
An official website of the United States government
The .gov means it’s official. Federal government websites often end in .gov or .mil. Before sharing sensitive information, make sure you’re on a federal government site.
The site is secure. The https:// ensures that you are connecting to the official website and that any information you provide is encrypted and transmitted securely.
- Publications
- Account settings
The PMC website is updating on October 15, 2024. Learn More or Try it out now .
- Advanced Search
- Journal List
- HHS Author Manuscripts


Case 6–2020: A 34-Year-Old Woman with Hyperglycemia
Presentation of case.
Dr. Max C. Petersen (Medicine): A 34-year-old woman was evaluated in the diabetes clinic of this hospital for hyperglycemia.
Eleven years before this presentation, the blood glucose level was 126 mg per deciliter (7.0 mmol per liter) on routine laboratory evaluation, which was performed as part of an annual well visit. The patient could not recall whether she had been fasting at the time the test had been performed. One year later, the fasting blood glucose level was 112 mg per deciliter (6.2 mmol per liter; reference range, <100 mg per deciliter [<5.6 mmol per liter]).
Nine years before this presentation, a randomly obtained blood glucose level was 217 mg per deciliter (12.0 mmol per liter), and the patient reported polyuria. At that time, the glycated hemoglobin level was 5.8% (reference range, 4.3 to 5.6); the hemoglobin level was normal. One year later, the glycated hemoglobin level was 5.9%. The height was 165.1 cm, the weight 72.6 kg, and the body-mass index (BMI; the weight in kilograms divided by the square of the height in meters) 26.6. The patient received a diagnosis of prediabetes and was referred to a nutritionist. She made changes to her diet and lost 4.5 kg of body weight over a 6-month period; the glycated hemoglobin level was 5.5%.
Six years before this presentation, the patient became pregnant with her first child. Her prepregnancy BMI was 24.5. At 26 weeks of gestation, the result of a 1-hour oral glucose challenge test (i.e., the blood glucose level obtained 1 hour after the oral administration of a 50-g glucose load in the nonfasting state) was 186 mg per deciliter (10.3 mmol per liter; reference range, <140 mg per deciliter [<7.8 mmol per liter]). She declined a 3-hour oral glucose tolerance test; a presumptive diagnosis of gestational diabetes was made. She was asked to follow a meal plan for gestational diabetes and was treated with insulin during the pregnancy. Serial ultrasound examinations for fetal growth and monitoring were performed. At 34 weeks of gestation, the fetal abdominal circumference was in the 76th percentile for gestational age. Polyhydramnios developed at 37 weeks of gestation. The child was born at 39 weeks 3 days of gestation, weighed 3.9 kg at birth, and had hypoglycemia after birth, which subsequently resolved. Six weeks post partum, the patient’s fasting blood glucose level was 120 mg per deciliter (6.7 mmol per liter), and the result of a 2-hour oral glucose tolerance test (i.e., the blood glucose level obtained 2 hours after the oral administration of a 75-g glucose load in the fasting state) was 131 mg per deciliter (7.3 mmol per liter; reference range, <140 mg per deciliter). Three months post partum, the glycated hemoglobin level was 6.1%. Lifestyle modification for diabetes prevention was recommended.
Four and a half years before this presentation, the patient became pregnant with her second child. Her prepregnancy BMI was 25.1. At 5 weeks of gestation, she had an elevated blood glucose level. Insulin therapy was started at 6 weeks of gestation, and episodes of hypoglycemia occurred during the pregnancy. Serial ultrasound examinations for fetal growth and monitoring were performed. At 28 weeks of gestation, the fetal abdominal circumference was in the 35th percentile for gestational age, and the amniotic fluid level was normal. Labor was induced at 38 weeks of gestation; the child weighed 2.6 kg at birth. Neonatal blood glucose levels were reported as stable after birth. Six weeks post partum, the patient’s fasting blood glucose level was 133 mg per deciliter (7.4 mmol per liter), and the result of a 2-hour oral glucose tolerance test was 236 mg per deciliter (13.1 mmol per liter). The patient received a diagnosis of type 2 diabetes mellitus; lifestyle modification was recommended. Three months post partum, the glycated hemoglobin level was 5.9% and the BMI was 30.0. Over the next 2 years, she followed a low-carbohydrate diet and regular exercise plan and self-monitored the blood glucose level.
Two years before this presentation, the patient became pregnant with her third child. Blood glucose levels were again elevated, and insulin therapy was started early in gestation. She had episodes of hypoglycemia that led to adjustment of her insulin regimen. The child was born at 38 weeks 5 days of gestation, weighed 3.0 kg at birth, and had hypoglycemia that resolved 48 hours after birth. After the birth of her third child, the patient started to receive metformin, which had no effect on the glycated hemoglobin level, despite adjustment of the therapy to the maximal dose.
One year before this presentation, the patient became pregnant with her fourth child. Insulin therapy was again started early in gestation. The patient reported that episodes of hypoglycemia occurred. Polyhydramnios developed. The child was born at 38 weeks 6 days of gestation and weighed 3.5 kg. The patient sought care at the diabetes clinic of this hospital for clarification of her diagnosis.
The patient reported following a low-carbohydrate diet and exercising 5 days per week. There was no fatigue, change in appetite, change in vision, chest pain, shortness of breath, polydipsia, or polyuria. There was no history of anemia, pancreatitis, hirsutism, proximal muscle weakness, easy bruising, headache, sweating, tachycardia, gallstones, or diarrhea. Her menstrual periods were normal. She had not noticed any changes in her facial features or the size of her hands or feet.
The patient had a history of acne and low-back pain. Her only medication was metformin. She had no known medication allergies. She lived with her husband and four children in a suburban community in New England and worked as an administrator. She did not smoke tobacco or use illicit drugs, and she rarely drank alcohol. She identified as non-Hispanic white. Both of her grandmothers had type 2 diabetes mellitus. Her father had hypertension, was overweight, and had received a diagnosis of type 2 diabetes at 50 years of age. Her mother was not overweight and had received a diagnosis of type 2 diabetes at 48 years of age. The patient had two sisters, neither of whom had a history of diabetes or gestational diabetes. There was no family history of hemochromatosis.
On examination, the patient appeared well. The blood pressure was 126/76 mm Hg, and the heart rate 76 beats per minute. The BMI was 25.4. The physical examination was normal. The glycated hemoglobin level was 6.2%.
A diagnostic test was performed.
DIFFERENTIAL DIAGNOSIS
Dr. Miriam S. Udler: I am aware of the diagnosis in this case and participated in the care of this patient. This healthy 34-year-old woman, who had a BMI just above the upper limit of the normal range, presented with a history of hyperglycemia of varying degrees since 24 years of age. When she was not pregnant, she was treated with lifestyle measures as well as metformin therapy for a short period, and she maintained a well-controlled blood glucose level. In thinking about this case, it is helpful to characterize the extent of the hyperglycemia and then to consider its possible causes.
CHARACTERIZING HYPERGLYCEMIA
This patient’s hyperglycemia reached a threshold that was diagnostic of diabetes 1 on two occasions: when she was 25 years of age, she had a randomly obtained blood glucose level of 217 mg per deciliter with polyuria (with diabetes defined as a level of ≥200 mg per deciliter [≥11.1 mmol per liter] with symptoms), and when she was 30 years of age, she had on the same encounter a fasting blood glucose level of 133 mg per deciliter (with diabetes defined as a level of ≥126 mg per deciliter) and a result on a 2-hour oral glucose tolerance test of 236 mg per deciliter (with diabetes defined as a level of ≥200 mg per deciliter). On both of these occasions, her glycated hemoglobin level was in the prediabetes range (defined as 5.7 to 6.4%). In establishing the diagnosis of diabetes, the various blood glucose studies and glycated hemoglobin testing may provide discordant information because the tests have different sensitivities for this diagnosis, with glycated hemoglobin testing being the least sensitive. 2 Also, there are situations in which the glycated hemoglobin level can be inaccurate; for example, the patient may have recently received a blood transfusion or may have a condition that alters the life span of red cells, such as anemia, hemoglobinopathy, or pregnancy. 3 These conditions were not present in this patient at the time that the glycated hemoglobin measurements were obtained. In addition, since the glycated hemoglobin level reflects the average glucose level typically over a 3-month period, discordance with timed blood glucose measurements can occur if there has been a recent change in glycemic control. This patient had long-standing mild hyperglycemia but met criteria for diabetes on the basis of the blood glucose levels noted.
Type 1 and Type 2 Diabetes
Now that we have characterized the patient’s hyperglycemia as meeting criteria for diabetes, it is important to consider the possible types. More than 90% of adults with diabetes have type 2 diabetes, which is due to progressive loss of insulin secretion by beta cells that frequently occurs in the context of insulin resistance. This patient had received a diagnosis of type 2 diabetes; however, some patients with diabetes may be given a diagnosis of type 2 diabetes on the basis of not having features of type 1 diabetes, which is characterized by autoimmune destruction of the pancreatic beta cells that leads to rapid development of insulin dependence, with ketoacidosis often present at diagnosis.
Type 1 diabetes accounts for approximately 6% of all cases of diabetes in adults (≥18 years of age) in the United States, 4 and 80% of these cases are diagnosed before the patient is 20 years of age. 5 Since this patient’s diabetes was essentially nonprogressive over a period of at least 9 years, she most likely does not have type 1 diabetes. It is therefore not surprising that she had received a diagnosis of type 2 diabetes, but there are several other types of diabetes to consider, particularly since some features of her case do not fit with a typical case of type 2 diabetes, such as her age at diagnosis, the presence of hyperglycemia despite a nearly normal BMI, and the mild and nonprogressive nature of her disease over the course of many years.
Less Common Types of Diabetes
Latent autoimmune diabetes in adults (LADA) is a mild form of autoimmune diabetes that should be considered in this patient. However, there is controversy as to whether LADA truly represents an entity that is distinct from type 1 diabetes. 6 Both patients with type 1 diabetes and patients with LADA commonly have elevated levels of diabetes-associated autoantibodies; however, LADA has been defined by an older age at onset (typically >25 years) and slower progression to insulin dependence (over a period of >6 months). 7 This patient had not been tested for diabetes-associated autoantibodies. I ordered these tests to help evaluate for LADA, but this was not my leading diagnosis because of her young age at diagnosis and nonprogressive clinical course over a period of at least 9 years.
If the patient’s diabetes had been confined to pregnancy, we might consider gestational diabetes, but she had hyperglycemia outside of pregnancy. Several medications can cause hyperglycemia, including glucocorticoids, atypical antipsychotic agents, cancer immunotherapies, and some antiretroviral therapies and immunosuppressive agents used in transplantation. 8 However, this patient was not receiving any of these medications. Another cause of diabetes to consider is destruction of the pancreas due to, for example, cystic fibrosis, a tumor, or pancreatitis, but none of these were present. Secondary endocrine disorders — including excess cortisol production, excess growth hormone production, and pheochromocytoma — were considered to be unlikely in this patient on the basis of the history, review of symptoms, and physical examination.
Monogenic Diabetes
A final category to consider is monogenic diabetes, which is caused by alteration of a single gene. Types of monogenic diabetes include maturity-onset diabetes of the young (MODY), neonatal diabetes, and syndromic forms of diabetes. Monogenic diabetes accounts for 1 to 6% of cases of diabetes in children 9 and approximately 0.4% of cases in adults. 10 Neonatal diabetes is diagnosed typically within the first 6 months of life; syndromic forms of monogenic diabetes have other abnormal features, including particular organ dysfunction. Neither condition is applicable to this patient.
MODY is an autosomal dominant condition characterized by primary pancreatic beta-cell dysfunction that causes mild diabetes that is diagnosed during adolescence or early adulthood. As early as 1964, the nomenclature “maturity-onset diabetes of the young” was used to describe cases that resembled adult-onset type 2 diabetes in terms of the slow progression to insulin use (as compared with the rapid progression in type 1 diabetes) but occurred in relatively young patients. 11 Several genes cause distinct forms of MODY that have specific disease features that inform treatment, and thus MODY is a clinically important diagnosis. Most forms of MODY cause isolated abnormal glucose levels (in contrast to syndromic monogenic diabetes), a manifestation that has contributed to its frequent misdiagnosis as type 1 or type 2 diabetes. 12
Genetic Basis of MODY
Although at least 13 genes have been associated with MODY, 3 genes — GCK , which encodes glucokinase, and HNF1A and HNF4A , which encode hepatocyte nuclear factors 1A and 4A, respectively — account for most cases. MODY associated with GCK (known as GCK-MODY) is characterized by mild, nonprogressive hyperglycemia that is present since birth, whereas the forms of MODY associated with HNF1A and HNF4A (known as HNF1A-MODY and HNF4A-MODY, respectively) are characterized by the development of diabetes, typically in the early teen years or young adulthood, that is initially mild and then progresses such that affected patients may receive insulin before diagnosis.
In patients with GCK-MODY, genetic variants reduce the function of glucokinase, the enzyme in pancreatic beta cells that functions as a glucose sensor and controls the rate of entry of glucose into the glycolytic pathway. As a result, reduced sensitivity to glucose-induced insulin secretion causes asymptomatic mild fasting hyperglycemia, with an upward shift in the normal range of the fasting blood glucose level to 100 to 145 mg per deciliter (5.6 to 8.0 mmol per liter), and also causes an upward shift in postprandial blood glucose levels, but with tight regulation maintained ( Fig. 1 ). 13 This mild hyperglycemia is not thought to confer a predisposition to complications of diabetes, 14 is largely unaltered by treatment, 15 and does not necessitate treatment outside of pregnancy.

Key features suggesting maturity-onset diabetes of the young (MODY) in this patient were an age of less than 35 years at the diagnosis of diabetes, a strong family history of diabetes with an autosomal dominant pattern of inheritance, and hyperglycemia despite a close-to-normal body-mass index. None of these features is an absolute criterion. MODY is caused by single gene–mediated disruption of pancreatic beta-cell function. In MODY associated with the GCK gene (known as GCK-MODY), disrupted glucokinase function causes a mild upward shift in glucose levels through-out the day and does not necessitate treatment. 13 In the pedigree, circles represent female family members, squares male family members, blue family members affected by diabetes, and green unaffected family members. The arrow indicates the patient.
In contrast to GCK-MODY, the disorders HNF1A-MODY and HNF4A-MODY result in progressive hyperglycemia that eventually leads to treatment. 16 Initially, there may be a normal fasting glucose level and large spikes in postprandial glucose levels (to >80 mg per deciliter [>4.4 mmol per liter]). 17 Patients can often be treated with oral agents and discontinue insulin therapy started before the diagnosis of MODY. 18 Of note, patients with HNF1A-MODY or HNF4A-MODY are typically sensitive to treatment with sulfonylureas 19 but may also respond to glucagon-like peptide-1 receptor agonists. 20
This patient had received a diagnosis of diabetes before 35 years of age, had a family history of diabetes involving multiple generations, and was not obese. These features are suggestive of MODY but do not represent absolute criteria for the condition ( Fig. 1 ). 1 Negative testing for diabetes-associated autoantibodies would further increase the likelihood of MODY. There are methods to calculate a patient’s risk of having MODY associated with GCK , HNF1A , or HNF4A . 21 , 22 Using an online calculator ( www.diabetesgenes.org/mody-probability-calculator ), we estimate that the probability of this patient having MODY is at least 75.5%. Genetic testing would be needed to confirm this diagnosis, and in patients at an increased risk for MODY, multigene panel testing has been shown to be cost-effective. 23 , 24
DR. MIRIAM S. UDLER’S DIAGNOSIS
Maturity-onset diabetes of the young, most likely due to a GCK variant.

DIAGNOSTIC TESTING
Dr. Christina A. Austin-Tse: A diagnostic sequencing test of five genes associated with MODY was performed. One clinically significant variant was identified in the GCK gene (NM_000162.3): a c.787T→C transition resulting in the p.Ser263Pro missense change. Review of the literature and variant databases revealed that this variant had been previously identified in at least three patients with early-onset diabetes and had segregated with disease in at least three affected members of two families (GeneDx: personal communication). 25 , 26 Furthermore, the variant was rare in large population databases (occurring in 1 out of 128,844 European chromosomes in gnomAD 27 ), a feature consistent with a disease-causing role. Although the serine residue at position 263 was not highly conserved, multiple in vitro functional studies have shown that the p.Ser263Pro variant negatively affects the stability of the glucokinase enzyme. 26 , 28 – 30 As a result, this variant met criteria to be classified as “likely pathogenic.” 31 As mentioned previously, a diagnosis of GCK-MODY is consistent with this patient’s clinical features. On subsequent testing of additional family members, the same “likely pathogenic” variant was identified in the patient’s father and second child, both of whom had documented hyperglycemia.
DISCUSSION OF MANAGEMENT
Dr. Udler: In this patient, the diagnosis of GCK-MODY means that it is normal for her blood glucose level to be mildly elevated. She can stop taking metformin because discontinuation is not expected to substantially alter her glycated hemoglobin level 15 , 32 and because she is not at risk for complications of diabetes. 14 However, she should continue to maintain a healthy lifestyle. Although patients with GCK-MODY are not typically treated for hyperglycemia outside of pregnancy, they may need to be treated during pregnancy.
It is possible for a patient to have type 1 or type 2 diabetes in addition to MODY, so this patient should be screened for diabetes according to recommendations for the general population (e.g., in the event that she has a risk factor for diabetes, such as obesity). 1 Since the mild hyperglycemia associated with GCK-MODY is asymptomatic (and probably unrelated to the polyuria that this patient had described in the past), the development of symptoms of hyperglycemia, such as polyuria, polydipsia, or blurry vision, should prompt additional evaluation. In patients with GCK-MODY, the glycated hemoglobin level is typically below 7.5%, 33 so a value rising above that threshold or a sudden large increase in the glycated hemoglobin level could indicate concomitant diabetes from another cause, which would need to be evaluated and treated.
This patient’s family members are at risk for having the same GCK variant, with a 50% chance of offspring inheriting a variant from an affected parent. Since the hyperglycemia associated with GCK-MODY is present from birth, it is necessary to perform genetic testing only in family members with demonstrated hyperglycemia. I offered site-specific genetic testing to the patient’s parents and second child.
Dr. Meridale V. Baggett (Medicine): Dr. Powe, would you tell us how you would treat this patient during pregnancy?
Dr. Camille E. Powe: During the patient’s first pregnancy, routine screening led to a presumptive diagnosis of gestational diabetes, the most common cause of hyperglycemia in pregnancy. Hyperglycemia in pregnancy is associated with adverse pregnancy outcomes, 34 and treatment lowers the risk of such outcomes. 35 , 36 Two of the most common complications — fetal overgrowth (which can lead to birth injuries, shoulder dystocia, and an increased risk of cesarean delivery) and neonatal hypoglycemia — are thought to be the result of fetal hyperinsulinemia. 37 Maternal glucose is freely transported across the placenta, and excess glucose augments insulin secretion from the fetal pancreas. In fetal life, insulin is a potent growth factor, and neonates who have hyperinsulinemia in utero often continue to secrete excess insulin in the first few days of life. In the treatment of pregnant women with diabetes, we strive for strict blood sugar control (fasting blood glucose level, <95 mg per deciliter [<5.3 mmol per liter]; 2-hour postprandial blood glucose level, <120 mg per deciliter) to decrease the risk of these and other hyperglycemia-associated adverse pregnancy outcomes. 38 – 40
In the third trimester of the patient’s first pregnancy, obstetrical ultrasound examination revealed a fetal abdominal circumference in the 76th percentile for gestational age and polyhydramnios, signs of fetal exposure to maternal hyperglycemia. 40 – 42 Case series involving families with GCK-MODY have shown that the effect of maternal hyperglycemia on the fetus depends on whether the fetus inherits the pathogenic GCK variant. 43 – 48 Fetuses that do not inherit the maternal variant have overgrowth, presumably due to fetal hyperinsulinemia ( Fig. 2A ). In contrast, fetuses that inherit the variant do not have overgrowth and are born at a weight that is near the average for gestational age, despite maternal hyperglycemia, presumably because the variant results in decreased insulin secretion ( Fig. 2B ). Fetuses that inherit GCK-MODY from their fathers and have euglycemic mothers appear to be undergrown, most likely because their insulin secretion is lower than normal when they and their mothers are euglycemic ( Fig. 2D ). Because fetal overgrowth and polyhydramnios occurred during this patient’s first pregnancy and neonatal hypoglycemia developed after the birth, the patient’s first child is probably not affected by GCK-MODY.

Pathogenic variants that lead to GCK-MODY, when carried by a fetus, change the usual relationship of maternal hyperglycemia to fetal hyperinsulinemia and fetal overgrowth. GCK-MODY–affected fetuses have lower insulin secretion than unaffected fetuses in response to the same maternal blood glucose level. In a hyperglycemic mother carrying a fetus who is unaffected by GCK-MODY, excessive fetal growth is usually apparent (Panel A). Studies involving GCK-MODY–affected hyperglycemic mothers have shown that fetal growth is normal despite maternal hyperglycemia when a fetus has the maternal GCK variant (Panel B). The goal of treatment of maternal hyperglycemia when a fetus is unaffected by GCK-MODY is to establish euglycemia to normalize fetal insulin levels and growth (Panel C); whether this can be accomplished in the case of maternal GCK-MODY is controversial, given the genetically determined elevated maternal glycemic set point. In the context of maternal euglycemia, GCK-MODY–affected fetuses may be at risk for fetal growth restriction (Panel D).
In accordance with standard care for pregnant women with diabetes who do not meet glycemic targets after dietary modification, 38 , 39 the patient was treated with insulin during her pregnancies. In her second pregnancy, treatment was begun early, after hyperglycemia was detected in the first trimester. Because she had not yet received the diagnosis of GCK-MODY during any of her pregnancies, no consideration of this condition was given during her obstetrical treatment. Whether treatment affects the risk of hyperglycemia-associated adverse pregnancy outcomes in pregnant women with known GCK-MODY is controversial, with several case series showing that the birth weight percentile in unaffected neonates remains consistent regardless of whether the mother is treated with insulin. 44 , 45 Evidence suggests that it may be difficult to overcome a genetically determined glycemic set point in patients with GCK-MODY with the use of pharmacotherapy, 15 , 32 and affected patients may have symptoms of hypoglycemia when the blood glucose level is normal because of an enhanced counterregulatory response. 49 , 50 Still, to the extent that it is possible, it would be desirable to safely lower the blood glucose level in a woman with GCK-MODY who is pregnant with an unaffected fetus in order to decrease the risk of fetal overgrowth and other consequences of mildly elevated glucose levels ( Fig. 2C ). 46 , 47 , 51 In contrast, there is evidence that lowering the blood glucose level in a pregnant woman with GCK-MODY could lead to fetal growth restriction if the fetus is affected ( Fig. 2D ). 45 , 52 During this patient’s second pregnancy, she was treated with insulin beginning in the first trimester, and her daughter’s birth weight was near the 16th percentile for gestational age; this outcome is consistent with the daughter’s ultimate diagnosis of GCK-MODY.
Expert opinion suggests that, in pregnant women with GCK-MODY, insulin therapy should be deferred until fetal growth is assessed by means of ultrasound examination beginning in the late second trimester. If there is evidence of fetal overgrowth, the fetus is presumed to be unaffected by GCK-MODY and insulin therapy is initiated. 53 After I have counseled women with GCK-MODY on the potential risks and benefits of insulin treatment during pregnancy, I have sometimes used a strategy of treating hyperglycemia from early in pregnancy using modified glycemic targets that are less stringent than the targets typically used during pregnancy. This strategy attempts to balance the risk of growth restriction in an affected fetus (as well as maternal hypoglycemia) with the potential benefit of glucose-lowering therapy for an unaffected fetus.
Dr. Udler: The patient stopped taking metformin, and subsequent glycated hemoglobin levels remained unchanged, at 6.2%. Her father and 5-year-old daughter (second child) both tested positive for the same GCK variant. Her father had a BMI of 36 and a glycated hemoglobin level of 7.8%, so I counseled him that he most likely had type 2 diabetes in addition to GCK-MODY. He is currently being treated with metformin and lifestyle measures. The patient’s daughter now has a clear diagnosis to explain her hyperglycemia, which will help in preventing misdiagnosis of type 1 diabetes, given her young age, and will be important for the management of any future pregnancies. She will not need any medical follow-up for GCK-MODY until she is considering pregnancy.
FINAL DIAGNOSIS
Maturity-onset diabetes of the young due to a GCK variant.
Acknowledgments
We thank Dr. Andrew Hattersley and Dr. Sarah Bernstein for helpful comments on an earlier draft of the manuscript.
This case was presented at the Medical Case Conference.
No potential conflict of interest relevant to this article was reported.
Disclosure forms provided by the authors are available with the full text of this article at NEJM.org .
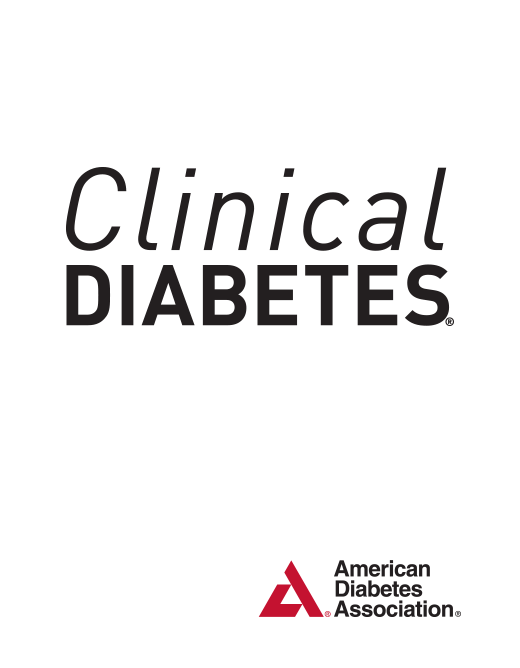
- Previous Article
- Next Article
Presentation
Clinical pearls, case study: a 36-year-old woman with type 2 diabetes and pregnancy.
- Split-Screen
- Article contents
- Figures & tables
- Supplementary Data
- Peer Review
- Open the PDF for in another window
- Cite Icon Cite
- Get Permissions
Diane M. Karl; Case Study: A 36-Year-Old Woman With Type 2 Diabetes and Pregnancy. Clin Diabetes 1 January 2001; 19 (1): 24–25. https://doi.org/10.2337/diaclin.19.1.24
Download citation file:
- Ris (Zotero)
- Reference Manager
C.M. is a 36-year-old Spanish-speaking Mexican-American woman with a 3-year history of type 2 diabetes. She was seen in her primary physician’s office because of a missed menstrual period; a pregnancy test was positive.
Her past obstetrical history included five vaginal deliveries and six miscarriages. All of her previous pregnancies occurred before the diagnosis of diabetes. Her previous medical care was in Mexico. She was never told of any glucose problem during her pregnancies, and she does not know the birth weights of her children. At the time of referral, she was 8 weeks pregnant and taking glyburide 10 mg twice daily. She was checking her blood glucose once daily in the morning with typical readings between 180 and 220 mg/dl on a plasma-referenced meter. Family history was positive for diabetes in her mother.
Her height was 62 inches, and her weight was 198 lb. Other than mild acanthosis nigricans and obesity, her physical examination was normal. She had no retinopathy and no evidence of neuropathy. Her glycosylated hemoglobin (HbA 1c ) level was 10.5% (normal <6.0%), and an office capillary blood glucose 4 h after lunch was 201 mg/dl.
She was started on insulin immediately and her glyburide was discontinued. She began monitoring her glucose before and after each meal, making daily adjustments in insulin. She received nutrition education with an appropriate calorie intake plus an emphasis on frequent smaller meals and limited carbohydrate intake. Within 1 week, her plasma glucose values were in the target range for pregnancy, but in the following week she had a spontaneous miscarriage. After her miscarriage, she discontinued insulin on her own and resumed taking glyburide 10 mg twice daily.
1. Is there a relationship between C.M.’s diabetes and her adverse obstetrical history?
2. What should have been done before her recent pregnancy to increase the odds of a favorable outcome?
3. What considerations affect the choice of therapy for her diabetes now?
In the past, most diabetic women who conceived had type 1 diabetes. Today, however, we see an increasing number of women who have preconception type 2 diabetes. One reason is the tendency for many women to delay pregnancy until a later age. Another important factor, however, is the increasing number of children and young adults, especially in minority groups, who are developing type 2 diabetes. 1
The presence of diabetes in a woman of childbearing years is a special challenge. Blood glucose control during the first 2 months of pregnancy is critical to normal organ development. Commonly, however, women do not seek medical attention until after this period of early fetal development. Many women do not yet realize they are pregnant during this important period, especially if the pregnancy is not planned, which is the situation in well over half of all pregnancies. For this reason, preconception counseling must be an important aspect of management in all diabetic women of childbearing years, regardless of whether there is an expressed desire to conceive. 2 , 3
Even though C.M.’s diabetes was diagnosed 3 years ago, the fact that she is already poorly controlled on maximal sulfonylurea treatment suggests a longer duration of diabetes. This supports the possibility that her poor obstetrical history may have been related to undiagnosed (and, therefore, uncontrolled) diabetes. Certainly during her most recent pregnancy, C.M. was poorly controlled during the critical period of organ development, possibly leading to an anomaly incompatible with fetal viability.
Comprehensive preconception counseling is now indicated for C.M. Oral diabetic medications have not been adequately studied for safety during pregnancy. Therefore, a woman who is taking oral medication and who wishes to conceive should be switched to insulin, and control should be established before she becomes pregnant. If C.M. plans another pregnancy or if she is not actively using birth control, she needs to resume insulin treatment.
Even patients whose diabetes is well controlled with diet and exercise are almost certain to require insulin during the later stages of gestation, when insulin resistance increases markedly. Preparing patients for this likelihood and teaching insulin administration as part of preconception counseling is advisable. Before pregnancy occurs is the ideal time to address any patient fears and misconceptions about insulin treatment.
For a woman of childbearing age who does not wish to become pregnant, choice of therapy can be important. Insulin resistance, almost universally present in type 2 diabetes, may be associated with decreased fertility. This is most clearly evident in polycystic ovary syndrome. 4 Oral diabetic medications that reduce insulin resistance, such as metformin and thiazolidinediones, 5 may also restore fertility. Thus, a previously infertile patient with type 2 diabetes may become unexpectedly pregnant after starting an insulin-sensitizing medication unless she is counseled regarding the need for birth control.
1. Preconception counseling is important for all women with diabetes, type 1 or type 2, who are in their childbearing years, since many pregnancies are not planned and poor glucose control early in pregnancy is associated with a higher incidence of major congenital defects.
2. Especially in minority populations, increasing numbers of women with type 2 diabetes who are treated with oral medications may be in their childbearing years. There are not adequate safety data to recommend the use of oral diabetic medications during pregnancy.
3. Oral diabetic medications that reduce insulin resistance may increase fertility in women previously unable to conceive.
Diane M. Karl, MD, is medical director of diabetes services at Adventist Health and an assistant professor of clinical medicine at Oregon Health Sciences University in Portland, Ore.
Email alerts
- Online ISSN 1945-4953
- Print ISSN 0891-8929
- Diabetes Care
- Clinical Diabetes
- Diabetes Spectrum
- Standards of Medical Care in Diabetes
- Scientific Sessions Abstracts
- BMJ Open Diabetes Research & Care
- ShopDiabetes.org
- ADA Professional Books
Clinical Compendia
- Clinical Compendia Home
- Latest News
- DiabetesPro SmartBrief
- Special Collections
- DiabetesPro®
- Diabetes Food Hub™
- Insulin Affordability
- Know Diabetes By Heart™
- About the ADA
- Journal Policies
- For Reviewers
- Advertising in ADA Journals
- Reprints and Permission for Reuse
- Copyright Notice/Public Access Policy
- ADA Professional Membership
- ADA Member Directory
- Diabetes.org
- X (Twitter)
- Cookie Policy
- Accessibility
- Terms & Conditions
- Get Adobe Acrobat Reader
- © Copyright American Diabetes Association
This Feature Is Available To Subscribers Only
Sign In or Create an Account

IMAGES
VIDEO
COMMENTS
The following case study illustrates the clinical role of advanced practice nurses in the management of a patient with type 2 diabetes.
Thomas-Dobersen D, Dobersen M: Case study: a 55-year-old man with obesity, hypertriglyceridemia, and newly diagnosed type 2 diabetes who collapsed and died.
A 47-year-old woman was found to have hyperglycemia at a health fair when a random blood glucose level was 227 mg/dL (12.6 mmol/L). Several days later, a fasting blood glucose value was 147 mg/dL (8.2 mmol/L).
Six weeks post partum, the patient’s fasting blood glucose level was 133 mg per deciliter (7.4 mmol per liter), and the result of a 2-hour oral glucose tolerance test was 236 mg per deciliter (13.1 mmol per liter). The patient received a diagnosis of type 2 diabetes mellitus; lifestyle modification was recommended.
Case 1: Failure of Metformin Monotherapy. A 68-year-old male patient with T2DM has received optimal metformin monotherapy for the last 2 years (current dose of 2000 mg/day) and tolerates the treatment well. Hypoglycemia has not been an issue to date.
Presentation. C.M. is a 36-year-old Spanish-speaking Mexican-American woman with a 3-year history of type 2 diabetes. She was seen in her primary physician’s office because of a missed menstrual period; a pregnancy test was positive. Her past obstetrical history included five vaginal deliveries and six miscarriages.