An official website of the United States government
Official websites use .gov A .gov website belongs to an official government organization in the United States.
Secure .gov websites use HTTPS A lock ( Lock Locked padlock icon ) or https:// means you've safely connected to the .gov website. Share sensitive information only on official, secure websites.
- Publications
- Account settings
- Advanced Search
- Journal List

The effect of sleep deprivation and restriction on mood, emotion, and emotion regulation: three meta-analyses in one
Cara c tomaso, anna b johnson, timothy d nelson.
- Author information
- Article notes
- Copyright and License information
Corresponding author. Cara C. Tomaso, Department of Psychology, 238 Burnett Hall, University of Nebraska-Lincoln, Lincoln, NE 68588-0308. Email: [email protected] .
Received 2020 Jun 5; Revised 2020 Dec 7; Collection date 2021 Jun.
This article is published and distributed under the terms of the Oxford University Press, Standard Journals Publication Model ( https://academic.oup.com/journals/pages/open_access/funder_policies/chorus/standard_publication_model )
Study Objectives
New theory and measurement approaches have facilitated nuanced investigation of how sleep loss impacts dimensions of affective functioning. To provide a quantitative summary of this literature, three conceptually related meta-analyses examined the effect of sleep restriction and sleep deprivation on mood, emotion, and emotion regulation across the lifespan (i.e. from early childhood to late adulthood).
A total of 241 effect sizes from 64 studies were selected for inclusion, and multilevel meta-analytic techniques were used when applicable.
There was a moderate, positive effect of sleep loss on negative mood ( g = 0.45), which was stronger for studies with younger samples, as well as a large, negative effect of sleep loss on positive mood ( g = −0.94). For negative mood only, studies that used total sleep deprivation had larger effect sizes than studies that restricted sleep. After correcting for publication bias, a modest but significant negative effect for sleep loss on emotion ( g = −0.11) was found; the valence of emotional stimuli did not change the direction of this effect, and type of sleep manipulation was also not a significant moderator. Finally, sleep restriction had a small, negative effect on adaptive emotion regulation ( g = −0.32), but no significant impact on maladaptive emotion regulation ( g = 0.14); all studies on adaptive emotion regulation were conducted with youth samples.
Conclusions
Sleep loss compromises optimal affective functioning, though the magnitude of effects varies across components. Findings underscore the importance of sleep for healthy affective outcomes.
Keywords: meta-analysis, sleep, mood, emotion, emotion regulation
Statement of Significance.
The current meta-analyses provide a necessary update on years of research examining the effect of sleep manipulation on positive and negative mood, emotion, and emotion regulation. Results show that sleep loss moderately increases negative mood states, especially among younger people, and has a large, diminishing effect on positive mood states. Sleep loss also has a modest blunting effect on emotional arousal and decreases adaptive management of emotions. These findings speak to the overall deleterious impact of insufficient sleep across affective outcomes, which has implications for the onset of psychopathology and overall wellbeing. Understanding how sleep loss impedes healthy affective functioning has broad relevance not only for clinical health psychology, but also for related disciplines, including cognitive and developmental psychology.
Introduction
It is well known that sleep is critical for a range of physical and psychological health outcomes, including mood [ 1 ]. In recent years, however, research examining associations between sleep and affective outcomes has expanded, requiring updated literature and meta-analytic reviews. To that end, the current meta-analyses examine the effect of sleep restriction and deprivation on three domains of affective functioning, including mood, emotion, and emotion regulation. This work builds on an older meta-analysis that focused exclusively on mood as a unidimensional construct [ 2 ] as well as more recent qualitative reviews of the impact of sleep on various aspects of affective functioning, which are informative but preclude objective conclusions [ 3 , 4 ].
Components of affective functioning: beyond semantic differences
The term affective functioning encompasses three related but conceptually distinct domains: mood, emotion, and emotion regulation [ 4 , 5 ]. Although the terms emotion and mood are often used interchangeably in everyday conversation, the differences between these constructs have been well-articulated among cognitive and clinical psychologists. It is generally agreed that the distinction is one of temporality and context [ 5 ]. Specifically, emotions are defined as relatively transient changes to an individual’s subjective experience, behavior, and physiology in response to internal or external stimuli that allow individuals to meet the demands of their environment [ 6 ]. Mood, in contrast, describes a positive or negative state of mind that is more diffuse and longer lasting and that typically emerges in the absence of an identifiable stimulus [ 4 , 7 ]. The last component of affective functioning, emotion regulation, involves a range of actions that seek to control which emotions we have, when we have them, and how we experience them [ 8 ]. Individuals may engage in adaptive strategies, such as reframing thoughts about a negative situation (i.e. cognitive reappraisal [ 6 ]), or nonadaptive strategies, such as putting off anxiety- or fear-provoking tasks (i.e. avoidance behaviors as a form of situation selection [ 6 ]). As Gross’ process model of emotion regulation [ 8 ] illustrates, there are several types of regulatory processes that unfold over time, either in anticipation of an emotion or after an emotion has already been experienced.
Watling and colleagues [ 4 ] developed a model of the interrelationships between sleep, mood, emotion, and emotion regulation, underscoring the idea that the differences between these constructs are conceptual as well as definitional. Their model suggests that sleep disruptions or insufficient sleep duration may exert a causal, unidirectional influence on emotion and emotion regulation, while the relationship between sleep outcomes and mood may be more bidirectional in nature, such that disturbances in sleep and mood reinforce each other in a process termed the sleep–mood cycle [ 9 ]. Watling et al.’s model [ 4 ] further proposes that a causal pathway exists from emotion to mood, such that experiencing more of any one particular emotion may result in more enduring mood states. Finally, emotion regulation is characterized as a moderator of mood and emotions, such that regulatory abilities reduce the intensity of negative affective experiences. In this way, sleep loss or disturbances have the potential to disrupt a particular domain of affective functioning, which in turn may affect the other components in a cascading, mutually reinforcing fashion. For example, insufficient sleep may lead to more intense negative emotions, resulting in persistent negative mood states that sleep-impaired emotion regulation abilities may be less effective at down-regulating.
Sleep and affective functioning
The component of affective functioning most studied in relation to sleep loss is mood, with most research finding that insufficient sleep increases self-reported negative mood states and decreases self-reported positive mood states [e.g. 10 , 11 ]. Building on this foundation, the literature has expanded to investigate the effect of sleep loss on other affective components, including emotion and emotion regulation. Studies focused on emotion have measured valence ratings and emotional arousal in response to positive and negative stimuli [e.g. 12 ], as well as more objective correlates of emotional responses, including vocal expressiveness [e.g. 13 ] and pupil dilation [e.g. 14 ]. However, this literature is less well-developed and has yielded somewhat inconsistent findings. Occasionally, studies have found that valence ratings of emotional stimuli trend toward becoming more negative following sleep loss [e.g. 15 ], but most studies report nonsignificant findings for both valence and/or arousal ratings [e.g. 12 , 16 , 17 ]. In contrast, studies utilizing objective metrics of emotion more consistently point to greater reactivity, particularly in response to negative stimuli, after sleep loss [e.g. 13 , 14 ]. This pattern suggests that heightened emotional responses may occur outside of individuals’ conscious awareness. Regarding emotion regulation, Palmer and Alfano [ 3 ] recently synthesized the literature on sleep and emotion regulation. Several conceptual and methodological factors make this body of work difficult to parse; for example, there has been frequent imprecise use of the term emotion regulation (e.g. applying the label when a regulatory strategy is not being assessed), and there has been greater empirical attention to emotion generation, rather than regulation, following insufficient sleep. Notwithstanding these challenges, they present a persuasive framework outlining our emerging understanding of the disruptive impact sleep loss has on adaptive identification, selection, and implementation of emotion regulation strategies.
Together, these parallel literatures speak to the importance of sleep for optimal affective functioning across all domains. However, the mechanisms underlying this association remain mostly theoretical (see Kahn et al. [ 11 ] and Simon et al. [ 18 ] for more detailed summaries of these processes). One compelling possibility is that connectivity between prefrontal control networks and the limbic system (and in particular, the amygdala) is interrupted in response to sleep loss [ 18–20 ]. This impaired top-down regulation of emotional responses may contribute to a negativity bias that amplifies responses to negative events and distorts neutral or positive events [ 21 ], leading to heightened emotional responses. Another explanation may center around rapid eye movement (REM) sleep. Based on evidence from neurocognitive studies, it has been hypothesized that increased limbic activity and greater theta wave oscillations during REM sleep contribute to therapeutic emotional processing and, by extension, support healthy affective functioning [ 22 ]. Abundant research pointing to the role of altered REM sleep in psychiatric mood disorders [ 23 ] lends further credence to this idea.
Despite our incomplete understanding of why sleep matters for affective functioning, it remains the case that characterizing the relationships between sleep loss and affective outcomes in healthy, typically developing populations has important public health implications. Sleep loss is a highly prevalent problem: roughly one-third of American adults get fewer than 7 h of sleep per night, and more than two-thirds of US high school students report sleeping less than 8 h per night [ 24 ]. The impact of sleep loss on affective outcomes is therefore likely to be widespread. Mood problems are linked with lower levels of subjective wellbeing [ 25 ], and heightened emotional reactivity and maladaptive emotion regulation abilities have been associated with the development of mood disorders [ 26 , 27 ]. Thus, what may begin as short-term consequences of occasional sleep loss may evolve into more chronic concerns, such as decreased quality of life and the development of psychopathology.
The relationship between sleep and components of affective functioning should also be considered through a developmental lens since the impact of sleep on affective outcomes, including mood, emotional experiences, and the ability to effectively manage affective responses, may have different consequences for youth. The neural networks that support emotion processing are still maturing into adolescence [ 28 ], and even typically developing adolescents without diagnosed mental health conditions exhibit increased emotional reactivity, both physiologically and behaviorally [ 29 , 30 ]. Incidence of bona fide psychopathology also tends to rise during late childhood and adolescence [ 31 ]. The psychosocial changes that are characteristic of this developmental transition, combined with changes in circadian rhythms that also interrupt sleep during adolescence, could heighten risk for affective dysfunction and related psychological disorders [ 32 , 33 ]. Risk for psychopathology aside, positive affective states are thought to be crucial for promoting all children’s overall wellbeing, with more positive moods associated with higher levels of academic performance and social and cognitive functioning [ 34 ]. In summary, although healthy sleep duration is important at all ages, it may be especially critical for children and adolescents given that the affective consequences of insufficient sleep are more costly for younger individuals.
The current study
In recent years, the literature examining sleep and affective outcomes has expanded well beyond just mood in terms of the specific constructs measured (e.g. multiple mood states, emotion, and emotion regulation) and the populations in which these constructs are studied (e.g. children and adolescents). The current study therefore aims to quantify the relationships between sleep deprivation/restriction and a range of affective outcomes across the lifespan, resulting in three conceptually related meta-analyses focused on mood, emotion, and emotion regulation.
This is an ambitious endeavor with an intentionally broad scope. There were several reasonable paths that analyses could have taken with regards to the operationalization of the independent and dependent variables. As to the former, we decided to focus on studies that manipulated sleep duration, rather than those that used measures of sleep quality, because experimental restriction/deprivation offers a more objective and uniform approach to measuring sleep. Regarding the operationalization of affective outcomes, we adhered to Watling et al.’s model [ 4 ] and other well-established frameworks (e.g. Gross [ 6 ]) to guide definitions for each construct. For mood, studies that used self-report measures of general affective states, in the absence of any specific affective stimulus, were included. Because the range of discrete mood states is extensive and tends to vary considerably across measures, measures were coded according to which valence of mood was assessed: positive (e.g. happy, content, and relaxed) or negative (e.g. angry and sad) [ 35 ]. For emotion, measures that used positive or negative visual affective stimuli to manipulate or induce brief changes in subjective emotional arousal were included. We chose to focus on subjective measures of emotion reactivity (i.e. participant-rated emotional arousal) rather than physiological responses to emotional stimuli (e.g. pupil dilation [ 14 ]). We also considered but decided against incorporating studies in which participants rated their perceptions of , rather than responses to, emotional stimuli. The decision to concentrate on subjective self-arousal reflects our goal of centering internal experiences of emotion, rather than external judgments of emotional stimuli. Finally, for emotion regulation, it was our initial intention to code this construct according to Gross’ process model [ 6 ] (i.e. situation selection, situation modification, attentional deployment, cognitive change, and response modulation). Since the majority of studies focus on cognitive change and response modulation, we instead opted to code strategies according to whether they were adaptive (i.e. effective and beneficial) or maladaptive (i.e. potentially effective in the moment but counterproductive in the long run).
It was expected that sleep loss would affect all domains of affective functioning [ 2–4 ]. This pattern would be consistent with accumulating evidence that sleep loss impacts all aspects of emotion processing [ 10 ]. Regarding mood, it was hypothesized that sleep loss would result in higher levels of negative mood states, but lower levels of positive mood states [e.g. 10 , 11 ]. Hypotheses regarding the impact of sleep loss on emotion were more tentative. Despite the preponderance of nonsignificant findings in the literature [e.g. 15 , 17 ], aggregating findings from multiple studies may amass sufficient power to detect small effects. It was cautiously expected that sleep loss would result in greater levels of emotional arousal in response to negative stimuli and lower levels of arousal in response to positive stimuli given our current understanding of sleep’s impact on emotional experiences (e.g. negativity bias [ 21 ]). Finally, for emotion regulation, it was hypothesized that sleep loss would result in reduced levels of adaptive emotion regulation strategies but increased levels of maladaptive emotion regulation strategies given evidence for the deleterious effect of sleep loss on optimal top-down regulation [e.g. 18 , 20 ]. Across all components of affective functioning, it was less clear whether there would be differences in the magnitude of effect sizes based on valence (i.e. negative vs. positive mood/emotion and adaptive vs. maladaptive emotion regulation), so specific hypotheses were not formulated. Finally, given children and adolescents’ greater sleep needs relative to adults [ 36 ] and the ongoing development of neural systems associated with affective functioning [ 28 ], it was hypothesized that younger samples’ affective functioning would be more negatively affected by sleep loss.
Study selection
Through March 2019, the authors completed systematic literature searches of empirical studies examining the relationship between sleep and the three components of affective functioning (i.e. mood, emotion, and emotion regulation) in the following databases: PsycINFO, PubMed, Google Scholar, Scopus, ERIC, and Academic Search Premier. Search keywords included the following strings: ( adolescen* OR child* OR youth OR toddler OR preschool OR pediatric ), ( sleep* OR sleep loss OR sleep deprivation OR sleep restriction ), ( executive function* OR executive control OR emotion OR emotion regulation OR emotion dysregulation OR self-regulation OR emotion reactivity OR emotion arousal OR emotion response ), ( affect* OR affective function* OR affective state ), and ( mood OR mood state ). In order to conduct a comprehensive search, keyword strings were entered together in different combinations in each database, (e.g. [ sleep* OR sleep loss OR sleep deprivation OR sleep restriction ] AND [ affect* OR affective function* OR affective state ]). To identify further studies, backward and forward reference strategies were applied to relevant articles, and the “Related Articles” and “Cited by” features in search engines were used. Finally, the reference sections of relevant articles were also hand-searched.
The following eligibility criteria were used to guide study selection:
1) Study participants had to be at least 2 years old. The capacity to comprehend and use language is a critical component of understanding emotions; between 18 and 24–30 months, children begin to talk more about their emotions [ 37 ], paralleling developmental gains in language comprehension and production. Additionally, although infants demonstrate basic emotion regulation strategies (e.g. thumb sucking, crying to get the attention of caregivers), it is not until between 2 and 3 years old that more sophisticated regulatory strategies begin to emerge [ 38 ]. However, no upper age limit was established; including a broad range of developmental stages provided the opportunity to examine age as a moderator of the relationship between sleep and each aspect of affective functioning.
2) In order to understand the relationship between affective functioning and sleep in physically and mentally healthy populations, participants had to be part of a nonclinical sample (i.e. not diagnosed with a behavioral, psychological, or sleep disorder or have a medical condition).
3) Only studies that manipulated sleep deprivation (i.e. total absence of sleep) or restriction (i.e. some reduction in total sleep duration) were eligible. Between-group designs in which participants were assigned to either a deprivation/restriction condition or a baseline sleep condition were acceptable, and repeated measures designs in which participants completed both conditions were also eligible. Studies of naturally occurring sleep deprivation/restriction because of work obligations (e.g. shiftwork), active military duty, or jet lag were ineligible. Finally, studies that included drug- and/or light-treatments in addition to manipulating sleep were not eligible.
4) Studies had to include at least one component of affective functioning as the dependent variable. Components were coded based on the following parameters:
Mood: mood assessment had to be consistent with Watling et al.’s conceptualization [ 4 ] of mood in their model of affective functioning (i.e. a state of mind that, compared with emotions, is more diffuse and longer lasting, and that typically emerges in the absence of an identifiable stimulus). Specifically, studies that used self-report measures of general mood in the absence of a stimulus intended to induce a particular affective state were included. Examples of general measures of mood include the Positive and Negative Affect Schedule [ 35 ] (PANAS) and the Profile of Mood States [ 39 ] (POMS). Other questionnaires that assessed more specific mood states, such as state anxiety (State–Trait Anxiety Inventory [ 40 ]) or depressive symptoms (e.g. Hamilton Depression Inventory [ 41 ]), were also included. Measures were coded according to positive (e.g. happy, content, and relaxed) or negative (e.g. angry and sad) [ 35 ] valence.
Emotion: similar to the approach taken with mood, the assessment of emotion had to be consistent with the conceptualization of this construct within Watling et al.’s model [ 4 ] of affective functioning and other well-established definitions of emotion (e.g. Gross [ 6 ]). Specifically, studies that used visual affective stimuli to manipulate or induce brief changes in the subjective emotional arousal were included and subsequently coded as measuring emotion. Emotion’s transiency makes its measurement in relation to sleep logistically challenging. One approach has been to assess emotion reactivity following sleep deprivation by measuring the intensity of responses to affective stimuli (e.g. emotionally charged excerpts from films or images from the International Affective Picture System [ 42 ]) via coded objective indicators of reactivity (e.g. facial expressions, verbal expressiveness, or pupillary reactivity) or subjective ratings of emotional arousal. For the current analyses, only subjective measures of emotion reactivity (i.e. participant-rated emotional arousal) were eligible for inclusion. Emotion arousal was further coded according to whether the stimuli used to generate an emotional response were intended to be positive or negative.
Emotion regulation: measures of emotion regulation had to assess attempts to control the kind of emotion experienced, when an emotion is experienced, or how an emotion is experienced [ 8 ]. One of the five points included in the process model of emotion regulation (i.e. situation selection, situation modification, attentional deployment, cognitive change, and response modulation) had to be clearly assessed and described. Examples of acceptable measures include questionnaires completed by the youth participant or their parents (e.g. the Emotion Regulation subscale of the Emotion Questionnaire [ 43 ]) and/or researcher-coded responses to task-based measures, such as having youth complete a challenging task designed to elicit an emotional response to be regulated.
5) Sufficient statistical information, including sample sizes, means, and standard deviations, had to be either included in the study or provided following email communication with the study’s authors.
6) Studies had to be published in peer-reviewed journals or available online as an unpublished thesis or dissertation, and written in English.
Literature searches resulted in 14,202 potentially relevant nonduplicate citations (sorted using Mendeley), of which 156 were retrieved for full-text analyses based on our screening of titles and abstracts. After examining these articles against our eligibility criteria, 92 articles were excluded and 64 studies were selected for inclusion (see Figure 1 for more complete information about the inclusion process). In several cases, multiple components of affective functioning were measured simultaneously within a single study (e.g. a study may have examined the effect of sleep manipulation on mood and emotion regulation [ 44 ]). The same study component was never used twice in the calculation of a pooled effect size. All procedures adhered to the guidelines established within the Preferred Reporting Items for Systematic Reviews and Meta-Analyses (PRIMSA) statement [ 45 ].
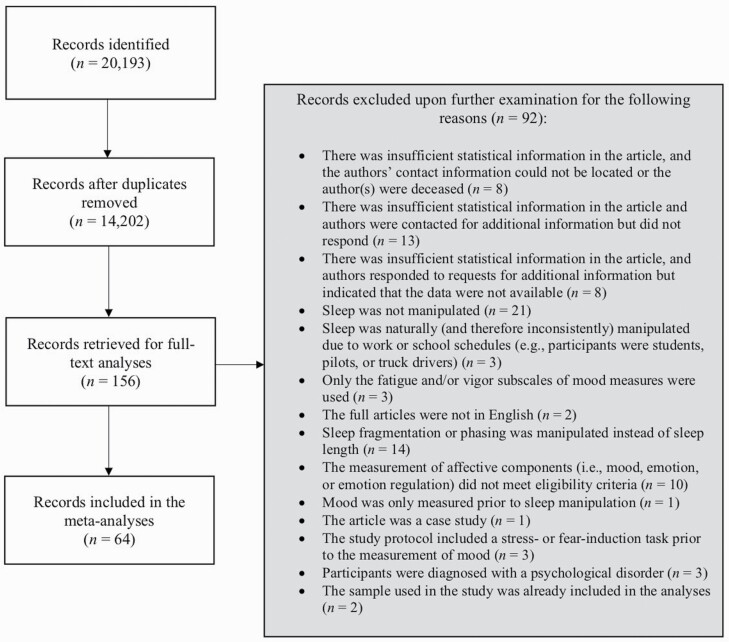
Study inclusion flowchart.
Study quality coding
Although the use of inclusion criteria accounts for many threats to internal and external validity, separately assessing study quality is a critical but often overlooked step in capturing heterogeneity in design, measures, and sampling methods used across studies selected for inclusion [ 46 ]. This step was especially important for the current analyses given the observed variability in how sleep manipulation was conducted and monitored, even among studies that satisfied inclusion criteria. Adapted from Lim and Dinges’ methodology [ 47 ] in their meta-analysis of sleep deprivation and cognitive functioning, seven factors were chosen as indices of each study’s reliability and validity. With the exception of control over confounding variables category (see below), a score of 0 or 1 was assigned in each domain and then added together to create an overall quality score for each study. Quality scores were included as a moderator in subsequent analyses. Categories of study quality included the following:
Initial equivalence.
This criterion was defined as whether efforts were made to ensure that study participants in both conditions were the same, on average, across all variables of interest. If the study was between-subjects, this was defined as use of random assignment of participants to conditions. If the study was within-subjects, this was defined as counterbalancing the order of conditions across participants.
Sampling strategy.
This criterion was defined as whether recruitment strategies were aimed at representing the general population. Was the general community recruited or was convenience sampling used (e.g. college students), and were male and female individuals represented?
Measurement adequacy.
This criterion was defined as whether appropriate, psychometrically validated measures of affective components were used. For mood, was an established measure of diffuse, general affective states used (e.g. PANAS [ 35 ] and POMS [ 39 ])? For emotion, were valid emotional stimuli used to elicit emotional arousal? For emotion regulation, were behavioral responses to emotion regulation tasks appropriately coded and/or was an established survey used to assess attempts to control which emotion was experienced or how/when an emotion was experienced?
Ongoing equivalence for the control condition.
This criterion was defined as whether efforts were made to ensure participants in the control condition experienced roughly the same procedures. Was some form of objective monitoring (e.g. experimenter observation or actigraphy) used to ensure all participants received approximately the same amount of sleep during the control condition?
Ongoing equivalence for the experimental condition.
This criterion was defined as whether efforts were made to ensure participants in the experimental condition experienced roughly the same procedures. Was some form of objective monitoring (either via experimenter observation or actigraphy) used to ensure all participants received no sleep (for deprivation protocols) or the same amount of reduced sleep (for restriction protocols) during the experimental condition?
Control of confounding variables.
This criterion was defined as whether efforts were made to eliminate the influence of confounding variables. Was caffeine use prohibited during the study? Were naps prohibited during the study? Were medications that affect sleep prohibited during the study? Were participants screened for sleep disorders? A score of 0 was assigned if none of these factors were considered, 0.5 was assigned if at least one factor was considered, and 1 was assigned if all factors (i.e. caffeine, naps, medications, and presence of sleep disorders) were considered.

Moderator coding
In addition to relevant statistical information, the following variables were coded for consideration as moderators: mean sample age as a continuous variable, the sample’s sex breakdown (i.e. percentage female vs. male), and aspects of study design, including whether studies used a within or between-groups design (0 = within groups), whether objective means such as live observation or actigraphy were used to assess sleep duration (0 = no objective monitoring), and whether sleep deprivation or restriction took place in a laboratory setting (0 = non-laboratory setting).
Coding reliability
The first author extracted relevant statistical information and coded study characteristics for all studies ( n = 64). The second author independently applied the same coding procedures to a random selection of approximately half the studies ( n = 31). For quantitative means, standard deviations, and sample ages, interrater reliability was excellent (average ICC = 0.99; ICC range = 0.97–1.00). For all categorical moderators and quality ratings, interrater reliability was also excellent (average κ = 0.94; κ range = 0.85–1.00). Inconsistencies were resolved through discussion until consensus was reached.
Effect size calculation
The formulas for deriving effect sizes for between- and within-group designs differ. However, from a statistical standpoint, effect sizes may be compared and included in the same analysis once transformed into a common metric, regardless of study design (e.g. Cohen’s d [ 48 ]). As such, studies that used between- and within-group designs were included together in subsequent meta-analytic computations, and study design was considered as a moderator. Effect sizes were first calculated in terms of Cohen’s d based on several reported statistics (primarily means, standard deviations, and sample sizes) and then adjusted to Hedges’ g using a correction factor that accounts for sample size [ 49 ]. Hedges’ g assumes that each study’s variance is an estimate, as opposed to a constant. The direction of effect sizes was uniformly adjusted across studies, such that positive effect sizes indicate increases in levels of an affective component following sleep manipulation, while negative effect sizes indicate decreases in levels of an affective components following sleep manipulation.
The formula for calculating within-group effect sizes uses the standard deviation of change scores, instead of the traditionally reported standard deviation of raw scores. If one assumes equal standard deviations between the pre- and post-intervention groups, a conversion equation may be used to estimate the within-group effect size based on the correlation ( r ) between pre- and post-intervention means. However, neither is the correlation between pre- and post-intervention means routinely reported in published studies. When this information was unavailable, r was estimated to be 0.50. This approach results in conservative estimates of effect sizes and a downward bias (toward zero) of unintended heterogeneity due to an r value that is potentially misestimated [ 50 ].
Study characteristics and effect sizes for all included studies are presented in Table 1 .
Overview of included studies
The phrase “typical sleep” is used when studies do not specify the length of sleep and describe it as typical, normal, or customary for the participants. BI-POMS, Profile of Mood States Bipolar Form [ 109 ]; BDI, Beck Depression Inventory, First Edition [ 110 ]; BRIEF, Behavior Rating Inventory of Executive Function, First Edition 111 ]; CTRS-R, Conners Teacher Rating Scale, Revised [ 112 ]; EAS Temperament Scale, Emotionality, Activity, Sociability, and Shyness Temperament Scale [ 113 ]; HARS, Hamilton Anxiety Rating Scale [ 114 ]; HDI, Hamilton Depression Inventory [ 41 ]; EQ, Emotion Questionnaire [ 43 ]; MADRAS, Montgomery–Asberg Depression Rating Scale [ 115 ]; IAPS, International Affective Picture System [ 42 ]; MASC, Mood and Anxiety Symptom Questionnaire [ 116 ]; NPRU, Naval Psychiatric Research Unit Mood Scale [ 117 ]; PANAS, Positive and Negative Affect Schedule [ 35 ]; PANAS-C, Positive and Negative Affect Schedule for Children [ 118 ]; POMS, Profile of Mood States [ 39 ]; STAI, State-Trait Anxiety Inventory [ 40 ]; STAI-C, State-Trait Anxiety Inventory for Children [ 119 ]; VAS, Visual Analog Scale.
a Babson et al. [ 53 ] used a BG study design with random assignment in the larger study; however, WG data were also reported and were used in analysis (therefore negating the random assignment).
b In Beutler et al. [ 55 ] used a larger protocol involving 2 nights of TSD. However, only the first night of TSD was used to calculate the effect size in order to maintain consistency with the majority of other studies included.
c In Cote et al. [ 60 ], the larger study randomized participants into 3 conditions (3, 5, or 8 h TIB vs. baseline 8 h TIB); WG data were obtained from the authors and were used to calculate effect sizes for 3 h TIB condition and 5 h TIB condition.
d In Kahn et al. [ 69 ], only data from the participants who completed the sleep restriction protocol (rather than the forced awakenings protocol) were used to calculate effect sizes.
e Lo et al. [ 74 ] employed a larger 2-week protocol involving control and sleep fragmentation groups, but the authors provided a subset of the data involving only the restriction group.
f Lo et al. [ 75 ] employed a larger 2-week protocol involving a control group, a sleep restriction/no-nap group, and a sleep restriction/nap group, but the authors provided a subset of the data involving only the sleep restriction/no-nap group.
g In both Studies 1 and 2, McMakin et al. [ 78 ] used a longer study design involving a second night of sleep restriction, but only the first night of restriction was used to calculate effect sizes to maintain consistency with the majority of other studies included.
h Pace-Schott et al. [ 83 ] used a BG study design with pseudo-random assignment in the larger study; however, WG data for the experimental group were provided by the author and used to calculate effect sizes.
i Peeke et al. [ 84 ] also examined associations between alcohol, sleep deprivation, and mood, but the effect size was calculated based on the conditions when alcohol was not administered.
j Reilley and Deykin [ 85 ] used a larger protocol involving 3 days of baseline sleep and 3 nights of sleep restriction (2.5 h TIB each evening). Only mood after the first baseline day and mood after the first restriction day were used to calculate the effect size in order to maintain consistency with the majority of other studies included.
k Rodgers et al.’s [ 110 ] larger protocol involved a sleep deprivation plus physical exertion condition. Only the sleep deprivation condition was used in the current analysis.
l Scott et al.’s [ 115 ] larger protocol involved a sleep deprivation plus intermittent exercise condition. Only the sleep deprivation condition was used in the current analysis.
m The sub-samples in Selvi et al. [ 94 ] were comprised of individuals with morning and evening chronotypes; effect sizes were calculated for the combined sub-samples (i.e. including both individuals with morning and evening chronotypes).
n Smith and Maben [ 96 ] also administered VAS measures of strength, mental quick-wittedness, attentiveness, incompetence, and interest, which were not included.
o Talbot et al.’s [ 118 ] sample was comprised of 3 subsets: early adolescents ( n = 20; M age = 11.50), mid-adolescents ( n = 24; M age = 14.29), and adults ( n = 20; M age = 41.10). However, only data for the whole sample (i.e. not separated by age group) were available for the PANAS-C. The PANAS-C was administered to all participants, regardless of age.
p In both SR conditions, Wu et al. [ 100 ] employed a larger 6-day protocol involving the baseline day, 4 days of SR, and 1 recovery day; however, effect sizes were calculated based on mood after baseline and mood after the first night of SR in both conditions. The SR conditions were separated by at least 10 days.
q Tempesta et al. [ 102 ] used a BG study design with random assignment in the larger study; however, WG data were also reported and were used in analysis (therefore negating the random assignment).
r Tempesta et al. [ 103 ] used a BG study design with three groups: poor sleepers, good sleepers, and sleep deprivation. Only WG data for the sleep deprivation group were used to calculate effect sizes. Additionally, although actigraphy was used to verify sleep in the poor sleepers group, the sleep deprivation group did wear actigraphs, which is reflected in the table.
s Danilewitz et al. [ 104 ] randomly assigned participants to different degrees of sleep restriction, but not all participants achieved the desired degree of restriction. The authors provided relevant statistical information for the subset of participants whose sleep was restricted by at least 20 minutes relative to baseline (verified with actigraphy), and these data were used to calculate the effect size.
t Gruber et al. [ 105 ] randomly assigned participants to a sleep extension or sleep restriction group. However, only the sleep restriction group was used in the current analyses, and the order of the baseline and sleep restriction groups was not counterbalanced, which is why the study is labeled quasi-experimental.
u Schumacher et al. [ 107 ] used an adapted version of the same task described in Miller et al. [ 106 ], in which 12 regulatory behaviors in response to an unsolvable puzzle task were coded. Only composite effect sizes for adaptive and maladaptive strategies were available for inclusion.
Meta-analytic procedures
The Metafor package [ 120 ] in R [ 121 ] was used to conduct all analyses. Pooled effect sizes were calculated according to the component of affective functioning measured:
Overall effect sizes were calculated according to valence (i.e. positive or negative), resulting in two subsets of analyses. Although all meta-analytic models presuppose a multilevel data structure [ 122 ], there was a need to account for additional interdependence within studies due to instances in which (1) single studies used measures that assess multiple positive or negative mood states (e.g. POMS [ 39 ]), (2) the same sample of participants was subjected to multiple conditions (e.g. the same sample completed sleep deprivation and restriction) [ 94 ], and/or (3) different sub-samples completed substantially similar study conditions (e.g. young and old sub-samples both completed the same sleep deprivation protocol [ 92 ]). To account for these sources of interdependence, three-level meta-analytic models (also referred to as multilevel meta-analysis) were analyzed for positive and negative mood using the function rma.mv() in R with restricted maximum likelihood estimation [ 123 ].
In multilevel meta-analysis, variance is partitioned into three levels: sampling variance around the estimation of the population effect size (Level 1), variance in effect sizes that is within studies (Level 2), and variance in effect sizes that is between studies (Level 3) [ 124 ]. In the models for positive and negative mood, loglikelihood ratio tests were used to examine whether there was statistical justification for accounting for variance at Level 2 and Level 3, respectively. Variance at Level 2 was constrained to zero to see whether its exclusion significantly deteriorated model fit, and the same procedure was applied for Level 3 variance. Then, the distribution of variance across all three levels was examined to determine which proportion was attributable to sampling error (Level 1), within study variability (Level 2), and between study variability (Level 3) [ 125 ]. Pooled effect sizes were calculated accordingly to account for each level of variance.
Traditional tests of publication bias have not been extensively studied in multilevel meta-analysis [ 123 ]. However, Egger’s regression test may be applied to multilevel models by including the standard error of effect sizes as a moderator [ 126 ]. If the intercept significantly deviates from zero, the overall relationship between the precision and the size of studies included in the analysis is considered asymmetrical and biased [ 127 ]. This method was used to examine publication bias in the studies examining positive and negative mood.
For heterogeneity tests, Q statistics were derived. Q is calculated as the weighted sum of squared differences between the individual study effects and the pooled effect across all included studies. To understand significant heterogeneity, moderators were examined using single and multivariate multilevel meta-regression analyses.
Because examination of raw effect sizes suggested that all effect sizes for emotion, regardless of the valence of the emotional stimuli, were negative, a single pooled effect size was calculated for the effect of sleep manipulation on emotion, with stimuli valence (i.e. positive or negative) considered as a moderator. As with mood, single studies of emotion often included multiple effect sizes in order to measure emotion in response to both positive and negative stimuli (i.e. 16 effect sizes nested within 8 studies). A multilevel meta-analytic approach was considered but not retained because 0% of the variance in the effect sizes was within studies.
In order to still account for heterogeneity in samples and study designs across studies, a random effects model was analyzed using the function rma() in R with restricted maximum likelihood estimation. To determine the effect of publication bias on overall effect sizes, “trim and fill” analyses were conducted [ 128 ]. Following this method, individual effect sizes are plotted against their precision (i.e. 1/SE), and the resulting plot is shaped like a funnel if no publication bias exists. If bias is noted, extreme effect sizes are trimmed, and mirror images of the trimmed studies are imputed, allowing for the calculation of adjusted overall effect sizes and confidence intervals (CIs). Finally, Q statistics were used to examine heterogeneity in effect sizes, and moderators were examined in univariate and multivariate meta-regression models.
Emotion regulation.
Separate pooled effect sizes were calculated according to whether strategies were adaptive or maladaptive, resulting in two groups of analyses. There was no need to consider the type of sleep manipulation (i.e. restriction or deprivation) as a moderator because all studies involving emotion regulation restricted rather than deprived sleep. Because single studies included multiple measures of emotion regulation, a multilevel meta-analytic approach was considered. For adaptive emotion regulation (11 effect sizes nested within 5 studies), variance within studies was already small (0.08), and constraining the variance to 0 did not decrease model fit ( p = 0.27). Similarly, for maladaptive emotion regulation (20 effect sizes nested within 5 studies), variance within studies was negligible (0.001), and constraining this variance to 0 did not decrease model fit ( p = 0.94). Therefore, random effects models for adaptive and maladaptive emotion regulation were analyzed following the same approach described above for emotion.
The effect of sleep loss on mood
Negative mood..
A total of 158 effect sizes nested within 55 studies examined negative mood states. The Level 2 variance (i.e. the variance of effect sizes within studies) was 0.14, and constraining this variance to zero decreased model fit, χ 2 (1) = 24.30, p < 0.01. As such, this level was retained. The Level 3 variance (i.e. the variance of effect sizes between studies) was 0.30, and constraining this variance to zero decreased model fit, χ 2 (1) = 329.70, p < 0.01. Therefore, this level was likewise retained. The distribution of variance partitioned across all three levels was also examined. The proportion of Level 1 variance (i.e. the variability in effect sizes around the mean estimate) was 7.45%. The proportion of Level 2 variance (i.e. the variability in effect sizes attributable to differences within studies) was 28.64%. Finally, the proportion of Level 3 variance (i.e. the variability in effect sizes attributable to differences between studies) was 63.91%. Following Egger’s regression test, no publication bias was detected ( p = 0.41).
Accounting for variance at Levels 2 and 3, the overall effect size of 0.45 was significant, indicating that sleep loss increased levels of negative mood states, SE = 0.076, t (157) = 6.10, p < 0.001, CI [0.30, 0.59]. The Q statistic was also significant, suggesting notable heterogeneity in effect sizes that was not accounted for by the effect of sleep manipulation alone, Q (157) = 1,374.73, p < 0.001. To explore the significant heterogeneity in effect sizes, several moderators were considered. Among the univariate meta-regression models, age, within vs. between-group study design, and type of sleep manipulation were significant. Older age was associated with smaller effect sizes, ES z = −0.04, SE = 0.01, t (156) = −6.06, p < 0.01, and heterogeneity decreased but remained significant, Q (156) = 1,368.66, p < 0.001. Compared with studies that restricted sleep, total sleep deprivation had larger effect sizes, ES z = 0.44, SE = 0.13, t (156) = 3.47, p < 0.01, and heterogeneity decreased but remained significant, Q (151) = 1,293.76, p < 0.001. Finally, compared with studies that used a between-groups design, studies that used a within groups design had smaller effect sizes, ES z = −0.767, SE = 0.28, t (156) = −2.72, p < 0.01, and heterogeneity decreased but remained significant, Q (156) = 1,263.74, p < 0.001. These moderators remained significant in a multivariate meta-regression model.
Positive mood.
A total of 36 effect sizes nested within 25 studies measured the effect of sleep loss on positive mood states. The Level 2 variance (i.e. the variance of effect sizes within studies) was 0.50, and constraining this variance to zero decreased model fit, χ 2 (1) = 9.76, p < 0.01. Therefore, this level of variance was retained. The Level 3 variance (i.e. the variance of effect sizes between studies) was 0.04. Constraining this variance to zero did not decrease model fit, χ 2 (1) = 1.89, p = 0.17, and this level was therefore not retained in further analyses. The distribution of variance partitioned across all three levels was examined: 6.11% was at Level 1, 86.82% was at Level 2, and 7.07% was at Level 3. Accounting for variance at Level 2 but not 3, the overall effect size of −0.94 was significant, indicating that sleep loss decreased levels of positive mood states, SE = 0.15, t (35) = −6.12, p < 0.001, CI [−1.23, −0.62]. A forest plot summarizing heterogeneity, effect size estimates, and effect size confidence intervals positive mood is displayed in Figure 2 . However, Egger’s regression test revealed significant publication bias ( p < 0.01). The Q statistic was also significant, Q (34) = 389.27, p < 0.001. To explore the significant heterogeneity among effect sizes, moderators were examined. The only significant moderator was within vs. between-groups design, such that compared with studies that used a between-groups design, studies that used a within groups design had less negative (and therefore smaller in absolute magnitude) effect sizes, ES z = 1.03, SE = 0.47, t (33) = 2.12, p < 0.01. After accounting for this variable, heterogeneity decreased but remained significant, Q (34) = 364.65, p < 0.001. Within- vs. between-group design as a moderator remained significant in a multivariate meta-regression model.
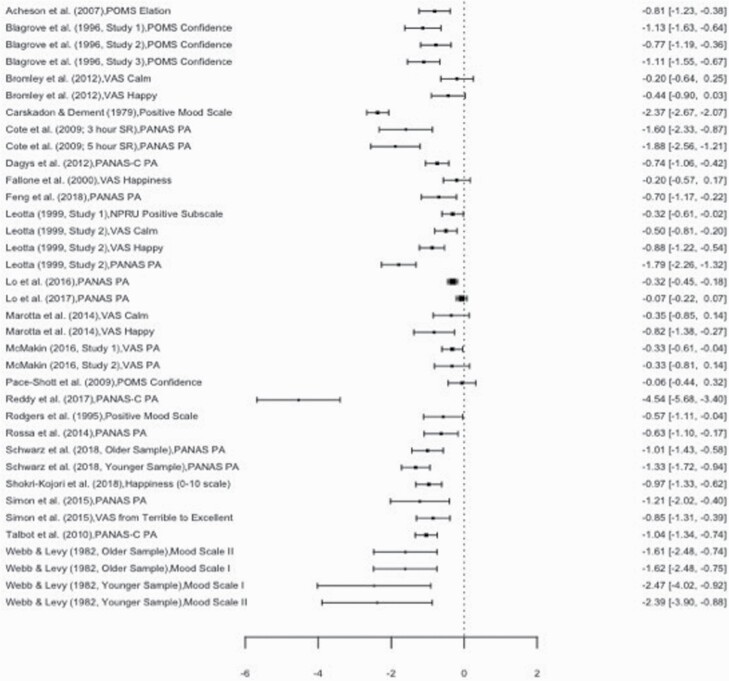
Forest plot summarizing heterogeneity, effect size estimates, and effect size confidence intervals for the effect of sleep loss on positive mood. PANAS, Positive and Negative Affect Schedule [ 35 ]; PANAS-C, Positive and Negative Affect Schedule for Children [ 118 ]; POMS, Profile of Mood States [ 39 ]; VAS, Visual Analog Scale.
The effect of sleep loss on emotion
A total of 16 effect sizes examined the effect of sleep loss on emotion. Using a random effects approach, the overall effect size of −0.18 was significant, indicating that sleep loss decreased levels of emotion, SE = 0.05, z (15) = −3.61, p < 0.01, CI [−0.28, −0.08]. A forest plot for the effect of sleep on emotion is included in Figure 3 . No significant heterogeneity in effect sizes was detected, Q (15) = 7.26, p = 0.95. The trim and fill method for addressing publication bias revealed asymmetry in the funnel plot; nine studies to the right of the mean were unmatched and imputed, resulting in a significant but smaller adjusted effect size of −0.11, SE = 0.04, z (24) = −2.40, p < 0.05, CI [−0.19, −0.02], with no significant heterogeneity, Q (24) = 18.10, p = 0.80. Consistent with the finding that there was no significant heterogeneity among effect sizes, none of the moderators (including the valence of emotional stimuli and study quality) were significant.
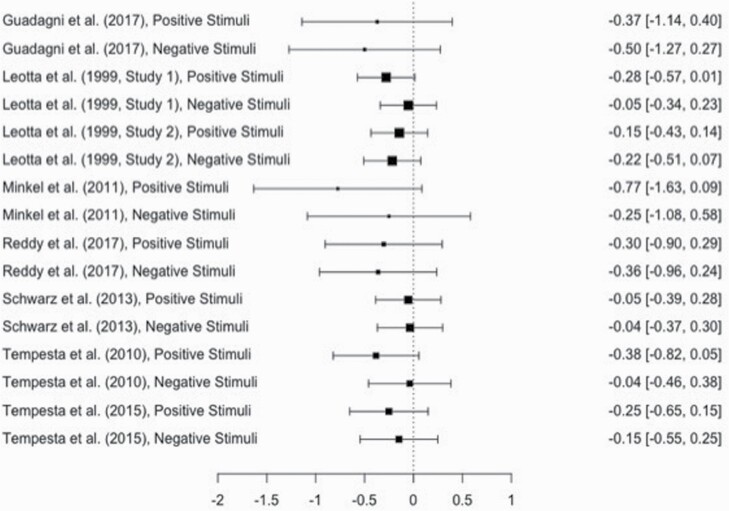
Forest plot summarizing heterogeneity, effect size estimates, and effect size confidence intervals for the effect of sleep loss on emotion.
The effect of sleep restriction on emotion regulation
Adaptive emotion regulation..
A total of 11 effect sizes examined the effect of sleep restriction on adaptive emotion regulation (range of mean ages = 2.82–15.50; grand mean = 7.68, SD = 5.14). In a random effects model, the overall effect size of −0.32 was significant, SE = 0.11, z (10) = −2.85, p < 0.01, CI [−0.54, −0.00], indicating that sleep restriction decreased adaptive emotion regulation. A forest plot for the studies examining the effect of sleep restriction on adaptive emotion regulation is in Figure 4 . The trim and fill method did not detect any asymmetry, indicating there was no publication bias. The Q statistic was significant, Q (10) = 28.96, p < 0.01. To explore the significant heterogeneity, several moderators (age, sex, within groups vs. between groups, and study quality) were examined. All studies used objective means to measure sleep and manipulated sleep in the home environment; these variables were therefore not considered as moderators. Only age emerged as significant, such that for everyone year increase in the mean age of the participants in the sample, the effect size became more negative by 0.04 units, SE = 0.017, z (9) = −2.24, p = 0.03. Heterogeneity decreased but remained significant, Q (9) = 16.15, p = 0.03. Age remained significant in a multivariate meta-regression model.
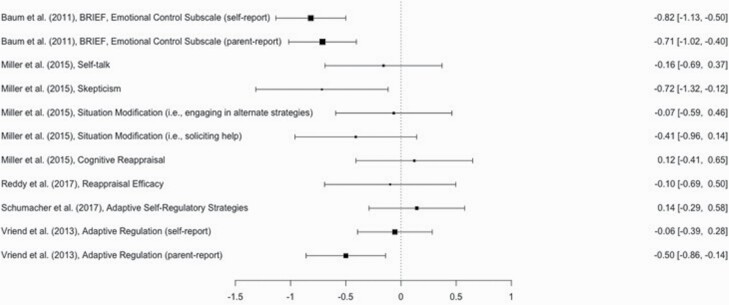
Forest plot summarizing heterogeneity, effect size estimates, and effect size confidence intervals for the effect of sleep restriction on adaptive emotion regulation. BRIEF, Behavior Rating Inventory of Executive Function, First Edition [ 111 ].
Maladaptive emotion regulation.
A total of 20 effect sizes examined the effect of sleep restriction on maladaptive emotion regulation. In a random effects model, the overall effect size of 0.14 was not significant, SE = 0.09, z (19) = 1.66, p = 0.10, CI [−0.03, 0.31]. A forest plot for the studies examining the effect of sleep restriction on maladaptive emotion regulation is in Figure 5 . The trim and fill method did not detect any asymmetry, suggesting no publication bias. The Q statistic was significant, Q (19) = 48.46, p < 0.01. To examine this significant heterogeneity, several moderators (age, sex, within groups vs. between groups, whether sleep was manipulated in a lab environment vs. home environment, and study quality) were considered. All studies used objective means to measure obtained sleep, and this variable was therefore not included. No moderators were significant. Although the majority of effect sizes were derived from studies with youth samples (range of mean ages = 2.82–14.29; grand mean = 8.30, SD = 5.05), one subsample within Talbot et al.’s study was comprised of adults ( M age = 41.20). Another random effects model was conducted without this subsample, and the overall effect size of increased modestly to 0.18 but remained nonsignificant, SE = 0.10, z (18) = 1.77, p = 0.08, CI [−0.02, 0.71]. No moderators were significant.
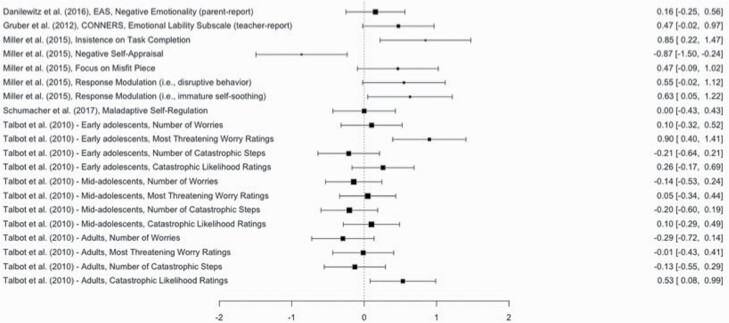
Forest plot summarizing heterogeneity, effect size estimates, and effect size confidence intervals for the effect of sleep restriction on maladaptive emotion regulation. CONNERS, Conners Teacher Rating Scale, Revised [ 112 ]; EAS, Emotionality, Activity, Sociability, and Shyness Temperament Scale [ 113 ].
The most recent comprehensive meta-analysis on sleep loss and mood was published over two decades ago [ 2 ], necessitating updated analyses that capture advances in statistical methodology, multiple dimensions of affective functioning, and more nuanced measurement of affective constructs. Thus, the current meta-analyses examined the effect of sleep loss on three domains of affective functioning (i.e. mood, emotion, and emotion regulation) [ 4 ]. In general, findings suggest that sleep loss negatively compromises affective functioning, though the strength of the effects varies across domains. This is consistent with what Simon and colleagues [ 19 ] have coined the “sleep-deficient emotional phenotype,” which is characterized by diminished socioemotional functioning via altered emotional reactivity, emotion evaluation, and emotion expression (p. 435).
As expected, sleep loss resulted in higher levels of negative mood, and the magnitude of this effect was medium ( g = 0.45). This effect was significantly greater in studies that deprived participants completely of sleep (vs. restriction), greater in studies with younger samples, and greater in studies that used a between (vs. within) groups design. Also consistent with our hypotheses, sleep loss resulted in reduced levels of positive mood, and the strength of this effect was large ( g = −0.94). The only significant moderator for positive mood was study design, which again suggested that studies that used a between (vs. within) groups design had effect sizes that were more negative and of larger magnitudes. It is unclear why this finding emerged, but it may be an artifact of the inclusion of significantly fewer studies with between-group designs, most of which produced substantial effect sizes. Interestingly, whether sleep was completely deprived or just restricted did not significantly affect the magnitude of effect sizes for positive mood. In other words, the loss of a few hours of sleep impacted positive mood to same degree that not getting any sleep impacted positive mood. It is also noteworthy that the overall effect was stronger for positive mood compared with negative mood, providing support for an observation discussed elsewhere [ 19 ]. This finding may help explain associations between sleep loss and the development of psychopathology, particularly depression, which is often characterized by anhedonia (i.e. an absence of pleasurable feelings).
Partially consistent with our hypotheses, sample age was only a significant moderator of the effect of sleep loss on negative mood, with no significant effect for positive mood. It is unclear why a similar pattern did not emerge for positive mood, but there may have been insufficient age variability to detect a significant interaction. Regardless, sleep loss appears to more strongly affect negative mood states among younger individuals relative to older individuals, perhaps because the neural processes that support affective functioning are not yet fully mature in childhood and adolescence [ 28 ] and are therefore more vulnerable to threats to optimal performance, such as sleep loss. This pattern could also stem from differences in how older and younger individuals process emotional information. For example, Wood and Kisley [ 129 ] found that negativity bias is attenuated in older people, as measured by reduced brain activity in their older subsample while viewing negative images as part of an event-related potential (ERP) study. Among its other benefits for youth, achieving healthy sleep duration appears to be especially critical for buffering negative mood states, which is important for general wellbeing and protecting against the development of psychopathology during a high-risk period [e.g. 33 ].
Our hypotheses for emotion yielded mixed support and point to a pattern of findings not immediately apparent in the individual studies. In general, research examining the impact of sleep loss on emotional arousal reports nonsignificant findings [ 12 , 16 , 17 ], yet when aggregated, there appears to be a significant negative effect for sleep loss on emotional arousal in response to both positive and negative emotional stimuli. However, the magnitude of this effect was modest ( g = −0.18) and was even further reduced after accounting for publication bias ( g = −0.11). This pattern was expected for positive stimuli given that impaired top-down regulation following sleep loss may distort interpretations of positive content. Disrupted regulatory function was also expected to amplify emotional responses to negative stimuli, yet this pattern tentatively suggests that sleep loss may result in mildly blunted responses to any emotionally salient stimuli regardless of its valence. It is possible that the impact of sleep on subjective emotional responses is more context-specific, perhaps because of sleep’s role in the functioning of the limbic system. For example, positive stimuli that are explicitly rewarding (e.g. pornographic images or desirable food or drugs) may elicit greater subjective responsivity following sleep loss due to increased reward responsivity at the neural level. In contrast, positive images that are pleasant but not necessarily rewarding (e.g. someone smiling) and less extreme negative images (e.g. someone frowning versus someone wounded in a war scene) may be subject to less limbic influence, resulting in only mild emotional responses.
These explanations remain speculative. Additionally, given our focus on internal emotional experiences, it is important to consider these results in light of the requirement that included studies assess subjective, self-rated emotional arousal. Other research not included in the current meta-analyses have examined the impact of sleep loss on emotion via other metrics, such as vocal expressiveness [ 13 ] and facial expressions [ 130 ]; these studies reveal a pattern similar to the current findings (at least for positive emotion), such that participants exhibit lower levels of positive vocal expressions when sleep has been reduced (though emotional expression is distinct from internal experiences). Further, there is a body of literature concerned with the effect of sleep loss on the interpretation and recognition of emotional stimuli, with findings suggesting that sleep loss results in biased interpretations [ 131 ]. Thus, it may be difficult for sleep-deprived individuals to accurately rate emotional arousal at the conscious level, or to even accurately process emotional information, which may explain the modest effect found here.
Emotion regulation
Partially consistent with our hypotheses, there was a small, negative effect of sleep restriction on adaptive emotion regulation ( g = −0.32), but the effect for maladaptive emotion regulation was not significant. All studies examining sleep loss and adaptive emotion regulation used youth samples (mean sample age range = 2.82–15.50), and all studies restricted rather than deprived sleep. Further, nearly all of the studies compared emotion regulation after 2–5 days of sleep restriction relative to 2–5 days of optimal sleep. With these points in mind, this pattern suggests that youth whose sleep is modestly reduced over a period of just a few days exhibit decreased levels of adaptive emotion regulation. The lack of effect for maladaptive emotion regulation implies that sleep restriction does not exacerbate baseline use of maladaptive strategies, but rather reduces use of adaptive regulatory strategies.
Interestingly, age was a significant moderator for adaptive regulation, but in the opposite direction than what was hypothesized. That is, the negative effect of sleep restriction on adaptive emotion regulation was stronger for older , not younger, youth. At first, this may seem counterintuitive, but younger children’s emotion regulation abilities may be less sophisticated relative to older children and adolescents. In other words, younger children may have fewer adaptive strategies at their disposal to be impacted by sleep loss in the first place. It is also possible that younger children may use lower levels of adaptive emotion regulation even when they are well-rested, because more adaptive strategies may not be used until more mature cognitive abilities have the chance to develop. Given that executive functioning and related cognitive abilities were not measured in many of the studies included in the current analyses, it was not possible to include this variable as a covariate or moderator. Thus, the significant interaction found here should be interpreted alongside the caveat that our approach of comparing effect sizes derived from samples ranging in mean age from 3 to 16 may overlook other factors besides sleep restriction—such as cognitive abilities—that could influence emotion regulation in children and adolescents. Regardless, these findings speak to the importance of healthy sleep duration in facilitating the use of adaptive emotion regulation strategies among youth, as such skills are critical for successfully managing strong emotions, coping with stress, and protecting against the development of psychopathology at a time when the neural systems underlying affective functioning are still maturing.
Study limitations and strengths
The current meta-analyses, while comprehensive in many respects, were not able to encompass every aspect of the growing literature on sleep and affective processes. These omissions highlight the need for more targeted individual investigations and leave the door open for smaller, more focused meta-analytic endeavors. For example, we concentrated on studies that manipulated sleep duration, which overlooks the importance of sleep quality for healthy affective functioning [ 132 , 133 ]. A future meta-analysis emphasizing sleep quality and affective outcomes would make an important contribution to the literature. Additionally, across all affective components, we chose to focus on healthy populations without behavioral or psychological disorders. Future meta-analyses could consider comparing the effect of sleep less on affective outcomes between clinical and nonclinical samples. It is also worth noting that we considered age as a continuous moderator in all analyses. As this literature expands beyond convenience samples of young adults and includes more children and adolescents, partitioning analyses into different age groups will allow for more nuanced and developmentally appropriate comparisons. The latter point is especially important for emotion regulation given that what constitutes adaptive and maladaptive regulatory strategies matures and changes over time.
Regarding emotion, we prioritized internal arousal over objective measures. This decision was partially made in light of the limited number of studies utilizing the latter approach and the fact that within these studies, there is considerable variability across measures (e.g. vocal expressiveness, facial expressions, and pupil dilation), which may limit interpretations of an overall effect size. In retrospect, however, one limitation of this approach is that one’s regulatory abilities modulate internal experiences of emotion. As such, our operationalization of emotion may be confounded with emotion regulation skills. As this literature expands, it will be informative to conduct meta-analytic comparisons of the impact of sleep loss on subjective and objective emotion or to test a meta-analytic structural equation model [ 134 ] with emotion regulation as a moderator of the effect of sleep loss on emotion. Additionally, we did not focus on interpretations of emotional information, which has also been implicated in wellbeing and emotional disorders [ 135 , 136 ]. In general, the effect of sleep on emotion has received less empirical attention, and additional research probing the effect of sleep duration and quality on a range of emotional indices, as well as eventual meta-analyses summarizing these findings, are warranted.
Regarding emotion regulation, there was insufficient variability in the types of emotion regulation measured to examine the effect of sleep loss on specific points within the process model of emotion regulation [ 8 ]. Instead, analyses focused on maladaptive vs. adaptive regulatory strategies, which may mask potential nuance in the relationship between sleep and emotion regulation. Future research examining the effect of sleep loss on specific points within the process model is recommended. Palmer and Alfano [ 3 ] also point out that there is a meaningful difference between the frequency and efficacy of emotion regulation strategy deployment. However, given the small number of studies eligible for inclusion and the corresponding limited variability in measurement approaches, our analyses do not account for this distinction. Future research on sleep and emotion regulation should consider differentiating between how often and how well various emotion regulation strategies are employed. Additionally, results should be interpreted in light of our decision to only include studies of participants without behavioral or psychological disorders, which are often characterized by emotion regulation deficits. Although we still detected a small, negative effect of sleep restriction on adaptive emotion regulation among youth, the regulatory capacity of psychologically healthy children may be better able to withstand the effects of sleep loss.
When interpreting findings across each set of analyses, several limitations must be considered. First, a meta-analysis is only as robust as the studies it includes. Despite establishing clear inclusion criteria, there remained substantial variability in the methodology of included studies, particularly with regards to when components of affective functioning were measured in relation to sleep loss, where sleep loss took place, and the duration of restful sleep achieved during the control condition. (For a comprehensive presentation of all study characteristics and methodology, refer to Table 1 .) To capture differences in methodological rigor, quality scores were calculated for each study and were considered as a moderator in each set of analysis (though no significant interactions emerged for any set of analyses). Notable heterogeneity between effect sizes remained even after accounting for study quality and other methodological differences, particularly for studies examining mood.
Second, the relative dearth of experimental research examining the effects of sleep loss on affective functioning among youth somewhat limits the interpretation of nonsignificant age interactions for positive mood and emotion. Most samples included were comprised of young adults, highlighting the need to devote more research attention to the consequences of sleep loss at different stages of development. Third, the overwhelming majority of samples were predominantly white, precluding examination of racial/ethnic identity as a moderator. Consistent with the minority stress model, people of color and Black people in particular experience health disparities that extend to sleep outcomes [ 137 ] and psychological wellbeing [ 138 ]. Improved efforts to understand the affective consequences of sleep loss among minority groups, as well as the mechanisms explaining these associations, are absolutely critical for facilitating better physical and mental health outcomes among people of color.
Fourth, the standard deviation of change scores or correlations between pre- and post-scores (at least one is needed for calculating effect sizes for within-group studies) were not provided in any of the published studies and were only available when authors responded to requests for additional information. This may have biased the calculation of some individual effect sizes. Fifth, publication bias was detected in the analysis for positive mood. Unfortunately, methods for correcting for publication bias in the context of multilevel meta-analysis remain speculative [ 123 ] and were therefore not applied, meaning that some caution is necessary when interpreting the overall effect size for positive mood. Sixth, although the decision to only include studies that manipulated sleep duration was made strategically to ease interpretation and to provide more confidence in the findings relative to self-reported sleep duration, other sleep outcomes, such as sleep variability and sleep quality, were not included. Therefore, these results should be considered in light of the strong likelihood that factors beyond sleep duration are also likely to impact affective functioning. Additionally, given the comprehensive scope of the current analyses, mood states were categorized broadly into positive or negative, which overlooks the differential impact of sleep manipulation on specific moods (e.g. excited, happy, annoyed, and sad). Likewise, focusing on responses to positive and negative visual emotional stimuli overlooks other categories of emotional triggers (e.g. irritating sounds [ 139 ]; fearful situations, like shocks [ 140 ]) but eases interpretability.
Finally, the current set of analyses are not appropriate for testing the directionality of the proposed relationships between sleep, mood, emotion, and emotion regulation outlined in Watling et al.’s conceptual model [ 4 ], which states that although sleep outcomes likely affect all domains of affective functioning, these effects may be mutually reinforcing. In addition to proposing unique effects of sleep on mood, emotion, and emotion regulation, the model posits that (1) a causal pathway exists from emotion to mood and (2) emotion regulation moderates both emotional experiences and mood. The effect of sleep loss on just one of these domains could therefore cascade across all aspects of affective functioning, in which impact on one domain could have a domino effect on other components. While these hypothesized paths are not testable in this meta-analytic context, it is nonetheless notable that among the significant effects found for mood, emotion, and adaptive emotion regulation, the strongest effect to emerge was for mood, with only a very small effect for emotion. It seems unlikely that the small effect of sleep loss on emotion would in turn impact mood, though more experimental research explicitly testing this path is needed. Additionally, our findings suggest that the moderating role of emotion regulation may be more likely to operate through decreases in adaptive emotion regulation in particular. Further research is needed to examine interrelations between these constructs.
Despite these limitations, the current analyses go well beyond extant meta-analytic work and narrative reviews to provide a quantitative summary of years of research in the field of sleep and affective functioning. First, the analyses are grounded in a strong theoretical framework [ 4 ], which provides a rationale for identifying the unique relationships between sleep loss and respective dimensions of affective functioning. Further, multilevel meta-analytic techniques were used when applicable, and the analyses accounted for the valence of mood states and emotional stimuli, as well as whether emotion regulation strategies were adaptive or maladaptive. Consideration of these factors revealed that sleep loss differentially impacts components of affective functioning, exerting stronger effects on positive mood compared with negative mood, a modest effect on responses to both positive and negative emotional stimuli, and a small but notable effect on adaptive emotion regulation. Additionally, meta-regression revealed that with regards to negative mood, younger individuals may be more vulnerable to the effects of sleep loss. In light of the alarming prevalence of insufficient sleep [ 24 ] combined with the importance of optimal affective functioning for psychological wellbeing [ 25–27 ], public health agencies as well as medical and psychological professionals have a responsibility to prioritize efforts to make healthy sleep duration a reality for all.
Acknowledgments
We would like to thank the authors who kindly provided additional information and raw data from their studies.
This work was supported by grants from the National Institute of Diabetes and Digestive and Kidney Diseases of the National Institutes of Health under award numbers F31DK122636, R01DK125651, and R01DK116693. The content is solely the responsibility of the authors and does not necessarily represent the official views of the National Institutes of Health.
Disclosure statement
Financial disclosure: None.
Nonfinancial disclosure: None.
- †. Denotes a study included in the meta-analysis.
- 1. Wong ML, et al. . The interplay between sleep and mood in predicting academic functioning, physical health and psychological health: a longitudinal study. J Psychosom Res. 2013;74(4):271–277. [ DOI ] [ PubMed ] [ Google Scholar ]
- 2. Pilcher JJ, et al. . Effects of sleep deprivation on performance: a meta-analysis. Sleep. 1996;19(4):318–326. [ DOI ] [ PubMed ] [ Google Scholar ]
- 3. Palmer CA, et al. . Sleep and emotion regulation: an organizing, integrative review. Sleep Med Rev. 2017;31:6–16. [ DOI ] [ PubMed ] [ Google Scholar ]
- 4. Watling J, et al. . Sleep loss and affective functioning: more than just mood. Behav Sleep Med. 2017;15(5):394–409. [ DOI ] [ PubMed ] [ Google Scholar ]
- 5. Gray E, et al. . Emotion, mood, and temperament: similarities, differences, and a synthesis. In: Payne RL, Cooper C, eds., Emotions at Work: Theory, Research and Applications for Management. West Sussex: John Wiley & Sons; 2001: 21–44. [ Google Scholar ]
- 6. Gross JJ. Emotion regulation: current status and future prospects. Psychol Inq. 2015;26(1):1–26. [ Google Scholar ]
- 7. Beedie CJ, et al. . Distinctions between emotion and mood. Cogn Emot. 2005;19(6):847–878. [ Google Scholar ]
- 8. Gross JJ. Emotion regulation: conceptual and empirical foundations. In: Gross JJ, ed. Handbook of Emotion Regulation. New York, NY: Guilford Press; 2014: 3–20. [ Google Scholar ]
- 9. Harvey AG. Insomnia, psychiatric disorders, and the transdiagnostic perspective. Curr Dir Psychol Sci. 2008;17(5):299–303. [ Google Scholar ]
- 10. Cote KA, et al. . The role of sleep in processing emotional information. In: Dringenberg HC, ed. Handbook of Sleep Research. 1st ed. London: Academic Press; 2019: 505–518. [ Google Scholar ]
- 11. Kahn M, et al. . Sleep and emotions: bidirectional links and underlying mechanisms. Int J Psychophysiol. 2013;89(2):218–228. [ DOI ] [ PubMed ] [ Google Scholar ]
- 12. Schwarz JF, et al. . Shortened night sleep impairs facial responsiveness to emotional stimuli. Biol Psychol. 2013;93(1):41–44. † [ DOI ] [ PubMed ] [ Google Scholar ]
- 13. McGlinchey EL, et al. . The effect of sleep deprivation on vocal expression of emotion in adolescents and adults. Sleep. 2011;34(9):1233–1241. [ DOI ] [ PMC free article ] [ PubMed ] [ Google Scholar ]
- 14. Franzen PL, et al. . Sleep deprivation alters pupillary reactivity to emotional stimuli in healthy young adults. Biol Psychol. 2009;80(3):300–305. [ DOI ] [ PMC free article ] [ PubMed ] [ Google Scholar ]
- 15. Reddy R, et al. . Impact of sleep restriction versus idealized sleep on emotional experience, reactivity and regulation in healthy adolescents. J Sleep Res. 2017;26(4):516–525. † [ DOI ] [ PubMed ] [ Google Scholar ]
- 16. Minkel J, et al. . Emotional expressiveness in sleep-deprived healthy adults. Behav Sleep Med. 2011;9(1):5–14. † [ DOI ] [ PMC free article ] [ PubMed ] [ Google Scholar ]
- 17. Leotta CR. Effects of Acute Sleep Restriction on Affective [dissertation]. Providence, RI: Brown University; 1999. † [ Google Scholar ]
- 18. Simon EB, et al. . Losing neutrality: the neural basis of impaired emotional control without sleep. J Neurosci. 2015;35(38):13194–13205. † [ DOI ] [ PMC free article ] [ PubMed ] [ Google Scholar ]
- 19. Ben Simon E, et al. . Sleep loss and the socio-emotional brain. Trends Cogn Sci. 2020;24(6):435–450. [ DOI ] [ PubMed ] [ Google Scholar ]
- 20. Gujar N, et al. . Sleep deprivation amplifies reactivity of brain reward networks, biasing the appraisal of positive emotional experiences. J Neurosci. 2011;31(12): 4466–4474. [ DOI ] [ PMC free article ] [ PubMed ] [ Google Scholar ]
- 21. Gobin CM, et al. . Poor sleep quality is associated with a negative cognitive bias and decreased sustained attention. J Sleep Res. 2015;24(5):535–542. [ DOI ] [ PubMed ] [ Google Scholar ]
- 22. Walker MP, et al. . Overnight therapy? The role of sleep in emotional brain processing. Psychol Bull. 2009;135(5):731–748. [ DOI ] [ PMC free article ] [ PubMed ] [ Google Scholar ]
- 23. Palagini L, et al. . REM sleep dysregulation in depression: state of the art. Sleep Med Rev. 2013;17(5):377–390. [ DOI ] [ PubMed ] [ Google Scholar ]
- 24. Sleep Health. CDC.gov. https://www.cdc.gov/nchs/fastats/sleep-health.htm . 2018. Accessed October 18, 2019.
- 25. Diener E, et al. . Personality, culture, and subjective well-being: emotional and cognitive evaluations of life. Annu Rev Psychol. 2003;54:403–425. [ DOI ] [ PubMed ] [ Google Scholar ]
- 26. Bylsma LM, et al. . A meta-analysis of emotional reactivity in major depressive disorder. Clin Psychol Rev. 2008;28(4):676–691. [ DOI ] [ PubMed ] [ Google Scholar ]
- 27. Gross JJ, et al. . Emotion, emotion regulation, and psychopathology: an affective science perspective. Clin Psychol Sci. 2014;2(4):387–401. [ Google Scholar ]
- 28. McRae K, et al. . The development of emotion regulation: an fMRI study of cognitive reappraisal in children, adolescents and young adults. Soc Cogn Affect Neurosci. 2012;7(1):11–22. [ DOI ] [ PMC free article ] [ PubMed ] [ Google Scholar ]
- 29. Casey BJ, et al. . The adolescent brain. Ann NY Acad Sci. 2008;11(24):111–126. [ DOI ] [ PMC free article ] [ PubMed ] [ Google Scholar ]
- 30. Somerville LH, et al. . Developmental neurobiology of cognitive control and motivational systems. Curr Opin Neurobiol. 2010;20(2):236–241. [ DOI ] [ PMC free article ] [ PubMed ] [ Google Scholar ]
- 31. Dahl RE, et al. . Heightened stress responsiveness and emotional reactivity during pubertal maturation: implications for psychopathology. Dev Psychopathol. 2009;21(1):1–6. [ DOI ] [ PubMed ] [ Google Scholar ]
- 32. Hagenauer MH, et al. . Adolescent changes in the homeostatic and circadian regulation of sleep. Dev Neurosci. 2009;31(4):276–284. [ DOI ] [ PMC free article ] [ PubMed ] [ Google Scholar ]
- 33. Tarokh L, et al. . Sleep in adolescence: physiology, cognition and mental health. Neurosci Biobehav Rev. 2016;70:182–188. [ DOI ] [ PMC free article ] [ PubMed ] [ Google Scholar ]
- 34. Davis M, et al. . Focusing on the positive: a review of the role of child positive affect in developmental psychopathology. Clin Child Fam Psychol Rev. 2014;17(2):97–124. [ DOI ] [ PubMed ] [ Google Scholar ]
- 35. Watson D, et al. . Development and validation of brief measures of positive and negative affect: the PANAS scales. J Pers Soc Psychol. 1988;54(6):1063–1070. [ DOI ] [ PubMed ] [ Google Scholar ]
- 36. National Sleep Foundation recommends new sleep times. Sleepfoundation.org. https://www.sleepfoundation.org/press-release/national-sleep-foundation-recommends-new-sleep-times . 2015. Accessed October 20, 2019.
- 37. Bretherton I, et al. . Learning to talk about emotions: a functionalist perspective. Child Dev. 1986;57(3):529–548. [ Google Scholar ]
- 38. Kopp CB. Regulation of distress and negative emotions: a developmental view. Dev Psychol. 1989;25(3):343–354. [ Google Scholar ]
- 39. McNair DM, et al. . Manual for the Profile of Mood States. San Diego, CA: Educational and Industrial Testing Service; 1971. [ Google Scholar ]
- 40. Spielberger CD, et al. . Manual for the State-Trait Anxiety Inventory. Palo Alto, CA: Consulting Psychologists Press; 1983. [ Google Scholar ]
- 41. Reynolds WM, et al.. HDI Hamilton Depression Inventory: A Self-report Version of the Hamilton Depression Rating Scale (HDRS). Odessa, FL: Psychological Assessment Resources; 1995. [ Google Scholar ]
- 42. Lang PJ, et al. . International Affective Picture System (IAPS): Affective Ratings of Pictures and Instruction Manual . Technical Report A-8 . Gainesville, FL: University of Florida; 2008. [ Google Scholar ]
- 43. Rydell AM, et al. . Emotion regulation in relation to social functioning: an investigation of child self-reports. Eur J Dev Psychol. 2007;4(3):293–313. [ Google Scholar ]
- 44. Baum KT, et al. . Sleep restriction worsens mood and emotion regulation in adolescents. J Child Psychol Psychiatry. 2014;55(2):180–190. † [ DOI ] [ PMC free article ] [ PubMed ] [ Google Scholar ]
- 45. Moher D, et al. . Preferred reporting items for systematic reviews and meta-analyses: the PRISMA statement. PLoS Med. 2009;6(7):e1000097. [ DOI ] [ PMC free article ] [ PubMed ] [ Google Scholar ]
- 46. Hohn RE, et al. . Primary study quality in psychological meta-analyses: an empirical assessment of recent practice. Front Psychol. 2018;9:2667. [ DOI ] [ PMC free article ] [ PubMed ] [ Google Scholar ]
- 47. Lim J, et al. . A meta-analysis of the impact of short-term sleep deprivation on cognitive variables. Psychol Bull. 2010;136(3):375–389. [ DOI ] [ PMC free article ] [ PubMed ] [ Google Scholar ]
- 48. Borenstein M, et al. . Introduction to Meta-Analysis. West Sussex: John Wiley & Sons; 2009. [ Google Scholar ]
- 49. Hedges LV. Fitting categorical models to effect sizes from a series of experiments. J Educ Behav Stat. 1982;7(2):119–137. [ Google Scholar ]
- 50. N. Card, Ph.D., personal communication, 2014. [ Google Scholar ]
- 51. Acheson A, et al. . Effects of sleep deprivation on impulsive behaviors in men and women. Physiol Behav. 2007;91(5):579–587. † [ DOI ] [ PubMed ] [ Google Scholar ]
- 52. Aydin A, et al. . Mood and metabolic consequences of sleep deprivation as a potential endophenotype’ in bipolar disorder. J Affect Disord. 2013;150(2):284–294. † [ DOI ] [ PubMed ] [ Google Scholar ]
- 53. Babson KA, et al. . A test of the effects of acute sleep deprivation on general and specific self-reported anxiety and depressive symptoms: an experimental extension. J Behav Ther Exp Psychiatry. 2010;41(3):297–303. † [ DOI ] [ PMC free article ] [ PubMed ] [ Google Scholar ]
- 54. Bernier D, et al. . Effects of overnight sleep restriction on brain chemistry and mood in women with unipolar depression and healthy controls. J Psychiatry Neurosci. 2009;34(5):352–360. † [ PMC free article ] [ PubMed ] [ Google Scholar ]
- 55. Beutler LE, et al. . The role of activation in the effect of total sleep deprivation on depressed mood. J Clin Psychol. 2003;59(3):369–384. † [ DOI ] [ PubMed ] [ Google Scholar ]
- 56. Blagrove M. Effects of length of sleep deprivation on interrogative suggestibility. J Exp Psychol Appl. 1996;2(1):48–59. † [ Google Scholar ]
- 57. Bromley LE, et al. . Sleep restriction decreases the physical activity of adults at risk for type 2 diabetes. Sleep. 2012;35(7):977–984. † [ DOI ] [ PMC free article ] [ PubMed ] [ Google Scholar ]
- 58. Carskadon MA, et al. . Effects of total sleep loss on sleep tendency. Percept Mot Skills. 1979;48(2):495–506. † [ DOI ] [ PubMed ] [ Google Scholar ]
- 59. Clark CP, et al. . Antidepressant response to partial sleep deprivation in unipolar depression is not related to state anxiety. Depress Anxiety. 2008;25(7):E12–E14. † [ DOI ] [ PMC free article ] [ PubMed ] [ Google Scholar ]
- 60. Cote KA, et al. . Physiological arousal and attention during a week of continuous sleep restriction. Physiol Behav. 2008;95(3):353–364. † [ DOI ] [ PubMed ] [ Google Scholar ]
- 61. Cote KA, et al. . CNS arousal and neurobehavioral performance in a short-term sleep restriction paradigm. J Sleep Res. 2009;18(3):291–303. † [ DOI ] [ PubMed ] [ Google Scholar ]
- 62. Dagys N, et al. . Double trouble? The effects of sleep deprivation and chronotype on adolescent affect. J Child Psychol Psychiatry. 2012;53(6):660–667. † [ DOI ] [ PMC free article ] [ PubMed ] [ Google Scholar ]
- 63. Drake CL, et al. . Effects of rapid versus slow accumulation of eight hours of sleep loss. Psychophysiology. 2001;38(6):979–987. † [ DOI ] [ PubMed ] [ Google Scholar ]
- 64. Fallone G, et al. . Prolonged sleep restriction in 11-and 12-year-old children: effects on behavior, sleepiness, and mood. Sleep. 2000;1(13). † [ Google Scholar ]
- 65. Feng P, et al. . Sleep deprivation affects fear memory consolidation: bi-stable amygdala connectivity with insula and ventromedial prefrontal cortex. Soc Cogn Affect Neurosci. 2018;13(2):145–155. † [ DOI ] [ PMC free article ] [ PubMed ] [ Google Scholar ]
- 66. Giesbrecht T, et al. . Acute dissociation after 1 night of sleep loss. Psychol Conscious. 2013;1(S):150–159. † [ DOI ] [ PubMed ] [ Google Scholar ]
- 67. Goldstein AN, et al. . Tired and apprehensive: anxiety amplifies the impact of sleep loss on aversive brain anticipation. J Neurosci. 2013;33(26):10607–10615. † [ DOI ] [ PMC free article ] [ PubMed ] [ Google Scholar ]
- 68. Hill DW, et al. . Influence of locus of control on mood state disturbance after short-term sleep deprivation. Sleep. 1996;19(1):41–46. † [ DOI ] [ PubMed ] [ Google Scholar ]
- 69. Kahn M, et al. . Effects of one night of induced night-wakings versus sleep restriction on sustained attention and mood: a pilot study. Sleep Med. 2014;15(7):825–832. † [ DOI ] [ PubMed ] [ Google Scholar ]
- 70. Kahn-Greene ET, et al. . The effects of sleep deprivation on symptoms of psychopathology in healthy adults. Sleep Med. 2007;8(3):215–221. † [ DOI ] [ PubMed ] [ Google Scholar ]
- 71. Kaida K, et al. . Total sleep deprivation decreases flow experience and mood status. Neuropsychiatr Dis Treat. 2014;10:19–25. † [ DOI ] [ PMC free article ] [ PubMed ] [ Google Scholar ]
- 72. Labbate LA, et al. . Sleep deprivation in social phobia and generalized anxiety disorder. Biol Psychiatry. 1998;43(11):840–842. † [ DOI ] [ PubMed ] [ Google Scholar ]
- 73. Lee HJ, et al. . Auditory event-related potentials and psychological changes during sleep deprivation. Neuropsychobiology. 2004;50(1):1–5. † [ DOI ] [ PubMed ] [ Google Scholar ]
- 74. Lo JC, et al. . Cognitive performance, sleepiness, and mood in partially sleep deprived adolescents: the need for sleep study. Sleep. 2016;39(3):687–698. † [ DOI ] [ PMC free article ] [ PubMed ] [ Google Scholar ]
- 75. Lo JC, et al. . Neurobehavioral impact of successive cycles of sleep restriction with and without naps in adolescents. Sleep. 2017;40(2):1–9. † [ DOI ] [ PMC free article ] [ PubMed ] [ Google Scholar ]
- 76. Marotta A, et al. . Poor vigilance affects attentional orienting triggered by central uninformative gaze and arrow cues. Cogn Process. 2014;15(4):503–513. † [ DOI ] [ PubMed ] [ Google Scholar ]
- 77. Mastin DF, et al.. Personality as a predictor of the objective and subjective impact of sleep deprivation. Pers Individ Differ. 2005;39(8):1471–1782. † [ Google Scholar ]
- 78. McMakin DL, et al. . The impact of experimental sleep restriction on affective functioning in social and nonsocial contexts among adolescents. J Child Psychol Psychiatry. 2016;57(9):1027–1037. † [ DOI ] [ PubMed ] [ Google Scholar ]
- 79. Meney I, et al. . The effect of one night’s sleep deprivation on temperature, mood, and physical performance in subjects with different amounts of habitual physical activity. Chronobiol Int. 1998;15(4):349–363. † [ DOI ] [ PubMed ] [ Google Scholar ]
- 80. Minkel JD. Affective Consequences of Sleep Deprivation [dissertation]. Philadelphia, PA: University of Pennsylvania; 2010. † [ Google Scholar ]
- 81. Miyata S, et al.. Impaired cortical oxygenation is related to mood disturbance resulting from three nights of sleep restriction. Sleep Biol Rhythms. 2015;13(4):387–394. † [ Google Scholar ]
- 82. Motomura Y, et al. . Sleep debt elicits negative emotional reaction through diminished amygdala-anterior cingulate functional connectivity. PLoS One. 2013;8(2):e56578. † [ DOI ] [ PMC free article ] [ PubMed ] [ Google Scholar ]
- 83. Pace-Schott EF, et al. . Failure to find executive function deficits following one night’s total sleep deprivation in university students under naturalistic conditions. Behav Sleep Med. 2009;7(3):136–163. † [ DOI ] [ PMC free article ] [ PubMed ] [ Google Scholar ]
- 84. Peeke SC, et al. . Combined effects of alcohol and sleep deprivation in normal young adults. Psychopharmacology (Berl). 1980;67(3):279–287. † [ DOI ] [ PubMed ] [ Google Scholar ]
- 85. Reilly T, et al. . Effects of partial sleep loss on subjective states, psychomotor, and physical performance tests. J Hum Mov. 1983;9:157–170. † [ Google Scholar ]
- 86. Riela S. Effects of Sleep Deprivation on Sociability, Closeness, and Interdependence [dissertation]. Stony Brook, NY: Stony Brook University; 2012. † [ Google Scholar ]
- 87. Rodgers CD, et al. . Sleep deprivation: effects on work capacity, self-paced walking, contractile properties and perceived exertion. Sleep. 1995;18(1):30–38. † [ DOI ] [ PubMed ] [ Google Scholar ]
- 88. Romney L, et al. . Reduced sleep acutely influences sedentary behavior and mood but not total energy intake in normal-weight and obese women. Behav Sleep Med. 2016;14(5):528–538. † [ DOI ] [ PubMed ] [ Google Scholar ]
- 89. Rossa KR, et al. . The effects of sleep restriction on executive inhibitory control and affect in young adults. J Adolesc Health. 2014;55(2):287–292. † [ DOI ] [ PubMed ] [ Google Scholar ]
- 90. Sagaspe P, et al. . Effects of sleep deprivation on color-word, emotional, and specific Stroop interference and on self-reported anxiety. Brain Cogn. 2006;60(1):76–87. † [ DOI ] [ PubMed ] [ Google Scholar ]
- 91. Schuh-Hofer S, et al. . One night of total sleep deprivation promotes a state of generalized hyperalgesia: a surrogate pain model to study the relationship of insomnia and pain. Pain. 2013;154(9):1613–1621. † [ DOI ] [ PubMed ] [ Google Scholar ]
- 92. Schwarz J, et al. . Mood impairment is stronger in young than in older adults after sleep deprivation. J Sleep Res. 2019;28(4):e12801. † [ DOI ] [ PMC free article ] [ PubMed ] [ Google Scholar ]
- 93. Scott JP, et al. . Effects of sleep deprivation and exercise on cognitive, motor performance and mood. Physiol Behav. 2006;87(2):396–408. † [ DOI ] [ PubMed ] [ Google Scholar ]
- 94. Selvi Y, et al. . Mood changes after sleep deprivation in morningness-eveningness chronotypes in healthy individuals. J Sleep Res. 2007;16(3):241–244. † [ DOI ] [ PubMed ] [ Google Scholar ]
- 95. Shokri-Kojori E, et al. . β-Amyloid accumulation in the human brain after one night of sleep deprivation. Proc Natl Acad Sci U S A. 2018;115(17):4483–4488. † [ DOI ] [ PMC free article ] [ PubMed ] [ Google Scholar ]
- 96. Smith A, et al. . Effects of sleep deprivation, lunch, and personality on performance, mood, and cardiovascular function. Physiol Behav. 1993;54(5):967–972. † [ DOI ] [ PubMed ] [ Google Scholar ]
- 97. Talbot LS, et al. . Sleep deprivation in adolescents and adults: changes in affect. Emotion. 2010;10(6):831–841. † [ DOI ] [ PMC free article ] [ PubMed ] [ Google Scholar ]
- 98. Vardar SA, et al. . Sleep deprivation induced anxiety and anaerobic performance. J Sports Sci Med. 2007;6(4):532–537. † [ PMC free article ] [ PubMed ] [ Google Scholar ]
- 99. Webb WB, et al. . Age, sleep deprivation, and performance. Psychophysiology. 1982;19(3):272–276. † [ DOI ] [ PubMed ] [ Google Scholar ]
- 100. Wu H, et al. . Effects of sleep restriction periods on serum cortisol levels in healthy men. Brain Res Bull. 2008;77(5):241–245. † [ DOI ] [ PubMed ] [ Google Scholar ]
- 101. Guadagni V. The Effects of Sleep Deprivation and Poor Sleep Quality on Emotional Empathy: The Behavioral and Neural Mechanisms in Healthy Controls [dissertation]. Calgary: University of Calgary; 2017. † [ Google Scholar ]
- 102. Tempesta D, et al. . Lack of sleep affects the evaluation of emotional stimuli. Brain Res Bull. 2010;82(1–2):104–108. † [ DOI ] [ PubMed ] [ Google Scholar ]
- 103. Tempesta D, et al. . Emotional memory processing is influenced by sleep quality. Sleep Med. 2015;16(7):862–870. † [ DOI ] [ PubMed ] [ Google Scholar ]
- 104. Danilewitz J, et al. . The relationship between emotion regulation and executive functioning after sleep restriction in healthy preschool children. J Western Undergraduate Psychol. 2016;4(1). http://ir.lib.uwo.ca/wupj/vol4/iss1/4 . † [ Google Scholar ]
- 105. Gruber R, et al. . Impact of sleep extension and restriction on children’s emotional lability and impulsivity. Pediatrics. 2012;130(5):e1155–e1161. † [ DOI ] [ PubMed ] [ Google Scholar ]
- 106. Miller AL, et al. . Toddler’s self-regulation strategies in a challenge context are nap-dependent. J Sleep Res. 2015;24(3):279–287. † [ DOI ] [ PMC free article ] [ PubMed ] [ Google Scholar ]
- 107. Schumacher AM, et al. . Sleep moderates the association between response inhibition and self-regulation in early childhood. J Clin Child Adolesc Psychol. 2017;46(2):222–235. † [ DOI ] [ PMC free article ] [ PubMed ] [ Google Scholar ]
- 108. Vriend JL, et al. . Manipulating sleep duration alters emotional functioning and cognitive performance in children. J Pediatr Psychol. 2013;38(10):1058–1069. † [ DOI ] [ PubMed ] [ Google Scholar ]
- 109. Lorr M, et al. . Profile of Mood States Bipolar Form (POMS-BI). San Diego, CA: Educational & Industrial Testing Service; 1980. [ Google Scholar ]
- 110. Beck A, et al.. Beck Depression Inventory Manual. New York, NY: Psychological Corporation; 1987. [ Google Scholar ]
- 111. Gioia GA, et al.. BRIEF: Behavior Rating Inventory of Executive Function. Odessa, FL: Psychological Assessment Resources; 2000. [ Google Scholar ]
- 112. Conners CK, et al. . Revision and restandardization of the Conners Teacher Rating Scale (CTRS-R): factor structure, reliability, and criterion validity. J Abnorm Child Psychol. 1998;26(4):279–291. [ DOI ] [ PubMed ] [ Google Scholar ]
- 113. Buss AH, et al.. Temperament: Early developing personality traits. Hillsdale, NJ: Lawrence Erlbaum Associates, Inc.; 1984. [ Google Scholar ]
- 114. Hamilton M. The assessment of anxiety states by rating. Br J Med Psychol. 1959;32(1):50–55. [ DOI ] [ PubMed ] [ Google Scholar ]
- 115. Montgomery SA, et al. . A new depression scale designed to be sensitive to change. Br J Psychiatry. 1979;134:382–389. [ DOI ] [ PubMed ] [ Google Scholar ]
- 116. Watson D, et al. . Testing a tripartite model: I. Evaluating the convergent and discriminant validity of anxiety and depression symptom scales. J Abnorm Psychol. 1995;104(1):3–14. [ DOI ] [ PubMed ] [ Google Scholar ]
- 117. Lubin A, et al. . The recuperative effects of REM sleep and stage 4 sleep on human performance after complete sleep loss: experiment I. Psychophysiology. 1974;11(2): 133–146. [ DOI ] [ PubMed ] [ Google Scholar ]
- 118. Laurent J, et al.. A measure of positive and negative affect for children: scale development and preliminary validation. Psychol Assess. 1999;11(3):326–338. [ Google Scholar ]
- 119. Spielberger CD, et al.. Preliminary Test Manual for the State-Trait Anxiety Inventory for Children (“How-I-Feel Questionnaire”). Mountain View, CA: Consulting Psychologists Press; 1973. [ Google Scholar ]
- 120. Viechtbauer W. Conducting meta-analyses in R with the metafor package. J Stat Softw. 2010;36(3):1–48. [ Google Scholar ]
- 121. R Core Team. R: A Language and Environment for Statistical Computing. Vienna: R Foundation for Citation Statistical Computing; 2013. [ Google Scholar ]
- 122. Pastor DA, et al. . On the multilevel nature of meta-analysis: a tutorial, comparison of software programs, and discussion of analytic choices. Multivariate Behav Res. 2018;53(1):74–89. [ DOI ] [ PubMed ] [ Google Scholar ]
- 123. Assink M, et al. . Fitting three-level meta-analytic models in R: a step-by-step tutorial. Quant Meth. 2006;12(3):154–174. [ Google Scholar ]
- 124. Van den Noortgate W, et al. . Three-level meta-analysis of dependent effect sizes. Behav Res Methods. 2013;45(2):576–594. [ DOI ] [ PubMed ] [ Google Scholar ]
- 125. Cheung MWL. Modeling dependent effect sizes with three-level meta-analyses: a structural equation modeling approach. Psychol Methods. 2014;19(2):211–229. [ DOI ] [ PubMed ] [ Google Scholar ]
- 126. Habeck CW, et al. . Community-level impacts of white-tailed deer on understorey plants in North American forests: a meta-analysis. AoB PLANTS. 2015;7:119. [ DOI ] [ PMC free article ] [ PubMed ] [ Google Scholar ]
- 127. Sterne JAC, et al. . Regression methods to detect publication and other bias in meta-analysis. In: Rothstein HR, Sutton AJ, Borenstein M, eds. Publication Bias in Meta-analysis: Prevention, Assessment, and Adjustments. Chichester: John Wiley & Sons; 2005: 99–110. [ Google Scholar ]
- 128. Duval S, et al. . A nonparametric “trim and fill” method of accounting for publication bias in meta-analysis. J Am Stat Assoc. 2000;95(449):89–98. [ Google Scholar ]
- 129. Wood S, et al. . The negativity bias is eliminated in older adults: age-related reduction in event-related brain potentials associated with evaluative categorization. Psychol Aging. 2006;21(4):815–820. [ DOI ] [ PubMed ] [ Google Scholar ]
- 130. Berger RH, et al. . Acute sleep restriction effects on emotion responses in 30- to 36-month-old children. J Sleep Res. 2012;21(3):235–246. [ DOI ] [ PMC free article ] [ PubMed ] [ Google Scholar ]
- 131. Killgore WDS, et al. . Sleep deprivation impairs recognition of specific emotions. Neurobiol Sleep Circadian Rhythms. 2017;3:10–16. [ DOI ] [ PMC free article ] [ PubMed ] [ Google Scholar ]
- 132. Mauss IB, et al. . Poorer sleep quality is associated with lower emotion-regulation ability in a laboratory paradigm. Cogn Emot. 2013;27(3):567–576. [ DOI ] [ PMC free article ] [ PubMed ] [ Google Scholar ]
- 133. Triantafillou S, et al. . Relationship between sleep quality and mood: Ecological Momentary Assessment Study. JMIR Ment Health. 2019;6(3):e12613. [ DOI ] [ PMC free article ] [ PubMed ] [ Google Scholar ]
- 134. Cheung MW, et al. . Applications of meta-analytic structural equation modelling in health psychology: examples, issues, and recommendations. Health Psychol Rev. 2017;11(3):265–279. [ DOI ] [ PubMed ] [ Google Scholar ]
- 135. Penton-Voak IS, et al. . Effects of emotion perception training on mood in undergraduate students: randomised controlled trial. Br J Psychiatry. 2012;201(1):71–72. [ DOI ] [ PubMed ] [ Google Scholar ]
- 136. Shook NJ, et al. . Negativity bias in attitude learning: a possible indicator of vulnerability to emotional disorders? J Behav Ther Exp Psychiatry. 2007;38(2):144–155. [ DOI ] [ PubMed ] [ Google Scholar ]
- 137. Petrov ME, et al. . Differences in sleep between black and white adults: an update and future directions. Sleep Med. 2016;18:74–81. [ DOI ] [ PubMed ] [ Google Scholar ]
- 138. Safran MA, et al. . Mental health disparities. Am J Public Health. 2009;99(11):1962–1966. [ DOI ] [ PMC free article ] [ PubMed ] [ Google Scholar ]
- 139. Krizan Z, et al. . Sleepy anger: restricted sleep amplifies angry feelings. J Exp Psychol Gen. 2019;148(7):1239–1250. [ DOI ] [ PubMed ] [ Google Scholar ]
- 140. Menz MM, et al. . The role of sleep and sleep deprivation in consolidating fear memories. Neuroimage. 2013;75:87–96. [ DOI ] [ PubMed ] [ Google Scholar ]
- View on publisher site
- PDF (5.1 MB)
- Collections
Similar articles
Cited by other articles, links to ncbi databases.
- Download .nbib .nbib
- Format: AMA APA MLA NLM
Add to Collections

IMAGES
VIDEO