Center for Drug Safety and Immunology, and Department of Psychiatry and Behavioral Sciences, as well as colleagues at Massachusetts General Hospital, North Carolina State University, Murdoch University (Australia), and the Broad Institute. Dr. Xu is corresponding author.
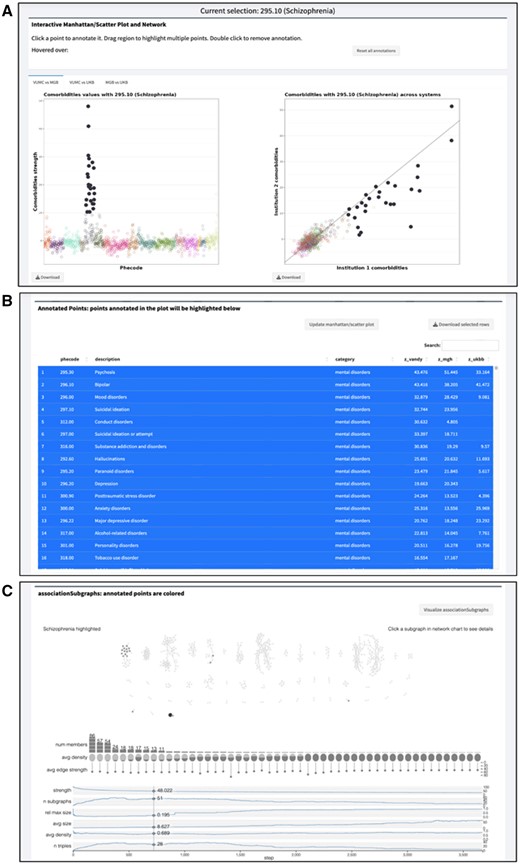
Tags: cloud computing , EHR , methods , network analysis , R , schizophrenia , Shiny
Leave a Response
You must be logged in to post a comment
Advertisement
Mobile learning: research context, methodologies and future works towards middle-aged adults – a systematic literature review
- Track 5: Multimedia and Education
- Published: 20 August 2022
Cite this article
- Syahida Mohtar ORCID: orcid.org/0000-0002-4462-8890 1 ,
- Nazean Jomhari 1 ,
- Mumtaz Begum Mustafa 1 &
- Zulkifli Mohd Yusoff 2
6220 Accesses
5 Citations
Explore all metrics
Over the past several years, mobile learning concepts have changed the way people perceived on mobile devices and technology in the learning environment. In earlier days, mobile devices were used mainly for communication purposes. Later, with many new advanced features of mobile devices, they have opened the opportunity for individuals to use them as mediated technology in learning. The traditional way of teaching and learning has shifted into a new learning dimension, where an individual can execute learning and teaching everywhere and anytime. Mobile learning has encouraged lifelong learning, in which everyone can have the opportunity to use mobile learning applications to gain knowledge. However, many of the previous studies on mobile learning have focused on the young and older adults, and less intention on middle-aged adults. In this research, it is targeted for the middle-aged adults which are described as those who are between the ages of 40 to 60. Middle-aged adults typically lead very active lives while at the same time are also very engaged in self-development programs aimed at enhancing their spiritual, emotional, and physical well-being. In this paper, we investigate the methodology used by researchers based on the research context namely, acceptance, adoption, effectiveness, impact, intention of use, readiness, and usability of mobile learning. The research context was coded to the identified methodologies found in the literature. This will help one to understand how mobile learning can be effectively implemented for middle-aged adults in future work. A systematic review was performed using EBSCO Discovery Service, Science Direct, Google Scholar, Scopus, IEEE and ACM databases to identify articles related to mobile learning adoption. A total of 65 journal articles were selected from the years 2016 to 2021 based on Kitchenham systematic review methodology. The result shows there is a need to strengthen research in the field of mobile learning with middle-aged adults.
Explore related subjects
- Artificial Intelligence
Avoid common mistakes on your manuscript.
1 Introduction
Adulthood can be categorized into early, middle and late adulthood. Middle-aged adults come between the ages of 40 to 60, in other words is when one is in between the younger and older generations [ 42 , 62 ]. This stage of age, notably is the age period that Hall [ 31 ] referred to as aging, where the signs of cognitive and physical ageing start to be noticeable, from the age of 40 and rapidly increase after the age of 65 [ 6 , 54 ]. According to Yaffe and Stewart [ 94 ], a large part of adult life is made up of the mid-life period. This has been associated with many descriptive terms: mid-life syndrome, mid-life crisis, middlescence, empty nest syndrome, second adolescence, second honeymoon, age of fulfillment, and menopause. Aging population contributes to healthcare issues, not only amongst the older adults but towards middle-aged adults too. As mentioned earlier, the healthcare issues amongst the middle-aged adults are related to the decline in physical abilities, relational, and psychological capacities. For example, women in their middle age experience menopause and perceived personality change, which lead to severe depression, physical, and emotional problems [ 80 ]. According to Yaffe and Stewart [ 94 ], the most frequently identified events or concerns among middle-aged adults were: increased personal concern for health, death of a friend or relative, change in wage/salary, and concern for change in physical appearance.
When middle-aged adults enter their 60s, their reaction time starts to slow down further, and they experience a significant declination in their performance. The brain may also no longer function at its optimal level, leading to problems like memory loss, dementia, and may have issues with other cognitive functions such as language, attention, and visuospatial abilities [ 35 , 61 ]. It has been widely assumed that the midlife period is a critical period, thinking about death and mortality, as well as experiencing decline in physical abilities, relational, and psychological capacities [ 80 ]. Therefore, early prevention should therefore be looked upon at the middle age stage to help with memory impairment, as well as emotional control.
Middle-aged adults typically lead very active lives while also engaging in self-development programs aimed at enhancing their spiritual, emotional, and physical well-being. Muslim adult, for instance, will prefer to go to the mosque, surau, or Islamic center to seek for Islamic education [ 42 ] to enrich their knowledge and gain serenity through the command of Islam. This indicates that an individual in the middle-aged is inclined to reflect and improve the quality of one’s daily practice. Unfortunately, during the Covid-19 pandemic outbreak, many lectures at the mosques and other institutions could not be held, resulting in many people having to work from the home. As a result, many have taken the initiative to hold religious lectures online through video conferences such as via Zoom, WebEx, Jitsi Meet, Google Meet applications, and many more [ 1 ]. There are also those who watch religious lectures that have been prerecorded on certain channels, such as YouTube or podcasts. However, the enthusiasm and motivation for online and prerecorded learning is not the same and less encouraging as compared to face-to-face lectures.
Health management apps have shown to be useful for treating a variety of illnesses such as chronic illnesses caused by obesity, high blood pressure, diabetes, and so on [ 32 ]. As middle-aged adults are smartphone and tablet active users, they can use these portable devices to track their healthy lifestyle habits, maintain social communication, prevent accidents, and seek information [ 91 ]. In addition to chronic illness management using mobile applications, there is also a concern on how middle-aged adults can utilize mobile technology in fulfilling their spiritual journey towards a quality lifestyle. For example, they can learn how to acquire a literal understanding of the Quran through a spiritual mobile application. This will help a Muslim to elevate their understanding, motivation, and devotion towards Islam, which eventually leads them to become a better person emotionally and psychologically. All of these exhibit many important experiences associated with middle-age adults, most involving work and family, and self-development [ 53 ].
Mobile devices such as smartphones have gained popularity because they allow people to stay in touch and provide easy access to information anywhere and anytime [ 89 ]. Therefore, investigating the acceptance and adoption of mobile learning by the middle-aged adults through a systematic literature is important in highlighting the gap for any future work.
This review paper presents the fundamentals of mobile learning and the utilization of mobile technology in the learning environments. Mobile learning theories are also highlighted to show the significance of mobile learning towards middle-aged adults. Based on the research context found in the selected literature, the researchers here provide a systematic mapping of the employed methodologies in the area of mobile learning research. The purpose of the systematic mapping is to determine the most appropriate methodology for future research on middle-aged adults in areas of mobile learning.
2 Mobile learning
M-learning is a subset of ‘e-learning’ while ‘e-learning’ is the subset of distance learning that focuses on learning across context and learning with mobile devices, which can take place anytime, anywhere [ 43 , 62 ]. For example, learning may happen at the workplace, at home, and at places of leisure. The learning may be related to work demands, self-improvement, or leisure; and it is mobile with respect to time where it happens at different times during the day, on working days, or on weekends [ 68 ].
According to Ozdamli and Cavus [ 70 ], learners, teacher, environment, content, and assessment are the basic elements of mobile learning. The core characteristics of mobile learning are ubiquitous, portable size of mobile tools, blended, private, interactive, collaborative, and instant information. They enable learners to be in the right place at the right time, that is, to be where they can experience the authentic joy of learning.
Since learning can be performed anywhere and anytime using electronic devices, Traxler [ 85 ] defines that mobile learning is a learning process that is delivered through the support of mobile devices such as personal digital assistants, smartphones, wireless laptops, and tablets. This understanding is supported by Keegan [ 45 ] who suggested that m-learning should be restricted to learning on small and portable devices as mobile devices that could be carried everywhere.
According to Nordin, et al. [ 69 ], the requirements for mobile learning environment include technology, that is, (1) highly portable (to support learning whenever and wherever), (2) individual(the design should be able to support individual learning, cater for individual learning styles and be adaptable to learners’ abilities), (3) unobtrusive(where learners should be able to retrieve knowledge without the technology becoming a deterrent), (4) available(enabling communication with friends, experts and/or teachers), (5) adaptable(the context of learning should be adaptable to situations and the individual’s skills and knowledge development), (6) persistent( able to manage the learner’s learning despite the changes in the technology itself), (7) useful(useful to learners for everyday chores), and (8) user-friendly(easy for people to use and must not create technophobia among new users).
3 Mobile technology
Today, it is fortunate that mobile technology’s on-demand capability puts learning back into the learner’s hands by allowing users to take the initiative in diagnosing their learning needs, formulating learning goals, identifying human, and material resources for learning, choosing and implementing appropriate learning strategies, and evaluating those learning outcomes [ 50 ].
Mobile technology covers a wide range of mobile devices such as portable electronic devices used to perform a wide variety of communication, business, productivity, and lifestyle tasks such as parenting [ 26 , 66 ]. It is also connected through a cellular communication network or a wireless connection. The common mobile technologies that allow these tasks are cellular phones, PDAs, handheld computers, tablets, laptops, and wearable devices. A standard mobile technology device, such as a cellular phone, may have one or more features such as a GPS, a web browser, an instant messenger system, an audio recorder, an audio player, a video recorder, and gaming systems [ 4 ].
In the area of healthcare, numerous studies have been conducted on the use of mobile devices with wearable devices [ 21 , 39 , 87 ] to monitor the health of the elderly and individuals with disabilities. By using mobile apps, the health of the elderly and young adults can also be tracked [ 12 , 20 , 40 ] and diagnosed using mobile game-based screening tools [ 34 ], especially when facing challenges and stressful time during the Covid-19 pandemic [ 79 ] , or during post-college life transition [ 27 ]. Not all older people are proficient in using mobile devices. Therefore, there are researchers who make studies related to how older and young adults (university students) manages their mobile device security and privacy settings of their mobile devices in the context of social interaction and motivation [ 64 , 67 , 90 ].
Besides the usage of mobile devices in the healthcare area, the growth of mobile devices is significant and impactful in the education area such as in teaching history using 3D [ 57 ] and safety education [ 13 ], personal learning and workplace learning [ 29 ]. The use of mobile devices such as smartphones and tablets has become truly ubiquitous and has a potential for improving student learning, which can happen in collaborative, authentic settings, i.e., real life contexts and use active learning approaches [ 18 ]. As smartphones have become popular devices among youth nowadays [ 36 , 65 ], these devices can be utilized and embraced in the classroom teaching environment. By having a smartphone with wi-fi connectivity, Bluetooth, camera, color display, audio/video recording capability, it is already suitable for a person to adopt m-learning [ 36 ]. Majority of students spend most of their time (6 to 24 hours) on the Internet using their smartphones [ 8 ].
Smartphones also have become essential communication tools for older adults to stay connected with their family and peers [ 93 ]. Compared with younger adults, older adults tend to be more likely to use mobile phones for their original design purpose—that is, making calls for instrumental reasons such as arranging plans and other instrumental activities rather than playing games, surfing the internet, or using auxiliary applications [ 91 ]. The intervention of mobile technology in older adults’ lifestyles can improve their well-being and keep their mind and body active as well as prevent or slow down cognitive decline. For instance, mobile games can be used to capture cognitive learning outcomes and the process of knowledge acquisition [ 92 ]. Through activities such as interacting with easy games [ 71 ], taking and managing photographs, sending messages via SMS, video or audio calls, and reading newspapers via webpages may help cognitive and noncognitive stimulation of older adults.
Mobile computing devices become more situated, personal, collaborative, and lifelong and these innovations will become embedded, ubiquitous, and equipped with enhanced features for rich social interaction, contextual awareness, and access to the Internet. Hence, extending learning outside the classroom and into the learner's environment, mobile learning can have a significant impact on middle-aged adults. However, based on the research context in the areas of mobile learning, existing studies have concentrated exclusively on aspects of the mobile device use, such as accessibility, usability, and adoption, among young and older adults, while middle-aged adults have received less attention. Thus, the use of mobile devices among middle-aged adults should be further investigated to determine how mobile devices can assist them in acquiring knowledge and developing themselves while leading hectic lifestyles and having to deal with the Covid-19 pandemic, towards their long-life wellbeing.
4 Multimedia in Mobile learning application
Using mobile device as a learning tool is a new way for learners to learn as they like, anywhere and anytime. Moreover, an application that contains multimedia elements such as text, animation, graphic and video will engage and attract the attention of the student. Mobile learning application used in mobile learning environments varies, such as Learning Management System (LMS), Short Messaging services (SMS), Podcasting, Social Networking, Instant Messaging, Blogging, Facebook, Microblogging, Wiki, QR, 3D and Augmented Reality [ 81 ].
SMS and videos have long been used as language learning tool through the use of mobile phones and personal digital assistants (PDAs) [ 68 ], and today, many have benefits from using WhatsApp, flashcards and mind maps, on-line videos, and social networks in learning. Recently, Duolingo is said to be a popular application for new language learning where learners can interact with intelligent chatbots that give corrective feedback and awards at the same time [ 49 ].
In the fast-aging population countries like China, senior users have become a significant new growth point that cannot be ignored in social network sites to keep continuous competitiveness. In China, WeChat is the most popular social software for senior citizens. This is due to the good user experience and operability, where some senior users manage to operate the application although they have no computer skills or they know little about the network [ 11 ].
On the other hand, instant messaging apps such as WhatsApp and Line have become a popular mobile app amongst students. In a classroom environment, the student may use these apps to interact with teachers outside the class and using smartphones to manage their group assignments. The use of instant messaging applications promotes collaborative learning [ 7 ] and flexible learning, improves student participation, increase communication and interaction between lecturers and students, as well as improve the performance of teaching and learning [ 10 ].
Text editors such as the Mobile MS office, content management systems such as Learning Management Systems (LMSs), and audio-video recording of lectures did not get much attention by the students in terms of its usage via the smartphone. The reason for the low usage of these functions and features could be due to the limited screens space, which makes it difficult to read large documents, and the small sized keypad makes data entry cumbersome [ 36 ]. To make mobile learning more interesting, game-based elements have been used to improve the students’ engagement and enjoyment in learning. For instance, Kahoot is a game-based technological platform that can be accessed from, for instance, smart devices or a laptop. The game-based learning application (app) can benefit working adults who are adult learners with diverse learning abilities. Chunking method was used to break down complex concepts into smaller parts in the form of multiple-choice questions. The students’ learning process is tested and corrected, in real time, through the statistics which are generated from this chunking process. Kahoot creates a safe environment for students to make mistakes through multiple choice questions, and yet relearn it without being judged by their peers. However, the drawback of Kahoot is, it does not adequately support the learning experience of adult learners [ 74 ].
To achieve a successful ageing life, positive spirituality indeed has a close relationship with physical and mental abilities. There have been studies that develop an Empathic-Virtual Coach (VC) to involve senior users in enjoying a healthy lifestyle with respect to diet, physical activity, and social interactions, while in turn supporting their carers [ 41 ]. Furthermore, in addition to physical support, adults also require emotional and spiritual help for a balanced lifestyle. For example, Sevkli, et al. [ 75 ] in their study had designed and developed mobile Hadith Learning Systems (HLS) that were able to encourage and promote hadith learning for young and middle-aged Muslims. Hence, mobile apps appear to be one of the tools that can be used to promote a balanced well-being lifestyle for the older people such as their social status, independence in their everyday activities, health status, standard of living, or leisure activities of the aging population.
5 Mobile learning theory
According to Lee, et al. [ 56 ], there is an increasing number of adult learners entering or returning to university. Despite the growing number of nontraditional adult students in online higher education, little is known about the dynamic processes of adult distance learning, through which adult students struggle to develop their learning ability to balance their life and study, and to become self-regulated learners, and ultimately as competent selves and lifelong learners. The implementations of mobile learning are supported and guided by theories such as Behaviorism, Cognitivism, Constructivism, Situated Learning, Problem-Based Learning, Context Awareness Learning, Socio-Cultural Theory, Collaborative Learning, Conversational Learning, Lifelong Learning, Informal Learning as well as Activity Theory, Connectivism, Navigationism, Location-based learning [ 46 , 68 ]. The classification of activities around the main theories and areas of learning relevant to learning with mobile technologies are shown in Table 1 .
Lifelong learning happens not only in learning institutions such as community colleges or higher learning institutions, but can also happen anytime and anywhere according to the needs of the individual [ 69 ]. Informal and lifelong learning are often referred to adult education or continuing education, which means a learning process that occurs as blended learning with everyday life unobtrusively and seamlessly [ 73 ]. The unique characteristic of lifelong learning is the fact that it is centered on the learner. Because of that, the use of technology in offering a flexible learning framework is often favored by adult learners [ 69 ]. In addition, when compared to conventional methods such as textbooks, mobile learning tools, especially learning through mobile apps, are intrinsically inspiring, provide greater satisfaction, increase student well-being, and have positive implications for long-term student persistence [ 78 ].
Lifelong adult learners are different from young learners (school or university students) who may devote significant amounts of time to study each day, as their learning time is scattered due to family responsibilities, work obligations, and other social obligations [ 44 ]. However, the keys to unlocking the secrets to successful adult learning online are embedded in the basic principles that guide adult learners. The subsequent six principles upon which Knowles [ 51 ] constructed his formal and andragogical concept are shown in Table 2 .
6 Methodology
This study carried out an extensive literature review to identify the research gap, focusing on the related literature published within the period of 2016 to 2021. The aim of this systematic review is to investigate the trend of previous research on the acceptance and adoption of mobile learning by middle-aged adults. In order to justify the research gap based on the previous studies, this article will also provide views on the existing mobile learning usage targeted at solving user’s adoption of mobile learning towards young and older adults.
To conduct the systematic review, the researchers followed the procedure defined by Kitchenham [ 48 ], which is one of the most complete and suitable methods for reviewing studies in computer science. We carried out this review in three main phases: 1) planning of systematic mapping; 2) conducting the review; and 3) reporting the review. The phases of this systematic review and the related activities are shown in Fig. 1 .

Phases of conducting this systematic review
Planning of the Systematic Mapping
Activities involved in this stage were aimed to identify the objectives of the review. These activities are as follows:
Discovering the gap of the existing systematic reviews
In this step, a comprehensive search was performed in the cyberspace to locate the related review studies in mobile learning. Some of the bibliographic databases accessed included EBSCO Discovery Service, Science Direct, Google Scholar, Scopus, and IEEE.
Specifying the research questions
The research questions we have formulated for this review attempt to acquire the understanding and to determine the research gap on mobile learning usage in assisting lifelong learning in the context of spiritual among middle-aged adults. These questions are related to the acceptance and adoption of mobile learning towards middle-aged adults. The research questions are:
What are the fundamentals and background of mobile learning in the learning environment, including its adoption, acceptance, and available applications?
What are the research methodologies employed in the current studies carried out in mobile learning field?
What are the core research gaps should be further investigated by researchers in mobile learning towards middle-aged adults?
Identifying the relevant bibliographic databases
To answer the research questions and find relevant studies, bibliographic databases that cover majority of journals and conference papers associated with the field of human-computer interaction and mobile learning were selected. Related literatures published within the period of 2016 to 2021 were chosen in this research and the relevant bibliographic databases are ACM ( https://www.acm.org/ ), Emerald ( https://www.emerald.com/insight/ ), EBSCO Discovery Service ( http://search.ebscohost.com ), Science Direct ( http://sciencedirect.com ), Google Scholar ( http://scholar.google.com ), Scopus ( http://scopus.com ), and IEEE ( http://ieee.com ).
Conducting the Review
Activities involved in this stage were aimed to selecting related studies. These activities are as follows:
Identifying the Relevant Studies
In identifying the relevant studies, a search using key words such as “human-computer interaction”, “mobile learning”, “middle-aged adults”, “us- ability” was conducted. Accordingly, Boolean OR was used for alternative spellings, synonyms, or alternative terms, and Boolean AND was applied to connect the main terms. The complete list of search keywords of the review is provided in Table 3 .
Two additional search strategies were applied to retrieve the maximum number of relevant papers. The first strategy was reviewing the reference list of selected papers to find more related papers. The second strategy was googling the authors of selected studies to find potential related research.
Defining Selection Criteria
For selecting the primary papers, the following criteria based on the purpose of this study are defined.
Inclusion Criteria:
Studies containing mobile learning, acceptance, and adoption among mobile devices users.
Studies dealing with factors that contribute to the adoption and acceptance of mobile learning in the educational environments or working environments.
Studies utilizing mobile learning applications related to education, health care, data collection, and engineering that motivate users to use mobile learning.
Studied involving mobile learning users in category young adults, middle-aged adults, and older adults.
Exclusion Criteria:
Studies in learning environments that do not relate to the mobile learning context.
Studies of mobile learning that involve children such as kindergarten students or users with special needs.
Studies that are reluctant to serious mobile learning.
Papers that are only available in the form of abstracts or PowerPoint presentations.
Papers that are not written in English.
Selecting Primary Studies
The titles and abstracts of searched papers were reviewed based on the inclusion and exclusion criteria. Every paper that met at least one of the criteria and without any of the exclusion criteria was included in the review. For papers that could not be excluded based on reading of the titles and abstracts, the full texts of the papers were reviewed. Through this process, 65 articles were selected from the 531 papers initially found. 292 papers were excluded only by reading the topics, 105 papers by reading the abstracts, and 65 papers by reading the full text.
Validation control of the Primary Studies
In order to maintain the quality of the selected studies, the primary studies chosen by the first reviewer were double-checked by a second author. The evaluation of the selected paper was based on the evaluation questions as follows:
Whether a proposed mobile learning solution is implemented in the research context?
Whether the methodology of mobile learning solution is suitable for middle-aged adult?
To what extent the proposed solution effects the middle-aged adult in mobile learning?
The procedure of selecting the primary papers is illustrated in Fig. 2 .
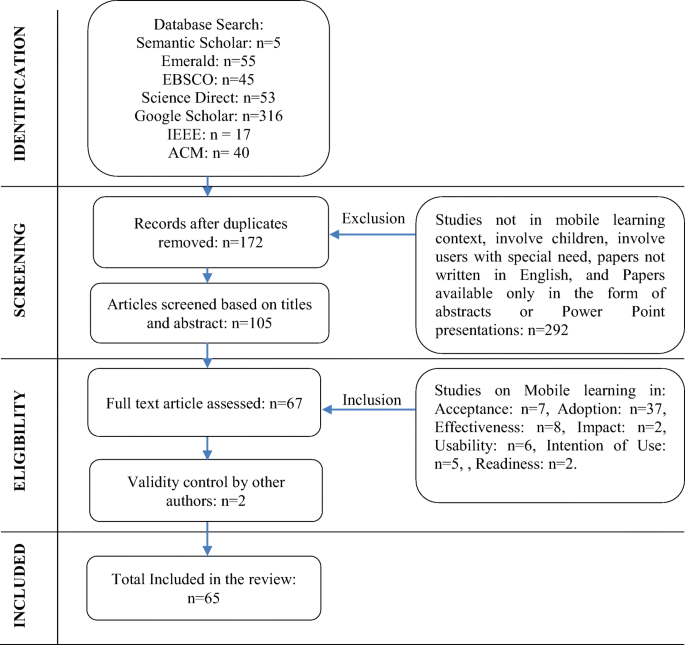
Selecting the primary papers
Data Extraction and Synthesis
In order to extract and synthesize the data to answer the research questions, the selected studies are classified into five categories as follows:
Mobile learning and their research context: This categorization answer the first research question and helps to find the fundamentals and background in mobile research based on research context such as acceptance, adoption, effectiveness, impact, intention of use, usability, and readiness.
Methodology in the mobile learning research area : In order to answer the second research question and find the methodologies employed in the related context, the research context with the methods employed by the researchers was mapped as shown in Table 7 . Based on this mapping, the instruments that have been used in mobile learning research involving middle-aged adults can be identified.
Instruments used in Mobile learning research context: This category answers the second research question. From the systematic mapping done, it was found that the common research instruments used were Questionnaire, Interview, Experiments and Task Analysis. Here, the most preferable instruments used in mobile learning research were highlighted.
Mobile learning solutions in general: This category answers the third research question in order to find the gap in mobile learning research. Articles found in this study include mobile learning articles for young and older adults to show the trend of research towards adulthood. Since the focus of this systematic mapping is on identifying mobile learning technology applied to the middle-aged adults, thus those works focusing on the application of mobile learning not on adult learners or studies on users with special needs were excluded.
Solution for middle-aged adult in mobile learning: This category also answers the third research question in presenting the future works related to mobile learning involving middle-aged adults. This article begins by explaining the use of mobile technology in a learning environment, and the mobile learning theories that form the basis for the comparison of the existing mobile learning solutions for middle-aged adults.
Effects of mobile learning on middle-aged adult: This category answer the importance of the mobile learning towards middle-aged adults for a healthy well-being by assessing the number of studies related to middle-aged adults.
Reporting the Review
In the following section, the outcomes of reviewing the selected studies were reported and the results were discussed in detail, to respond to the defined research questions.
7 Results of the systematic mapping
From the search procedure and criteria, a total number of 65 articles are extracted. The distribution of the primary studies according to the publishing year is shown in Table 4 and Fig. 3 . The articles searched for this systematic review study are from 2016 to 2021. The reason is that this study aims to identify the latest research trends in the field of mobile learning with middle-aged adults. Finding shows that there are several studies from 2016 to 2018 that focus on this topic. The number of articles on mobile learning increased significantly from 2019 to 2020, which may be due to the outbreak of the Covid 19 pandemic. In education, for example, many institutions and organizations have drastically shifted from the traditional teaching and learning approach to online platforms. As a result, there is a considerable amount of research on mobile learning focusing on students in schools, universities, and academic staff. Meanwhile, a lot of study has been done in the field of healthcare with the elderly and middle-aged individuals, because their health begins to decline at this age.
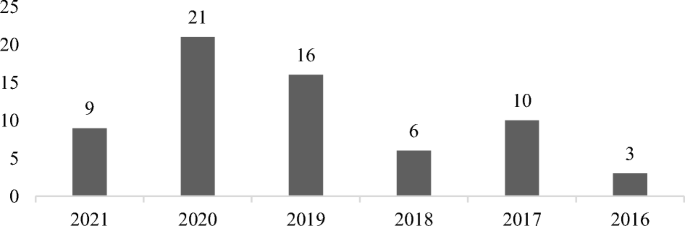
Distribution of reviewed studies by year
It would also be interesting to find out the distribution of studies by countries, as shown in Table 5 . This shows that China had contributed the most research articles in this area of mobile learning. In article [ 13 , 21 , 72 , 77 , 81 , 88 ], the country where the study was conducted was not specified.
8 Participants
The categories of participants in the selected studies consist of young adults, middle-aged adults, and older adults. The number of studies based on age category is illustrated in Table 6 and Fig. 4 . It is found that the number of studies involving young adults is higher compared to studies involving older adults and middle-aged adults. This is due to the fact that young adults are frequent users of smartphones and are more adept at using mobile apps. Furthermore, since they are unable to attend college or universities due to the Covid-19 outbreak, many students are required to study online from home using mobile devices.
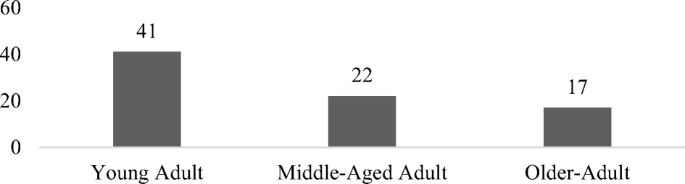
Number of studies based on participants’ age category
The details of the reference pertaining to the articles based on participants’ categories (older adults (OA), middle-aged adults (MA), and young adults (YA)) are listed in Table 8 .
9 Research context in Mobile learning
The articles obtained for this study were categorized by research area, as shown in Table 7 . Based on the results, mobile learning was studied in the following areas: Education, Healthcare, Usability, Transactional Services, and Social and Communication. Figure 5 illustrates the number of articles published on each research topic. The finding shows that many researchers prefer to conduct research in the field of education. This is because computers and mobile devices are widely used in educational institutions among young adults. On the other hand, studies that focus on middle-aged and older adults are usually concerned with language or vocabulary learning. The healthcare field is also receiving a lot of attention from researchers, and studies on mobile learning in this field are usually related to elderly and middle-aged people because older people and middle-aged people tend to be more vulnerable to health problems. The number of articles from other fields is low because studies on middle-aged adults and mobile learning did not match the scope and range of years defined for this study.
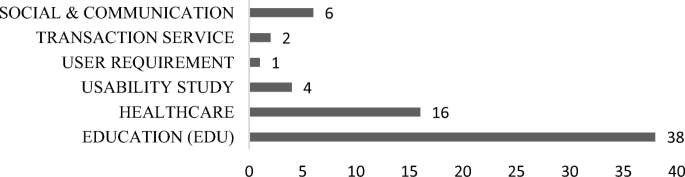
Number of articles in the research domain
Because the study related to mobile learning is very broad, therefore the article obtained has been classified into research context. Research context was determined based on the previous and current research in the field of mobile learning. It was found that many researchers in the field of mobile learning have studied the acceptance, adoption, effectiveness, impact, intention of use, readiness, and usability of mobile learning. The categorized articles are listed in Table 9 in section 11, with additional information on the methodology used in each study. Figure 6 shows the number of articles obtained by research context.
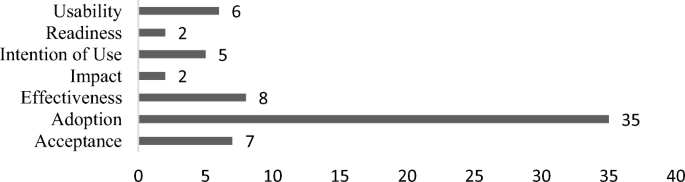
Number of papers by research context
10 Mobile learning towards the middle-aged adults
From these articles, not many researchers have examined the adoption of mobile learning by middle-aged adults. As mentioned earlier, a person in his or her forties is already inclined to focus on and enhance the standard of daily practice while also finding serenity. At this stage, many people have developed an inclination and willingness to gain more religious knowledge. Adult Muslims who work during the day, would rather choose to visit a mosque or surau to learn about Islam through religious lectures in the evening or at night. During the Covid-19 pandemic outbreak, many people were forced to work from home, and many lectures at the mosque were cancelled. As a result, many have taken the initiative to hold religious lectures via video conferences over the internet (e.g.: Zoom, WebEx). Others tend to watch religious lectures that have been posted on YouTube or other related platforms. However, as opposed to face-to-face seminars, the excitement and encouragement to attend online and prerecorded learning is lacking. Midlife brings with it a multitude of significant life experiences, the majority of which revolve around work, family, especially parenting, and self-development. Tablets are being used more commonly by middle-aged adults to monitor healthy lifestyle behaviors, maintain social contact, avoid injuries, and search information.
Many middle-aged and older adults are using the Internet to obtain information about health conditions and treatments, to get social support and advice from others with similar health-related experiences, and to access apps to help them manage their health [ 28 ]. For instance, Huang, et al. [ 32 ], studied on the attitude of middle-aged adults towards health app usage. From the study, they discovered that middle-aged adults who have no habits in health management tend to consider health applications as valuable tools and have a positive impact on them, while those who already have the habit, do not tend to consider health applications as valuable tool to be used in their daily routines. There are also some middle-aged adults who decide not to use health apps due to some sentimental reasons and the confidence of middle-aged adults in using a smartphone influences their cognitive assessment of health apps.
Table 8 shows the list of studies that are related to middle-aged adults. The age range of the middle-aged adults by each researcher varies. In this study, the age range of the adult is between 40 to 60 years old, which means the selected articles involve participants in this age range. A total of 22 articles were selected that involved middle-aged adults. In the field of language learning, two papers were identified. From these articles, it is found that the study of mobile learning with middle-aged adults is widely conducted in education area. The use of mobile apps in healthcare is also considered important, as this area is also the focus of researchers. The remaining articles are related to the study of user requirements, usability, and the design and development of mobile apps for middle-aged adults.
11 Research methodology
Research methodology is the main key to perform academic research and the strength of a research. The research methodology found used in the selected articles are Questionnaire, Interview, Systematic Literature Review, Literature Review, Reporting, Task Analysis and Experiment. Figure 7 shows the most popular research method used by a researcher in the field of mobile learning is questionnaire (n=24). This methodology has been used in studies that require a large amount of data from many respondents. The second most popular research method used in mobile learning research area is the Interview (n=9). There are also studies that require the use of multiple research methods to answer research questions.
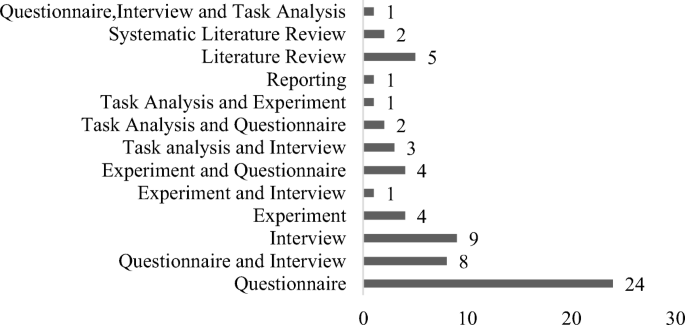
Number of articles by research methodology
Table 9 shows the methodologies employed in the selected articles. However, articles [ 23 , 49 , 63 , 72 , 81 , 83 , 88 ], and [ 77 ] are not included because these articles are review articles.
In the literature, the questionnaire was found to be the most common method used by researchers for data collection involving many participants among young adults and middle-aged adults. On the other hand, the interview method only involved small groups of participants and was carried out in a short time period. Task analysis with interview method was used in three research studies to evaluate the usability, acceptance, and adoption. The studies were done towards young adults and older adults.
In the quantitative research method, the questionnaire instrument was used by the researchers to understand users’ motivation to use e-learning as a medium of learning [ 60 ]; the use of mobile technology and means of internet access [ 24 ]; awareness in using mobile devices towards mobile learning [ 14 ]; investigate the perception of students related to educational use of mobile phones [ 36 , 55 , 76 ]; investigate students’ behavioral intentions [ 3 ] and knowledge transfer among adult workers [ 52 ]; identify factors that affect the intention to use m-learning by learning the experience of the m-learning system by the participants [ 84 ], measure usability [ 19 , 75 ]; use and engagement with m-learning [ 2 ]; collaborative learning experience in social media environment [ 7 ], students’ immersion in the game and their perceived learning outcomes [ 33 ], and the use of mobile application [ 11 , 65 ].
Almost all researchers have formally collected demographic data such as gender, age, degree program, year of study, and race of the participants. There is only one study that collects data on working background because the participants in the study involved working adults. Amongst the selected articles , Al-Adwan, et al. [ 3 ] and Lazar, et al. [ 55 ], validated the content of the survey using experts before the questionnaire was distributed to participants. Dhanapal, et al. [ 19 ] and Huizenga, et al. [ 33 ] carried out a pilot test to identify the flaws and improves the questionnaire. All but one of the researchers used Point Likert scale, while MICAN [ 65 ] uses short answer questions, multiple choices with 1 or n answers, single or two-dimensional questions. The duration of data collection was less than 40 weeks depending on the targeted number of participants.
For the qualitative research method, data was collected via task analysis and interviews. Data were captured through multiple channels including video data analysis and interview content analysis. From the selected articles, it is found that task analysis and interview method were employed in the mobile learning domain to understand participants’ actions, performance, and usability towards mobile apps. The task activities that have been examined by researchers are navigation tasks (with task activity duration of 1.5 hours for older adults to complete searching and navigating using several mobile applications) [ 58 ], quiz activities using Kahoot application (held within 13 weeks for working adult and the task activities were perform in a classroom environment) [ 74 ], mobile devices usage training ( duration of 9 months of training intervention involving older people), and the task activities (e.g.: sending messages, video and audio calls ) was performed in a hospital [ 15 ]; Vocabulary learning [ 86 , 97 ]; games application with task duration of 5 to 20 minutes [ 71 ]; and usability testing [ 30 ]. Open-ended questions were used in the interview sessions [ 71 ] and all the audio recordings of the interviews were transcribed verbatim for analysis purposes [ 58 ].
In the experimental research design, two groups were created with specific condition applied. The treatment group and the control group involved in the experiment and questionnaire research approach can be seen in articles [ 9 , 16 , 38 , 92 ] as listed in Table 9 . For instance, in Bensalem [ 9 ], aims at investigating students' perceptions about the use of WhatsApp in learning vocabulary and in the study, twenty-one participants were randomly assigned to the experimental group. Participants from the experimental group are required to complete and submit their vocabulary assignments via WhatsApp. In the assignment, students are required to search the meaning of new words in a dictionary and build sentences using each word. On the other hand, participants from a control group need to submit the same homework assignment using the traditional paper and pencil method. Later, a questionnaire was distributed to the participants and the collected data was used to measure the participants’ perception of the use of WhatsApp in vocabulary learning.
12 Discussion
In this article, a systematic review was conducted to provide a thorough analysis on the methodologies adopted by researchers in mobile learning. The number of research papers in the year 2020 exceeds the number of research papers in the previous year. This could be due to the outbreak of the Covid-19 pandemic that triggered higher number of papers. During the pandemic, everyone had to work from home, and many organizations, including public and private higher learning institutions, were unable to carry out traditional teaching and learning activities. As a result, many studies or meetings were required to be conducted online.
The country with the highest number of research papers in the field of mobile learning is China with 11 articles. There is a lack of study in mobile learning that focuses on middle-aged adults. Out of 65 research papers, a total of 22 research papers are related to middle-aged adults whereby the distribution of research can be seen in countries such as in Czech Republic (n=1), United States (n=5), China (n=3), Germany (n=1). Singapore (n=2), Turkey (n=1), Brazil (n=1), Poland (n=1), Bangladesh (n=1), United Kingdom (n=2) and 2 articles did not mention the country in which the research was carried out. Studies related to middle-aged adults in Malaysia are not very encouraging, therefore the study of middle-aged adults in the field of mobile learning needs to be given more attention.
The articles selected in this systematic review were classified by research context to identify the focus of previous researchers on the use of mobile learning by middle-aged individuals. Overall, it was found that studies related to the adoption of mobile learning, mobile applications and mobile devices have gained significant attention among researchers, followed by studies related to the acceptance and mobile learning usage. However, studies on examining the adoption and effectiveness of mobile learning usage towards middle-aged adults are still lacking. Examining the effectiveness of mobile learning usage is crucial to provide guidance towards decision making and development work in the future.
The field of education is a popular field for researchers as it involves teachers and young adults who are mainly engaged in the learning environments. Research on middle-aged adults in the educational field is found in seven articles, where two of the articles focused on vocabulary learning. One study on Hadith learning for middle-aged adults, which has been classified as a study on spiritual learning under the educational research domain was also identified. The remaining four articles are respectively related to the use of game applications in teaching adults, the use of mobile devices in sharing information among adult workers, and the readiness of the teachers in adopting mobile learning in a classroom. Besides that, there is also a lack of research towards middle-aged adults in the area of mobile usability and user requirements. Research in the healthcare domain mostly involves older adults where most researchers extensively investigate the use of mobile devices and mobile applications towards healthy ageing and wellbeing.
The coding of the research methods was based on the methods reported by the researchers in their methodology section. Questionnaire is a popular instrument used across quantitative and mixed research approaches for data collection. The questionnaire developed by the researcher will be validated by the experts and tested before it was distributed accordingly to the targeted participants. Task analysis and interview approach can be used to observe the behavior of the users and to evaluate users’ feedback in the learning environment. Even though the method was not extensively used by the researchers from the selected literature focusing on middle-aged adults, this method to be employed in the mobile learning research to gain more insight on the effectiveness of mobile technologies in the learning environment of middle-aged adults was suggested.
Nowadays, almost everyone owns a smartphone, as smartphone prices have dropped significantly, making them affordable for more users. All smartphone users are capable to use most of the basic features of the mobile device, such as downloading applications from the Apple Store or Google Play. Given that middle-aged individuals are heavy smartphone users, it is critical to understand how users utilize mobile technology such as smartphones not just for work, leisure, and entertainment, but also for knowledge acquisition.
Middle-aged adults are self-directed, able to take responsibility for their learning, have a variety of experiences and backgrounds, and are motivated and willing to learn while effectively managing real-world situations. Hence, middle-aged adults can benefit from webinars and short courses delivered online. Therefore, more research should be conducted on mobile learning for middle-aged adults.
13 Conclusion and future work
The novelty of this study is that it contributes to the understanding of the research trends based on research context and methods used in research related to middle-aged adults in mobile learning. It is noted that there are still few studies that address the adoption and effectiveness of mobile apps in the area of religious orientation, especially among middle-aged adults. For instance, before the Covid-19 outbreak, middle-aged Muslims in Malaysia preferred to attend religious courses and trainings to improve their spiritual and religious orientation [ 96 ] based on face-to-face with teachers in a classroom. Therefore, it is critical to determine whether middle-aged adults intend and consent to religious and spiritual learning, such as learning the Quran to be conducted via mobile devices. It is hoped that the use of mobile learning will enable adults' lifelong learning to be improved and done continuously under any situation in the future. This study suggests further studies on middle-aged in the field of mobile learning as follows:
Skills and Knowledge Development
The use of mobile learning among middle-aged adults begins with an awareness and intention to use mobile devices. Generally, middle-aged adults who own smartphones, they already have skills to download apps from the Google Store or App Store and set security preferences. Hence, they must intend to use mobile learning to develop their skills and knowledge. This is because between the ages of 40 and 60, they are usually busy with their work while facing problems such as increasing concerns about health, death of a friend or relative, changes in wages/salaries, and concerns about changes in physical appearance. Therefore, middle-aged adults need to seek knowledge that will make them be satisfied and enable them to lead a better and healthier lifestyle. For example, middle-aged Muslims can learn to understand the Quran through mobile learning to achieve a better quality of life because the Quran is the final revelation and book from Allah s.w.t to humankind as guidance and direction to the right path.
Mobile Learning Application with Multimedia
Mobile learning Application with multimedia plays a great role in motivating learners in learning via digital devices such as smartphones. It is crucial to design and develop mobile learning apps with appropriate multimedia elements such as texts, images, icons, and animations that meet the needs of middle-aged adult learners. In addition, middle-aged adults need to be helped to increase their motivation to learn and improve their memory performance in vocabulary memorization. Therefore, for future work, mobile app development needs to be carefully developed based on user needs especially for the multimedia elements such as the text, graphic, video and animation.
Mobile Learning Application and Quick Assessment
Assessment is a critical component of learning since it demonstrates progress. Because most of the learning occurs online and involves many students, a teacher develops easy assessment tools and procedures that enable them to rapidly assess their students’ learning progress. Numerous game-based apps have aided in the facilitation of teaching and may be used to measure a student learning progress. Additionally, to make mobile learning more interesting, game-based elements have been used to improve the students’ engagement and enjoyment in learning. For instance, Kahoot is a game-based technological platform that can be accessed using, for instance, a smart device or a laptop. The game-based learning application (app) can benefit working adults who are adult learners with diverse learning abilities. Chunking method was used to break down complex concepts into smaller parts in the form of multiple-choice questions. The students’ learning process is tested and corrected, in real time, through the statistics which are generated from this chunking process. Kahoot creates a safe environment for students to make mistakes through multiple choice questions, and yet relearn it without being judged by their peers. However, the drawback of Kahoot is, it does not adequately support the learning experience of adult learners Seah [ 74 ]. Therefore, in the future, the development of mobile learning apps for middle-aged adults might include a gamification aspect that allows easy assessment for self-monitoring of learning progress.
Research Methodology
The finding of this study shows that questionnaire is a popular instrument used across quantitative and mixed research approaches for data collection. The questionnaire developed by the researcher will be validated by the experts and tested before it was distributed accordingly to the targeted participants. However, based on the research context and methodologies found in the literature, the study on middle-aged adults was not getting the enough intention among researchers. Furthermore, as Covid-19 pandemic has impacted people’s life, many are reluctant to participate in answering questionnaires as they may be unmotivated due to job loss, adaptation to new norms or due to the death of their family members. Therefore, in the future, it is hereby recommended that a contribution back to society such as given some tokens to the participants [ 66 , 90 ] can be practiced in the research methodology. Besides that, a researcher also can conduct a free intensive course of related field to a group of respondents to upgrade the lifestyle and well-being among respondents. Hence, this can increase public participation in research, especially when involving busy and elderly respondents and at the same time the respondents can learn new knowledge while also contributing to the research study.
Adedoyin OB, Soykan E (2020) Covid-19 pandemic and online learning: the challenges and opportunities. Interact Learn Environ:1–13
Al Masarweh M (2019) Evaluating M-Learning System Adoption by Faculty Members in Saudi Arabia Using Concern Based Adoption Model (CBAM) Stages of Concern. Int J Emerg Technol Learn 14(5)
Al-Adwan AS, Al-Madadha A, Zvirzdinaite Z (2018) Modeling students’ readiness to adopt mobile learning in higher education: An empirical study. Int Rev Res Open Dist Learn 19(1)
Alkhezzi F, Ahmed MS (2020) A Review of Mobile Learning Technology Integration: Models, Frameworks, and Theories. Coll Stud J 54(4):491–504, Winter2020
Google Scholar
Al-Sabbagh KW, Bradley L, Bartram L (2019) Mobile language learning applications for Arabic speaking migrants–a usability perspective. Lang Learn Higher Educ 9(1):71–95
Article Google Scholar
Amarya S, Singh K, Sabharwal M (2018) Ageing process and physiological changes. In: Gerontology. IntechOpen
Ansari JAN, Khan NA (2020) Exploring the role of social media in collaborative learning the new domain of learning. Smart Learn Environ 7(1):1–16
Anshari M, Almunawar MN, Shahrill M, Wicaksono DK, Huda M (2017) Smartphones usage in the classrooms: learning aid or interference? Educ Inf Technol 22(6):3063–3079
Bensalem E (2018) The Impact of WhatsApp on EFL students' Vocabulary Learning. Arab World Eng J 9(1):23–03
Bere A, Rambe P (2019) Understanding Mobile learning using a social embeddedness approach: a case of instant messaging. Int J Educ Dev Using Inf Commun Technol 15(2):132–153
Cao Y, Qin X, Li J, Long Q, Hu B (2020) Exploring seniors’ continuance intention to use mobile social network sites in China: a cognitive-affective-conative model. Univ Access Inf Soc 21:1–22
Cheng C-H, Chen Y-S, Sangaiah AK, Su Y-H (2018) Evidence-based personal applications of medical computing models in risk factors of cardiovascular disease for the middle-aged and elderly. Pers Ubiquit Comput 22(5):921–936
Chittaro L (2016) Designing serious games for safety education:“learn to brace” Versus traditional pictorials for aircraft passengers. IEEE Trans Vis Comput Graph 22(5):1527–1539
Article MathSciNet Google Scholar
Christensen R, Knezek G (2017) Readiness for integrating mobile learning in the classroom: challenges, preferences and possibilities. Comput Hum Behav 76:112–121. https://doi.org/10.1016/j.chb.2017.07.014
Cid A, Sotelo R, Leguisamo M, Ramírez-Michelena M (2020) Tablets for deeply disadvantaged older adults: challenges in long-term care facilities. Int J Human-Comput Stud 144:102504
Daungcharone K, Panjaburee P, Thongkoo K (2019) A mobile game-based C programming language learning: results of university students' achievement and motivations. Int J Mobile Learn Org 13(2):171–192
de Lara SMA, de Mattos Fortes RP, Russo CM, Freire AP (2016) A study on the acceptance of website interaction aids by older adults. Univ Access Inf Soc 15(3):445–460
Devshikha Bose KP (2020) Lana Grover, "a Mobile learning Community in a Living Learning Community : perceived impact on digital fluency and communication," the online journal of new horizons in education
Dhanapal S, Salman NW, Raman Kutty GK, Ansari KMS, Shah SA (2019) 'XYZ' Application as a Tool for Teaching and Learning in Institutions of Higher Learning: An Exploratory Study. Aplikasi 'XYZ' Sebagai Alat untuk Pengajaran dan Pembelajaran di Institusi Pengajian Tinggi: Satu Kajian Tinjauan (55):1–01
Martino FD, Delmastro F, Dolciotti C (2021) Malnutrition risk assessment in frail older adults using m-health and machine learning. ICC 2021 - IEEE International Conference on Communications, pp 1–6
Ejaz A, Rahim M, Khoja SA (2019) "The Effect of Cognitive Load on Gesture Acceptability of Older Adults in Mobile Application," in 2019 IEEE 10th Annual Ubiquitous Computing, Electronics & Mobile Communication Conference (UEMCON): IEEE, pp. 0979–0986.
Farivar S, Abouzahra M, Ghasemaghaei M (2020) Wearable device adoption among older adults: a mixed-methods study. Int J Inf Manag 55:102209
Galić S, Lušić Z, Stanivuk T (2020) E-LEARNING IN MARITIME AFFAIRS. J Nav Archit Mar Eng 17(1):39, 01//
Gan CL, Balakrishnan V (2018) Mobile Technology in the Classroom: what drives student-lecturer interactions? Int Human-Comput Interact 34(7):666–679. https://doi.org/10.1080/10447318.2017.1380970
García Botero G, Questier F, Zhu C (2019) Self-directed language learning in a mobile-assisted, out-of-class context: do students walk the talk? Comput Assist Lang Learn 32(1–2):71–97
Garg R (2021) "Its changes so often": Parental non-/use of mobile devices while caring for infants and toddlers at home. Proc. ACM Hum.-Comput. Interact., 5(CSCW2), Article 369. https://doi.org/10.1145/3479513
Gong C, Saha K, Chancellor S (2021) "The Smartest Decision for My Future": Social Media Reveals Challenges and Stress During Post-College Life Transition. Proc ACM Human-Comput Interact 5(CSCW2):1–29
Gordon NP, Crouch E (2019) Digital information technology use and patient preferences for internet-based health education modalities: cross-sectional survey study of middle-aged and older adults with chronic health conditions. JMIR aging 2(1):e12243
Grant MM (2019) Difficulties in defining mobile learning: analysis, design characteristics, and implications. Educ Technol Res Dev 67(2):361–388
Guinibert M (2020) Learn from your environment: A visual literacy learning model. Australas J Educ Technol 36(4):173–188. https://doi.org/10.14742/ajet.5200
Hall GS (1922) Senescence, the last half of life. Appleton, New York
Book Google Scholar
Huang Y-M, Lou S-J, Huang T-C, Jeng Y-L (2019) Middle-aged adults’ attitudes toward health app usage: a comparison with the cognitive-affective-conative model. Univ Access Inf Soc 18(4):927–938
Huizenga J, Admiraal W, Ten Dam G, Voogt J (2019) Mobile game-based learning in secondary education: students’ immersion, game activities, team performance and learning outcomes. Comput Hum Behav 99:137–143
Intarasirisawat J, Ang CS, Efstratiou C, Dickens L, Sriburapar N, Sharma D, Asawathaweeboon B (2020) An automated Mobile game-based screening tool for patients with alcohol dependence. Proc ACM Interact, Mobile, Wearab Ubiquitous Technol 4(3):1–23
Introduction to psychology: lifespan development. https://courses.lumenlearning.com/wmopen-psychology/chapter/reading-adulthood/ . Accessed 16 Feb 2021
Iqbal S (2017) Mobile phone usage and students’ perception towards m-learning: A case of undergraduate students in Pakistan. J Distance Educ/Revue de l'ducation Dist 32(1)
Islam MN, Ahmed MA, Islam AN (2020) Chakuri-bazaar: a mobile application for illiterate and semi-literate people for searching employment. Int Mobile Human Comput Interact (IJMHCI) 12(2):22–39
Jeno LM, Dettweiler U, Grytnes J-A (2020) The effects of a goal-framing and need-supportive app on undergraduates' intentions, effort, and achievement in mobile science learning. Comput Educ 159, 12/01/December 2020. https://doi.org/10.1016/j.compedu.2020.104022
Jones SL, Hue W, Kelly RM, Barnett R, Henderson V, Sengupta R (2021) Determinants of longitudinal adherence in smartphone-based self-tracking for chronic health conditions: evidence from axial Spondyloarthritis. Proc ACM Interact, Mobile, Wearab Ubiquitous Technol 5(1):1–24
Jones J, Yuan Y, Yarosh S (2021) Be consistent, work the program, be present every day: exploring Technologies for Self-Tracking in early recovery. Proc ACM Interact, Mobile, Wearab Ubiquitous Technol 5(4):1–26
Justo R et al (2020) Analysis of the interaction between elderly people and a simulated virtual coach. J Ambient Intell Humaniz Comput:1–16
Kadir MA, Arifin S, Latipun, Fuad AN (2016) Adult Learners' understanding in learning Islam using andragogy approach: a study in Kampung Siglap mosque and Al-Zuhri higher learning institute. J Educ Pract 7(32):1–10
Kadirire J, Guy R (2009) Mobile learning demystified. Evol Mobile Teach learn:15–56
Kang H, Lin X (2019) Lifelong learning on the go: English language Mobile learning in China. New Direct Adult Contin Educ 2019(162):49–60
Keegan D (2005)The incorporation of mobile learning into mainstream education and training. In: World Conference on Mobile Learning, Cape Town, vol 11, pp 1–17
Keskin NO, Metcalf D (2011) The current perspectives, theories and practices of mobile learning. Turkish Online J Educ Technol-TOJET 10(2):202–208
Kiconco RI, Rooks G, Snijders C (2020) Learning mobile money in social networks: comparing a rural and urban region in Uganda. Comput Hum Behav 103:214–225
Kitchenham B (2004) Procedures for performing systematic reviews. Keele, UK, Keele University 33(2004):1–26
Klimova B (2020) Benefits of the use of Mobile applications for learning a foreign language by elderly population. Procedia Comput Sci 176:2184–2191
Knowles MS (1975) Self-directed learning: A guide for learners and teachers.
Lippitt GL, Knowles MS, Knowles MS (1984) Andragogy in action: applying modern principles of adult learning
Kuciapski M (2017) A model of mobile technologies acceptance for knowledge transfer by employees. J Knowl Manag 21(5):1053–1076. https://doi.org/10.1108/JKM-03-2016-0136
Lachman ME, Lewkowicz C, Marcus A, Peng Y (1994) Images of midlife development among young, middle-aged, and older adults. J Adult Dev 1(4):201–211
Lachman ME, Teshale S, Agrigoroaei S (2015) Midlife as a pivotal period in the life course: balancing growth and decline at the crossroads of youth and old age. Int J Behav Dev 39(1):20–31
Lazar IM, Panisoara G, Panisoara IO (2020) Digital technology adoption scale in the blended learning context in higher education: Development, validation and testing of a specific tool. PLoS One 15(7):e0235957. https://doi.org/10.1371/journal.pone.0235957
Lee K, Choi H, Cho YH (2019) Becoming a competent self: a developmental process of adult distance learning. Internet High Educ 41:25–33
Lewis R, Taylor-Poleskey M (2021) Hidden town in 3D: teaching and reinterpreting slavery virtually at a living history museum. J Comput Cultural Heritage (JOCCH) 14(2):1–14
Li Q, Luximon Y (2020) Older adults’ use of mobile device: usability challenges while navigating various interfaces. Behav Inform Technol 39(8):837–861
Lin CJ, Ho S-H (2020) The development of a mobile user interface ability evaluation system for the elderly. Appl Ergon 89:103215
Lin X, Su S (2020) Chinese college Students' attitude and intention of adopting Mobile learning. Int J Educ Dev Using Inf Commun Technol 16(2):6–21
Manca M et al (2021) The impact of serious games with humanoid robots on mild cognitive impairment older adults. Int J Hum Comput Stud 145. https://doi.org/10.1016/j.ijhcs.2020.102509
Masrom M, Nadzari AS, Mahmood NHN, Zakaria WNW, Ali NRM (2016) Mobile learning in Malaysia education institutions. Issues in Information Systems 17(4):152-157
Mather CA, Gale F, Cummings EA (2017) Governing mobile technology use for continuing professional development in the Australian nursing profession. BMC Nurs 16(1):1–11
Mendel T, Toch E (2019) My mom was getting this popup: understanding motivations and processes in helping older relatives with mobile security and privacy. Proc ACM Interact, Mobile, Wearab Ubiquitous Technol 3(4):1–20
Mican D (2019) Examining Adoption of Mobile Devices and Applications among College Students in Romania. J App Comput Sci Math 13(28)
Michelson R, DeWitt A, Nagar R, Hiniker A, Yip J, Munson SA, Kientz JA (2021) Parenting in a pandemic: juggling multiple roles and managing technology use in family life during COVID-19 in the United States. In: Proceedings of the ACM on Human-Computer Interaction, 5(CSCW2), pp 1–39
Moore M, DeBruhl B (2019) Investigating university student desires and use of smartphone privacy settings. J Comput Sci Colleges 34(4):134–141
Naismith L, Lonsdale P, Vavoula GN, Sharples M (2004) Literature review in mobile technologies and learning. Future Lab Report, 11
Nordin N, Embi MA, Yunus MM (2010) Mobile learning framework for lifelong learning. Procedia Soc Behav Sci 7:130–138
Ozdamli F, Cavus N (2011) Basic elements and characteristics of mobile learning. Procedia Soc Behav Sci 28:937–942
Palacio RR, Acosta CO, Cortez J, Morán AL (2017) Usability perception of different video game devices in elderly users. Univ Access Inf Soc 16(1):103–113
Palalas A, Wark N (2020) The relationship between mobile learning and self-regulated learning: A systematic review. Australas J Educ Technol 36(4):151–172. https://doi.org/10.14742/ajet.5650
Sarrab M, Baghdadi Y, Al-Shihi H, Bourdoucen H (2016) A model for mobile learning non-functional requirement elicitation. Int J Mobile Learn Org 10(3):129–158
Seah D (2020) Using Kahoot in law school: differentiated instruction for working adults with diverse learning abilities. Int J Mobile Learn Org 14(1):36–48
Sevkli AZ, Motiwalla L, Abdulkarem HF (2017) The design and implementation of a context-aware mobile hadith learning system. Int J Mobile Learn Org 11(4):295–313
Sharma SK, Sarrab M, Al-Shihi H (2017) Development and validation of mobile learning acceptance measure. Interact Learn Environ 25(7):847–858
Shuib L, Ghani NA, Elaish MM, Yadegaridehkordi E (2019) Mobile English language learning (MELL): a literature review. Educ Rev 71(2):257–276. https://doi.org/10.1080/00131911.2017.1382445
Shukla S (2020) M-learning adoption of management students’: a case of India. Educ Inf Technol 26:1–32
Sin F, Berger S, Kim I-J, Yoon D (2021) Digital social interaction in older adults during the COVID-19 pandemic. Proc ACM Human-Comput Interact 5(CSCW2):1–20
Stewart AJ, Ostrove JM, Helson R (2001) Middle aging in women: patterns of personality change from the 30s to the 50s. J Adult Dev 8(1):23–37
Sundgren M (2017) Blurring time and place in higher education with bring your own device applications: a literature review. Educ Inf Technol 22(6):3081–3119
Swanson JA (2020) Assessing the Effectiveness of the Use of Mobile Technology in a Collegiate Course: A Case Study in M-learning. Technol Knowl Learn 25(2):389, 06//
Tan E, Law R (2016) mLearning as a softer visitor management approach for sustainable tourism. J Sustain Tour 24(1):132, 01//
Thongsri N, Shen L, Bao Y, Alharbi IM (2018) "Integrating UTAUT and UGT to explain behavioural intention to use M-learning: a developing country’s perspective," Journal of Systems and Information Technology
Traxler J (2009) Current state of mobile learning. Mobile Learning: Trans del Educ Train 1:9–24
Tu Y, Zou D, Zhang R (2020) A comprehensive framework for designing and evaluating vocabulary learning apps from multiple perspectives. Int J Mobile Learn Org 14(3):370–397
Vacher M, Aman F, Rossato S, Portet F, Lecouteux B (2019) Making emergency calls more accessible to older adults through a hands-free speech interface in the house. ACM Transact Acces Comput (TACCESS) 12(2):1–25
Venkataraman JB, Ramasamy S (2018) Factors influencing mobile learning: a literature review of selected journal papers. Int J Mob Learn Org 12:99–112
Vicente P, Lopes I (2016) Attitudes of older mobile phone users towards mobile phones. Communications 41(1):71–86
Wan Z, Bao L, Gao D, Toch E, Xia X, Mendel T, Lo D (2019) Appmod: helping older adults manage mobile security with online social help. Proc ACM Interact, Mobile, Wearab Ubiquitous Technol 3(4):1–22
Wang Y, Matz-Costa C, Miller J, Carr DC, Kohlbacher F (2018) Uses and gratifications sought from mobile phones and loneliness among japanese midlife and older Adults: a Mediation Analysis. Innov Aging 2(3)
Wardaszko M, Podgórski B (2017) Mobile learning game effectiveness in cognitive learning by adults: a comparative study. Simul Gaming 48(4):435–454
Wong CY, Ibrahim R, Hamid TA, Mansor EI (2018) Usability and design issues of smartphone user interface and mobile apps for older adults. In: International Conference on User Science and Engineering. Springer, pp 93–104
Chapter Google Scholar
Yaffe MJ, Stewart MA (1984) The problems and concerns of middle age. Can Fam Physician 30:1089–1093
Yang H-L, Lin S-L (2019) The reasons why elderly mobile users adopt ubiquitous mobile social service. Comput Hum Behav 93:62–75
Zainal A, Ahmad NA, Razak FHA, Nordin A (2017) Older adults’ requirements of Islamic Mobile applications: a multimethod exploration. Adv Sci Lett 23(5):4236–4240. https://doi.org/10.1166/asl.2017.8335
Zhang R, Zou D (2020) Influential factors of working adults' perceptions of mobile-assisted vocabulary learning with multimedia annotations. Int J Mobile Learn Org 14(4):533–548
Download references
Acknowledgments
We would like to thank you to Universiti Teknikal Malaysia Melaka (UTeM) for sponsoring the corresponding author in this research numbered UTeM.02.13.04/500-4/12/16/1/2(91)
Conflict of interest
The authors declare that there is no conflict of interest regarding the publication of this paper.
Availability of data and materials
The datasets analyzed during the current study are available from the corresponding author on reasonable request.
The corresponding author is sponsored by Universiti Teknikal Malaysia Melaka (UTeM) numbered UTeM.02.13.04/500-4/12/16/1/2(91) to pursue her PhD degree in these studies.
Author information
Authors and affiliations.
Department of Software Engineering, Faculty of Computer Science and Information Technology, Universiti Malaya, 50603, Kuala Lumpur, Malaysia
Syahida Mohtar, Nazean Jomhari & Mumtaz Begum Mustafa
Department of Al-Quran and Al-Hadith, Academy of Islamic Studies, Universiti Malaya, 50603, Kuala Lumpur, Malaysia
Zulkifli Mohd Yusoff
You can also search for this author in PubMed Google Scholar
Corresponding author
Correspondence to Syahida Mohtar .
Additional information
Publisher’s note.
Springer Nature remains neutral with regard to jurisdictional claims in published maps and institutional affiliations.
Rights and permissions
Reprints and permissions
About this article
Mohtar, S., Jomhari, N., Mustafa, M.B. et al. Mobile learning: research context, methodologies and future works towards middle-aged adults – a systematic literature review. Multimed Tools Appl (2022). https://doi.org/10.1007/s11042-022-13698-y
Download citation
Received : 02 August 2021
Revised : 17 June 2022
Accepted : 15 August 2022
Published : 20 August 2022
DOI : https://doi.org/10.1007/s11042-022-13698-y
Share this article
Anyone you share the following link with will be able to read this content:
Sorry, a shareable link is not currently available for this article.
Provided by the Springer Nature SharedIt content-sharing initiative
- Mobile application
- Self-directed learning
- Middle-aged adults
- Methodology
- Find a journal
- Publish with us
- Track your research
- Skip to main content
- Skip to search
- Skip to footer
Products and Services

Configuration engineer
Ben Harting
"I decided to make a career change when I was twenty-four. I chose to pursue Cisco Certifications because I knew it would put me in the best position to start a career in networking."
How it all began
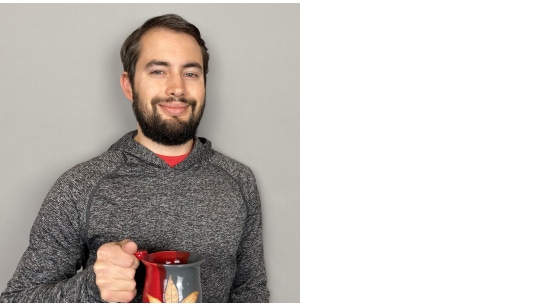
Certifications can improve your ability to do your job
My dad is a systems administrator, so I grew up around computers. Just watching him tinker with them was cool. However, when I went to college, I earned a bachelor’s degree in philosophy. Next, I got a teaching credential, then taught elementary school for a few years. But when I turned 24, I decided I wanted a career change. So, I picked up a book on TCP/IP networking, started studying it, and realized that I really enjoyed it. My dad wasn’t into networking that much, so I didn’t know much about it, and got into it more organically. I considered pursuing Cisco certifications right away since the idea of learning about networking led straight to these credentials. I took a networking class at a local community college, then obtained my Cisco CCNA certification.
I enjoy system administration, I’ve gained communications skills, and I’ve learned tech skills, in Microsoft active directory systems, administration, and networking virtualization. General troubleshooting is a big part of my job, too. Most importantly, I’ve gained the flexibility to work around difficulties and learn new technologies. Just being able to keep up with the constant change in technologies is incredibly valuable.

Certifications

Columbus, OH

Reading Hiking Learning new tech
What does having a Cisco Certification mean to you?
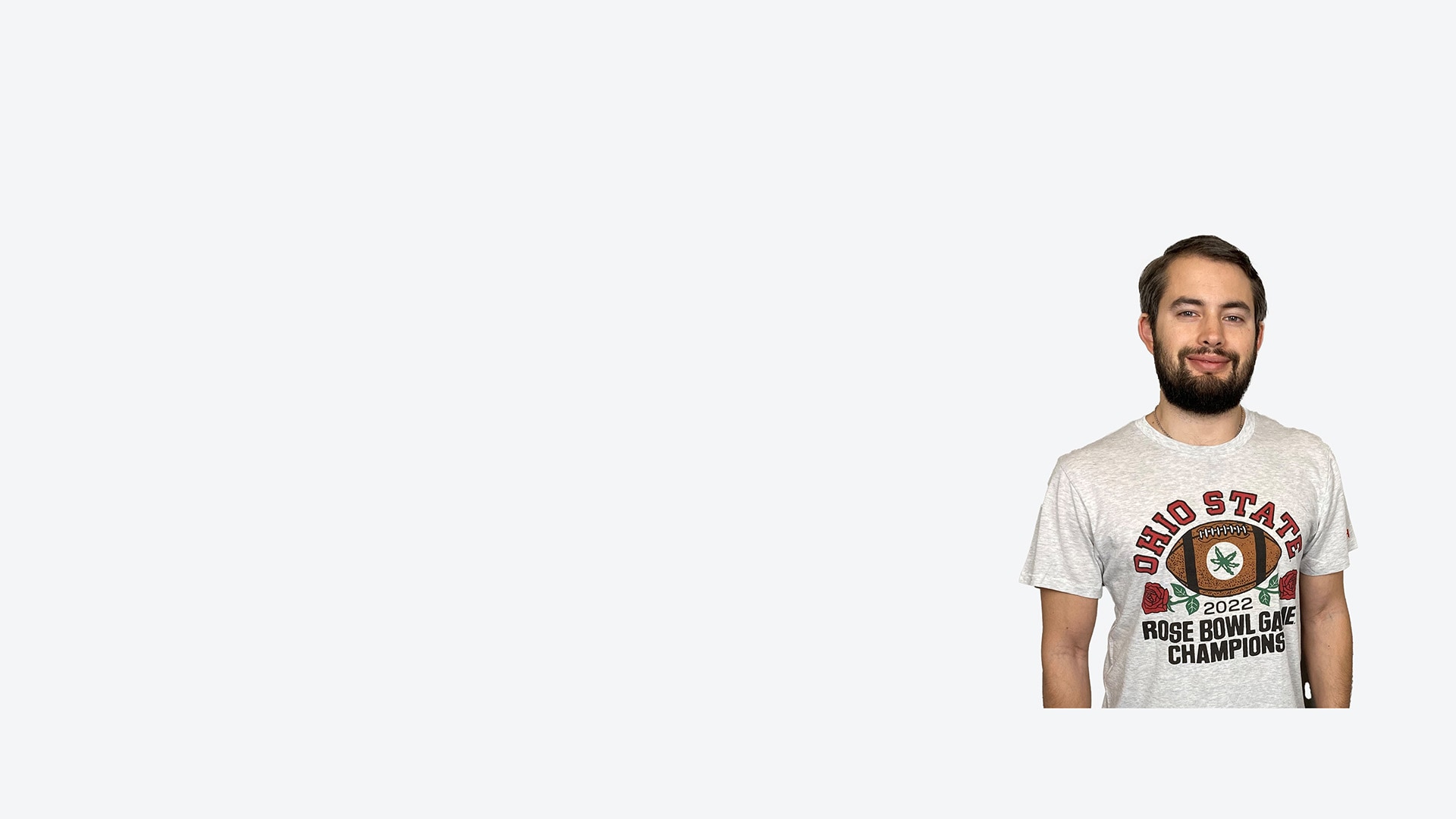
"I’ve gained more knowledge and different skill sets. I’ve opened myself up for more opportunities. And it’s a validation of me and what I’ve learned."
The biggest challenge was where to start, there where a lot of options. A lot of them involved going back to school or spending a lot of money. CCNA was the most cost-effective, and it would put me in the best position to start a career. Cisco is a leader in that. I took a bootcamp course at the local community college that lasted 8 weeks in total.
I was already in my job in IT in tier 1 support, help desk and the CCNA Certification was a catalyst for my first promotion as a second tier support security.

What would you tell your younger self?
Definitely try different things and go all in on whatever interests you the most.
What would you tell a friend?
I’d tell them to go for it. It will open up a lot of opportunities for them. They’ll have all the knowledge they need to go forward in their career.
Ben's journey
Career path.
Current role
Configuration engineers work on systems and network administration.
Previous role
IT Help Desk Support Primary School Educator
Certification path
Most recent certification
Cisco Certified Network Associate (CCNA) certification is the first step toward a career in IT Networking. The CCNA exam covers networking fundamentals, IP services, security fundamentals, automation and programmability.
Read more certification success stories
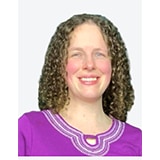
"The guidance I gained from earning the certification helped— in near real time—to determine what was happening on the job, when I became an SOC operator."
Network security analyst CyberOps Associate

Yasser Auda
"You can do anything. You just need to decide to do it, have the will to do it, and never give up. Be confident in yourself and stop the barriers in your mind."
Network security architect CCNA, CCNP Enterprise, CCNP Security, CyberOps Associate, CCIE Enterprise Infrastructure, Cisco Certified DevNet Associate, Cisco Certified DevNet Professional

Olivia Wolf
"The knowledge that I’ve got from studying for those certifications gave me the confidence that I’ll always be able to get a job if I need to."
Systems engineer CCNA, CCNP Enterprise, DevNet Associate
Share your Cisco Certification Success Story
Has earning a Cisco certification positively changed your life or career, or both? Do you think your Cisco certification story would help encourage other people to earn their Cisco certification? If so, we want to talk to you!

IMAGES
COMMENTS
Artificial intelligence (AI) techniques have grown rapidly in recent years in the context of computing with smart mobile phones that typically allows the devices to function in an intelligent manner. Popular AI techniques include machine learning and deep learning methods, natural language processing, as well as knowledge representation and expert systems, can be used to make the target mobile ...
Abstract — Mobile Cloud Computing (MCC) is an emerging field. Due to the wide usage of mobile devices and variety of applications, mobile cloud computing becomes a necessary part for mobile ...
Explore the latest full-text research PDFs, articles, conference papers, preprints and more on MOBILE COMPUTING. Find methods information, sources, references or conduct a literature review on ...
The MobiCASE 2020 proceedings address topics in in mobile computing and services, mobile applications and technologies that improve the mobile eco-system. ... held in Shanghai, China, in September 2020. The conference was held virtually due to the COVID-19 pandemic. The 15 full papers were carefully reviewed and selected from 49 submissions ...
The academic justification for the topic "AI in mobile computing under the human factor background" is evident in the growing interest and research output in this field (Van Maanen et al., 2005).A comparison of the existing literature in terms of volume of publications in recent years highlights the increasing importance and relevance of this interdisciplinary area.
The rise of the fifth generation of mobile wireless communications (5G) is driving significant scientific and technological progress in the area of mobile systems and networks. This first appearance of the new Mobile Communications and Networks Series addresses some of the most significant aspects of 5G networks, providing key insights into relevant system and network design challenges, as ...
The Internet of Things (IoT)-centric concepts like augmented reality, high-resolution video streaming, self-driven cars, smart environment, e-health care, etc. have a ubiquitous presence now. These applications require higher data-rates, large bandwidth, increased capacity, low latency and high throughput. In light of these emerging concepts, IoT has revolutionized the world by providing ...
research outlook containing an ensemble of promising research directions for MEC. A. Mobile Computing for 5G: From Clouds to Edges In the past decade, the popularity of mobile devices and the exponential growth of mobile Internet traffic have been driving the tremendous advancements in wireless communications and networking.
Together with an explosive growth of the mobile application and emerging of cloud computing concept, mobile cloud computing (MCC) has been introduced to be a potential technology for mobile services. This paper gives a survey of MCC, which helps general readers have an overview of the MCC including the definition, architecture, and applications ...
1) Framework: cloud computing systems actually can be considered as a collection of different services, thus the framework of cloud computing is divided into three layers, which are infrastructure layer, platform layer, and application layer (see Fig. 2). Fig. 2: The Framework of Cloud Computing.
Google is committed to realizing the potential of the mobile web to transform how people interact with computing technology. Google engineers and researchers work on a wide range of problems in mobile computing and networking, including new operating systems and programming platforms (such as Android and ChromeOS); new interaction paradigms ...
MobiCom, Leading Venue for Mobile Computing and Wireless Networking Research, Goes Virtual. New York, NY, September 17, 2020 — The Association for Computing Machinery's Special Interest Group on Mobility of Systems, Users, Data and Computing (SIGMOBILE) will hold its International Conference on Mobile Computing and Networking (MobiCom 2020 ...
Smart computing: Research trends and perspectives: Special Issue On Selected Papers from IEEE SMARTCOMP 2020 Last update 22 March 2022 Smart computing is based on the synergistic combination of advances in Sensor-based technologies, the Internet of Things (IoT), Cyber-Physical Systems, Edge cloud computing, Big Data analytics, Machine Learning ...
The advancement of mobile computing and the Internet have played a central role in the development of the current digital age. ... in this paper, we focus on mobile data science and corresponding intelligent apps, where the most popular AI techniques including machine learning and deep learning methods, the concept of natural language ...
Driven by the visions of Internet of Things and 5G communications, recent years have seen a paradigm shift in mobile computing, from the centralized Mobile Cloud Computing towards Mobile Edge Computing (MEC). The main feature of MEC is to push mobile computing, network control and storage to the network edges (e.g., base stations and access points) so as to enable computation-intensive and ...
ABSTRACT. In today's world, the swift increase of utilizing mobile services and simultaneously discovering of the cloud. computing services, made the Mobile Cloud Computing (MCC) selected as a ...
Mobile cloud computing (MCC) is an emerging concept that is gaining popularity in the IT sector. It is a significant topic of debate because it is being discussed as one of the most important trends for the future. After the COVID-19 pandemic, we saw the rapid emergence of mobile computing, which also created massive hype over mobile application usage. This survey paper's introduction is a ...
The paper is organized as follows. In Section 3, the background of fog computing is introduced, which includes a review of cloudlet, mobile cloud computing, mobile-edge computing, and fog computing architecture.Related works are summarised in 4, which reviews the recent articles on fog computing. Section 5 discusses the security threats of fog computing, focusing more on the most troubling and ...
Wireless Communications and Mobile Computing. Volume 2020, Issue 1 8853468. Research Article. ... So far, the research community has not determined whether it can successfully automate the feature extraction step for classification. Therefore, the automatic and accurate recognition of fraudulent phone calls has become a challenge, and this is ...
Mobile Cloud Computing (MCC) which combines mobile computing and cloud computing, has become one of the industry buzz words and a major discussion thread in the IT world since 2009. As MCC is still at the early stage of development, it is necessary to grasp a thorough understanding of the technology in order to point out the direction of future research. With the latter aim, this paper ...
This brings out a great business and research opportunity in mobile cloud November-December-2020 computing (MCC). This paper first discusses the market trend and related business driving forces and opportunities. ... been a focused subject in both academic and industry. As Computing mobile apps become more sophisticated, they III. Mobile Cloud ...
This study aims to synthesise the findings of research on cloud computing adoption and use in libraries. This systematic literature review is based on Preferred Reporting Items for Systematic Revie...
T-Mobile Delivers Industry-Leading Growth in Customers, Service Revenues and Profitability in Q2, Raises 2024 Customer and Cash Flow Guidance July 31, 2024 | 15 min read Business Press Release
The rapid advance of mobile computing technology and wireless networking, there is a significant increase of mobile subscriptions. This drives a strong demand for mobile cloud applications and services for mobile device users. This brings out a great business and research opportunity in mobile cloud computing (MCC). This paper first discusses the market trend and related business driving ...
Siwei Zhang is first author of JAMIA paper. Posted by duthip1 on Tuesday, August 13, 2024 in News.. Congratulations to PhD candidate Siwei Zhang, alumnus Nicholas Strayer (PhD 2020; now at Posit), senior biostatistician Yajing Li, and assistant professor Yaomin Xu on the publication of "PheMIME: an interactive web app and knowledge base for phenome-wide, multi-institutional multimorbidity ...
Mobile computing devices become more situated, personal, collaborative, and lifelong and these innovations will become embedded, ubiquitous, and equipped with enhanced features for rich social interaction, contextual awareness, and access to the Internet. ... The number of research papers in the year 2020 exceeds the number of research papers ...
Switches der Cisco Catalyst 9300-Serie sind auf Sicherheit, IoT und die Cloud ausgelegt. Schaffen Sie eine sichere Grundlage, die optimierte Automatisierung und Einfachheit sowie umfassende Einblicke ermöglicht.
Early career researchers may apply to one of eight Office of Science program offices: Advanced Scientific Computing Research; Biological and Environmental Research; Basic Energy Sciences; Fusion Energy Sciences; High Energy Physics; Nuclear Physics; Accelerator R&D and Production; and Isotope R&D and Production.
I enjoy system administration, I've gained communications skills, and I've learned tech skills, in Microsoft active directory systems, administration, and networking virtualization.