- Research article
- Open access
- Published: 04 June 2021

Coronavirus disease (COVID-19) pandemic: an overview of systematic reviews
- Israel Júnior Borges do Nascimento 1 , 2 ,
- Dónal P. O’Mathúna 3 , 4 ,
- Thilo Caspar von Groote 5 ,
- Hebatullah Mohamed Abdulazeem 6 ,
- Ishanka Weerasekara 7 , 8 ,
- Ana Marusic 9 ,
- Livia Puljak ORCID: orcid.org/0000-0002-8467-6061 10 ,
- Vinicius Tassoni Civile 11 ,
- Irena Zakarija-Grkovic 9 ,
- Tina Poklepovic Pericic 9 ,
- Alvaro Nagib Atallah 11 ,
- Santino Filoso 12 ,
- Nicola Luigi Bragazzi 13 &
- Milena Soriano Marcolino 1
On behalf of the International Network of Coronavirus Disease 2019 (InterNetCOVID-19)
BMC Infectious Diseases volume 21 , Article number: 525 ( 2021 ) Cite this article
17k Accesses
35 Citations
14 Altmetric
Metrics details
Navigating the rapidly growing body of scientific literature on the SARS-CoV-2 pandemic is challenging, and ongoing critical appraisal of this output is essential. We aimed to summarize and critically appraise systematic reviews of coronavirus disease (COVID-19) in humans that were available at the beginning of the pandemic.
Nine databases (Medline, EMBASE, Cochrane Library, CINAHL, Web of Sciences, PDQ-Evidence, WHO’s Global Research, LILACS, and Epistemonikos) were searched from December 1, 2019, to March 24, 2020. Systematic reviews analyzing primary studies of COVID-19 were included. Two authors independently undertook screening, selection, extraction (data on clinical symptoms, prevalence, pharmacological and non-pharmacological interventions, diagnostic test assessment, laboratory, and radiological findings), and quality assessment (AMSTAR 2). A meta-analysis was performed of the prevalence of clinical outcomes.
Eighteen systematic reviews were included; one was empty (did not identify any relevant study). Using AMSTAR 2, confidence in the results of all 18 reviews was rated as “critically low”. Identified symptoms of COVID-19 were (range values of point estimates): fever (82–95%), cough with or without sputum (58–72%), dyspnea (26–59%), myalgia or muscle fatigue (29–51%), sore throat (10–13%), headache (8–12%) and gastrointestinal complaints (5–9%). Severe symptoms were more common in men. Elevated C-reactive protein and lactate dehydrogenase, and slightly elevated aspartate and alanine aminotransferase, were commonly described. Thrombocytopenia and elevated levels of procalcitonin and cardiac troponin I were associated with severe disease. A frequent finding on chest imaging was uni- or bilateral multilobar ground-glass opacity. A single review investigated the impact of medication (chloroquine) but found no verifiable clinical data. All-cause mortality ranged from 0.3 to 13.9%.
Conclusions
In this overview of systematic reviews, we analyzed evidence from the first 18 systematic reviews that were published after the emergence of COVID-19. However, confidence in the results of all reviews was “critically low”. Thus, systematic reviews that were published early on in the pandemic were of questionable usefulness. Even during public health emergencies, studies and systematic reviews should adhere to established methodological standards.
Peer Review reports
The spread of the “Severe Acute Respiratory Coronavirus 2” (SARS-CoV-2), the causal agent of COVID-19, was characterized as a pandemic by the World Health Organization (WHO) in March 2020 and has triggered an international public health emergency [ 1 ]. The numbers of confirmed cases and deaths due to COVID-19 are rapidly escalating, counting in millions [ 2 ], causing massive economic strain, and escalating healthcare and public health expenses [ 3 , 4 ].
The research community has responded by publishing an impressive number of scientific reports related to COVID-19. The world was alerted to the new disease at the beginning of 2020 [ 1 ], and by mid-March 2020, more than 2000 articles had been published on COVID-19 in scholarly journals, with 25% of them containing original data [ 5 ]. The living map of COVID-19 evidence, curated by the Evidence for Policy and Practice Information and Co-ordinating Centre (EPPI-Centre), contained more than 40,000 records by February 2021 [ 6 ]. More than 100,000 records on PubMed were labeled as “SARS-CoV-2 literature, sequence, and clinical content” by February 2021 [ 7 ].
Due to publication speed, the research community has voiced concerns regarding the quality and reproducibility of evidence produced during the COVID-19 pandemic, warning of the potential damaging approach of “publish first, retract later” [ 8 ]. It appears that these concerns are not unfounded, as it has been reported that COVID-19 articles were overrepresented in the pool of retracted articles in 2020 [ 9 ]. These concerns about inadequate evidence are of major importance because they can lead to poor clinical practice and inappropriate policies [ 10 ].
Systematic reviews are a cornerstone of today’s evidence-informed decision-making. By synthesizing all relevant evidence regarding a particular topic, systematic reviews reflect the current scientific knowledge. Systematic reviews are considered to be at the highest level in the hierarchy of evidence and should be used to make informed decisions. However, with high numbers of systematic reviews of different scope and methodological quality being published, overviews of multiple systematic reviews that assess their methodological quality are essential [ 11 , 12 , 13 ]. An overview of systematic reviews helps identify and organize the literature and highlights areas of priority in decision-making.
In this overview of systematic reviews, we aimed to summarize and critically appraise systematic reviews of coronavirus disease (COVID-19) in humans that were available at the beginning of the pandemic.
Methodology
Research question.
This overview’s primary objective was to summarize and critically appraise systematic reviews that assessed any type of primary clinical data from patients infected with SARS-CoV-2. Our research question was purposefully broad because we wanted to analyze as many systematic reviews as possible that were available early following the COVID-19 outbreak.
Study design
We conducted an overview of systematic reviews. The idea for this overview originated in a protocol for a systematic review submitted to PROSPERO (CRD42020170623), which indicated a plan to conduct an overview.
Overviews of systematic reviews use explicit and systematic methods for searching and identifying multiple systematic reviews addressing related research questions in the same field to extract and analyze evidence across important outcomes. Overviews of systematic reviews are in principle similar to systematic reviews of interventions, but the unit of analysis is a systematic review [ 14 , 15 , 16 ].
We used the overview methodology instead of other evidence synthesis methods to allow us to collate and appraise multiple systematic reviews on this topic, and to extract and analyze their results across relevant topics [ 17 ]. The overview and meta-analysis of systematic reviews allowed us to investigate the methodological quality of included studies, summarize results, and identify specific areas of available or limited evidence, thereby strengthening the current understanding of this novel disease and guiding future research [ 13 ].
A reporting guideline for overviews of reviews is currently under development, i.e., Preferred Reporting Items for Overviews of Reviews (PRIOR) [ 18 ]. As the PRIOR checklist is still not published, this study was reported following the Preferred Reporting Items for Systematic Reviews and Meta-Analyses (PRISMA) 2009 statement [ 19 ]. The methodology used in this review was adapted from the Cochrane Handbook for Systematic Reviews of Interventions and also followed established methodological considerations for analyzing existing systematic reviews [ 14 ].
Approval of a research ethics committee was not necessary as the study analyzed only publicly available articles.
Eligibility criteria
Systematic reviews were included if they analyzed primary data from patients infected with SARS-CoV-2 as confirmed by RT-PCR or another pre-specified diagnostic technique. Eligible reviews covered all topics related to COVID-19 including, but not limited to, those that reported clinical symptoms, diagnostic methods, therapeutic interventions, laboratory findings, or radiological results. Both full manuscripts and abbreviated versions, such as letters, were eligible.
No restrictions were imposed on the design of the primary studies included within the systematic reviews, the last search date, whether the review included meta-analyses or language. Reviews related to SARS-CoV-2 and other coronaviruses were eligible, but from those reviews, we analyzed only data related to SARS-CoV-2.
No consensus definition exists for a systematic review [ 20 ], and debates continue about the defining characteristics of a systematic review [ 21 ]. Cochrane’s guidance for overviews of reviews recommends setting pre-established criteria for making decisions around inclusion [ 14 ]. That is supported by a recent scoping review about guidance for overviews of systematic reviews [ 22 ].
Thus, for this study, we defined a systematic review as a research report which searched for primary research studies on a specific topic using an explicit search strategy, had a detailed description of the methods with explicit inclusion criteria provided, and provided a summary of the included studies either in narrative or quantitative format (such as a meta-analysis). Cochrane and non-Cochrane systematic reviews were considered eligible for inclusion, with or without meta-analysis, and regardless of the study design, language restriction and methodology of the included primary studies. To be eligible for inclusion, reviews had to be clearly analyzing data related to SARS-CoV-2 (associated or not with other viruses). We excluded narrative reviews without those characteristics as these are less likely to be replicable and are more prone to bias.
Scoping reviews and rapid reviews were eligible for inclusion in this overview if they met our pre-defined inclusion criteria noted above. We included reviews that addressed SARS-CoV-2 and other coronaviruses if they reported separate data regarding SARS-CoV-2.
Information sources
Nine databases were searched for eligible records published between December 1, 2019, and March 24, 2020: Cochrane Database of Systematic Reviews via Cochrane Library, PubMed, EMBASE, CINAHL (Cumulative Index to Nursing and Allied Health Literature), Web of Sciences, LILACS (Latin American and Caribbean Health Sciences Literature), PDQ-Evidence, WHO’s Global Research on Coronavirus Disease (COVID-19), and Epistemonikos.
The comprehensive search strategy for each database is provided in Additional file 1 and was designed and conducted in collaboration with an information specialist. All retrieved records were primarily processed in EndNote, where duplicates were removed, and records were then imported into the Covidence platform [ 23 ]. In addition to database searches, we screened reference lists of reviews included after screening records retrieved via databases.
Study selection
All searches, screening of titles and abstracts, and record selection, were performed independently by two investigators using the Covidence platform [ 23 ]. Articles deemed potentially eligible were retrieved for full-text screening carried out independently by two investigators. Discrepancies at all stages were resolved by consensus. During the screening, records published in languages other than English were translated by a native/fluent speaker.
Data collection process
We custom designed a data extraction table for this study, which was piloted by two authors independently. Data extraction was performed independently by two authors. Conflicts were resolved by consensus or by consulting a third researcher.
We extracted the following data: article identification data (authors’ name and journal of publication), search period, number of databases searched, population or settings considered, main results and outcomes observed, and number of participants. From Web of Science (Clarivate Analytics, Philadelphia, PA, USA), we extracted journal rank (quartile) and Journal Impact Factor (JIF).
We categorized the following as primary outcomes: all-cause mortality, need for and length of mechanical ventilation, length of hospitalization (in days), admission to intensive care unit (yes/no), and length of stay in the intensive care unit.
The following outcomes were categorized as exploratory: diagnostic methods used for detection of the virus, male to female ratio, clinical symptoms, pharmacological and non-pharmacological interventions, laboratory findings (full blood count, liver enzymes, C-reactive protein, d-dimer, albumin, lipid profile, serum electrolytes, blood vitamin levels, glucose levels, and any other important biomarkers), and radiological findings (using radiography, computed tomography, magnetic resonance imaging or ultrasound).
We also collected data on reporting guidelines and requirements for the publication of systematic reviews and meta-analyses from journal websites where included reviews were published.
Quality assessment in individual reviews
Two researchers independently assessed the reviews’ quality using the “A MeaSurement Tool to Assess Systematic Reviews 2 (AMSTAR 2)”. We acknowledge that the AMSTAR 2 was created as “a critical appraisal tool for systematic reviews that include randomized or non-randomized studies of healthcare interventions, or both” [ 24 ]. However, since AMSTAR 2 was designed for systematic reviews of intervention trials, and we included additional types of systematic reviews, we adjusted some AMSTAR 2 ratings and reported these in Additional file 2 .
Adherence to each item was rated as follows: yes, partial yes, no, or not applicable (such as when a meta-analysis was not conducted). The overall confidence in the results of the review is rated as “critically low”, “low”, “moderate” or “high”, according to the AMSTAR 2 guidance based on seven critical domains, which are items 2, 4, 7, 9, 11, 13, 15 as defined by AMSTAR 2 authors [ 24 ]. We reported our adherence ratings for transparency of our decision with accompanying explanations, for each item, in each included review.
One of the included systematic reviews was conducted by some members of this author team [ 25 ]. This review was initially assessed independently by two authors who were not co-authors of that review to prevent the risk of bias in assessing this study.
Synthesis of results
For data synthesis, we prepared a table summarizing each systematic review. Graphs illustrating the mortality rate and clinical symptoms were created. We then prepared a narrative summary of the methods, findings, study strengths, and limitations.
For analysis of the prevalence of clinical outcomes, we extracted data on the number of events and the total number of patients to perform proportional meta-analysis using RStudio© software, with the “meta” package (version 4.9–6), using the “metaprop” function for reviews that did not perform a meta-analysis, excluding case studies because of the absence of variance. For reviews that did not perform a meta-analysis, we presented pooled results of proportions with their respective confidence intervals (95%) by the inverse variance method with a random-effects model, using the DerSimonian-Laird estimator for τ 2 . We adjusted data using Freeman-Tukey double arcosen transformation. Confidence intervals were calculated using the Clopper-Pearson method for individual studies. We created forest plots using the RStudio© software, with the “metafor” package (version 2.1–0) and “forest” function.
Managing overlapping systematic reviews
Some of the included systematic reviews that address the same or similar research questions may include the same primary studies in overviews. Including such overlapping reviews may introduce bias when outcome data from the same primary study are included in the analyses of an overview multiple times. Thus, in summaries of evidence, multiple-counting of the same outcome data will give data from some primary studies too much influence [ 14 ]. In this overview, we did not exclude overlapping systematic reviews because, according to Cochrane’s guidance, it may be appropriate to include all relevant reviews’ results if the purpose of the overview is to present and describe the current body of evidence on a topic [ 14 ]. To avoid any bias in summary estimates associated with overlapping reviews, we generated forest plots showing data from individual systematic reviews, but the results were not pooled because some primary studies were included in multiple reviews.
Our search retrieved 1063 publications, of which 175 were duplicates. Most publications were excluded after the title and abstract analysis ( n = 860). Among the 28 studies selected for full-text screening, 10 were excluded for the reasons described in Additional file 3 , and 18 were included in the final analysis (Fig. 1 ) [ 25 , 26 , 27 , 28 , 29 , 30 , 31 , 32 , 33 , 34 , 35 , 36 , 37 , 38 , 39 , 40 , 41 , 42 ]. Reference list screening did not retrieve any additional systematic reviews.
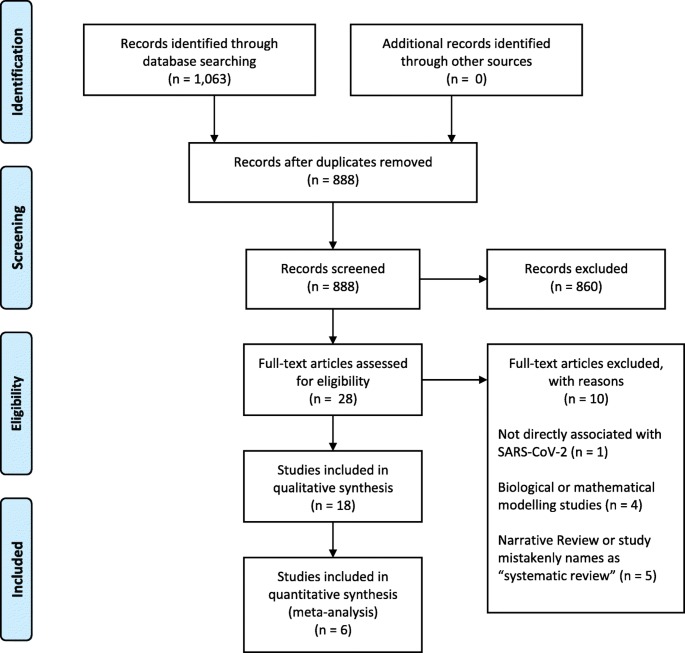
PRISMA flow diagram
Characteristics of included reviews
Summary features of 18 systematic reviews are presented in Table 1 . They were published in 14 different journals. Only four of these journals had specific requirements for systematic reviews (with or without meta-analysis): European Journal of Internal Medicine, Journal of Clinical Medicine, Ultrasound in Obstetrics and Gynecology, and Clinical Research in Cardiology . Two journals reported that they published only invited reviews ( Journal of Medical Virology and Clinica Chimica Acta ). Three systematic reviews in our study were published as letters; one was labeled as a scoping review and another as a rapid review (Table 2 ).
All reviews were published in English, in first quartile (Q1) journals, with JIF ranging from 1.692 to 6.062. One review was empty, meaning that its search did not identify any relevant studies; i.e., no primary studies were included [ 36 ]. The remaining 17 reviews included 269 unique studies; the majority ( N = 211; 78%) were included in only a single review included in our study (range: 1 to 12). Primary studies included in the reviews were published between December 2019 and March 18, 2020, and comprised case reports, case series, cohorts, and other observational studies. We found only one review that included randomized clinical trials [ 38 ]. In the included reviews, systematic literature searches were performed from 2019 (entire year) up to March 9, 2020. Ten systematic reviews included meta-analyses. The list of primary studies found in the included systematic reviews is shown in Additional file 4 , as well as the number of reviews in which each primary study was included.
Population and study designs
Most of the reviews analyzed data from patients with COVID-19 who developed pneumonia, acute respiratory distress syndrome (ARDS), or any other correlated complication. One review aimed to evaluate the effectiveness of using surgical masks on preventing transmission of the virus [ 36 ], one review was focused on pediatric patients [ 34 ], and one review investigated COVID-19 in pregnant women [ 37 ]. Most reviews assessed clinical symptoms, laboratory findings, or radiological results.
Systematic review findings
The summary of findings from individual reviews is shown in Table 2 . Overall, all-cause mortality ranged from 0.3 to 13.9% (Fig. 2 ).
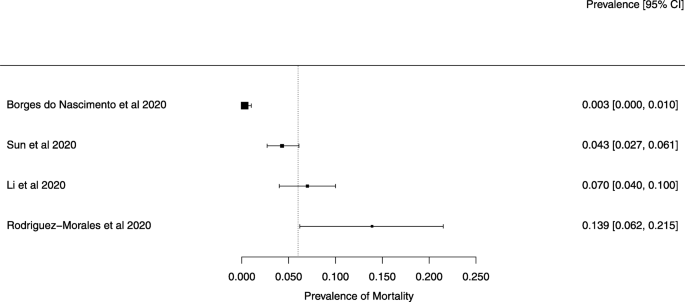
A meta-analysis of the prevalence of mortality
Clinical symptoms
Seven reviews described the main clinical manifestations of COVID-19 [ 26 , 28 , 29 , 34 , 35 , 39 , 41 ]. Three of them provided only a narrative discussion of symptoms [ 26 , 34 , 35 ]. In the reviews that performed a statistical analysis of the incidence of different clinical symptoms, symptoms in patients with COVID-19 were (range values of point estimates): fever (82–95%), cough with or without sputum (58–72%), dyspnea (26–59%), myalgia or muscle fatigue (29–51%), sore throat (10–13%), headache (8–12%), gastrointestinal disorders, such as diarrhea, nausea or vomiting (5.0–9.0%), and others (including, in one study only: dizziness 12.1%) (Figs. 3 , 4 , 5 , 6 , 7 , 8 and 9 ). Three reviews assessed cough with and without sputum together; only one review assessed sputum production itself (28.5%).
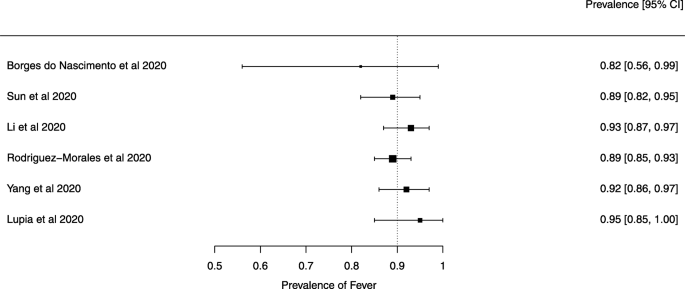
A meta-analysis of the prevalence of fever
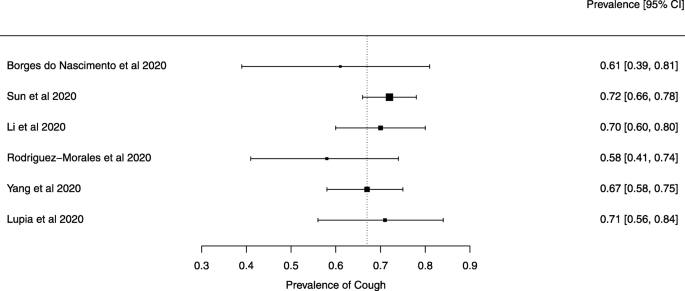
A meta-analysis of the prevalence of cough
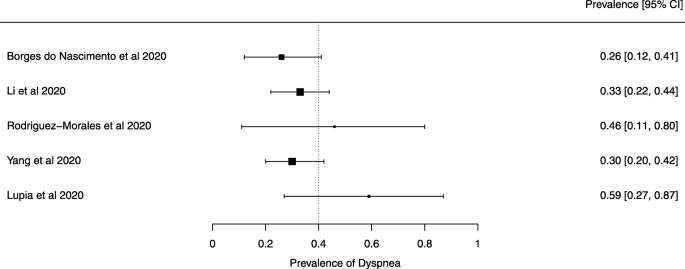
A meta-analysis of the prevalence of dyspnea
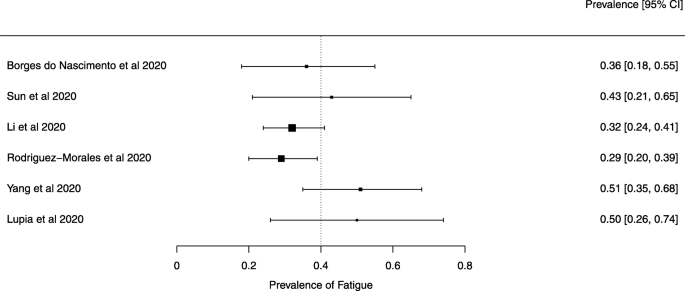
A meta-analysis of the prevalence of fatigue or myalgia
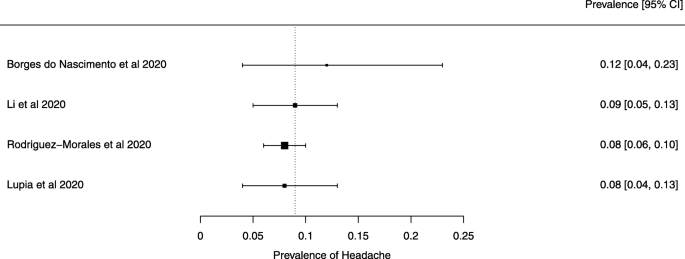
A meta-analysis of the prevalence of headache
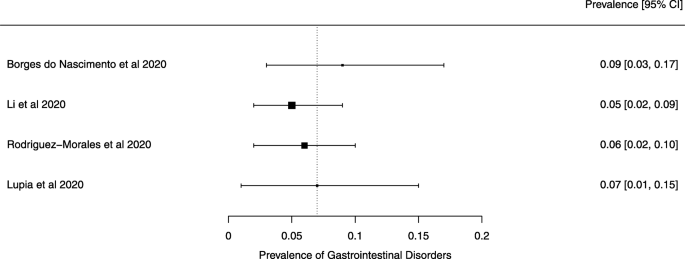
A meta-analysis of the prevalence of gastrointestinal disorders
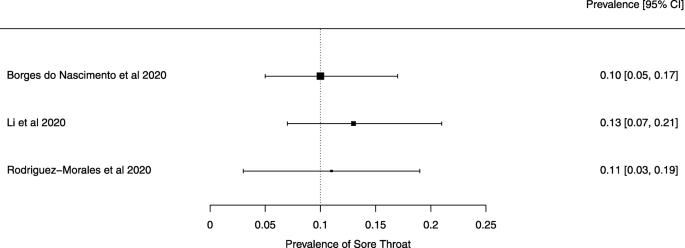
A meta-analysis of the prevalence of sore throat
Diagnostic aspects
Three reviews described methodologies, protocols, and tools used for establishing the diagnosis of COVID-19 [ 26 , 34 , 38 ]. The use of respiratory swabs (nasal or pharyngeal) or blood specimens to assess the presence of SARS-CoV-2 nucleic acid using RT-PCR assays was the most commonly used diagnostic method mentioned in the included studies. These diagnostic tests have been widely used, but their precise sensitivity and specificity remain unknown. One review included a Chinese study with clinical diagnosis with no confirmation of SARS-CoV-2 infection (patients were diagnosed with COVID-19 if they presented with at least two symptoms suggestive of COVID-19, together with laboratory and chest radiography abnormalities) [ 34 ].
Therapeutic possibilities
Pharmacological and non-pharmacological interventions (supportive therapies) used in treating patients with COVID-19 were reported in five reviews [ 25 , 27 , 34 , 35 , 38 ]. Antivirals used empirically for COVID-19 treatment were reported in seven reviews [ 25 , 27 , 34 , 35 , 37 , 38 , 41 ]; most commonly used were protease inhibitors (lopinavir, ritonavir, darunavir), nucleoside reverse transcriptase inhibitor (tenofovir), nucleotide analogs (remdesivir, galidesivir, ganciclovir), and neuraminidase inhibitors (oseltamivir). Umifenovir, a membrane fusion inhibitor, was investigated in two studies [ 25 , 35 ]. Possible supportive interventions analyzed were different types of oxygen supplementation and breathing support (invasive or non-invasive ventilation) [ 25 ]. The use of antibiotics, both empirically and to treat secondary pneumonia, was reported in six studies [ 25 , 26 , 27 , 34 , 35 , 38 ]. One review specifically assessed evidence on the efficacy and safety of the anti-malaria drug chloroquine [ 27 ]. It identified 23 ongoing trials investigating the potential of chloroquine as a therapeutic option for COVID-19, but no verifiable clinical outcomes data. The use of mesenchymal stem cells, antifungals, and glucocorticoids were described in four reviews [ 25 , 34 , 35 , 38 ].
Laboratory and radiological findings
Of the 18 reviews included in this overview, eight analyzed laboratory parameters in patients with COVID-19 [ 25 , 29 , 30 , 32 , 33 , 34 , 35 , 39 ]; elevated C-reactive protein levels, associated with lymphocytopenia, elevated lactate dehydrogenase, as well as slightly elevated aspartate and alanine aminotransferase (AST, ALT) were commonly described in those eight reviews. Lippi et al. assessed cardiac troponin I (cTnI) [ 25 ], procalcitonin [ 32 ], and platelet count [ 33 ] in COVID-19 patients. Elevated levels of procalcitonin [ 32 ] and cTnI [ 30 ] were more likely to be associated with a severe disease course (requiring intensive care unit admission and intubation). Furthermore, thrombocytopenia was frequently observed in patients with complicated COVID-19 infections [ 33 ].
Chest imaging (chest radiography and/or computed tomography) features were assessed in six reviews, all of which described a frequent pattern of local or bilateral multilobar ground-glass opacity [ 25 , 34 , 35 , 39 , 40 , 41 ]. Those six reviews showed that septal thickening, bronchiectasis, pleural and cardiac effusions, halo signs, and pneumothorax were observed in patients suffering from COVID-19.
Quality of evidence in individual systematic reviews
Table 3 shows the detailed results of the quality assessment of 18 systematic reviews, including the assessment of individual items and summary assessment. A detailed explanation for each decision in each review is available in Additional file 5 .
Using AMSTAR 2 criteria, confidence in the results of all 18 reviews was rated as “critically low” (Table 3 ). Common methodological drawbacks were: omission of prospective protocol submission or publication; use of inappropriate search strategy: lack of independent and dual literature screening and data-extraction (or methodology unclear); absence of an explanation for heterogeneity among the studies included; lack of reasons for study exclusion (or rationale unclear).
Risk of bias assessment, based on a reported methodological tool, and quality of evidence appraisal, in line with the Grading of Recommendations Assessment, Development, and Evaluation (GRADE) method, were reported only in one review [ 25 ]. Five reviews presented a table summarizing bias, using various risk of bias tools [ 25 , 29 , 39 , 40 , 41 ]. One review analyzed “study quality” [ 37 ]. One review mentioned the risk of bias assessment in the methodology but did not provide any related analysis [ 28 ].
This overview of systematic reviews analyzed the first 18 systematic reviews published after the onset of the COVID-19 pandemic, up to March 24, 2020, with primary studies involving more than 60,000 patients. Using AMSTAR-2, we judged that our confidence in all those reviews was “critically low”. Ten reviews included meta-analyses. The reviews presented data on clinical manifestations, laboratory and radiological findings, and interventions. We found no systematic reviews on the utility of diagnostic tests.
Symptoms were reported in seven reviews; most of the patients had a fever, cough, dyspnea, myalgia or muscle fatigue, and gastrointestinal disorders such as diarrhea, nausea, or vomiting. Olfactory dysfunction (anosmia or dysosmia) has been described in patients infected with COVID-19 [ 43 ]; however, this was not reported in any of the reviews included in this overview. During the SARS outbreak in 2002, there were reports of impairment of the sense of smell associated with the disease [ 44 , 45 ].
The reported mortality rates ranged from 0.3 to 14% in the included reviews. Mortality estimates are influenced by the transmissibility rate (basic reproduction number), availability of diagnostic tools, notification policies, asymptomatic presentations of the disease, resources for disease prevention and control, and treatment facilities; variability in the mortality rate fits the pattern of emerging infectious diseases [ 46 ]. Furthermore, the reported cases did not consider asymptomatic cases, mild cases where individuals have not sought medical treatment, and the fact that many countries had limited access to diagnostic tests or have implemented testing policies later than the others. Considering the lack of reviews assessing diagnostic testing (sensitivity, specificity, and predictive values of RT-PCT or immunoglobulin tests), and the preponderance of studies that assessed only symptomatic individuals, considerable imprecision around the calculated mortality rates existed in the early stage of the COVID-19 pandemic.
Few reviews included treatment data. Those reviews described studies considered to be at a very low level of evidence: usually small, retrospective studies with very heterogeneous populations. Seven reviews analyzed laboratory parameters; those reviews could have been useful for clinicians who attend patients suspected of COVID-19 in emergency services worldwide, such as assessing which patients need to be reassessed more frequently.
All systematic reviews scored poorly on the AMSTAR 2 critical appraisal tool for systematic reviews. Most of the original studies included in the reviews were case series and case reports, impacting the quality of evidence. Such evidence has major implications for clinical practice and the use of these reviews in evidence-based practice and policy. Clinicians, patients, and policymakers can only have the highest confidence in systematic review findings if high-quality systematic review methodologies are employed. The urgent need for information during a pandemic does not justify poor quality reporting.
We acknowledge that there are numerous challenges associated with analyzing COVID-19 data during a pandemic [ 47 ]. High-quality evidence syntheses are needed for decision-making, but each type of evidence syntheses is associated with its inherent challenges.
The creation of classic systematic reviews requires considerable time and effort; with massive research output, they quickly become outdated, and preparing updated versions also requires considerable time. A recent study showed that updates of non-Cochrane systematic reviews are published a median of 5 years after the publication of the previous version [ 48 ].
Authors may register a review and then abandon it [ 49 ], but the existence of a public record that is not updated may lead other authors to believe that the review is still ongoing. A quarter of Cochrane review protocols remains unpublished as completed systematic reviews 8 years after protocol publication [ 50 ].
Rapid reviews can be used to summarize the evidence, but they involve methodological sacrifices and simplifications to produce information promptly, with inconsistent methodological approaches [ 51 ]. However, rapid reviews are justified in times of public health emergencies, and even Cochrane has resorted to publishing rapid reviews in response to the COVID-19 crisis [ 52 ]. Rapid reviews were eligible for inclusion in this overview, but only one of the 18 reviews included in this study was labeled as a rapid review.
Ideally, COVID-19 evidence would be continually summarized in a series of high-quality living systematic reviews, types of evidence synthesis defined as “ a systematic review which is continually updated, incorporating relevant new evidence as it becomes available ” [ 53 ]. However, conducting living systematic reviews requires considerable resources, calling into question the sustainability of such evidence synthesis over long periods [ 54 ].
Research reports about COVID-19 will contribute to research waste if they are poorly designed, poorly reported, or simply not necessary. In principle, systematic reviews should help reduce research waste as they usually provide recommendations for further research that is needed or may advise that sufficient evidence exists on a particular topic [ 55 ]. However, systematic reviews can also contribute to growing research waste when they are not needed, or poorly conducted and reported. Our present study clearly shows that most of the systematic reviews that were published early on in the COVID-19 pandemic could be categorized as research waste, as our confidence in their results is critically low.
Our study has some limitations. One is that for AMSTAR 2 assessment we relied on information available in publications; we did not attempt to contact study authors for clarifications or additional data. In three reviews, the methodological quality appraisal was challenging because they were published as letters, or labeled as rapid communications. As a result, various details about their review process were not included, leading to AMSTAR 2 questions being answered as “not reported”, resulting in low confidence scores. Full manuscripts might have provided additional information that could have led to higher confidence in the results. In other words, low scores could reflect incomplete reporting, not necessarily low-quality review methods. To make their review available more rapidly and more concisely, the authors may have omitted methodological details. A general issue during a crisis is that speed and completeness must be balanced. However, maintaining high standards requires proper resourcing and commitment to ensure that the users of systematic reviews can have high confidence in the results.
Furthermore, we used adjusted AMSTAR 2 scoring, as the tool was designed for critical appraisal of reviews of interventions. Some reviews may have received lower scores than actually warranted in spite of these adjustments.
Another limitation of our study may be the inclusion of multiple overlapping reviews, as some included reviews included the same primary studies. According to the Cochrane Handbook, including overlapping reviews may be appropriate when the review’s aim is “ to present and describe the current body of systematic review evidence on a topic ” [ 12 ], which was our aim. To avoid bias with summarizing evidence from overlapping reviews, we presented the forest plots without summary estimates. The forest plots serve to inform readers about the effect sizes for outcomes that were reported in each review.
Several authors from this study have contributed to one of the reviews identified [ 25 ]. To reduce the risk of any bias, two authors who did not co-author the review in question initially assessed its quality and limitations.
Finally, we note that the systematic reviews included in our overview may have had issues that our analysis did not identify because we did not analyze their primary studies to verify the accuracy of the data and information they presented. We give two examples to substantiate this possibility. Lovato et al. wrote a commentary on the review of Sun et al. [ 41 ], in which they criticized the authors’ conclusion that sore throat is rare in COVID-19 patients [ 56 ]. Lovato et al. highlighted that multiple studies included in Sun et al. did not accurately describe participants’ clinical presentations, warning that only three studies clearly reported data on sore throat [ 56 ].
In another example, Leung [ 57 ] warned about the review of Li, L.Q. et al. [ 29 ]: “ it is possible that this statistic was computed using overlapped samples, therefore some patients were double counted ”. Li et al. responded to Leung that it is uncertain whether the data overlapped, as they used data from published articles and did not have access to the original data; they also reported that they requested original data and that they plan to re-do their analyses once they receive them; they also urged readers to treat the data with caution [ 58 ]. This points to the evolving nature of evidence during a crisis.
Our study’s strength is that this overview adds to the current knowledge by providing a comprehensive summary of all the evidence synthesis about COVID-19 available early after the onset of the pandemic. This overview followed strict methodological criteria, including a comprehensive and sensitive search strategy and a standard tool for methodological appraisal of systematic reviews.
In conclusion, in this overview of systematic reviews, we analyzed evidence from the first 18 systematic reviews that were published after the emergence of COVID-19. However, confidence in the results of all the reviews was “critically low”. Thus, systematic reviews that were published early on in the pandemic could be categorized as research waste. Even during public health emergencies, studies and systematic reviews should adhere to established methodological standards to provide patients, clinicians, and decision-makers trustworthy evidence.
Availability of data and materials
All data collected and analyzed within this study are available from the corresponding author on reasonable request.
World Health Organization. Timeline - COVID-19: Available at: https://www.who.int/news/item/29-06-2020-covidtimeline . Accessed 1 June 2021.
COVID-19 Dashboard by the Center for Systems Science and Engineering (CSSE) at Johns Hopkins University (JHU). Available at: https://coronavirus.jhu.edu/map.html . Accessed 1 June 2021.
Anzai A, Kobayashi T, Linton NM, Kinoshita R, Hayashi K, Suzuki A, et al. Assessing the Impact of Reduced Travel on Exportation Dynamics of Novel Coronavirus Infection (COVID-19). J Clin Med. 2020;9(2):601.
Chinazzi M, Davis JT, Ajelli M, Gioannini C, Litvinova M, Merler S, et al. The effect of travel restrictions on the spread of the 2019 novel coronavirus (COVID-19) outbreak. Science. 2020;368(6489):395–400. https://doi.org/10.1126/science.aba9757 .
Article CAS PubMed PubMed Central Google Scholar
Fidahic M, Nujic D, Runjic R, Civljak M, Markotic F, Lovric Makaric Z, et al. Research methodology and characteristics of journal articles with original data, preprint articles and registered clinical trial protocols about COVID-19. BMC Med Res Methodol. 2020;20(1):161. https://doi.org/10.1186/s12874-020-01047-2 .
EPPI Centre . COVID-19: a living systematic map of the evidence. Available at: http://eppi.ioe.ac.uk/cms/Projects/DepartmentofHealthandSocialCare/Publishedreviews/COVID-19Livingsystematicmapoftheevidence/tabid/3765/Default.aspx . Accessed 1 June 2021.
NCBI SARS-CoV-2 Resources. Available at: https://www.ncbi.nlm.nih.gov/sars-cov-2/ . Accessed 1 June 2021.
Gustot T. Quality and reproducibility during the COVID-19 pandemic. JHEP Rep. 2020;2(4):100141. https://doi.org/10.1016/j.jhepr.2020.100141 .
Article PubMed PubMed Central Google Scholar
Kodvanj, I., et al., Publishing of COVID-19 Preprints in Peer-reviewed Journals, Preprinting Trends, Public Discussion and Quality Issues. Preprint article. bioRxiv 2020.11.23.394577; doi: https://doi.org/10.1101/2020.11.23.394577 .
Dobler CC. Poor quality research and clinical practice during COVID-19. Breathe (Sheff). 2020;16(2):200112. https://doi.org/10.1183/20734735.0112-2020 .
Article Google Scholar
Bastian H, Glasziou P, Chalmers I. Seventy-five trials and eleven systematic reviews a day: how will we ever keep up? PLoS Med. 2010;7(9):e1000326. https://doi.org/10.1371/journal.pmed.1000326 .
Lunny C, Brennan SE, McDonald S, McKenzie JE. Toward a comprehensive evidence map of overview of systematic review methods: paper 1-purpose, eligibility, search and data extraction. Syst Rev. 2017;6(1):231. https://doi.org/10.1186/s13643-017-0617-1 .
Pollock M, Fernandes RM, Becker LA, Pieper D, Hartling L. Chapter V: Overviews of Reviews. In: Higgins JPT, Thomas J, Chandler J, Cumpston M, Li T, Page MJ, Welch VA (editors). Cochrane Handbook for Systematic Reviews of Interventions version 6.1 (updated September 2020). Cochrane. 2020. Available from www.training.cochrane.org/handbook .
Higgins JPT, Thomas J, Chandler J, Cumpston M, Li T, Page MJ, et al. Cochrane handbook for systematic reviews of interventions version 6.1 (updated September 2020). Cochrane. 2020; Available from www.training.cochrane.org/handbook .
Pollock M, Fernandes RM, Newton AS, Scott SD, Hartling L. The impact of different inclusion decisions on the comprehensiveness and complexity of overviews of reviews of healthcare interventions. Syst Rev. 2019;8(1):18. https://doi.org/10.1186/s13643-018-0914-3 .
Pollock M, Fernandes RM, Newton AS, Scott SD, Hartling L. A decision tool to help researchers make decisions about including systematic reviews in overviews of reviews of healthcare interventions. Syst Rev. 2019;8(1):29. https://doi.org/10.1186/s13643-018-0768-8 .
Hunt H, Pollock A, Campbell P, Estcourt L, Brunton G. An introduction to overviews of reviews: planning a relevant research question and objective for an overview. Syst Rev. 2018;7(1):39. https://doi.org/10.1186/s13643-018-0695-8 .
Pollock M, Fernandes RM, Pieper D, Tricco AC, Gates M, Gates A, et al. Preferred reporting items for overviews of reviews (PRIOR): a protocol for development of a reporting guideline for overviews of reviews of healthcare interventions. Syst Rev. 2019;8(1):335. https://doi.org/10.1186/s13643-019-1252-9 .
Moher D, Liberati A, Tetzlaff J, Altman DG, PRISMA Group. Preferred reporting items for systematic reviews and meta-analyses: the PRISMA statement. Open Med. 2009;3(3):e123–30.
Krnic Martinic M, Pieper D, Glatt A, Puljak L. Definition of a systematic review used in overviews of systematic reviews, meta-epidemiological studies and textbooks. BMC Med Res Methodol. 2019;19(1):203. https://doi.org/10.1186/s12874-019-0855-0 .
Puljak L. If there is only one author or only one database was searched, a study should not be called a systematic review. J Clin Epidemiol. 2017;91:4–5. https://doi.org/10.1016/j.jclinepi.2017.08.002 .
Article PubMed Google Scholar
Gates M, Gates A, Guitard S, Pollock M, Hartling L. Guidance for overviews of reviews continues to accumulate, but important challenges remain: a scoping review. Syst Rev. 2020;9(1):254. https://doi.org/10.1186/s13643-020-01509-0 .
Covidence - systematic review software. Available at: https://www.covidence.org/ . Accessed 1 June 2021.
Shea BJ, Reeves BC, Wells G, Thuku M, Hamel C, Moran J, et al. AMSTAR 2: a critical appraisal tool for systematic reviews that include randomised or non-randomised studies of healthcare interventions, or both. BMJ. 2017;358:j4008.
Borges do Nascimento IJ, et al. Novel Coronavirus Infection (COVID-19) in Humans: A Scoping Review and Meta-Analysis. J Clin Med. 2020;9(4):941.
Article PubMed Central Google Scholar
Adhikari SP, Meng S, Wu YJ, Mao YP, Ye RX, Wang QZ, et al. Epidemiology, causes, clinical manifestation and diagnosis, prevention and control of coronavirus disease (COVID-19) during the early outbreak period: a scoping review. Infect Dis Poverty. 2020;9(1):29. https://doi.org/10.1186/s40249-020-00646-x .
Cortegiani A, Ingoglia G, Ippolito M, Giarratano A, Einav S. A systematic review on the efficacy and safety of chloroquine for the treatment of COVID-19. J Crit Care. 2020;57:279–83. https://doi.org/10.1016/j.jcrc.2020.03.005 .
Li B, Yang J, Zhao F, Zhi L, Wang X, Liu L, et al. Prevalence and impact of cardiovascular metabolic diseases on COVID-19 in China. Clin Res Cardiol. 2020;109(5):531–8. https://doi.org/10.1007/s00392-020-01626-9 .
Article CAS PubMed Google Scholar
Li LQ, Huang T, Wang YQ, Wang ZP, Liang Y, Huang TB, et al. COVID-19 patients’ clinical characteristics, discharge rate, and fatality rate of meta-analysis. J Med Virol. 2020;92(6):577–83. https://doi.org/10.1002/jmv.25757 .
Lippi G, Lavie CJ, Sanchis-Gomar F. Cardiac troponin I in patients with coronavirus disease 2019 (COVID-19): evidence from a meta-analysis. Prog Cardiovasc Dis. 2020;63(3):390–1. https://doi.org/10.1016/j.pcad.2020.03.001 .
Lippi G, Henry BM. Active smoking is not associated with severity of coronavirus disease 2019 (COVID-19). Eur J Intern Med. 2020;75:107–8. https://doi.org/10.1016/j.ejim.2020.03.014 .
Lippi G, Plebani M. Procalcitonin in patients with severe coronavirus disease 2019 (COVID-19): a meta-analysis. Clin Chim Acta. 2020;505:190–1. https://doi.org/10.1016/j.cca.2020.03.004 .
Lippi G, Plebani M, Henry BM. Thrombocytopenia is associated with severe coronavirus disease 2019 (COVID-19) infections: a meta-analysis. Clin Chim Acta. 2020;506:145–8. https://doi.org/10.1016/j.cca.2020.03.022 .
Ludvigsson JF. Systematic review of COVID-19 in children shows milder cases and a better prognosis than adults. Acta Paediatr. 2020;109(6):1088–95. https://doi.org/10.1111/apa.15270 .
Lupia T, Scabini S, Mornese Pinna S, di Perri G, de Rosa FG, Corcione S. 2019 novel coronavirus (2019-nCoV) outbreak: a new challenge. J Glob Antimicrob Resist. 2020;21:22–7. https://doi.org/10.1016/j.jgar.2020.02.021 .
Marasinghe, K.M., A systematic review investigating the effectiveness of face mask use in limiting the spread of COVID-19 among medically not diagnosed individuals: shedding light on current recommendations provided to individuals not medically diagnosed with COVID-19. Research Square. Preprint article. doi : https://doi.org/10.21203/rs.3.rs-16701/v1 . 2020 .
Mullins E, Evans D, Viner RM, O’Brien P, Morris E. Coronavirus in pregnancy and delivery: rapid review. Ultrasound Obstet Gynecol. 2020;55(5):586–92. https://doi.org/10.1002/uog.22014 .
Pang J, Wang MX, Ang IYH, Tan SHX, Lewis RF, Chen JIP, et al. Potential Rapid Diagnostics, Vaccine and Therapeutics for 2019 Novel coronavirus (2019-nCoV): a systematic review. J Clin Med. 2020;9(3):623.
Rodriguez-Morales AJ, Cardona-Ospina JA, Gutiérrez-Ocampo E, Villamizar-Peña R, Holguin-Rivera Y, Escalera-Antezana JP, et al. Clinical, laboratory and imaging features of COVID-19: a systematic review and meta-analysis. Travel Med Infect Dis. 2020;34:101623. https://doi.org/10.1016/j.tmaid.2020.101623 .
Salehi S, Abedi A, Balakrishnan S, Gholamrezanezhad A. Coronavirus disease 2019 (COVID-19): a systematic review of imaging findings in 919 patients. AJR Am J Roentgenol. 2020;215(1):87–93. https://doi.org/10.2214/AJR.20.23034 .
Sun P, Qie S, Liu Z, Ren J, Li K, Xi J. Clinical characteristics of hospitalized patients with SARS-CoV-2 infection: a single arm meta-analysis. J Med Virol. 2020;92(6):612–7. https://doi.org/10.1002/jmv.25735 .
Yang J, Zheng Y, Gou X, Pu K, Chen Z, Guo Q, et al. Prevalence of comorbidities and its effects in patients infected with SARS-CoV-2: a systematic review and meta-analysis. Int J Infect Dis. 2020;94:91–5. https://doi.org/10.1016/j.ijid.2020.03.017 .
Bassetti M, Vena A, Giacobbe DR. The novel Chinese coronavirus (2019-nCoV) infections: challenges for fighting the storm. Eur J Clin Investig. 2020;50(3):e13209. https://doi.org/10.1111/eci.13209 .
Article CAS Google Scholar
Hwang CS. Olfactory neuropathy in severe acute respiratory syndrome: report of a case. Acta Neurol Taiwanica. 2006;15(1):26–8.
Google Scholar
Suzuki M, Saito K, Min WP, Vladau C, Toida K, Itoh H, et al. Identification of viruses in patients with postviral olfactory dysfunction. Laryngoscope. 2007;117(2):272–7. https://doi.org/10.1097/01.mlg.0000249922.37381.1e .
Rajgor DD, Lee MH, Archuleta S, Bagdasarian N, Quek SC. The many estimates of the COVID-19 case fatality rate. Lancet Infect Dis. 2020;20(7):776–7. https://doi.org/10.1016/S1473-3099(20)30244-9 .
Wolkewitz M, Puljak L. Methodological challenges of analysing COVID-19 data during the pandemic. BMC Med Res Methodol. 2020;20(1):81. https://doi.org/10.1186/s12874-020-00972-6 .
Rombey T, Lochner V, Puljak L, Könsgen N, Mathes T, Pieper D. Epidemiology and reporting characteristics of non-Cochrane updates of systematic reviews: a cross-sectional study. Res Synth Methods. 2020;11(3):471–83. https://doi.org/10.1002/jrsm.1409 .
Runjic E, Rombey T, Pieper D, Puljak L. Half of systematic reviews about pain registered in PROSPERO were not published and the majority had inaccurate status. J Clin Epidemiol. 2019;116:114–21. https://doi.org/10.1016/j.jclinepi.2019.08.010 .
Runjic E, Behmen D, Pieper D, Mathes T, Tricco AC, Moher D, et al. Following Cochrane review protocols to completion 10 years later: a retrospective cohort study and author survey. J Clin Epidemiol. 2019;111:41–8. https://doi.org/10.1016/j.jclinepi.2019.03.006 .
Tricco AC, Antony J, Zarin W, Strifler L, Ghassemi M, Ivory J, et al. A scoping review of rapid review methods. BMC Med. 2015;13(1):224. https://doi.org/10.1186/s12916-015-0465-6 .
COVID-19 Rapid Reviews: Cochrane’s response so far. Available at: https://training.cochrane.org/resource/covid-19-rapid-reviews-cochrane-response-so-far . Accessed 1 June 2021.
Cochrane. Living systematic reviews. Available at: https://community.cochrane.org/review-production/production-resources/living-systematic-reviews . Accessed 1 June 2021.
Millard T, Synnot A, Elliott J, Green S, McDonald S, Turner T. Feasibility and acceptability of living systematic reviews: results from a mixed-methods evaluation. Syst Rev. 2019;8(1):325. https://doi.org/10.1186/s13643-019-1248-5 .
Babic A, Poklepovic Pericic T, Pieper D, Puljak L. How to decide whether a systematic review is stable and not in need of updating: analysis of Cochrane reviews. Res Synth Methods. 2020;11(6):884–90. https://doi.org/10.1002/jrsm.1451 .
Lovato A, Rossettini G, de Filippis C. Sore throat in COVID-19: comment on “clinical characteristics of hospitalized patients with SARS-CoV-2 infection: a single arm meta-analysis”. J Med Virol. 2020;92(7):714–5. https://doi.org/10.1002/jmv.25815 .
Leung C. Comment on Li et al: COVID-19 patients’ clinical characteristics, discharge rate, and fatality rate of meta-analysis. J Med Virol. 2020;92(9):1431–2. https://doi.org/10.1002/jmv.25912 .
Li LQ, Huang T, Wang YQ, Wang ZP, Liang Y, Huang TB, et al. Response to Char’s comment: comment on Li et al: COVID-19 patients’ clinical characteristics, discharge rate, and fatality rate of meta-analysis. J Med Virol. 2020;92(9):1433. https://doi.org/10.1002/jmv.25924 .
Download references
Acknowledgments
We thank Catherine Henderson DPhil from Swanscoe Communications for pro bono medical writing and editing support. We acknowledge support from the Covidence Team, specifically Anneliese Arno. We thank the whole International Network of Coronavirus Disease 2019 (InterNetCOVID-19) for their commitment and involvement. Members of the InterNetCOVID-19 are listed in Additional file 6 . We thank Pavel Cerny and Roger Crosthwaite for guiding the team supervisor (IJBN) on human resources management.
This research received no external funding.
Author information
Authors and affiliations.
University Hospital and School of Medicine, Universidade Federal de Minas Gerais, Belo Horizonte, Minas Gerais, Brazil
Israel Júnior Borges do Nascimento & Milena Soriano Marcolino
Medical College of Wisconsin, Milwaukee, WI, USA
Israel Júnior Borges do Nascimento
Helene Fuld Health Trust National Institute for Evidence-based Practice in Nursing and Healthcare, College of Nursing, The Ohio State University, Columbus, OH, USA
Dónal P. O’Mathúna
School of Nursing, Psychotherapy and Community Health, Dublin City University, Dublin, Ireland
Department of Anesthesiology, Intensive Care and Pain Medicine, University of Münster, Münster, Germany
Thilo Caspar von Groote
Department of Sport and Health Science, Technische Universität München, Munich, Germany
Hebatullah Mohamed Abdulazeem
School of Health Sciences, Faculty of Health and Medicine, The University of Newcastle, Callaghan, Australia
Ishanka Weerasekara
Department of Physiotherapy, Faculty of Allied Health Sciences, University of Peradeniya, Peradeniya, Sri Lanka
Cochrane Croatia, University of Split, School of Medicine, Split, Croatia
Ana Marusic, Irena Zakarija-Grkovic & Tina Poklepovic Pericic
Center for Evidence-Based Medicine and Health Care, Catholic University of Croatia, Ilica 242, 10000, Zagreb, Croatia
Livia Puljak
Cochrane Brazil, Evidence-Based Health Program, Universidade Federal de São Paulo, São Paulo, Brazil
Vinicius Tassoni Civile & Alvaro Nagib Atallah
Yorkville University, Fredericton, New Brunswick, Canada
Santino Filoso
Laboratory for Industrial and Applied Mathematics (LIAM), Department of Mathematics and Statistics, York University, Toronto, Ontario, Canada
Nicola Luigi Bragazzi
You can also search for this author in PubMed Google Scholar
Contributions
IJBN conceived the research idea and worked as a project coordinator. DPOM, TCVG, HMA, IW, AM, LP, VTC, IZG, TPP, ANA, SF, NLB and MSM were involved in data curation, formal analysis, investigation, methodology, and initial draft writing. All authors revised the manuscript critically for the content. The author(s) read and approved the final manuscript.
Corresponding author
Correspondence to Livia Puljak .
Ethics declarations
Ethics approval and consent to participate.
Not required as data was based on published studies.
Consent for publication
Not applicable.
Competing interests
The authors declare no conflict of interest.
Additional information
Publisher’s note.
Springer Nature remains neutral with regard to jurisdictional claims in published maps and institutional affiliations.
Supplementary Information
Additional file 1: appendix 1..
Search strategies used in the study.
Additional file 2: Appendix 2.
Adjusted scoring of AMSTAR 2 used in this study for systematic reviews of studies that did not analyze interventions.
Additional file 3: Appendix 3.
List of excluded studies, with reasons.
Additional file 4: Appendix 4.
Table of overlapping studies, containing the list of primary studies included, their visual overlap in individual systematic reviews, and the number in how many reviews each primary study was included.
Additional file 5: Appendix 5.
A detailed explanation of AMSTAR scoring for each item in each review.
Additional file 6: Appendix 6.
List of members and affiliates of International Network of Coronavirus Disease 2019 (InterNetCOVID-19).
Rights and permissions
Open Access This article is licensed under a Creative Commons Attribution 4.0 International License, which permits use, sharing, adaptation, distribution and reproduction in any medium or format, as long as you give appropriate credit to the original author(s) and the source, provide a link to the Creative Commons licence, and indicate if changes were made. The images or other third party material in this article are included in the article's Creative Commons licence, unless indicated otherwise in a credit line to the material. If material is not included in the article's Creative Commons licence and your intended use is not permitted by statutory regulation or exceeds the permitted use, you will need to obtain permission directly from the copyright holder. To view a copy of this licence, visit http://creativecommons.org/licenses/by/4.0/ . The Creative Commons Public Domain Dedication waiver ( http://creativecommons.org/publicdomain/zero/1.0/ ) applies to the data made available in this article, unless otherwise stated in a credit line to the data.
Reprints and permissions
About this article
Cite this article.
Borges do Nascimento, I.J., O’Mathúna, D.P., von Groote, T.C. et al. Coronavirus disease (COVID-19) pandemic: an overview of systematic reviews. BMC Infect Dis 21 , 525 (2021). https://doi.org/10.1186/s12879-021-06214-4
Download citation
Received : 12 April 2020
Accepted : 19 May 2021
Published : 04 June 2021
DOI : https://doi.org/10.1186/s12879-021-06214-4
Share this article
Anyone you share the following link with will be able to read this content:
Sorry, a shareable link is not currently available for this article.
Provided by the Springer Nature SharedIt content-sharing initiative
- Coronavirus
- Evidence-based medicine
- Infectious diseases
BMC Infectious Diseases
ISSN: 1471-2334
- General enquiries: [email protected]
- Fact sheets
- Facts in pictures
- Publications
- Questions and answers
- Tools and toolkits
- Endometriosis
- Excessive heat
- Mental disorders
- Polycystic ovary syndrome
- All countries
- Eastern Mediterranean
- South-East Asia
- Western Pacific
- Data by country
- Country presence
- Country strengthening
- Country cooperation strategies
- News releases
- Feature stories
- Press conferences
- Commentaries
- Photo library
- Afghanistan
- Cholera
- Coronavirus disease (COVID-19)
- Greater Horn of Africa
- Israel and occupied Palestinian territory
- Disease Outbreak News
- Situation reports
- Weekly Epidemiological Record
- Surveillance
- Health emergency appeal
- International Health Regulations
- Independent Oversight and Advisory Committee
- Classifications
- Data collections
- Global Health Observatory
- Global Health Estimates
- Mortality Database
- Sustainable Development Goals
- Health Inequality Monitor
- Global Progress
- World Health Statistics
- Partnerships
- Committees and advisory groups
- Collaborating centres
- Technical teams
- Organizational structure
- Initiatives
- General Programme of Work
- WHO Academy
- Investment in WHO
- WHO Foundation
- External audit
- Financial statements
- Internal audit and investigations
- Programme Budget
- Results reports
- Governing bodies
- World Health Assembly
- Executive Board
- Member States Portal
- Coronavirus disease (COVID-19) /
Global research on coronavirus disease (COVID-19)
Section navigation.
- WHO COVID-19 Solidarity Therapeutics Trial
- "Solidarity II" global serologic study for COVID-19
- Accelerating a safe and effective COVID-19 vaccine
- Solidarity Trial Vaccines
WHO is bringing the world’s scientists and global health professionals together to accelerate the research and development process, and develop new norms and standards to contain the spread of the coronavirus pandemic and help care for those affected.
The R&D Blueprint has been activated to accelerate diagnostics, vaccines and therapeutics for this novel coronavirus.
The solidarity of all countries will be essential to ensure equitable access to COVID-19 health products.
WHO COVID-19 Research Database
The WHO COVID-19 Research Database was a resource created in response to the Public Health Emergency of International Concern (PHEIC). It contained citations with abstracts to scientific articles, reports, books, preprints, and clinical trials on COVID-19 and related literature. The WHO Covid-19 Research Database was maintained by the WHO Library & Digital Information Networks and was funded by COVID-19 emergency funds. The database was built by BIREME, the Specialized Center of PAHO/AMRO. Its content spanned the time period March 2020 to June 2023. It has now been archived, and no longer searchable since January 2024.
Click through the PLOS taxonomy to find articles in your field.
For more information about PLOS Subject Areas, click here .
Loading metrics
Open Access
Peer-reviewed
Research Article
The impact of the COVID-19 pandemic on scientific research in the life sciences
Roles Conceptualization, Formal analysis, Methodology, Writing – original draft, Writing – review & editing
Affiliation AXES, IMT School for Advanced Studies Lucca, Lucca, Italy
Roles Conceptualization, Data curation, Formal analysis, Methodology, Software, Visualization, Writing – original draft, Writing – review & editing
* E-mail: [email protected]
Affiliation Chair of Systems Design D-MTEC, ETH Zürich, Zurich, Switzerland

- Massimo Riccaboni,
- Luca Verginer
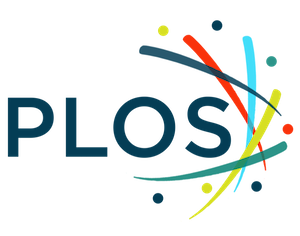
- Published: February 9, 2022
- https://doi.org/10.1371/journal.pone.0263001
- Reader Comments
The COVID-19 outbreak has posed an unprecedented challenge to humanity and science. On the one side, public and private incentives have been put in place to promptly allocate resources toward research areas strictly related to the COVID-19 emergency. However, research in many fields not directly related to the pandemic has been displaced. In this paper, we assess the impact of COVID-19 on world scientific production in the life sciences and find indications that the usage of medical subject headings (MeSH) has changed following the outbreak. We estimate through a difference-in-differences approach the impact of the start of the COVID-19 pandemic on scientific production using the PubMed database (3.6 Million research papers). We find that COVID-19-related MeSH terms have experienced a 6.5 fold increase in output on average, while publications on unrelated MeSH terms dropped by 10 to 12%. The publication weighted impact has an even more pronounced negative effect (-16% to -19%). Moreover, COVID-19 has displaced clinical trial publications (-24%) and diverted grants from research areas not closely related to COVID-19. Note that since COVID-19 publications may have been fast-tracked, the sudden surge in COVID-19 publications might be driven by editorial policy.
Citation: Riccaboni M, Verginer L (2022) The impact of the COVID-19 pandemic on scientific research in the life sciences. PLoS ONE 17(2): e0263001. https://doi.org/10.1371/journal.pone.0263001
Editor: Florian Naudet, University of Rennes 1, FRANCE
Received: April 28, 2021; Accepted: January 10, 2022; Published: February 9, 2022
Copyright: © 2022 Riccaboni, Verginer. This is an open access article distributed under the terms of the Creative Commons Attribution License , which permits unrestricted use, distribution, and reproduction in any medium, provided the original author and source are credited.
Data Availability: The processed data, instructions on how to process the raw PubMed dataset as well as all code are available via Zenodo at https://doi.org/10.5281/zenodo.5121216 .
Funding: The author(s) received no specific funding for this work.
Competing interests: The authors have declared that no competing interests exist.
Introduction
The COVID-19 pandemic has mobilized the world scientific community in 2020, especially in the life sciences [ 1 , 2 ]. In the first three months after the pandemic, the number of scientific papers about COVID-19 was fivefold the number of articles on H1N1 swine influenza [ 3 ]. Similarly, the number of clinical trials related to COVID-19 prophylaxis and treatments skyrocketed [ 4 ]. Thanks to the rapid mobilization of the world scientific community, COVID-19 vaccines have been developed in record time. Despite this undeniable success, there is a rising concern about the negative consequences of COVID-19 on clinical trial research, with many projects being postponed [ 5 – 7 ]. According to Evaluate Pharma, clinical trials were one of the pandemic’s first casualties, with a record number of 160 studies suspended for reasons related to COVID-19 in April 2020 [ 8 , 9 ] reporting a total of 1,200 trials suspended as of July 2020. As a consequence, clinical researchers have been impaired by reduced access to healthcare research infrastructures. Particularly, the COVID-19 outbreak took a tall on women and early-career scientists [ 10 – 13 ]. On a different ground, Shan and colleagues found that non-COVID-19-related articles decreased as COVID-19-related articles increased in top clinical research journals [ 14 ]. Fraser and coworker found that COVID-19 preprints received more attention and citations than non-COVID-19 preprints [ 1 ]. More recently, Hook and Porter have found some early evidence of ‘covidisation’ of academic research, with research grants and output diverted to COVID-19 research in 2020 [ 15 ]. How much should scientists switch their efforts toward SARS-CoV-2 prevention, treatment, or mitigation? There is a growing consensus that the current level of ‘covidisation’ of research can be wasteful [ 4 , 5 , 16 ].
Against this background, in this paper, we investigate if the COVID-19 pandemic has induced a shift in biomedical publications toward COVID-19-related scientific production. The objective of the study is to show that scientific articles listing covid-related Medical Subject Headings (MeSH) when compared against covid-unrelated MeSH have been partially displaced. Specifically, we look at several indicators of scientific production in the life sciences before and after the start of the COVID-19 pandemic: (1) number of papers published, (2) impact factor weighted number of papers, (3) opens access, (4) number of publications related to clinical trials, (5) number of papers listing grants, (6) number of papers listing grants existing before the pandemic. Through a natural experiment approach, we analyze the impact of the pandemic on scientific production in the life sciences. We consider COVID-19 an unexpected and unprecedented exogenous source of variation with heterogeneous effects across biomedical research fields (i.e., MeSH terms).
Based on the difference in difference results, we document the displacement effect that the pandemic has had on several aspects of scientific publishing. The overall picture that emerges from this analysis is that there has been a profound realignment of priorities and research efforts. This shift has displaced biomedical research in fields not related to COVID-19.
The rest of the paper is structured as follows. First, we describe the data and our measure of relatedness to COVID-19. Next, we illustrate the difference-in-differences specification we rely on to identify the impact of the pandemic on scientific output. In the results section, we present the results of the difference-in-differences and network analyses. We document the sudden shift in publications, grants and trials towards COVID-19-related MeSH terms. Finally, we discuss the findings and highlight several policy implications.
Materials and methods
The present analysis is based primarily on PubMed and the Medical Subject Headings (MeSH) terminology. This data is used to estimate the effect of the start of the COVID 19 pandemic via a difference in difference approach. This section is structured as follows. We first introduce the data and then the econometric methodology. This analysis is not based on a pre-registered protocol.
Selection of biomedical publications.
We rely on PubMed, a repository with more than 34 million biomedical citations, for the analysis. Specifically, we analyze the daily updated files up to 31/06/2021, extracting all publications of type ‘Journal Article’. For the principal analysis, we consider 3,638,584 papers published from January 2019 to December 2020. We also analyze 11,122,017 papers published from 2010 onwards to identify the earliest usage of a grant and infer if it was new in 2020. We use the SCImago journal ranking statistics to compute the impact factor weighted number (IFWN) of papers in a given field of research. To assign the publication date, we use the ‘electronically published’ dates and, if missing, the ‘print published’ dates.
Medical subject headings.
We rely on the Medical Subject Headings (MeSH) terminology to approximate narrowly defined biomedical research fields. This terminology is a curated medical vocabulary, which is manually added to papers in the PubMed corpus. The fact that MeSH terms are manually annotated makes this terminology ideal for classification purposes. However, there is a delay between publication and annotation, on the order of several months. To address this delay and have the most recent classification, we search for all 28 425 MeSH terms using PubMed’s ESearch utility and classify paper by the results. The specific API endpoint is https://eutils.ncbi.nlm.nih.gov/entrez/eutils/esearch.fcgi , the relevant scripts are available with the code. For example, we assign the term ‘Ageusia’ (MeSH ID D000370) to all papers listed in the results of the ESearch API. We apply this method to the whole period (January 2019—December 2020) and obtain a mapping from papers to the MeSH terms. For every MeSH term, we keep track of the year they have been established. For instance, COVID-19 terms were established in 2020 (see Table 1 ): in January 2020, the WHO recommended 2019-nCoV and 2019-nCoV acute respiratory disease as provisional names for the virus and disease. The WHO issued the official terms COVID-19 and SARS-CoV-2 at the beginning of February 2020. By manually annotating publications, all publications referring to COVID-19 and SARS-CoV-2 since January 2020 have been labelled with the related MeSH terms. Other MeSH terms related to COVID-19, such as coronavirus, for instance, have been established years before the pandemic (see Table 2 ). We proxy MeSH term usage via search terms using the PubMed EUtilities API; this means that we are not using the hand-labelled MeSH terms but rather the PubMed search results. This means that the accuracy of the MeSH term we assign to a given paper is not perfect. In practice, this means that we have assigned more MeSH terms to a given term than a human annotator would have.
- PPT PowerPoint slide
- PNG larger image
- TIFF original image
https://doi.org/10.1371/journal.pone.0263001.t001
The list contains only terms with at least 100 publications in 2020.
https://doi.org/10.1371/journal.pone.0263001.t002
Clinical trials and publication types.
We classify publications using PubMed’s ‘PublicationType’ field in the XML baseline files (There are 187 publication types, see https://www.nlm.nih.gov/mesh/pubtypes.html ). We consider a publication to be related to a clinical trial if it lists any of the following descriptors:
- D016430: Clinical Trial
- D017426: Clinical Trial, Phase I
- D017427: Clinical Trial, Phase II
- D017428: Clinical Trial, Phase III
- D017429: Clinical Trial, Phase IV
- D018848: Controlled Clinical Trial
- D065007: Pragmatic Clinical Trial
- D000076362: Adaptive Clinical Trial
- D000077522: Clinical Trial, Veterinary
In our analysis of the impact of COVID-19 on publications related to clinical trials, we only consider MeSH terms that are associated at least once with a clinical trial publication over the two years. We apply this restriction to filter out MeSH terms that are very unlikely to be relevant for clinical trial types of research.
Open access.
We proxy the availability of a journal article to the public, i.e., open access, if it is available from PubMed Central. PubMed Central archives full-text journal articles and provides free access to the public. Note that the copyright license may vary across participating publishers. However, the text of the paper is for all effects and purposes freely available without requiring subscriptions or special affiliation.
We infer if a publication has been funded by checking if it lists any grants. We classify grants as either ‘old’, i.e. existed before 2019, or ‘new’, i.e. first observed afterwards. To do so, we collect all grant IDs for 11,122,017 papers from 2010 on-wards and record their first appearance. This procedure is an indirect inference of the year the grant has been granted. The basic assumption is that if a grant number has not been listed in any publication since 2010, it is very likely a new grant. Specifically, an old grant is a grant listed since 2019 observed at least once from 2010 to 2018.
Note that this procedure is only approximate and has a few shortcomings. Mistyped grant numbers (e.g. ‘1234-M JPN’ and ‘1234-M-JPN’) could appear as new grants, even though they existed before, or new grants might be classified as old grants if they have a common ID (e.g. ‘Grant 1’). Unfortunately, there is no central repository of grant numbers and the associated metadata; however, there are plans to assign DOI numbers to grants to alleviate this problem (See https://gitlab.com/crossref/open_funder_registry for the project).
Impact factor weighted publication numbers (IFWN).
In our analysis, we consider two measures of scientific output. First, we simply count the number of publications by MeSH term. However, since journals vary considerably in terms of impact factor, we also weigh the number of publications by the impact factor of the venue (e.g., journal) where it was published. Specifically, we use the SCImago journal ranking statistics to weigh a paper by the impact factor of the journal it appears in. We use the ‘citation per document in the past two years’ for 45,230 ISSNs. Note that a journal may and often has more than one ISSN, i.e., one for the printed edition and one for the online edition. SCImago applies the same score for a venue across linked ISSNs.
For the impact factor weighted number (IFWN) of publication per MeSH terms, this means that all publications are replaced by the impact score of the journal they appear in and summed up.
COVID-19-relatedness.
To measure how closely related to COVID-19 is a MeSH term, we introduce an index of relatedness to COVID-19. First, we identify the focal COVID-19 terms, which appeared in the literature in 2020 (see Table 1 ). Next, for all other pre-existing MeSH terms, we measure how closely related to COVID-19 they end up being.
Our aim is to show that MeSH terms that existed before and are related have experienced a sudden increase in the number of (impact factor weighted) papers.
Intuitively we can read this measure as: what is the probability in 2020 that a COVID-19 MeSH term is present given that we chose a paper with MeSH term i ? For example, given that in 2020 we choose a paper dealing with “Ageusia” (i.e., Complete or severe loss of the subjective sense of taste), there is a 96% probability that this paper also lists COVID-19, see Table 1 .
Note that a paper listing a related MeSH term does not imply that that paper is doing COVID-19 research, but it implies that one of the MeSH terms listed is often used in COVID-19 research.
In sum, in our analysis, we use the following variables:
- Papers: Number of papers by MeSH term;
- Impact: Impact factor weighted number of papers by MeSH term;
- PMC: Papers listed in PubMed central by MeSH term, as a measure of Open Access publications;
- Trials: number of publications of type “Clinical Trial” by MeSH term;
- Grants: number of papers with at least one grant by MeSH term;
- Old Grants: number of papers listing a grant that has been observed between 2010 and 2018, by MeSH term;
Difference-in-differences
The difference-in-differences (DiD) method is an econometric technique to imitate an experimental research design from observation data, sometimes referred to as a quasi-experimental setup. In a randomized controlled trial, subjects are randomly assigned either to the treated or the control group. Analogously, in this natural experiment, we assume that medical subject headings (MeSH) have been randomly assigned to be either treated (related) or not treated (unrelated) by the pandemic crisis.
Before the COVID, for a future health crisis, the set of potentially impacted medical knowledge was not predictable since it depended on the specifics of the emergency. For instance, ageusia (loss of taste), a medical concept existing since 1991, became known to be a specific symptom of COVID-19 only after the pandemic.
Specifically, we exploit the COVID-19 as an unpredictable and exogenous shock that has deeply affected the publication priorities for biomedical scientific production, as compared to the situation before the pandemic. In this setting, COVID-19 is the treatment, and the identification of this new human coronavirus is the event. We claim that treated MeSH terms, i.e., MeSH terms related to COVID-19, have experienced a sudden increase in terms of scientific production and attention. In contrast, research on untreated MeSH terms, i.e., MeSH terms not related to COVID-19, has been displaced by COVID-19. Our analysis compares the scientific output of COVID-19 related and unrelated MeSH terms before and after January 2020.
In our case, some of the terms turn out to be related to COVID-19 in 2020, whereas most of the MeSH terms are not closely related to COVID-19.
Thus β 1 identifies the overall effect on the control group after the event, β 2 the difference across treated and control groups before the event (i.e. the first difference in DiD) and finally the effect on the treated group after the event, net of the first difference, β 3 . This last parameter identifies the treatment effect on the treated group netting out the pre-treatment difference.
For the DiD to have a causal interpretation, it must be noted that pre-event, the trends of the two groups should be parallel, i.e., the common trend assumption (CTA) must be satisfied. We will show that the CTA holds in the results section.
To specify the DiD model, we need to define a period before and after the event and assign a treatment status or level of exposure to each term.
Before and after.
The pre-treatment period is defined as January 2019 to December 2019. The post-treatment period is defined as the months from January 2020 to December 2020. We argue that the state of biomedical research was similar in those two years, apart from the effect of the pandemic.
Treatment status and exposure.
The treatment is determined by the COVID-19 relatedness index σ i introduced earlier. Specifically, this number indicates the likelihood that COVID-19 will be a listed MeSH term, given that we observe the focal MeSH term i . To show that the effect becomes even stronger the closer related the subject is, and for ease of interpretation, we also discretize the relatedness value into three levels of treatment. Namely, we group MeSH terms with a σ between, 0% to 20%, 20% to 80% and 80% to 100%. The choice of alternative grouping strategies does not significantly affect our results. Results for alternative thresholds of relatedness can be computed using the available source code. We complement the dichotomized analysis by using the treatment intensity (relatedness measure σ ) to show that the result persists.
Panel regression.
In this work, we estimate a random effects panel regression where the units of analysis are 28 318 biomedical research fields (i.e. MeSH terms) observed over time before and after the COVID-19 pandemic. The time resolution is at the monthly level, meaning that for each MeSH term, we have 24 observations from January 2019 to December 2020.
The outcome variable Y it identifies the outcome at time t (i.e., month), for MeSH term i . As before, P t identifies the period with P t = 0 if the month is before January 2020 and P t = 1 if it is on or after this date. In (3) , the treatment level is measure by the relatedness to COVID-19 ( σ i ), where again the γ 1 identifies pre-trend (constant) differences and δ 1 the overall effect.
In total, we estimate six coefficients. As before, the δ l coefficient identifies the DiD effect.
Verifying the Common Trend Assumption (CTA).
We show that the CTA holds for this model by comparing the pre-event trends of the control group to the treated groups (COVID-19 related MeSH terms). Namely, we show that the pre-event trends of the control group are the same as the pre-event trends of the treated group.
Co-occurrence analysis
To investigate if the pandemic has caused a reconfiguration of research priorities, we look at the MeSH term co-occurrence network. Precisely, we extract the co-occurrence network of all 28,318 MeSH terms as they appear in the 3.3 million papers. We considered the co-occurrence networks of 2018, 2019 and 2020. Each node represents a MeSH term in these networks, and a link between them indicates that they have been observed at least once together. The weight of the edge between the MeSH terms is given by the number of times those terms have been jointly observed in the same publications.
Medical language is hugely complicated, and this simple representation does not capture the intricacies, subtle nuances and, in fact, meaning of the terms. Therefore, we do not claim that we can identify how the actual usage of MeSH terms has changed from this object, but rather that it has. Nevertheless, the co-occurrence graph captures rudimentary relations between concepts. We argue that absent a shock to the system, their basic usage patterns, change in importance (within the network) would essentially be the same from year to year. However, if we find that the importance of terms changes more than expected in 2020, it stands to reason that there have been some significant changes.
To show that that MeSH usage has been affected, we compute for each term in the years 2018, 2019 and 2020 their PageRank centrality [ 17 ]. The PageRank centrality tells us how likely a random walker traversing a network would be found at a given node if she follows the weights of the empirical edges (i.e., co-usage probability). Specifically, for the case of the MeSH co-occurrence network, this number represents how often an annotator at the National Library of Medicine would assign that MeSH term following the observed general usage patterns. It is a simplistic measure to capture the complexities of biomedical research. Nevertheless, it captures far-reaching interdependence across MeSH terms as the measure uses the whole network to determine the centrality of every MeSH term. A sudden change in the rankings and thus the position of MeSH terms in this network suggests that a given research subject has risen as it is used more often with other important MeSH terms (or vice versa).
We then compare the growth for each MeSH i term in g i (2019), i.e. before the the COVID-19 pandemic, with the growth after the event ( g i (2020)).
Publication growth
Changes in output and COVID-19 relatedness
Before we show the regression results, we provide descriptive evidence that publications from 2019 to 2020 have drastically increased. By showing that this growth correlates strongly with a MeSH term’s COVID-19 relatedness ( σ ), we demonstrate that (1) σ captures an essential aspect of the growth dynamics and (2) highlight the meteoric rise of highly related terms.
We look at the year over year growth in the number of the impact weighted number of publications per MeSH term from 2018 to 2019 and 2019 to 2020 as defined in the methods section.
Fig 1 shows the yearly growth of the impact weighted number of publications per MeSH term. By comparing the growth of the number of publications from the years 2018, 2019 and 2020, we find that the impact factor weighted number of publications has increased by up to a factor of 100 compared to the previous year for Betacoronavirus, one of the most closely related to COVID-19 MeSH term.
Each dot represents, a MeSH term. The y axis (growth) is in symmetric log scale. The x axis shows the COVID-19 relatedness, σ . Note that the position of the dots on the x-axis is the same in the two plots. Below: MeSH term importance gain (PageRank) and their COVID-19 relatedness.
https://doi.org/10.1371/journal.pone.0263001.g001
Fig 1 , first row, reveals how strongly correlated the growth in the IFWN of publication is to the term’s COVID-19 relatedness. For instance, we see that the term ‘Betacoronavirus’ skyrocketed from 2019 to 2020, which is expected given that SARS-CoV-2 is a species of the genus. Conversely, the term ‘Alphacoronavirus’ has not experienced any growth given that it is twin a genus of the Coronaviridae family, but SARS-CoV-2 is not one of its species. Note also the fast growth in the number of publications dealing with ‘Quarantine’. Moreover, MeSH terms that grew significantly from 2018 to 2019 and were not closely related to COVID-19, like ‘Vaping’, slowed down in 2020. From the graph, the picture emerges that publication growth is correlated with COVID-19 relatedness σ and that the growth for less related terms slowed down.
To show that the usage pattern of MeSH terms has changed following the pandemic, we compute the PageRank centrality using graph-tool [ 18 ] as discussed in the Methods section.
Fig 1 , second row, shows the change in the PageRank centrality of the MeSH terms after the pandemic (2019 to 2020, right plot) and before (2018 to 2019, left plot). If there were no change in the general usage pattern, we would expect the variance in PageRank changes to be narrow across the two periods, see (left plot). However, PageRank scores changed significantly more from 2019 to 2020 than from 2018 to 2019, suggesting that there has been a reconfiguration of the network.
To further support this argument, we carry out a DiD regression analysis.
Common trends assumption
As discussed in the Methods section, we need to show that the CTA assumption holds for the DiD to be defined appropriately. We do this by estimating for each month the number of publications and comparing it across treatment groups. This exercise also serves the purpose of a placebo test. By assuming that each month could have potentially been the event’s timing (i.e., the outbreak), we show that January 2020 is the most likely timing of the event. The regression table, as noted earlier, contains over 70 estimated coefficients, hence for ease of reading, we will only show the predicted outcome per month by group (see Fig 2 ). The full regression table with all coefficients is available in the S1 Table .
The y axis is in log scale. The dashed vertical line identifies January 2020. The dashed horizontal line shows the publications in January 2019 for the 0–20% group before the event. This line highlights that the drop happens after the event. The bands around the lines indicate the 95% confidence interval of the predicted values. The results are the output of the Stata margins command.
https://doi.org/10.1371/journal.pone.0263001.g002
Fig 2 shows the predicted number per outcome variable obtained from the panel regression model. These predictions correspond to the predicted value per relatedness group using the regression parameters estimated via the linear panel regression. The bands around the curves are the 95% confidence intervals.
All outcome measures depict a similar trend per month. Before the event (i.e., January 2020), there is a common trend across all groups. In contrast, after the event, we observe a sudden rise for the outcomes of the COVID-19 related treated groups (green and red lines) and a decline in the outcomes for the unrelated group (blue line). Therefore, we can conclude that the CTA assumption holds.
Regression results
Table 3 shows the DiD regression results (see Eq (3) ) for the selected outcome measures: number of publications (Papers), impact factor weighted number of publications (Impact), open access (OA) publications, clinical trial related publications, and publications with existing grants.
https://doi.org/10.1371/journal.pone.0263001.t003
Table 3 shows results for the discrete treatment level version of the DiD model (see Eq (4) ).
Note that the outcome variable is in natural log scale; hence to get the effect of the independent variable, we need to exponentiate the coefficient. For values close to 0, the effect is well approximated by the percentage change of that magnitude.
In both specifications we see that the least related group, drops in the number of publications between 10% and 13%, respectively (first row of Tables 3 and 4 , exp(−0.102) ≈ 0.87). In line with our expectations, the increase in the number of papers published by MeSH term is positively affected by the relatedness to COVID-19. In the discrete model (row 2), we note that the number of documents with MeSH terms with a COVID-19 relatedness between 20 and 80% grows by 18% and highly related terms by a factor of approximately 6.6 (exp(1.88)). The same general pattern can be observed for the impact weighted publication number, i.e., Model (2). Note, however, that the drop in the impact factor weighted output is more significant, reaching -19% for COVID-19 unrelated publications, and related publications growing by a factor of 8.7. This difference suggests that there might be a bias to publish papers on COVID-19 related subjects in high impact factor journals.
https://doi.org/10.1371/journal.pone.0263001.t004
By looking at the number of open access publications (PMC), we note that the least related group has not been affected negatively by the pandemic. However, the number of COVID-19 related publications has drastically increased for the most COVID-19 related group by a factor of 6.2. Note that the substantial increase in the number of papers available through open access is in large part due to journal and editorial policies to make preferentially COVID research immediately available to the public.
Regarding the number of clinical trial publications, we note that the least related group has been affected negatively, with the number of publications on clinical trials dropping by a staggering 24%. At the same time, publications on clinical trials for COVID-19-related MeSH have increased by a factor of 2.1. Note, however, that the effect on clinical trials is not significant in the continuous regression. The discrepancy across Tables 3 and 4 highlights that, especially for trials, the effect is not linear, where only the publications on clinical trials closely related to COVID-19 experiencing a boost.
It has been reported [ 19 ] that while the number of clinical trials registered to treat or prevent COVID-19 has surged with 179 new registrations in the second week of April 2020 alone. Only a few of these have led to publishable results in the 12 months since [ 20 ]. On the other hand, we find that clinical trial publications, considering related MeSH (but not COVID-19 directly), have had significant growth from the beginning of the pandemic. These results are not contradictory. Indeed counting the number of clinical trial publications listing the exact COVID-19 MeSH term (D000086382), we find 212 publications. While this might seem like a small number, consider that in 2020 only 8,485 publications were classified as clinical trials; thus, targeted trials still made up 2.5% of all clinical trials in 2020 . So while one might doubt the effectiveness of these research efforts, it is still the case that by sheer number, they represent a significant proportion of all publications on clinical trials in 2020. Moreover, COVID-19 specific Clinical trial publications in 2020, being a delayed signal of the actual trials, are a lower bound estimate on the true number of such clinical trials being conducted. This is because COVID-19 studies could only have commenced in 2020, whereas other studies had a head start. Thus our reported estimates are conservative, meaning that the true effect on actual clinical trials is likely larger, not smaller.
Research funding, as proxied by the number of publications with grants, follows a similar pattern, but notably, COVID-19-related MeSH terms list the same proportion of grants established before 2019 as other unrelated MeSH terms, suggesting that grants which were not designated for COVID-19 research have been used to support COVID-19 related research. Overall, the number of publications listing a grant has dropped. Note that this should be because the number of publications overall in the unrelated group has dropped. However, we note that the drop in publications is 10% while the decline in publications with at least one grant is 15%. This difference suggests that publications listing grants, which should have more funding, are disproportionately COVID-19 related papers. To further investigate this aspect, we look at whether the grant was old (pre-2019) or appeared for the first time in or after 2019. It stands to reason that an old grant (pre-2019) would not have been granted for a project dealing with the pandemic. Hence we would expect that COVID-19 related MeSH terms to have a lower proportion of old grants than the unrelated group. In models (6) in Table 4 we show that the number of old grants for the unrelated group drops by 13%. At the same time, the number of papers listing old grants (i.e., pre-2019) among the most related group increased by a factor of 3.1. Overall, these results suggest that COVID-19 related research has been funded largely by pre-existing grants, even though a specific mandate tied to the grants for this use is unlikely.
The scientific community has swiftly reallocated research efforts to cope with the COVID-19 pandemic, mobilizing knowledge across disciplines to find innovative solutions in record time. We document this both in terms of changing trends in the biomedical scientific output and the usage of MeSH terms by the scientific community. The flip side of this sudden and energetic prioritization of effort to fight COVID-19 has been a sudden contraction of scientific production in other relevant research areas. All in all, we find strong support to the hypotheses that the COVID-19 crisis has induced a sudden increase of research output in COVID-19 related areas of biomedical research. Conversely, research in areas not related to COVID-19 has experienced a significant drop in overall publishing rates and funding.
Our paper contributes to the literature on the impact of COVID-19 on scientific research: we corroborate previous findings about the surge of COVID-19 related publications [ 1 – 3 ], partially displacing research in COVID-19 unrelated fields of research [ 4 , 14 ], particularly research related to clinical trials [ 5 – 7 ]. The drop in trial research might have severe consequences for patients affected by life-threatening diseases since it will delay access to new and better treatments. We also confirm the impact of COVID-19 on open access publication output [ 1 ]; also, this is milder than traditional outlets. On top of this, we provide more robust evidence on the impact weighted effect of COVID-19 and grant financed research, highlighting the strong displacement effect of COVID-19 on the allocation of financial resources [ 15 ]. We document a substantial change in the usage patterns of MeSH terms, suggesting that there has been a reconfiguration in the way research terms are being combined. MeSH terms highly related to COVID-19 were peripheral in the MeSH usage networks before the pandemic but have become central since 2020. We conclude that the usage patterns have changed, with COVID-19 related MeSH terms occupying a much more prominent role in 2020 than they did in the previous years.
We also contribute to the literature by estimating the effect of COVID-19 on biomedical research in a natural experiment framework, isolating the specific effects of the COVID-19 pandemic on the biomedical scientific landscape. This is crucial to identify areas of public intervention to sustain areas of biomedical research which have been neglected during the COVID-19 crisis. Moreover, the exploratory analysis on the changes in usage patterns of MeSH terms, points to an increase in the importance of covid-related topics in the broader biomedical research landscape.
Our results provide compelling evidence that research related to COVID-19 has indeed displaced scientific production in other biomedical fields of research not related to COVID-19, with a significant drop in (impact weighted) scientific output related to non-COVID-19 and a marked reduction of financial support for publications not related to COVID-19 [ 4 , 5 , 16 ]. The displacement effect is persistent to the end of 2020. As vaccination progresses, we highlight the urgent need for science policy to re-balance support for research activity that was put on pause because of the COVID-19 pandemic.
We find that COVID-19 dramatically impacted clinical research. Reactivation of clinical trials activities that have been postponed or suspended for reasons related to COVID-19 is a priority that should be considered in the national vaccination plans. Moreover, since grants have been diverted and financial incentives have been targeted to sustain COVID-19 research leading to an excessive entry in COVID-19-related clinical trials and the ‘covidisation’ of research, there is a need to reorient incentives to basic research and otherwise neglected or temporally abandoned areas of biomedical research. Without dedicated support in the recovery plans for neglected research of the COVID-19 era, there is a risk that more medical needs will be unmet in the future, possibly exacerbating the shortage of scientific research for orphan and neglected diseases, which do not belong to COVID-19-related research areas.
Limitations
Our empirical approach has some limits. First, we proxy MeSH term usage via search terms using the PubMed EUtilities API. This means that the accuracy of the MeSH term we assign to a given paper is not fully validated. More time is needed for the completion of manually annotated MeSH terms. Second, the timing of publication is not the moment the research has been carried out. There is a lead time between inception, analysis, write-up, review, revision, and final publication. This delay varies across disciplines. Nevertheless, given that the surge in publications happens around the alleged event date, January 2020, we are confident that the publication date is a reasonable yet imperfect estimate of the timing of the research. Third, several journals have publicly declared to fast-track COVID-19 research. This discrepancy in the speed of publication of COVID-19 related research and other research could affect our results. Specifically, a surge or displacement could be overestimated due to a lag in the publication of COVID-19 unrelated research. We alleviate this bias by estimating the effect considering a considerable time after the event (January 2020 to December 2020). Forth, on the one hand, clinical Trials may lead to multiple publications. Therefore we might overestimate the impact of COVID-19 on the number of clinical trials. On the other hand, COVID-19 publications on clinical trials lag behind, so the number of papers related COVID-19 trials is likely underestimated. Therefore, we note that the focus of this paper is scientific publications on clinical trials rather than on actual clinical trials. Fifth, regarding grants, unfortunately, there is no unique centralized repository mapping grant numbers to years, so we have to proxy old grants with grants that appeared in publications from 2010 to 2018. Besides, grant numbers are free-form entries, meaning that PubMed has no validation step to disambiguate or verify that the grant number has been entered correctly. This has the effect of classifying a grant as new even though it has appeared under a different name. We mitigate this problem by using a long period to collect grant numbers and catch many spellings of the same grant, thereby reducing the likelihood of miss-identifying a grant as new when it existed before. Still, unless unique identifiers are widely used, there is no way to verify this.
So far, there is no conclusive evidence on whether entry into COVID-19 has been excessive. However, there is a growing consensus that COVID-19 has displaced, at least temporally, scientific research in COVID-19 unrelated biomedical research areas. Even though it is certainly expected that more attention will be devoted to the emergency during a pandemic, the displacement of biomedical research in other fields is concerning. Future research is needed to investigate the long-run structural consequences of the COVID-19 crisis on biomedical research.
Supporting information
S1 table. common trend assumption (cta) regression table..
Full regression table with all controls and interactions.
https://doi.org/10.1371/journal.pone.0263001.s001
- View Article
- Google Scholar
- PubMed/NCBI
- 8. Brown A, Edwin E, Fagg J. Evaluate Pharma 2021 Preview; 2020. https://www.evaluate.com/thought-leadership/vantage/evaluate-vantage-2021-preview .
- 15. Hook D, Porter S. The COVID Brain Drain; 2020. https://www.natureindex.com/news-blog/covid-brain-drain-scientific-research .
- 17. Page L, Brin S, Motwani R, Winograd T. The PageRank citation ranking: Bringing order to the web. Stanford InfoLab; 1999.
- Search Search
- CN (Chinese)
- DE (German)
- ES (Spanish)
- FR (Français)
- JP (Japanese)
- Open science
- Booksellers
- Peer Reviewers
- Springer Nature Group ↗
Coronavirus (COVID-19) Research Highlights
On the 5th may 2023 the who declared an end to the public health emergency of international concern for covid-19. this page remains as a point of reference for interested parties..
Springer Nature is committed to supporting the global response to COVID-19 enabling fast and direct access to the latest available research, evidence, and data.
The need for continued access to research and learning has never been more important. We recognise our role in this and we continue to work with global organisations, such as the World Health Organisation and the initiative from the White House Office of Science and Technology to make all relevant global research, and data, immediately available. We also continue to work directly with teachers, lecturers, librarians, students and institutions to support their work. See more from our CEO, Frank Vrancken Peeters on our response to the pandemic.
Across the pandemic* we:
- Enabled free access to over 70,000 articles, book chapters, reference works and protocols on our platforms
- Published over 67,000 new COVID-19 articles and supported all researchers in making their underlying experimental data sets available for free and re-use
- Made available, via Research Square, over 10,000 COVID-19 preprint articles
Below, you will find links to key resources, free content and updated information related to COVID-19, alongside key policies and information in supporting remote access and working. Please contact our Customer Services Team if you require further support.
* counted as 2019-2021
Free access to COVID-19 journal and book content
Remote access: information & resources for librarians, remote access: resources for institutions and students, remote access: learn about remote access for researchers, report: impact of covid-19 on the research enterprise, supporting covid-19 research, how can ai support the research community in times of crisis.
Learn how AI was used to build a rapid prototype report to give a quick and simple overview of coronavirus research.
In Review and Preprints
Springer Nature encourages early sharing of research submitted to all our journals through preprints, and our In Review preprint service is available for many journals.
For API purposes, we have made additional content free via the SN OpenAccess API.
Access COVID-19 Research Papers & Articles Across Journals, Books & More
Featured research, reviews and comment.
from
| from
| from
| from
|
from
| from
| from
|
Books and Chapters
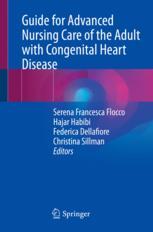
- More highlighted COVID-19 articles and chapters
- Explore registered COVID-19 Research Highlights Trials
- Access COVID-19 datasets
- Discover our Article Latest Collections
Access more COVID-19 research on our platforms
A pioneer of open access, BMC’s journals include broad scope titles such as and and more specialist journals such as and . This collection draws together coronavirus research from across BMC.
|
To support research to combat the COVID-19 outbreak, have opened a collection covering the biology of coronavirus infection, its detection, treatment and evolution, research into the epidemiology of emerging viral diseases, and coverage of current events.
|
In response to the COVID-19 pandemic, Springer have made corona-related research free to access. This content spans across our journals, books, protocols, conference papers, and other content types.
|
from
| from
| from
| from
|
from
| from
| from
| from
|
Featured blog posts from "The Source" blog See all
Providing insight and discussion from researchers during the pandemic
Research in the time of a pandemic: SENTINEL- A proactive, early warning system to pre-empt future pandemics
Dr Sabeti and Dr Happi talk about building a pandemic preemption system to safeguard us from future pandemics.
Research in the time of a pandemic: Coronavirus, Class and Mutual Aid in the UK
In this week's blog we discuss how the economic systems in place played a role into how the pandemic has developed.
Research in the time of a pandemic: Africa Centres for Disease Control and Prevention
Read about the Centres for Disease Control and Prevention in Africa and their role during the pandemic.
Research in the time of a pandemic: Leading the Oxford Vaccine Trial
Dr Sarah Gilbert, the lead for the Oxford Vaccine Trial for COVID-19 talks about the vaccine.
Read our blogs
The brain complications of COVID-19
The clinical trials fighting COVID-19: vaccines, testing and other conditions
How is the WHO trial registry network responding to the COVID-19 pandemic?
The clinical trials fighting COVID-19: platform and repurposed drug trials
Keep up with the latest news on covid-19 research, nature briefing.
Sign up to a free daily update on COVID-19, plus a selection of the most important non-coronavirus science news
Nature provides authoritative, timely news and opinion articles on the novel coronavirus and COVID-19
Scientific American International
Scientific American’s international network of magazines now brings you the latest science news and expert analysis on the pandemic in one place

Additional Resources
Adisinsight.
AdisInsight provides profiles of drug development programs actively investigating treatments for Coronavirus and summaries of the clinical trials being conducted for those drugs.
Nano – a Nature Research Solution
Access manually extracted nanomaterial summaries in addition to thousands of freely available patents and article links on the interaction, diagnostics and therapeutic effects of nanomaterials on coronavirus.
Springer Nature Experiments
Covers over 230 well established protocols and methods for identifying & detecting coronavirus, protocols on designing the vaccine for the epidemic, understanding the ultrastructure of viruses to design new drugs.
External resources
|
|
|
Stay up to date
Here to foster information exchange with the library community
Connect with us on LinkedIn and stay up to date with news and development.
- Tools & Services
- Account Development
- Sales and account contacts
- Professional
- Press office
- Locations & Contact
We are a world leading research, educational and professional publisher. Visit our main website for more information.
- © 2024 Springer Nature
- General terms and conditions
- Your US State Privacy Rights
- Your Privacy Choices / Manage Cookies
- Accessibility
- Legal notice
- Help us to improve this site, send feedback.
- Open access
- Published: 02 September 2024
Interaction effects of the COVID-19 pandemic and regional deprivation on self-rated health: a cross-sectional study
- Hajae Jeon 1 ,
- Junbok Lee 2 ,
- Mingee Choi 3 ,
- Bomgyeol Kim 1 ,
- Sang Gyu Lee 3 &
- Jaeyong Shin 3
BMC Public Health volume 24 , Article number: 2382 ( 2024 ) Cite this article
Metrics details
Recent studies have attempted to analyze the changes in self-rated health (SRH) during the coronavirus disease 2019 (COVID-19) pandemic. However, the results have been inconsistent. Notably, SRH is subjective, and responses may vary across and within countries because of sociocultural differences. Thus, we aimed to examine whether the interaction effects between the COVID-19 pandemic and regional deprivation influenced SRH in South Korea.
The study population comprised 877,778 participants from the Korea Community Health Survey. The data were collected from 2018 to 2021. Multiple regression analysis was employed to determine the relationship between SRH and the interaction between the COVID-19 pandemic status and the socioeconomic level of residential areas.
The post-pandemic groups (odds ratio [OR] = 2.25, P < .0001; OR = 2.29, P < .0001) had significantly higher odds of reporting favorable SRH than the pre-pandemic groups (OR = 0.96, P < .0001). However, the difference in ORs based on regional socioeconomic status was small.
Conclusions
SRH showed an overall increase in the post-pandemic groups relative to that in the disadvantaged pre-pandemic group. Possible reasons include changes in individuals’ health perceptions through social comparison and the effective implementation of COVID-19 containment measures in South Korea. This paradoxical phenomenon has been named the “Eye of the Hurricane,” as the vast majority of people who had not been infected by the virus may have viewed their health situation more favorably than they ordinarily would.
Peer Review reports
The coronavirus disease 2019 (COVID-19) pandemic considerably affected daily life [ 1 , 2 , 3 , 4 ]. To prevent the spread of COVID-19, various measures such as social distancing, telecommuting, and restrictions on private gatherings were implemented, leading to social disruption and isolation. In South Korea, this response included strict compliance with social distancing guidelines with concomitant extensive testing, contact tracing, and isolation of confirmed cases [ 5 , 6 ] (see Appendix 1 ). The COVID-19 outbreak and the consequent disruption of daily life generated stress and adversely affected the mental and physical well-being of individuals by reducing social contact [ 3 , 4 ]. Therefore, investigating the effect of the COVID-19 pandemic on health-related indicators will provide important insights into relevant health measures to improve health.
Self-rated health (SRH) is the most common health measure used in large population surveys. SRH primarily captures individuals’ subjective assessments of their health, although it is also linked to objective health conditions. SRH is an important indicator because it is widely used to examine patterns and disparities in population health in relation to socioeconomic factors [ 7 , 8 ]. SRH is influenced by a range of complex factors, encompassing the physical characteristics of the shared environment, including walkability, accessibility of public transportation, and availability of healthcare services; socioeconomic and sociocultural characteristics of the local community, and biological and genetic traits of the individuals [ 9 , 10 , 11 ].
In a previous study, Tak explored the correlation between regional deprivation levels and the SRH of residents in South Korea while considering the moderating effect of neighborhood relationships [ 12 ]. The study revealed notable disparities in health outcomes across various regions. Studies have also analyzed the relationship between physical activity and SRH to understand the changes in health levels resulting from lifestyle modifications and the decline in quality of life during the COVID-19 pandemic [ 13 ]. However, these studies primarily focused on lifestyle modifications and did not specifically investigate the differences between the pre- and post-pandemic periods, indicating a limitation in their scope.
Conversely, studies conducted abroad have shown that SRH, which is an integrated evaluation of one’s physical, mental, social, and functional health, tended to improve following the COVID-19 pandemic, despite the pandemic’s negative impact on mental and social health [ 7 , 14 , 15 ]. Notably, SRH is subjective, and responses may vary across and within countries because of sociocultural differences [ 16 ]. The impact on the health and well-being of populations is anticipated to differ across countries because of variations in COVID-19 prevalence and regulations as well as pre-existing disparities in well-being and healthcare systems prior to the onset of the pandemic [ 7 , 17 ]. Therefore, gaps exist in the current literature, and they highlight the need to investigate and analyze the socioeconomic factors and SRH in South Korea before and after the COVID-19 pandemic while considering the changes in lifestyle patterns resulting from the pandemic.
To address such gaps, we aimed to determine whether the interaction effects between the COVID-19 pandemic and regional deprivation influenced SRH in South Korea.
Study design and setting
This cross-sectional study used data from the Korea Community Health Survey (KCHS) conducted in 2018, 2019, 2020, and 2021 by the Korea Disease Control and Prevention Agency to confirm the interaction effects between the COVID-19 pandemic and regional deprivation on SRH. The KCHS is conducted annually from August 16 to October 31 by public health centers nationwide and targets adults aged 19 years and older. Trained surveyors visit sample households selected using stratified cluster sampling and conduct one-on-one interviews (or electronic surveys) with the final sample households. The survey comprises household and individual components. The household component includes variables such as household type and household income. The individual components include variables related to health behaviors, medical service utilization, prevalent diseases, vaccination, accidents and poisoning, activity limitations and quality of life, healthcare facility utilization, education, employment status, women’s health, cardiopulmonary resuscitation, and socio-physical environmental factors [ 18 , 19 , 20 , 21 ].
In addition to the KCHS data, we used data from the Population and Housing Census. This is a basic statistical survey conducted by the government to determine the size and characteristics of the South Korean population and their housing. Although statistics on population, households, and housing based on administrative data using the registration census are produced annually, a field survey is conducted every five years to collect the practical data needed for policymaking on welfare, economy, transportation, and so on in each region; finally, a 20% sample of all households in South Korea is selected for the field survey. As this study used publicly available data that lacked personal identifiers, institutional review board or ethics committee approval was not sought.
Participants
The participants were South Koreans aged 19 years or older living in 17 cities and counties in South Korea, who participated in the 2018–2021 KCHS. A total of 915,950 adults (228,340 in 2018; 229,099 in 2019; 229,269 in 2020; 229,242 in 2021) completed the survey. A total of 38,172 observations (approximately 4%) with missing data on the outcome variable were excluded from the analyses. A total of 877,778 eligible participants (214,929 in 2018; 219,938 in 2019; 219,907 in 2020; 223,004 in 2021) were included in the analysis (Fig. 1 ).
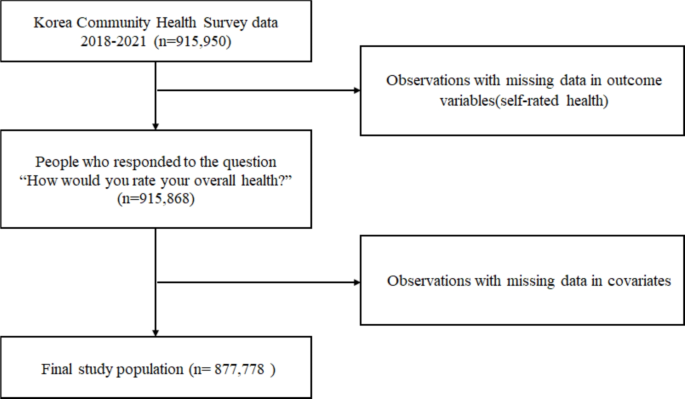
Selection process of the study population
Dependent variable
The dependent variable in this study, SRH, consists of a single item. This measurement method is one of the most widely employed approaches to assess general health status in health research. It is relatively simple to measure and allows for international comparisons. SRH was assessed using the question, “How would you rate your overall health?” with the following response options: “very good,” “good,” “fair,” “poor,” and “very poor.” Based on previous studies, we classified (1) individuals who responded “very good” or “good” as the “high” group and (2) individuals who responded “fair,” “poor,” or “very poor” as the “low” group. Participants who responded with “refusal” or “don’t know” were not included in the analysis.
Variable of interest
The variable of interest was the interaction between the COVID-19 pandemic (pre-COVID-19/post-COVID-19) and regional socioeconomic level. As the first case of COVID-19 in South Korea was diagnosed on January 20, 2020, the years 2018 and 2019 were classified as pre-COVID-19, whereas 2020 and 2021 were classified as post-COVID-19 [ 22 ]. The socioeconomic status of the region was measured using the neighborhood deprivation index. This index extends the traditional concept of poverty, which is defined in terms of resource deprivation or material needs, by including non-monetary resources such as capabilities and social participation to measure multidimensional deprivation in a community [ 23 ]. The level of community deprivation was categorized into below-average (advantaged) and above-average (disadvantaged) groups based on the national average neighborhood deprivation index.
We used data from the 2015 Population and Housing Census to calculate the neighborhood deprivation index. The index was calculated based on nine indicators (low social class, deteriorated housing environment, low educational level, car non-ownership, single-person households, divorced or separated status, female-headed households, older population, and non-residence in apartments), and standardized z-scores were calculated for each indicator. The z-scores were then summed to obtain the overall index. This index was applied at the administrative district level [ 23 ].
The interaction variable between the COVID-19 pandemic and regional socioeconomic level (COVID-19–neighborhood deprivation) was categorized into four groups based on the COVID-19 pandemic status and regional socioeconomic level. The categories are as follows: pre-COVID-19–advantaged (“pre in advantaged”), pre-COVID-19–disadvantaged (“pre in disadvantaged”), post-COVID-19–advantaged (“post in advantaged”), and post-COVID-19–disadvantaged (“post in disadvantaged”) [ 24 , 25 ].
Other covariates were considered, including the participants’ sociodemographic status (gender, age, income level, and employment status) and other related factors that could affect SRH, such as perceived stress, experiences of depressive symptoms, alcohol consumption, smoking status, physical activity, experiences of hypertension, and experiences of diabetes.
Statistical analysis
Descriptive statistics of SRH were presented based on the participants’ demographic and socioeconomic characteristics. Chi-square tests were conducted to examine differences in SRH based on the participants’ characteristics, presence of the COVID-19 pandemic, and socioeconomic status of participants’ residential areas. Multivariate regression analysis was employed to determine the relationship between SRH and the interaction of the COVID-19 pandemic status with the socioeconomic level of residential areas. All statistical analyses were performed using SAS version 9.4 (SAS Institute Inc., Cary, NC, USA).
Table 1 presents the general characteristics of the participants. Of the 877,778 participants, 39.6% ( n = 347,469) exhibited high SRH. The proportion of individuals who rated their health as high varied depending on the interaction between the COVID-19 pandemic and the socioeconomic level of their residential areas. Specifically, in the “pre in disadvantaged” group, the proportion of individuals with high SRH was the lowest at 31.4% ( n = 61,588). In the “post in advantaged” group, the proportion of individuals with high SRH was the highest at 47.7% ( n = 116,143).
Table 2 presents the logistic regression results regarding factors related to SRH, with a focus on the effects of COVID-19 and neighborhood deprivation. Compared with the “pre in disadvantaged” group, the “pre in advantaged” group exhibited lower odds of reporting a favorable SRH (0.96 [95% CI 0.94–0.98; P < .0001]). Meanwhile, the “post in disadvantaged” group (2.25 [95% CI 2.17–2.34; P < .0001]) and “post in advantaged” group (2.29 [95% CI 2.21–2.38; P < .0001]) showed significantly higher odds of reporting a favorable SRH compared with the “pre in disadvantaged” group.
These findings indicated a notable increase in the ORs for reporting a favorable SRH in the post-COVID-19 period. However, there were marginal differences in ORs based on the regional socioeconomic level, suggesting minimal disparities in SRH with respect to regional socioeconomic factors.
To evaluate the additional risk posed by the interaction between COVID-19 and regional deprivation on SRH, we conducted further analyses presented in Appendix 2 . These analyses applied measures such as the Relative Excess Risk due to Interaction (RERI), Attributable Proportion due to Interaction (AP), and Synergy Index (SI). The RERI value of 0.04 (95% CI: 0.04–0.05) suggested an additional risk when both factors were present. The AP value of 0.03 (95% CI: 0.02–0.03) represented the proportion of risk attributable to the interaction, while the SI value of 1.07 (95% CI: 1.07–1.08) indicated a positive interaction between the two factors.
We analyzed SRH in relation to the occurrence of the COVID-19 pandemic and regional socioeconomic level. By comparing the average SRH before and after the COVID-19 pandemic, with the “pre in disadvantaged” group as the reference, we observed increased odds of reporting high SRH after the COVID-19 pandemic. However, we found minimal or inconclusive differences in SRH based on the regional socioeconomic level. This result suggests that the impact of the COVID-19 pandemic may have overshadow any regional differences in socioeconomic levels on SRH.
Additionally, we conducted subgroup analyses according to age and income levels to further explore these relationships. As shown in Appendix 3 , for individuals aged ≥ 60 years, the OR for reporting high SRH was 1.15 (95% CI 1.11–1.19; P < .0001) in the “pre in advantaged” group, 2.44 (95% CI 2.31–2.59; P < .0001) in the “post in disadvantaged” group, and 2.77 (95% CI 2.62–2.93; P < .0001) in the “post in advantaged” group, relative to the “pre in disadvantaged” group. This indicates that the impact of regional deprivation on SRH significantly varies across different age groups, with older adults showing more pronounced differences.
Similarly, among individuals in the lowest income quartile, the OR for reporting high SRH was 1.10 (95% CI 1.05–1.15; P < .0001) in the “pre in advantaged” group, 2.65 (95% CI 2.45–2.87; P < .0001) in the “post in disadvantaged” group, and 3.01 (95% CI 2.78–3.26; P < .0001) in the “post in advantaged” group, relative to the “pre in disadvantaged” group (Appendix 4 ). This indicates that income level influences the relationship between regional deprivation and SRH, with the lowest income group showing the most substantial differences. These findings suggest that the health-related variables we adjusted for critically influence the relationship between regional deprivation and SRH. Therefore, it is essential to consider these variables in order to elucidate the nuanced effects of regional deprivation on SRH. Moreover, we conducted additional analyses with different sets of covariates to understand their effects, with the results of these analyses being presented in Appendix 5 .
Additionally, we conducted further analyses to examine the interaction effect between the COVID-19 pandemic and neighborhood deprivation on SRH (Appendix 2 ). The findings indicated that the combined effect of the COVID-19 pandemic and neighborhood deprivation on SRH is greater than the sum of their individual effects. This highlights the importance of considering interaction effects in understanding health disparities during the COVID-19 pandemic.
Individuals’ positive health ratings during the pandemic have various explanations. First, individuals’ health perceptions could have changed because of social comparisons, as people tend to evaluate their health by comparing themselves with others [ 26 ]. The survey conducted in this study targeted individuals who had not contracted COVID-19. Individuals who had not been infected with the virus may have evaluated themselves more positively than they would under normal circumstances.
Furthermore, previous studies involving individuals who had not contracted COVID-19 have shown relatively high rates of improved SRH after the pandemic rather than a decline in SRH [ 7 , 14 , 15 ]. A study in France described this finding as the “Eye of the Hurricane” paradox, suggesting that individuals who had not been infected with COVID-19 may have assessed their health more positively than they typically would [ 15 ]. In a study with Dutch respondents, the majority of the sample (66.7%) reported the same SRH before and during the pandemic, whereas 10.8% reported a decrease and 22.5% reported an increase [ 7 ]. A similar result was found in a study by Peters, in which variations in SRH before and during the pandemic were studied using a large German sample [ 14 ]. More than half of the participants (56%) stated that their SRH had not changed, 32% said it had improved, and 12% said it had decreased. This result aligns with the findings of the present study, which revealed higher odds of positive SRH after the COVID-19 pandemic.
Second, the robust implementation of effective containment measures in South Korea may have influenced individuals’ SRH. South Korea received substantial recognition for its successful efforts to control the spread of COVID-19 during the height of the pandemic. Among the 33 member countries of the Organisation for Economic Cooperation and Development, South Korea was evaluated as the top performer in COVID-19 containment [ 27 ]. The country efficiently carried out prompt testing, contact tracing, and isolation of confirmed cases, while the majority of the population adhered to mask wearing, resulting in minimal economic impact of the pandemic [ 27 ]. Against this backdrop, the social comparison mechanism may come into play and influence individuals’ SRH. Effective public health responses can alleviate anxiety and stress, leading to improved overall well-being. For example, a previous study reported a correlation of effective COVID-19 precautionary measures with reduced psychological distress and improved mental health outcomes within the general population [ 28 ]. Given the efficient implementation of disease prevention measures in South Korea, [ 29 ] the observed differences between before and after the COVID-19 pandemic may have had a more substantial effect on SRH than variations between neighborhood deprivation.
This study has certain limitations. First, it did not include objective disease indicators, meaning that the observed increase in average SRH may not reflect an actual improvement in objective health. When comparing the number of individuals with one or more chronic illnesses for each year, we observed an overall increasing trend: 32.3% in 2018, 32.7% in 2019, 32.1% in 2020, and 33.4% in 2021. These findings suggest that the prevalence of chronic conditions among individuals did not decline during the study period (Table 3 ). Future research should include objective health indicators to provide a more comprehensive assessment of health trends.
Second, we conducted a cross-sectional study because of the limitations of the data; the data used were not followed up, and interviewers were recruited every year. Nevertheless, we exerted efforts to minimize these limitations by using reliable data that could represent the population of South Korea. Additionally, we provided detailed demographic characteristics of the study participants for each year in Appendix 6 , which showed stability across the years, and thus reinforces the robustness of our analysis despite the cross-sectional design.
Third, the international comparisons of SRH have limitations. Differences in survey question construction, especially, differences in survey scales, can affect the comparability of responses [ 30 ]. The question-and-answer categories used in the survey questions vary from one country to another, thus limiting international comparisons. Internationally standardized indicators should be developed to address this limitation.
Nevertheless, the strength of the current study relative to existing research is that it analyzed SRH before and after COVID-19, in combination with the neighborhood effect. Moreover, this study is the first of its kind in the context of South Korea. While accurate international comparisons are not possible, this study can be used to compare trends in other international studies that have analyzed subjective health before and after COVID-19.
In conclusion, the SRH status showed an overall increase in the “post in disadvantaged” and “post in advantaged” groups relative to the “pre in disadvantaged” group. The possible reasons for this difference include changes in individuals’ health perceptions through social comparisons (e.g., “Eye of the Hurricane”) and the effective implementation of containment measures in South Korea.
The study conclusively demonstrates an overall improvement in SRH in both “post in disadvantaged” and “post in advantaged” groups compared to the “pre in disadvantaged” group, after the onset of the COVID-19 pandemic. This unexpected improvement suggests a significant impact of the pandemic on individuals’ perception of their health, potentially influenced by social comparison phenomena, such as the “Eye of the Hurricane” effect, and the successful implementation of COVID-19 containment measures in South Korea. These findings underscore the complex interplay between a public health crisis and social factors affecting health perceptions, highlighting the need for continued exploration of these dynamics to inform public health strategies and interventions.
Data availability
This study comprised data from the Korea Community Health Survey conducted in 2018, 2019, 2020, and 2021 by the Korea Disease Control and Prevention Agency. Data can be downloaded from the KCHS official website ( https://chs.kdca.go.kr/chs/index.do ).
Abbreviations
Attributable proportion due to interaction
Coronavirus disease 2019
Korea community health survey
Self-rated health
Relative Excess risk due to interaction
Synergy index
Waddington GS. Covid-19, mental health and physical activity. J Sci Med Sport. 2021;24(4):319.
Article PubMed PubMed Central Google Scholar
Balanzá-Martínez V, et al. Lifestyle behaviours during the COVID-19 - time to connect. Acta Psychiatr Scand. 2020;141(5):399–400.
Gammon J, Hunt J. Source isolation and patient wellbeing in healthcare settings. Br J Nurs. 2018;27(2):88–91.
Article PubMed Google Scholar
Xiong J, et al. Impact of COVID-19 pandemic on mental health in the general population: a systematic review. J Affect Disord. 2020;277:55–64.
Article CAS PubMed PubMed Central Google Scholar
Agency KDCaP. Updates on COVID-19 in South Korea. 2021; https://www.kdca.go.kr/
Organization WH. Republic of Korea: WHO Coronavirus Disease (COVID-19) Dashboard. 2021; https://covid19.who.int/region/wpro/country/kr
van de Weijer MP, et al. Self-rated health when population health is challenged by the COVID-19 pandemic; a longitudinal study. Soc Sci Med. 2022;306:115156.
Assari S, Lankarani MM. Does multi-morbidity mediate the effect of socioeconomics on self-rated health? Cross-country differences. Int J Prev Med, 2015. 6.
Gunasekara FI, Carter K, Blakely T. Change in income and change in self-rated health: systematic review of studies using repeated measures to control for confounding bias. Volume 72. Social Science & Medicine; 2011. pp. 193–201. 2.
Giltay EJ, Vollaard AM, Kromhout D. Self-rated health and physician-rated health as independent predictors of mortality in elderly men. Age Ageing. 2011;41(2):165–71.
Moon S. Gender differences in the impact of socioeconomic, health-related, and health behavioral factors on the health-related quality of life of the Korean elderly. J Digit Convergence. 2017;15(6):259–71.
Google Scholar
Tak JH. Contextual effects of Area Deprivation on Self-rated health: moderating role of Neighborship. Korean J Social Welf Res. 2016;50:111–33.
Lee DB, Ahn JH, Nam JY. Self-rated health according to change of lifestyle after COVID-19: differences between age groups. Korean J Health Educ Promot, 2022. 39(2).
Peters A, et al. The impact of the COVID-19 pandemic on self-reported health: early evidence from the German National Cohort. Volume 117. Deutsches Ärzteblatt International; 2020. p. 861. 50.
Recchi E, et al. The eye of the hurricane paradox: an unexpected and unequal rise of well-being during the Covid-19 lockdown in France. Res Social Stratification Mobil. 2020;68:100508.
Article Google Scholar
OECD. Health at a glance 2021. Organ. Econ. Co-op. Dev, 2021.
Helliwell JF, et al. Social environments for world happiness. Volume 2020. World happiness report; 2020. pp. 13–45. 1.
yeong Go. Korea Centers for Disease Control and Prevention Korea Centers for Disease Control and Prevention. 2018 Community Health Survey guidelines. Chronic Disease Control and Prevention, Community Research Team; 2018. K.y. taek, Editor.
Ok P. 2019 Community Health Survey guidelines. Editor: K.y. taek; 2019.
Ok P. 2020 Community Health Survey guidelines. Lee Yeon-kyung; 2020.
Dong-gyo Y. 2021 Community Health Survey guidelines. Editor: L. Seonkyu; 2021.
Namsoon K. COVID-19 Current status and challenges health and welfare Issue & Focus, 2020. 373: pp. 1–13.
Kim D, et al. Developing health inequalities indicators and monitoring the status of health inequalities in Korea. Seoul: Korea institute for health and social affairs; 2013. pp. 166–79.
Shin J, et al. The cross-interaction between global and age-comparative self-rated health on depressive symptoms–considering both the individual and combined effects. BMC Psychiatry. 2016;16(1):433.
Kim J-H, et al. Impact of the gap between socioeconomic stratum and subjective social class on depressive symptoms: unique insights from a longitudinal analysis. Volume 120. Social Science & Medicine; 2014. pp. 49–56.
Fayers PM, Sprangers MA. Understanding self-rated health. Lancet. 2002;359(9302):187–8.
Sachs J et al. The sustainable development goals and COVID-19. Sustainable development report, 2020.
Wang C et al. Immediate psychological responses and Associated Factors during the initial stage of the 2019 Coronavirus Disease (COVID-19) epidemic among the General Population in China. Int J Environ Res Public Health, 2020. 17(5).
Lim B, et al. COVID-19 in Korea: Success based on past failure. Asian Economic Papers. 2021;20(2):41–62.
Doctors O. Health at a glance 2021: OECD indicators. Paris, France: OECD Publishing; 2021.
Download references
Acknowledgements
This research was supported by a fund by Korea Disease Control and Prevention Agency for Chronic Disease Control Division (code: ISSN 2733-5488).
This research was supported by the Korea Health Industry Development Institute (KHIDI), Republic of Korea (HD22C20450012982076870002; Gachon University, 202210380002).
Author information
Authors and affiliations.
Department of Public Health, Graduate School, Yonsei University, Seoul, Republic of Korea
Hajae Jeon & Bomgyeol Kim
Health-IT Center, Yonsei University Health System Seoul, Seoul, Republic of Korea
Department of Preventive Medicine, Institute of Health Services Research, Yonsei University College of Medicine, 50 Yonsei-ro, Seodaemun-gu, Seoul, 03722, Republic of Korea
Mingee Choi, Sang Gyu Lee & Jaeyong Shin
You can also search for this author in PubMed Google Scholar
Contributions
Conceptualization: HJ and JS. Data curation: JL and MC. Formal analysis: HJ and BK. Methodology: HJ, JS, and SL. Software: JL. Validation: BK and JL. Investigation: MC. Writing–original draft: HJ and JL. Writing–review & editing: JS and SL.
Corresponding author
Correspondence to Jaeyong Shin .
Ethics declarations
Ethics approval and consent to participate.
This study did not require prior consent or approval from an institutional review board because the KCHS is a secondary dataset consisting of already de-identified data that are available in the public domain.
Consent for publication
Not applicable.
Human ethics and consent to participate declarations
Competing interests.
The authors declare no competing interests.
Additional information
Publisher’s note.
Springer Nature remains neutral with regard to jurisdictional claims in published maps and institutional affiliations.
Electronic supplementary material
Below is the link to the electronic supplementary material.
Supplementary Material 1
Rights and permissions.
Open Access This article is licensed under a Creative Commons Attribution 4.0 International License, which permits use, sharing, adaptation, distribution and reproduction in any medium or format, as long as you give appropriate credit to the original author(s) and the source, provide a link to the Creative Commons licence, and indicate if changes were made. The images or other third party material in this article are included in the article’s Creative Commons licence, unless indicated otherwise in a credit line to the material. If material is not included in the article’s Creative Commons licence and your intended use is not permitted by statutory regulation or exceeds the permitted use, you will need to obtain permission directly from the copyright holder. To view a copy of this licence, visit http://creativecommons.org/licenses/by/4.0/ . The Creative Commons Public Domain Dedication waiver ( http://creativecommons.org/publicdomain/zero/1.0/ ) applies to the data made available in this article, unless otherwise stated in a credit line to the data.
Reprints and permissions
About this article
Cite this article.
Jeon, H., Lee, J., Choi, M. et al. Interaction effects of the COVID-19 pandemic and regional deprivation on self-rated health: a cross-sectional study. BMC Public Health 24 , 2382 (2024). https://doi.org/10.1186/s12889-024-19814-x
Download citation
Received : 19 February 2024
Accepted : 16 August 2024
Published : 02 September 2024
DOI : https://doi.org/10.1186/s12889-024-19814-x
Share this article
Anyone you share the following link with will be able to read this content:
Sorry, a shareable link is not currently available for this article.
Provided by the Springer Nature SharedIt content-sharing initiative
- Socioeconomic factors
- Health status indicators
- Diagnostic self-evaluation
BMC Public Health
ISSN: 1471-2458
- General enquiries: [email protected]

An official website of the United States government
The .gov means it’s official. Federal government websites often end in .gov or .mil. Before sharing sensitive information, make sure you’re on a federal government site.
The site is secure. The https:// ensures that you are connecting to the official website and that any information you provide is encrypted and transmitted securely.
- Publications
- Account settings
- My Bibliography
- Collections
- Citation manager
Save citation to file
Email citation, add to collections.
- Create a new collection
- Add to an existing collection
Add to My Bibliography
Your saved search, create a file for external citation management software, your rss feed.
- Search in PubMed
- Search in NLM Catalog
- Add to Search
From AIDS to COVID-19: the interplay between dual pandemics in social perceptions of disease
Affiliations.
- 1 Department of Women and Gender Studies, International Affairs Program, University of Colorado, Boulder, CO, USA.
- 2 Department of Sociology, University of Colorado, Boulder, CO, USA.
- PMID: 39243172
- DOI: 10.1080/13691058.2024.2401006
This paper is one of the few to examine how people who have lived through both COVID-19 and AIDS understand these pandemics in relation to each other. Data were collected in Uganda, and we found that the AIDS epidemic proved to be a key reference point for people in explaining why COVID-19 was perceived as so worrisome. In addition, AIDS-related stigma was a problematically common frame when discussing responsibility for HIV versus SARS-CoV-2 infection, and there was evidence of some forgetfulness regarding the toll AIDS had taken on the country. More positively, the legacy of AIDS made many people more attentive to social inequalities tied to health risks, and this at times prompted a more nuanced understanding of the socially varied effects of COVID-19. Overall, we argue that how individuals respond to a novel epidemic is shaped not only by their understandings of current threats but also by enduring perceptions of epidemics and pandemics that may have preceded it.
Keywords: AIDS; COVID-19; HIV; social perceptions.
PubMed Disclaimer
- Citation Manager
NCBI Literature Resources
MeSH PMC Bookshelf Disclaimer
The PubMed wordmark and PubMed logo are registered trademarks of the U.S. Department of Health and Human Services (HHS). Unauthorized use of these marks is strictly prohibited.
- About Our Founder
- Mission, Vision and Values
- 2028 Vision
- Board of Trustees
- Host-Pathogen Interactions (HPI)
- Disease Intervention & Prevention (DIP)
- Population Health (PH)
- International Center for the Advancement of Research and Education
- Southwest National Primate Research Center (SNPRC)
- High Containment
- Data Analysis Unit
- Cellular Biology Unit
- Microscopy Unit
- Molecular Unit
- Vivarium Unit
- Our Partners
- Our Technology
- Our Expertise
- Begin Discussion
- Disease Models
- Virus Request Form
- Reverse Genetics Plasmids Request Form
- Community Outreach Programs
- Education Program Leadership
- Curricular Units
- Internship Program Structure
- Teacher Professional Development
- High School Tours
- Valero Young Scientist Program
- American Cancer Society Post-Baccalaureate Fellows Program at Texas Biomed
- Media Contacts
- Media Inquiries
- Fact Sheets
- TxBiomed Magazine Summer 2022
- Media Library
- Experts Available for Comment
- Funding Priorities
- How To Give
- Connect With Us
- Giving Partners
- Make a Contribution
- Request Information
- Planned Giving
- Capital & Endowment Giving
- Honor & Memorial Giving
- The Argyle Membership Donation
- Texas Biomedical Forum
- Founder’s Council Board Members
- Founder’s Council Events
- Founder’s Council Membership
- Initiate / Renew Membership
- (X) Twitter
Monoclonal antibody shows protection against all COVID variants
Antibody developed in part by Associate Professor Greg Ippolito, Ph.D., works against a wide range of COVID-19 variants and related coronaviruses, including past, present and potentially future strains.
SAN ANTONIO (Sept. 5, 2024) — A monoclonal antibody appears effective at neutralizing the numerous variants of SARS-CoV-2, as well as related viruses in animals that could pose a threat if they were to begin spreading in people. The antibody, called SC27, was recently described in Cell Reports Medicine .
The finding opens the possibility of broader, more effective treatments to work against current and future COVID variants.
Monoclonal antibody SC27 was identified, developed and provisionally patented by a team of researchers led by Greg Ippolito , Ph.D., who recently joined Texas Biomedical Research Institute (Texas Biomed), from University of Texas at Austin. Other team leaders included Jason Lavinder, Ph.D., at UT and Ralph Baric, Ph.D., at University of North Carolina at Chapel Hill.
“Other COVID-19 antibodies have been rendered ineffective as SARS-CoV-2 has evolved over the past several years,” says Dr. Ippolito, an Associate Professor. “Our new study suggests the virus is less likely to escape this treatment because SC27 targets and attaches to multiple parts of the virus’s spike protein, including sections that are not mutating as frequently.”
SC27 appears to work in two ways: it blocks the ACE2 binding site, which the virus uses to bind to, enter and infect cells. It also binds to a hidden or “cryptic” site on the underside of the spike protein that is largely unchanged or “conserved” between variants, which means SC27 can broadly recognize variants and related viruses. This is critical because if an antibody’s shape does not match enough with a virus – like two puzzle pieces that don’t quite fit – the antibody can’t effectively neutralize the virus and the virus sneaks by the body’s immune defense system.
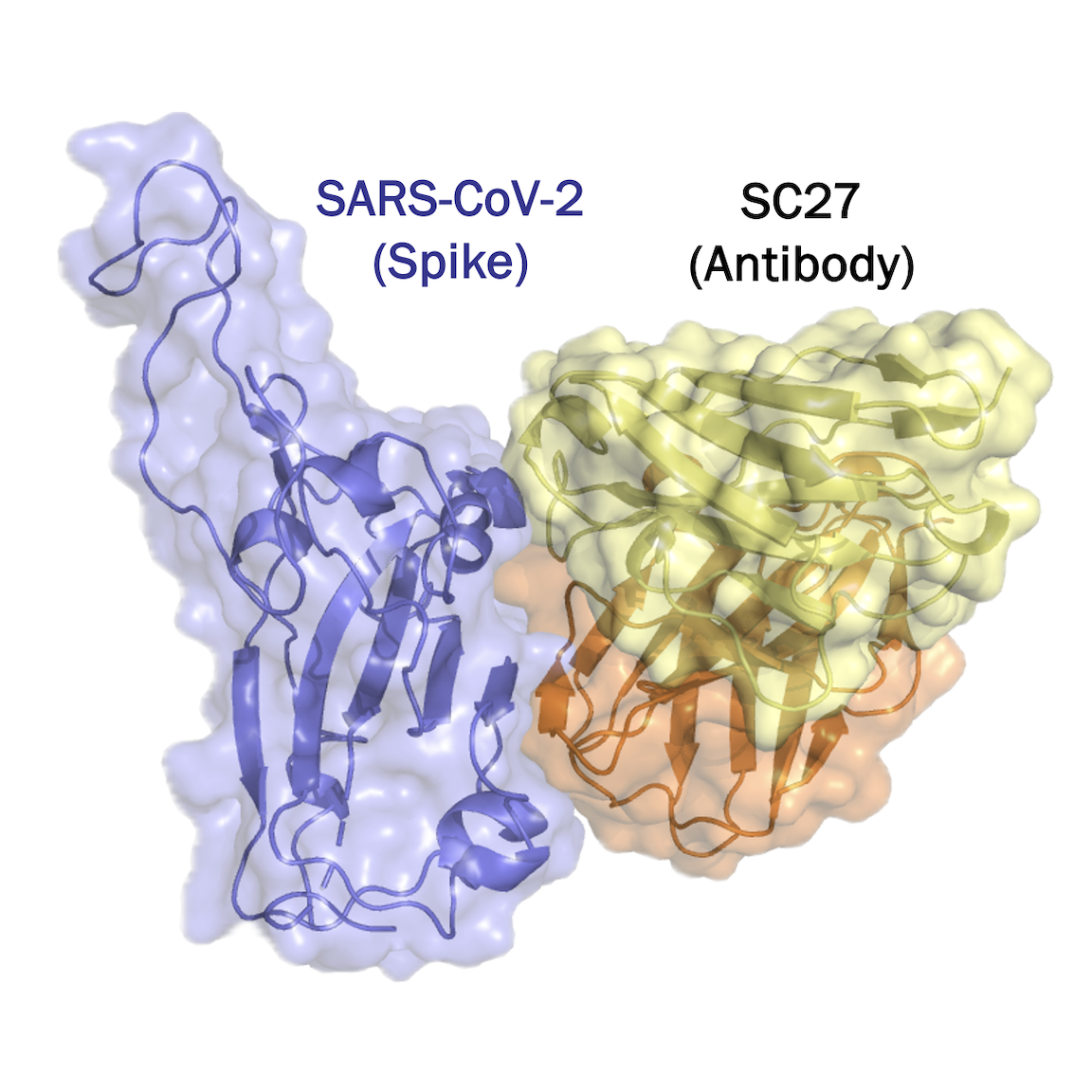
The researchers tested SC27 against 12 viruses, from the original SARS-CoV-2 to currently circulating variants, as well as related SARS-1 and several other coronaviruses found in bats and pangolins. The antibody was effective against all of them in a petri dish and protected mice against both variants tested.
“This makes it broader and more effective than any other monoclonal antibody reported in scientific literature to date and the former FDA-approved cocktails,” says Dr. Ippolito, adding the caveat that SC27 still needs to be tested in human clinical trials.
The team is looking to collaborate with industry to further develop the SC27 monoclonal antibody treatment, which could potentially benefit immunocompromised patients who are unable to get vaccines. It also could serve as an emergency treatment during future outbreaks of new variants or coronaviruses. Next steps would include preclinical studies in larger animal models, including nonhuman primates, which are the gold standard to evaluate how complete immune systems respond to a treatment before safely moving to human clinical trials.
Notably, SC27 was found in individuals following vaccination with mRNA COVID-19 vaccines. Previously, this type of “class 1/4” antibody – which attaches to two distinct areas or “epitopes” of the spike protein – was only detected following natural infection from SARS-1.
“This is fantastic news that vaccines can prompt the generation of these more robust and effective antibodies,” explains Dr. Ippolito. “It means that future vaccine development can be tailored to generate these antibodies and have a clear metric for measuring which vaccines will be most effective.”
About Texas Biomed
Texas Biomed is a nonprofit research institute dedicated to protecting the global community from infectious diseases. Through basic research, preclinical testing and applied innovation, we accelerate diagnostics, therapies and vaccines for the world’s deadliest pathogens. Our San Antonio campus hosts high containment laboratories and the Southwest National Primate Research Center. Our scientists collaborate with industry and researchers globally, and have helped deliver the first COVID-19 vaccine, the first Ebola treatment and first Hepatitis C therapy. For more information, visit txbiomed.org .
Enhancing classification of lung diseases by optimizing training hyperparameters of the deep learning network
- Published: 09 September 2024
Cite this article
- Hardeep Saini ORCID: orcid.org/0000-0002-9309-8240 1 &
- Davinder Singh Saini ORCID: orcid.org/0000-0003-3177-1193 1
The COVID-19 pandemic was triggered by the SARS-CoV-2 virus which caused multiple ill-health conditions in infected individuals. There were many cases that culminated in death. Chest X-ray images became a proven method for spotting thoracic ailments. The resultant availability of huge public datasets of chest X-ray images has great potential in deep learning for lung ailment detection. This paper presents a classification that aims at acquiring the optimal hyperparameters using the metaheuristic algorithm for various pre-trained CNN training processes. The experimental results show that HSAGWO (Hybrid Simulated Annealing Grey Wolf Optimization) outperforms the other contemporary models for optimizing training hyperparameters in the ResNet50 network. The accuracy, precision, sensitivity (recall), specificity, and F1-score values obtained are 98.78%, 98.10%, 99.31%, and 98.64%, respectively, which are significantly better than the values obtained for the existing methods. The objective of this work is to improve classification accuracy and reduce false negatives while keeping computational time to a minimum.
This is a preview of subscription content, log in via an institution to check access.
Access this article
Subscribe and save.
- Get 10 units per month
- Download Article/Chapter or eBook
- 1 Unit = 1 Article or 1 Chapter
- Cancel anytime
Price includes VAT (Russian Federation)
Instant access to the full article PDF.
Rent this article via DeepDyve
Institutional subscriptions
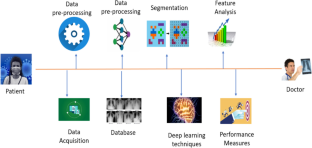
Explore related subjects
- Artificial Intelligence
- Medical Imaging
Data availability
Data is available on request.
Das R, Arshad M, Manjhi PK, Thepade SD (2020) Covid-19 identification with chest X-ray images merging handcrafted and automated features for enhanced feature generalization. In: 2020 5th International Conference on Computing, Communication and Security (ICCCS). Patna, India, pp 1–6. https://doi.org/10.1109/ICCCS49678.2020.9277482
Tahamtan A, Ardebili A (2020) Real-time RT-PCR in COVID-19 detection: issues affecting the results. Expert Rev Mol Diagn 20(5):453–454. https://doi.org/10.1080/14737159.2020.1757437
Article Google Scholar
Alghamdi HS, Amoudi G, Elhag S, Saeedi K, Nasser J (2021) Deep Learning Approaches for Detecting COVID-19 from Chest X-Ray Images: A Survey. IEEE Access 9:20235–20254. https://doi.org/10.1109/ACCESS.2021.3054484
Shetty S, S., A.V. & Mahale. (2023) A. Multimodal medical tensor fusion network-based DL framework for abnormality prediction from the radiology CXRs and clinical text reports. Multimed Tools Appl 82:44431–44478. https://doi.org/10.1007/s11042-023-14940-x
Deng J, Dong W, Socher R, Li LJ, Kai Li, Li Fei-Fei (2009). ImageNet: A large-scale hierarchical image database. IEEE Conference on Computer Vision and Pattern Recognition, pp. 248–255. https://doi.org/10.1109/CVPR.2009.5206848 .
Lee J et al (2017) Deep Learning in Medical Imaging : General Overview. Korean J Radiol 18(4):570–584
Darwish A, Hassanien AE, Das S (2020) A survey of swarm and evolutionary computing approaches for deep learning. Artif Intell Rev 53(3):1767–1812. https://doi.org/10.1007/s10462-019-09719-2
Woźniak M, Połap D (2018) Bio-inspired methods modeled for respiratory disease detection from medical images. Swarm Evol Comput 41:69–96. https://doi.org/10.1016/j.swevo.2018.01.008
Mirjalili S, Saremi S, Mirjalili SM, Coelho LDS (2016) Multi-objective grey wolf optimizer: A novel algorithm for multi-criterion optimization. Expert Syst Appl 47:106–119. https://doi.org/10.1016/j.eswa.2015.10.039
Van Laarhoven PJM, Aarts EHL (1987) Simulated annealing. In: Simulated Annealing: Theory and Applications. Mathematics and Its Applications, Springer, Dordrecht, vol. 37, pp. 7–15, doi. : https://doi.org/10.1007/978-94-015-7744-1_2 .
Serte S, Serener A, Al-turjman F (2022) Deep learning in medical imaging: a brief review. Trans Emerg Telecommun Technol 33:e4080. https://doi.org/10.1002/ett.4080
Hemdan EED, Shouman MA, Karar ME (2020) Covidx-net: a framework of deep learning classifiers to diagnose covid-19 in x-ray images. arXiv preprint arXiv:2003.11055 .
Punn NS, Agarwal S (2020) Automated diagnosis of COVID-19 with limited posteroanterior chest X-ray images using fine-tuned deep neural networks. Appl Intell 51(5):2689–2702
Ozturk T, Talo M, Azra E, Baran U, Yildirim O, Acharya UR (2020) Automated detection of COVID-19 cases using deep neural networks with X-ray images. Comput Biol Med 121:103792. https://doi.org/10.1016/j.compbiomed.2020.103792
Jain G, Mittal D, Thakur D, Mittal MK (2020) A deep learning approach to detect Covid-19 coronavirus with X-Ray images. Biocybern Biomed Eng 40(4):1391–1405. https://doi.org/10.1016/j.bbe.2020.08.008
Apostolopoulos ID, Bessiana T (2020) Covid-19: Automatic detection from X-Ray images utilizing Transfer Learning with Convolutional Neural Networks. Phys Eng Sci Med 43(2):635–640
Bandyopadhyay R, Basu A, Cuevas E, Sarkar R (2021) Harris Hawks optimization with Simulated Annealing as a deep feature selection method for screening of COVID-19 CT-scans. Appl Soft Comput 111:107698. https://doi.org/10.1016/j.asoc.2021.107698
Abbas A, Abdelsamea MM, Gaber MM (2021) Classification of COVID-19 in chest X-ray images using DeTraC deep convolutional neural network. Appl Intell 51(2):854–864
Rahimzadeh M, Attar A (2020) A modified deep convolutional neural network for detecting COVID-19 and pneumonia from chest X-ray images based on the concatenation of Xception and ResNet50V2. Informatics Med Unlocked 19:100360. https://doi.org/10.1016/j.imu.2020.100360
Narin A, Kaya C, Pamuk Z (2021) Automatic detection of coronavirus disease (COVID-19) using X-ray images and deep convolutional neural networks. Pattern Anal Appl 24(3):1207–1220. https://doi.org/10.1007/s10044-021-00984-y
Kumar N, Gupta M, Gupta D et al (2023) Novel deep transfer learning model for COVID-19 patient detection using X-ray chest images. J Ambient Intell Human Comput 14:469–478. https://doi.org/10.1007/s12652-021-03306-6
Loey M, Manogaran G, Khalifa NEM (2020) A deep transfer learning model with classical data augmentation and CGAN to detect COVID-19 from chest CT radiography digital images. Neural Comput Appl, pp. 1–13, https://doi.org/10.1007/s00521-020-05437-x .
Al Mehedi Hasan M, Shin J, Parvin F, (2021) Deep Transfer Learning Based Detection of COVID-19 from Chest X-ray Images. ACM Int Conf Proceeding Biomed Eng Tech, pp. 64–70, https://doi.org/10.1145/3460238.3460249 .
Domhan T, Springenberg JT, Hutter F (2015) Speeding up automatic hyperparameter optimization of deep neural networks by extrapolation of learning curves. In: Twenty-fourth international joint conference on artificial intelligence. https://api.semanticscholar.org/CorpusID:369457
Riaz M, Bashir M, Younas I (2022) Metaheuristics based COVID-19 detection using medical images: a review. Comput Biol Med 144:105344. https://doi.org/10.1016/j.compbiomed.2022.105344
Aszemi NM, Dominic PDD (2019) Hyperparameter optimization in convolutional neural network using genetic algorithms. Int J Adv Comput Sci Appl 10(6):269–278. https://doi.org/10.14569/ijacsa.2019.0100638
Goel T, Murugan R, Mirjalili S, Chakrabartty DK (2021) OptCoNet: an optimized convolutional neural network for an automatic diagnosis of COVID-19. Appl Intell 51(3):1351–1366. https://doi.org/10.1007/s10489-020-01904-z
Rajpal S, Lakhyani N, Singh AK, Kohli R, Kumar N (2021) Using handpicked features in conjunction with ResNet-50 for improved detection of COVID-19 from chest X-ray images. Chaos, Solitons Fractals 145:110749. https://doi.org/10.1016/j.chaos.2021.110749
Pathan S, Siddalingaswamy PC, Ali T (2021) Automated Detection of Covid-19 from Chest X-ray scans using an optimized CNN architecture. Appl Soft Comput J 104(1):107238–107250. https://doi.org/10.1016/j.asoc.2021.107238
Ezzat D, ell Hassanien A, Ella HA (2020) GSA-DenseNet121-COVID-19: a Hybrid Deep Learning Architecture for the Diagnosis of COVID-19 Disease based on Gravitational Search Optimization Algorithm. arXiv, pp. 1–29. https://doi.org/10.1016/j.asoc.2020.106742 .
Shankar K et al (2021) An optimal cascaded recurrent neural network for intelligent COVID-19 detection using Chest X-ray images. Appl Soft Comput 113:107878. https://doi.org/10.1016/j.asoc.2021.107878
Ezzat D, Hassanien AE, Ella HA (2021) An optimized deep learning architecture for the diagnosis of COVID-19 disease based on gravitational search optimization. Appl Soft Comput 98:106742. https://doi.org/10.1016/j.asoc.2020.106742
Dhiman G, Chang V, Kant Singh K, Shankar A (2022) ADOPT: automatic deep learning and optimization-based approach for detection of novel coronavirus COVID-19 disease using X-ray images. J Biomol Struct Dyn 40(13):5836–5847. https://doi.org/10.1080/07391102.2021.1875049
Alshmrani GM, M., et al (2023) A deep learning architecture for multi-class lung diseases classification using chest X-ray (CXR) images. Alex Eng J 64:923–935
Ravi V et al (2023) A multichannel EfficientNet deep learning-based stacking ensemble approach for lung disease detection using chest X-ray images. Clust Comput 26(2):1181–1203. https://doi.org/10.1007/s10586-022-03664-6
Constantinou Marios, Exarchos Themis, Vrahatis Aristidis G, Vlamos Panagiotis (2023) "COVID-19 Classification on Chest X-ray Images Using Deep Learning Methods. Int J Environ Res Public Health 20(3):2035. https://doi.org/10.3390/ijerph20032035
Farhan, Abobaker Mohammed Qasem, and Shangming Yang.( (2023), "Automatic lung disease classification from the chest X-ray images using hybrid deep learning algorithm." Multimedia Tools and Applications, 1–27. https://doi.org/10.1007/s11042-023-15047-z .
Prasanta Baruah, Pankaj Pratap Singh and Sanjiv kumar Ojah, (2023 ) A Novel Framework for Risk Prediction in the Health Insurance Sector using GIS and Machine Learning. Int J Adv Comp Sci Appl (IJACSA), 14(12), https://doi.org/10.14569/IJACSA.2023.0141249 .
Mirjalili S, Mohammad S, Lewis A (2014) Advances in Engineering Software Grey Wolf Optimizer. Adv Eng Softw 69:46–61. https://doi.org/10.1016/j.advengsoft.2013.12.007
Kirkpatrick S, Gelatt CD, Vecchi MP (1983) Optimization by simulated annealing. Science 220(4598):671–680. https://doi.org/10.1126/science.220.4598.671
Article MathSciNet Google Scholar
Kennedy J (2006) Swarm Intelligence. In: Zomaya AY (eds) Handbook of Nature-Inspired and Innovative Computing. Springer, Boston, MA. https://doi.org/10.1007/0-387-27705-6_6 .
Abdellatef E, Allah MF (2024) Hybrid whale optimization and canonical correlation based COVID-19 classification approach. Multim Tools Appl, 1–22. https://doi.org/10.1007/s11042-024-18153-8 .
Dhar J (2021) Multistage ensemble learning model with weighted voting and genetic algorithm optimization strategy for detecting chronic obstructive pulmonary disease. IEEE Access 9:48640–48657. https://doi.org/10.1109/ACCESS.2021.3067949
COVID-19 Database—SIRM. Available online: https://sirm.org/category/covid-19/ (accessed on 25 April 2023).
COVID-19-Image-Repository. Available online: https://github.com/ml-workgroup/covid-19-image-repository (accessed on 25 April 2023).
Mooney P (2018) Chest X-ray images (pneumonia) Available online: https://www.kaggle.com/datasets/paultimothymooney/chest-xray-pneumonia (accessed on 25 April 2023).
Sethy PK, Behera SK, Ratha PK, Biswas P (2020) Detection of coronavirus disease (COVID-19) based on deep features and support vector machine. Int J Math Eng Manag Sci 5(4):643–651. https://doi.org/10.33889/IJMEMS.2020.5.4.052
Goel T, Murugan R, Mirjalili S, Chakrabartty DK (2022) Multi-COVID-Net: Multi-objective optimized network for COVID-19 diagnosis from chest X-ray images. Appl Soft Comput 115:108250. https://doi.org/10.1016/j.asoc.2021.108250
Download references
No funding was requested nor received for conducting this study.
Author information
Authors and affiliations.
Department of Electronics and Communication Engineering, Chandigarh College of Engineering and Technology, Chandigarh, India
Hardeep Saini & Davinder Singh Saini
You can also search for this author in PubMed Google Scholar
Corresponding author
Correspondence to Hardeep Saini .
Ethics declarations
Conflict of interest.
No conflict of interest has been disclosed by the authors.
Ethical approval
This research did not involve experiments or studies that required ethical approval involving human participants or animals.
Additional information
Publisher's note.
Springer Nature remains neutral with regard to jurisdictional claims in published maps and institutional affiliations.
Rights and permissions
Springer Nature or its licensor (e.g. a society or other partner) holds exclusive rights to this article under a publishing agreement with the author(s) or other rightsholder(s); author self-archiving of the accepted manuscript version of this article is solely governed by the terms of such publishing agreement and applicable law.
Reprints and permissions
About this article
Saini, H., Saini, D.S. Enhancing classification of lung diseases by optimizing training hyperparameters of the deep learning network. Multimed Tools Appl (2024). https://doi.org/10.1007/s11042-024-20085-2
Download citation
Received : 05 November 2023
Revised : 10 August 2024
Accepted : 13 August 2024
Published : 09 September 2024
DOI : https://doi.org/10.1007/s11042-024-20085-2
Share this article
Anyone you share the following link with will be able to read this content:
Sorry, a shareable link is not currently available for this article.
Provided by the Springer Nature SharedIt content-sharing initiative
- Deep Learning
- Simulating Annealing
- Grey Wolf Optimization
- Chest X-rays
- Lung Diseases
- Hyperparameters
Advertisement
- Find a journal
- Publish with us
- Track your research
- Health Tech
- Health Insurance
- Medical Devices
- Gene Therapy
- Neuroscience
- H5N1 Bird Flu
- Health Disparities
- Infectious Disease
- Mental Health
- Cardiovascular Disease
- Chronic Disease
- Alzheimer's
- Coercive Care
- The Obesity Revolution
- The War on Recovery
- Adam Feuerstein
- Matthew Herper
- Jennifer Adaeze Okwerekwu
- Ed Silverman
- CRISPR Tracker
- Breakthrough Device Tracker
- Generative AI Tracker
- Obesity Drug Tracker
- 2024 STAT Summit
- All Summits
- STATUS List
- STAT Madness
- STAT Brand Studio
Don't miss out
Subscribe to STAT+ today, for the best life sciences journalism in the industry
Medicine struggles to define chronic Lyme. Long Covid has only made it harder

By Isabella Cueto and Alia Sajani
Sept. 9, 2024

Going to the doctor is already tricky enough for people with chronic symptoms of Lyme disease. Their concerns often dismissed by mainstream medicine, those patients now face an additional hurdle: ruling out long Covid.
The two illnesses — one seeping in over the course of decades and another suddenly springing to life on a massive scale — share many qualities, including being widely misunderstood. But as efforts to demystify long Covid intensify, so does interest in studying neglected conditions, including persistent complications from Lyme disease.
advertisement
Long Covid was a crash course in chronic conditions that start with infection. “As a result, Lyme patients are now met with more compassion and understanding than ever in the past. It is now harder for a physician to dismiss the possibility of a Lyme diagnosis out of hand than it was before the pandemic,” said Bernadette Clavier, facilitator of the Coalition for Infection-Associated Chronic Conditions and Illnesses Research.
New studies aim to tease apart the diseases’ differences and similarities in hopes of finding treatments for patients that have gone without vetted, tailored therapeutics, in some cases for decades.
Related: Lyme disease cases rise, but largely due to a change in data collection
All told, over 10 million people in the United States have long-term symptoms of Covid or Lyme disease, according to recent estimates. Up to a quarter of people treated with antibiotics for Lyme disease go on to develop chronic manifestations, a condition called post-treatment Lyme disease syndrome by the Centers for Disease Control and Prevention. Many others go untreated, leaving the bacteria carried by blacklegged ticks with unfettered access. (While some believe a continuing infection is at play in post-treatment Lyme, others think there’s a different underlying reason for continuing symptoms.)
The condition, like long Covid, can cause a litany of confusing symptoms, like body pain, profound fatigue, and brain fog. And neither group of patients has a clear-cut way of knowing if an infection was the source of their problems.
That’s a real issue for clinicians, says John Aucott, director of the Johns Hopkins Lyme Disease Clinical Research Center. Even to an expert like him, long Covid, post-treatment Lyme and other post-infectious conditions like myalgic encephalomyelitis “all look remarkably similar,” he said. There’s a lot of symptom overlap, and simple blood tests are insufficient to know what the root cause is. In many cases, patients may not have tested for the Covid virus or the bacteria Borrelia burgdorferi that causes Lyme. They may not even recall being sick, or getting bitten by a tick.
“It requires meticulous attention to getting that history and documenting it,” said Aucott, who says multiple of his patients developed long Covid after being diagnosed with post-treatment Lyme. “There’s no shortcuts to it right now.”
Related: Long Covid is more prevalent among Americans with disabilities, new CDC data show
That makes getting answers even more difficult. Doctors famously like quick things — tests, scans, biomarkers. Patients in post-infection limbo have no such outs. Sure, there are PCR and antibody tests, including what’s considered the “golden ticket” in Lyme: the western blot, which can detect specific proteins in the blood. People with suspected chronic Lyme often clamor for the blot, thinking it will grant them a sense of medical legitimacy and treatment. But the blot, like Covid antibody tests, taps into the immune system’s memory; it can confirm that a person was exposed at some point, but can’t tell whether someone has long Covid or chronic Lyme, Aucott said.
On the flip side, a negative antibody test could mean a long time has passed since the exposure, or that the test produced a false negative .
Lyme disease is the most common tick-borne disease in the U.S., but ticks can also carry parasites or viruses. Some of those may get caught with standard tests and treated with antibiotics, but not all of them. People may be infected by multiple pathogens at once, or acquire multiple over time. And both Lyme and Covid may make patients more susceptible to other infections, as well as autoimmune diseases.
Researchers are looking for specific biomarkers — and even using machine learning to do so — to distinguish an immune response to, say, a virus versus bacteria. Others are trying to figure out if the body is reacting to lingering virus or bacteria, or just having an overextended immune response to a long-gone infection.
In the meantime, patients and providers are left to sift through a vague set of facts and symptoms and figure out how best to manage a potentially life-altering condition.

‘You feel like you’re crazy’
Almas Eftekhari, a 40-year-old Lyme disease advocate, shrugged off her first, super-mild bout of Covid in August of 2023. The worst of it was losing her sense of taste and smell. But two months later, Eftekhari crashed.
Symptoms she’d attributed to the long tail of her Lyme — diagnosed with a positive western blot after many tick bites in woods near her Northern Virginia home — worsened. Joint pain would migrate unpredictably around her body, and up her neck. Gastrointestinal issues popped up out of nowhere. “Some things are there for three months and they totally disappear. And then you get some new stuff,” she said. ”You feel like you’re crazy.”
Her brain fog got so bad she could hardly form a sentence, much less read a book. Eftekhari said her memory slowed, too. She recalls filling her dog’s bowl with water and forgetting for hours to close the faucet, and needing to buy lanyards to attach her cell phone to her body, because she’d lose it so frequently. Was it Lyme or was it long Covid?
Related: ‘I’m Not Crazy, I’m Sick’: A film dives deep into chronic Lyme disease
Immune dysregulation can make it so the body keeps fighting a pathogen after it’s been cleared (or at least becomes undetectable on common tests). This chronic inflammation can lead to long-term disruptions in the brain, heart and gut, experts say. Researchers at the Lyme Disease Research Center at Johns Hopkins Medicine observed altered brain activity in the white matter of people with Lyme.
Crosstalk between the immune and nervous systems, mediated by specialized cells, has been found to be altered in monkeys infected with the bacteria that causes Lyme. Neuroinflammation also occurs during Covid, and another monkey study showed aged or diabetic animals fared worse. Other studies have suggested Covid’s “priming” of the nervous system could make people more susceptible to later infections, too.
Eftekhari and her partner, who also has disabling chronic symptoms from Lyme, would argue about it often, she said. “I was like, ‘This is Lyme.’ And he’s like, no, it’s Covid. And I’m like, no, it’s not.”
She was soon thereafter diagnosed with postural orthostatic tachycardia syndrome, or POTS. It’s a condition that can cause, among other symptoms, lightheadedness when going from sitting to standing. The POTS explained the extreme dizziness that flattened her on many days. But also, it’s yet another look-alike syndrome: like long Covid and Lyme, POTS can cause fatigue, cognitive issues, and digestive system problems. Eftekhari wonders if her dizziness isn’t just from babesiosis, a disease caused by ticks infected with a parasite.
With few treatment options available, Eftekhari has to help herself. She’s trying experimental protocols, mostly consisting of supplements and antibiotics. “I’m hoping that it cures me or at least gets me to a point of remission, but I’m not really sure,” she said.

Antigens and therapy candidates
An elevated immune response can be associated with autoimmune, metabolic, neurological, and endocrine dysfunction, said Beth Pollack, a long Covid and ME/CFS research scientist at the MIT department of biological engineering. Tracking symptoms will help scientists better understand the biology of Lyme and Covid, she said.
Scientists have identified the parts of the pathogens that most strongly provoke immune activation and lead to chronic inflammation. The antigen that triggers the inflammatory responses in chronic Lyme symptoms is found on the outer layer of the bacterial cell wall. The mesh-like scaffolding, called peptidoglycan, is a popular target for antibiotics. This peptidoglycan coats the bacteria and also readily absorbs these drugs. However, pieces of these bacterial cell walls can linger in the body even post infection. This may contribute to the prolonged inflammation that leads to chronic illness. These hidden reservoirs of persistence, in the form of tiny pieces of bacteria that may linger in the neurons or spinal cord, can go undetected because of an inability to test for it.
Related: Long Covid feels like a gun to my head
A similar trend of leftover pathogen pieces in the body may also happen with Covid. While studies have not shown the presence of leftover viral pieces, scientists have concern that these fragments can cause prolonged inflammation.
Detecting the presence of leftover bacterial and viral fragments that can have long-term immune and neurological effects is hard — but identifying a biomarker that reflects chronic inflammation could help. Aucott says immune markers like serum interferon-alpha and the chemokine CCL19 can be useful in research settings, even though they haven’t been validated or accepted as biomarkers by the FDA. Patients are often not tested for these.
Without clear biomarkers, the disorienting overlap between conditions impedes scientific research, some experts say. It will continue to be difficult to tell whether a potential participant in a trial is sick because of long Covid or due to Lyme, or some other condition. Uniformity in study groups will be challenging without knowing all of the triggers participants were exposed to.
“We have to come up with a plan to care for these patients, a plan to do the research that’s needed. It ain’t going to go away,” Aucott told STAT.
There are currently no FDA-approved therapies for chronic Lyme, or full-scale clinical treatment trials — a strikingly similar position to many other chronic conditions, including long Covid. NIH funding for Lyme research has historically been low, but advocates are hoping the spotlight on long Covid will lead to more attention for other chronic inflammatory illnesses . The need is evident in social media support groups, in the sometimes desperate measures patients will take to get some relief. It’s obvious when looking at monthslong waitlists for appointments at clinics specializing in chronic Lyme and long Covid: people need help.
However, there is some movement. Researchers at Johns Hopkins and other institutions have a list of potential new therapies they’d like to study — everything from inflammatory arthritis treatments to antibiotic combination therapies, brain stimulation and magic mushrooms.
Before Covid, the NIH released a research plan that called for greater efforts to understand the cause of long-term symptoms in Lyme disease. Last year, the National Institute of Allergy and Infectious Diseases funded seven research projects into persistent Lyme symptoms, which will run until 2028. NIAID is also trying to design a study to follow Lyme patients starting at the time of diagnosis, to better grasp just how often the disease turned into chronic or recurring symptoms, an NIH program officer said in a recent meeting.
Sign up for Morning Rounds
Understand how science, health policy, and medicine shape the world every day
In July, the NIH announced a funding opportunity for research on neurological and psychiatric manifestations of infection-associated chronic illnesses, including post-treatment Lyme and long Covid. It’s one step in the direction of what advocates and some researchers have been asking for: research infrastructure without silos, so murky areas between diseases and body systems can be explored.
It’s possible, Pollack says, that cross-illness trials are a key — one way to decipher and help patients with multiple chronic inflammatory illnesses. These kinds of studies cast a wide net for participants with shared symptoms, and then analyze in-depth the overlaps in their conditions (for example, a long Covid trial with an arm for participants with POTS, and another for ME/CFS patients).
“We need to study the most complex patients, not just the ones who fit into boxes,” she said.
STAT’s coverage of chronic health issues is supported by a grant from Bloomberg Philanthropies . Our financial supporters are not involved in any decisions about our journalism.
About the Authors
Isabella cueto.
Chronic Disease Reporter
Isabella Cueto covers the leading causes of death and disability: chronic diseases. Her focus includes autoimmune conditions and diseases of the lungs, kidneys, liver (and more). She writes about intriguing research, the promises and pitfalls of treatment, and what can be done about the burden of disease.
Alia Sajani
AAAS Mass Media Fellow
chronic disease
Coronavirus
infectious disease
STAT encourages you to share your voice. We welcome your commentary, criticism, and expertise on our subscriber-only platform, STAT+ Connect
To submit a correction request, please visit our Contact Us page .
Recommended Stories

STAT Plus: With a win in lung cancer, biotech’s wealthiest outsider surfs to new heights

How Paralympic athletes are helping science learn more about neuropathic pain

STAT Plus: UnitedHealth pledged a hands-off approach after buying a Connecticut medical group. Then it upended how doctors practice

STAT Plus: Summit Therapeutics vs. Merck: Prepping for a big lung cancer study reveal

STAT Plus: Alnylam versus BridgeBio: The ATTR-CM debate

An official website of the United States government
The .gov means it’s official. Federal government websites often end in .gov or .mil. Before sharing sensitive information, make sure you’re on a federal government site.
The site is secure. The https:// ensures that you are connecting to the official website and that any information you provide is encrypted and transmitted securely.
- Publications
- Account settings
Preview improvements coming to the PMC website in October 2024. Learn More or Try it out now .
- Advanced Search
- Journal List
- Trop Med Infect Dis

COVID-19: Current Challenges and Future Perspectives
Peter a. leggat.
1 World Health Organization Collaborating Centre for Vector-borne and Neglected Tropical Diseases, College of Public Health, Medical and Veterinary Sciences, James Cook University, Townsville, QLD 4811, Australia
2 School of Public Health, Faculty of Health Sciences, University of the Witwatersrand, Johannesburg 2000, South Africa
3 Centre for Emerging Zoonotic and Parasitic Diseases, National Institute for Communicable Diseases, Johannesburg 2131, South Africa; az.ca.dcin@fnhoj (J.F.); az.ca.dcin@bellicul (L.B.)
Lucille Blumberg
Associated data.
Not applicable.
This Special Issue focuses on recent global research on the current coronavirus (COVID-19) pandemic. The disease is caused by a novel virus, severe acute respiratory syndrome coronavirus 2 (SARS-CoV-2) [ 1 , 2 ]. The International Committee on Taxonomy of Viruses (ICTV) named the virus SARS-CoV-2, as it is genetically related to the coronavirus responsible for the SARS outbreak of 2003 [ 2 ]. While related, the two viruses are quite different in their behaviour. At the time of submission for publication (7 January 2022), COVID-19, named by the World Health Organization (WHO) on 11 February 2020, had caused more than 296.5 million cases and over 5.5 million deaths with over 2.6 million new cases in the past 24 h [ 2 ]. The COVID-19 pandemic has greatly affected the capacity of health systems providing essential health care [ 1 ], but more than 9.195 billion vaccine doses have been administered as of 10 January 2021 [ 2 ]. There have been 22 papers published upon peer review acceptance in this Special Issue, including one editorial, twelve research papers [ 3 , 4 , 5 , 6 , 7 , 8 , 9 , 10 , 11 , 12 , 13 , 14 ], three review papers [ 15 , 16 , 17 ] and seven other papers [ 18 , 19 , 20 , 21 , 22 , 23 , 24 ], including one perspective, two case reports, one brief report, two viewpoints and one commentary. They each contribute to a much better understanding of COVID-19.
The contributions of these papers can be summarized as follows for the 12 research papers. The first of the research papers was a study of mortalities due to COVID-19 through a retrospective, observational study that included all inpatients from a major hospital in Nepal. Interestingly, 16% of patients also showed microbiological evidence of secondary infection [ 3 ]. The second of these studies was a cross-sectional survey exploring the knowledge and perceptions of healthcare workers (HCWs) regarding COVID-19 issues during the second wave of the pandemic, in four tertiary care hospitals in Greece. This study reveals some misconceptions and knowledge gaps in HCWs’ everyday practice, especially regarding hand hygiene and antimicrobial use in COVID-19 patients [ 4 ]. The third study looked at COVID-19 vaccination in those aged 75 years and older in Brazil. COVID-19 vaccines were highly effective in reducing the number of COVID-19-related deaths in over 75-year-olds [ 5 ]. The fourth paper studied persistent symptoms in post-COVID-19 patients attending a follow-up clinic at a tertiary care hospital in Nepal. Of these, 97 (82.2%) patients reported that they had at least one persistent/new symptom beyond two weeks from the diagnosis of COVID-19, including dyspnoea, fatigue, chest heaviness, and cough, emphasizing the need to extend the monitoring of symptoms after discharge [ 6 ]. The fifth of these papers was a study to examine the impact of the COVID-19 pandemic on tuberculosis (TB) and human immunodeficiency virus (HIV) management in Zimbabwe. Impacts were demonstrated on the management of both diseases [ 7 ]. The sixth of these papers was a similar study conducted in Malawi, where there were similar impacts of the COVID-19 pandemic on TB and HIV management [ 8 ]. The seventh of these papers was a similar study conducted in Kenya, where there were similar impacts of the COVID-19 pandemic on TB and HIV management [ 9 ]. The eighth was a case series of five clinically- and laboratory-confirmed COVID-19 patients from Bangladesh, who suffered a second episode of COVID-19 illness after 70 symptom-free days. The study suggested the need for COVID-19 vaccination and continuation of other preventive measures to further mitigate the pandemic [ 10 ]. The ninth of these papers was a case of Plasmodium falciparum malaria in a patient asymptomatically co-infected with COVID-19, which reinforced the need not to miss the diagnosis of malaria infection [ 11 ]. The tenth of these papers was a study looking for common respiratory viruses amongst HCWs with upper and lower respiratory tract infection symptoms in a major hospital in Manila, Philippines. Of these, 38 (13%) identified positive for another viral etiologic agent, compared with seven (2%) who were positive for COVID-19 [ 12 ]. The eleventh of these papers sought to estimate the seroprevalence of COVID-19 in a cohort of general practitioners working in Catania, Italy, after the first wave of COVID-19, which was low (3%) [ 13 ]. The last paper was a survey by the WHO Special Programme for Research and Training in Tropical Disease (TDR) and collaborators of health workers trained through the Structured Operational Research and Training IniTiative (SORT IT). This survey was designed to determine whether they were contributing to the COVID-19 pandemic response and, if so, to map where and how they were applying their SORT IT skills. It showed that investing in training ahead of such public health emergencies is vital [ 14 ].
There were three review papers in this Special Issue. The first of these was a review of the literature on the influenza pandemics of the 20th century, and of the coronavirus and influenza pandemics of the 21st century. There are remarkable similarities among them and it was concluded that the public health response to the COVID-19 pandemic constitutes the basis for delineating best practices to confront future pandemics [ 15 ]. The second was a review of the efficacy and safety of lopinavir–ritonavir (LPV/RTV) in COVID-19. This review did not reveal any significant advantage in the efficacy of LPV/RTV for the treatment of COVID-19 over standard care [ 16 ]. The third review was an evaluation of severity and mortality in COVID-19 patients with underlying kidney and liver diseases. This study found an increased risk of severity and mortality in COVID-19 patients with liver diseases or chronic kidney disease [ 17 ]. There were seven other papers in this Special Issue. The first was a perspective, examining how operational research can improve the delivery of established health interventions and ensure the deployment of new interventions as they become available, irrespective of diseases [ 18 ]. The second was a case report of two cases of heart transplantation with concomitant infections with SARS-CoV-2, with rapid progression to death due to Chagas disease and cytomegalovirus dissemination [ 19 ]. The third was a brief report, which evaluated faecal calprotectin (FC) concentrations in 25 in-patients with COVID-19 pneumonia without gastrointestinal symptoms. Of these, 21 patients showed increased FC and a strong positive correlation between FC and D-dimer [ 20 ]. The fourth was a viewpoint providing an overview of common and different findings for both malaria and COVID-19, with possible mutual influences of one on the other, especially in countries with limited resources [ 21 ]. The fifth was also a viewpoint providing an overview of the potential impact of COVID-19 on TB programs and disease burden, as well as possible strategies that could help to mitigate the impact [ 22 ]. The sixth was a case report involving a patient with severe COVID-19, who was treated with a non-weight-based dosage of tocilizumab to prevent the onset of a cytokine storm. It was concluded that tocilizumab played a substantial role in his ability to avert clinical decline, particularly the need for mechanical ventilation [ 23 ]. The last was a commentary that looked at the challenges faced due to the pandemic, and the steps taken to protect the safety of trial participants and the integrity of the trial in the STREAM Clinical Trial, which is the largest trial for MDR-TB [ 24 ].
The diversity of papers, the depth of the topics and the relative geographical reach of the authors in this Special Issue confirm the continued collective major interest in COVID-19. There are 223 contributors to the 22 papers published in this Special Issue, with affiliations in Europe, Africa, North America, South America and Asia-Pacific. This wide-ranging open access collection contributes to a much better understanding on the epidemiology, presentation, diagnosis, treatment, prevention and control of COVID-19. As the editors of this Special Issue, we trust that you find the content useful, as the authors are pleased to share their knowledge with an international audience.
We currently have another opportunity to update advances in this field through a second Special Issue, “COVID-19: Current Status and Future Prospects”. We encourage you to publish your work in, or propose a Special Issue for, Tropical Medicine and Infectious Disease ( https://www.mdpi.com/journal/tropicalmed ).
Acknowledgments
The Special Issue editors acknowledge all the contributors to this Special Issue.
This research received no external funding.
Institutional Review Board Statement
Informed consent statement, data availability statement, conflicts of interest.
The authors declare no conflict of interest.
Publisher’s Note: MDPI stays neutral with regard to jurisdictional claims in published maps and institutional affiliations.
Thank you for visiting nature.com. You are using a browser version with limited support for CSS. To obtain the best experience, we recommend you use a more up to date browser (or turn off compatibility mode in Internet Explorer). In the meantime, to ensure continued support, we are displaying the site without styles and JavaScript.
- View all journals
- Explore content
- About the journal
- Publish with us
- Sign up for alerts
- Review Article
- Published: 08 September 2024
Peering into the mind: unraveling schizophrenia’s secrets using models
- João V. Nani ORCID: orcid.org/0000-0002-4336-1709 1 , 2 ,
- Alysson R. Muotri 3 &
- Mirian A. F. Hayashi ORCID: orcid.org/0000-0001-6437-4923 1 , 2
Molecular Psychiatry ( 2024 ) Cite this article
Metrics details
- Biological techniques
- Neuroscience
Schizophrenia (SCZ) is a complex mental disorder characterized by a range of symptoms, including positive and negative symptoms, as well as cognitive impairments. Despite the extensive research, the underlying neurobiology of SCZ remain elusive. To overcome this challenge, the use of diverse laboratory modeling techniques, encompassing cellular and animal models, and innovative approaches like induced pluripotent stem cell (iPSC)-derived neuronal cultures or brain organoids and genetically engineered animal models, has been crucial. Immortalized cellular models provide controlled environments for investigating the molecular and neurochemical pathways involved in neuronal function, while iPSCs and brain organoids, derived from patient-specific sources, offer significant advantage in translational research by facilitating direct comparisons of cellular phenotypes between patient-derived neurons and healthy-control neurons. Animal models can recapitulate the different psychopathological aspects that should be modeled, offering valuable insights into the neurobiology of SCZ. In addition, invertebrates’ models are genetically tractable and offer a powerful approach to dissect the core genetic underpinnings of SCZ, while vertebrate models, especially mammals, with their more complex nervous systems and behavioral repertoire, provide a closer approximation of the human condition to study SCZ-related traits. This narrative review provides a comprehensive overview of the diverse modeling approaches, critically evaluating their strengths and limitations. By synthesizing knowledge from these models, this review offers a valuable source for researchers, clinicians, and stakeholders alike. Integrating findings across these different models may allow us to build a more holistic picture of SCZ pathophysiology, facilitating the exploration of new research avenues and informed decision-making for interventions.
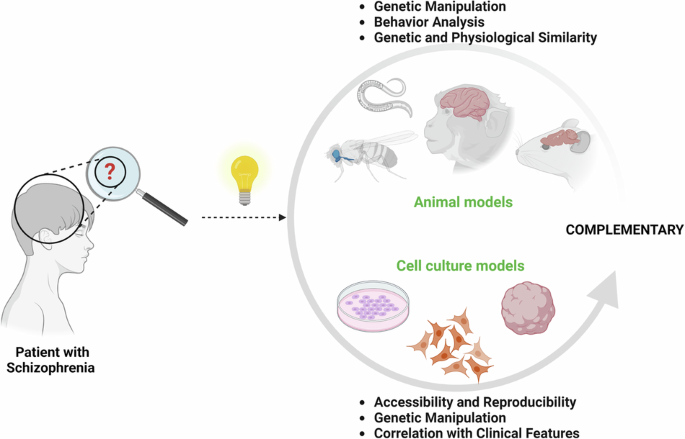
This is a preview of subscription content, access via your institution
Access options
Subscribe to this journal
Receive 12 print issues and online access
251,40 € per year
only 20,95 € per issue
Buy this article
- Purchase on SpringerLink
- Instant access to full article PDF
Prices may be subject to local taxes which are calculated during checkout
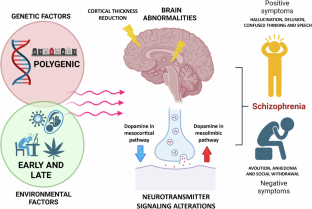
Similar content being viewed by others
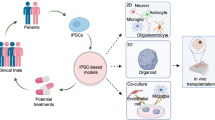
Opportunities and limitations for studying neuropsychiatric disorders using patient-derived induced pluripotent stem cells
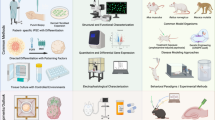
Advancing preclinical models of psychiatric disorders with human brain organoid cultures
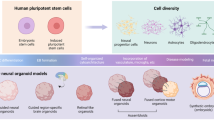
Human pluripotent stem cell (hPSC) and organoid models of autism: opportunities and limitations
Reynolds EH, Kinnier Wilson JV. Psychoses of epilepsy in Babylon: the oldest account of the disorder. Epilepsia. 2008;49:1488–90. https://doi.org/10.1111/j.1528-1167.2008.01614.x .
Article PubMed Google Scholar
Evans K, McGrath J, Milns R. Searching for schizophrenia in ancient Greek and Roman literature: a systematic review. Acta Psychiatr Scand. 2003;107:323–30. https://doi.org/10.1034/j.1600-0447.2003.00053.x .
Article CAS PubMed Google Scholar
Falkai P, Rossner MJ, Schulze TG, Hasan A, Brzózka MM, Malchow B, et al. Kraepelin revisited: schizophrenia from degeneration to failed regeneration. Mol Psychiatry. 2015;20:671–6. https://doi.org/10.1038/mp.2015.35 .
Jablensky A. The diagnostic concept of schizophrenia: its history, evolution, and future prospects. Dialogues Clin Neurosci. 2010;12:271–87. https://doi.org/10.31887/DCNS.2010.12.3/ajablensky .
Article PubMed PubMed Central Google Scholar
Rössler W, Riecher-Rössler A, Meise U. Wilhelm Griesinger and the concept of community care in 19th-century Germany. Hosp Community Psychiatry. 1994;45:818–22. https://doi.org/10.1176/ps.45.8.818 .
Glucksman ML. Freud’s “Project”: The Mind-Brain Connection Revisited. Psychodyn Psychiatry. 2016;44:69–90. https://doi.org/10.1521/pdps.2016.44.1.69 .
Tran The J. Freud, Griesinger and Foville: the influence of the nineteenth-century psychiatric tradition in the Freudian concept of delusion as an ‘attempt at recovery. Hist Psychiatry. 2021;32:323–34. https://doi.org/10.1177/0957154X211013726 .
Kane JM, Correll CU. Past and present progress in the pharmacologic treatment of schizophrenia. J Clin Psychiatry. 2010;71:1115–24. https://doi.org/10.4088/JCP.10r06264yel .
Kesby JP, Eyles DW, McGrath JJ, Scott JG. Dopamine, psychosis and schizophrenia: the widening gap between basic and clinical neuroscience. Transl Psychiatry. 2018;8:30 https://doi.org/10.1038/s41398-017-0071-9 .
Article CAS PubMed PubMed Central Google Scholar
Jauhar S, Johnstone M, McKenna PJ. Schizophrenia. Lancet. 2022;399:473–86. https://doi.org/10.1016/S0140-6736(21)01730-X .
Haijma SV, Van Haren N, Cahn W, Koolschijn PC, Hulshoff Pol HE, Kahn RS. Brain volumes in schizophrenia: a meta-analysis in over 18 000 subjects. Schizophr Bull. 2013;39:1129–38. https://doi.org/10.1093/schbul/sbs118 .
Ban TA. Neuropsychopharmacology and the genetics of schizophrenia: a history of the diagnosis of schizophrenia. Prog Neuropsychopharmacol Biol Psychiatry. 2004;28:753–62. https://doi.org/10.1016/j.pnpbp.2004.05.021 .
Janoutová J, Janácková P, Serý O, Zeman T, Ambroz P, Kovalová M, et al. Epidemiology and risk factors of schizophrenia. Neuro Endocrinol Lett. 2016;37:1–8.
PubMed Google Scholar
Radua J, Ramella-Cravaro V, Ioannidis JPA, Reichenberg A, Phiphopthatsanee N, Amir T, et al. What causes psychosis? An umbrella review of risk and protective factors. World Psychiatry. 2018;17:49–66. https://doi.org/10.1002/wps.20490 .
Tandon R, Gaebel W, Barch DM, Bustillo J, Gur RE, Heckers S, et al. Definition and description of schizophrenia in the DSM-5. Schizophr Res. 2013;150:3–10. https://doi.org/10.1016/j.schres.2013.05.028 .
Hjorthøj C, Stürup AE, McGrath JJ, Nordentoft M. Years of potential life lost and life expectancy in schizophrenia: a systematic review and meta-analysis. Lancet Psychiatry. 2017;4:295–301. https://doi.org/10.1016/S2215-0366(17)30078-0 .
Correll CU, Schooler NR. Negative symptoms in schizophrenia: a review and clinical guide for recognition, assessment, and treatment. Neuropsychiatr Dis Treat. 2020;16:519–34. https://doi.org/10.2147/NDT.S225643 .
Leucht S, Cipriani A, Spineli L, Mavridis D, Orey D, Richter F, et al. Comparative efficacy and tolerability of 15 antipsychotic drugs in schizophrenia: a multiple-treatments meta-analysis. Lancet. 2013;382:951–62. https://doi.org/10.1016/S0140-6736(13)60733-3 .
Maric NP, Jovicic MJ, Mihaljevic M, Miljevic C. Improving current treatments for schizophrenia. Drug Dev Res. 2016;77:357–67. https://doi.org/10.1002/ddr.21337 .
Nucifora FC Jr, Woznica E, Lee BJ, Cascella N, Sawa A. Treatment resistant schizophrenia: clinical, biological, and therapeutic perspectives. Neurobiol Dis. 2019;131:104257. https://doi.org/10.1016/j.nbd.2018.08.016 .
Kane J, Honigfeld G, Singer J, Meltzer H. Clozapine for the treatment-resistant schizophrenic. A double-blind comparison with chlorpromazine. Arch Gen Psychiatry. 1988;45:789–96. https://doi.org/10.1001/archpsyc.1988.01800330013001 .
Leucht S, Corves C, Arbter D, Engel RR, Li C, Davis JM. Second-generation versus first-generation antipsychotic drugs for schizophrenia: a meta-analysis. Lancet. 2009;373:31–41. https://doi.org/10.1016/S0140-6736(08)61764-X .
Fonseca M, Carmo F, Martel F. Metabolic effects of atypical antipsychotics: molecular targets. J Neuroendocrinol. 2023;35:e13347. https://doi.org/10.1111/jne.13347 .
Ribeiro ELA, de Mendonça Lima T, Vieira MEB, Storpirtis S, Aguiar PM. Efficacy and safety of aripiprazole for the treatment of schizophrenia: an overview of systematic reviews. Eur J Clin Pharmacol. 2018;74:1215–33. https://doi.org/10.1007/s00228-018-2498-1 .
Gaitonde SA, Avet C, de la Fuente Revenga M, Blondel-Tepaz E, Shahraki A, Pastor AM, et al. Pharmacological fingerprint of antipsychotic drugs at the serotonin 5-HT2A receptor. Mol Psychiatry. 2024. https://doi.org/10.1038/s41380-024-02531-7 .
Korth C, Fangerau H. Blood tests to diagnose schizophrenia: self-imposed limits in psychiatry. Lancet Psychiatry. 2020;7:911–4. https://doi.org/10.1016/S2215-0366(20)30058-4 .
Owen MJ, Sawa A, Mortensen PB. Schizophrenia. Lancet. 2016;388:86–97. https://doi.org/10.1016/S0140-6736(15)01121-6 .
Ferrer I, Martinez A, Boluda S, Parchi P, Barrachina M. Brain banks: benefits, limitations and cautions concerning the use of post-mortem brain tissue for molecular studies. Cell Tissue Bank. 2008;9:181–94. https://doi.org/10.1007/s10561-008-9077-0 .
Abashkin DA, Kurishev AO, Karpov DS, Golimbet VE. Cellular models in schizophrenia research. Int J Mol Sci. 2021;22:8518. https://doi.org/10.3390/ijms22168518 .
Strickland JC. Guide to research techniques in neuroscience. J Undergrad Neurosci Educ. 2014;13:R1–2.
PubMed Central Google Scholar
Maqsood MI, Matin MM, Bahrami AR, Ghasroldasht MM. Immortality of cell lines: challenges and advantages of establishment. Cell Biol Int. 2013;37:1038–45. https://doi.org/10.1002/cbin.10137 .
Wiatrak B, Kubis-Kubiak A, Piwowar A, Barg E. PC12 cell line: cell types, coating of culture vessels, differentiation and other culture conditions. Cells. 2020;9:958. https://doi.org/10.3390/cells9040958 .
Westerink RH, Ewing AG. The PC12 cell as model for neurosecretion. Acta Physiol. 2008;192:273–85. https://doi.org/10.1111/j.1748-1716.2007.01805.x .
Article CAS Google Scholar
Wang L, Chen Q, Ma R, Zhang B, Yang P, Cao T, et al. Insight into mitochondrial dysfunction mediated by clozapine-induced inhibition of PGRMC1 in PC12 cells. Toxicology. 2023;491:153515. https://doi.org/10.1016/j.tox.2023.153515 .
Jankowska U, Skupien-Rabian B, Swiderska B, Prus G, Dziedzicka-Wasylewska M, Kedracka-Krok S. Proteome analysis of PC12 cells reveals alterations in translation regulation and actin signaling induced by clozapine. Neurochem Res. 2021;46:2097–111. https://doi.org/10.1007/s11064-021-03348-4 .
Zhuo C, Xu Y, Hou W, Chen J, Li Q, Liu Z, et al. Mechanistic/mammalian target of rapamycin and side effects of antipsychotics: insights into mechanisms and implications for therapy. Transl Psychiatry. 2022;12:13. https://doi.org/10.1038/s41398-021-01778-w .
Xiong YJ, Song YZ, Zhu Y, Zuo WQ, Zhao YF, Shen X, et al. Neuroprotective effects of olanzapine against rotenone-induced toxicity in PC12 cells. Acta Pharmacol Sin. 2020;41:508–15. https://doi.org/10.1038/s41401-020-0378-6 .
Wei Z, Bai O, Richardson JS, Mousseau DD, Li XM. Olanzapine protects PC12 cells from oxidative stress induced by hydrogen peroxide. J Neurosci Res. 2003;73:364–8. https://doi.org/10.1002/jnr.10668 .
Magliaro BC, Saldanha CJ. Clozapine protects PC-12 cells from death due to oxidative stress induced by hydrogen peroxide via a cell-type specific mechanism involving inhibition of extracellular signal-regulated kinase phosphorylation. Brain Res. 2009;1283:14–24. https://doi.org/10.1016/j.brainres.2009.05.063 .
Wang H, Xu H, Dyck LE, Li XM. Olanzapine and quetiapine protect PC12 cells from beta-amyloid peptide(25-35)-induced oxidative stress and the ensuing apoptosis. J Neurosci Res. 2005;81:572–80. https://doi.org/10.1002/jnr.20570 .
Ishizuka K, Paek M, Kamiya A, Sawa A. A review of disrupted-in-schizophrenia-1 (DISC1): neurodevelopment, cognition, and mental conditions. Biol Psychiatry. 2006;59:1189–97. https://doi.org/10.1016/j.biopsych.2006.03.065 .
Dahoun T, Trossbach SV, Brandon NJ, Korth C, Howes OD. The impact of disrupted-in-schizophrenia 1 (DISC1) on the dopaminergic system: a systematic review. Transl Psychiatry. 2017;7:e1015. https://doi.org/10.1038/tp.2016.282 .
Kamiya A, Tomoda T, Chang J, Takaki M, Zhan C, Morita M, et al. DISC1-NDEL1/NUDEL protein interaction, an essential component for neurite outgrowth, is modulated by genetic variations of DISC1. Hum Mol Genet. 2006;15:3313–23. https://doi.org/10.1093/hmg/ddl407 .
Taya S, Shinoda T, Tsuboi D, Asaki J, Nagai K, Hikita T, et al. DISC1 regulates the transport of the NUDEL/LIS1/14-3-3epsilon complex through kinesin-1. J Neurosci. 2007;27:15–26. https://doi.org/10.1523/JNEUROSCI.3826-06.2006 .
Pletnikov MV, Xu Y, Ovanesov MV, Kamiya A, Sawa A, Ross CA. PC12 cell model of inducible expression of mutant DISC1: new evidence for a dominant-negative mechanism of abnormal neuronal differentiation. Neurosci Res. 2007;8:234–44. https://doi.org/10.1016/j.neures.2007.03.003 .
Pezzini F, Bettinetti L, Di Leva F, Bianchi M, Zoratti E, Carrozzo R, et al. Transcriptomic profiling discloses molecular and cellular events related to neuronal differentiation in SH-SY5Y neuroblastoma cells. Cell Mol Neurobiol. 2017;37:665–82. https://doi.org/10.1007/s10571-016-0403-y .
Kovalevich J, Langford D. Considerations for the use of SH-SY5Y neuroblastoma cells in neurobiology. Methods Mol Biol. 2013;1078:9–21. https://doi.org/10.1007/978-1-62703-640-5_2 .
Shin JH, Park SJ, Kim ES, Jo YK, Hong J, Cho DH. Sertindole, a potent antagonist at dopamine D 2 receptors, induces autophagy by increasing reactive oxygen species in SH-SY5Y neuroblastoma cells. Biol Pharm Bull. 2012;35:1069–75. https://doi.org/10.1248/bpb.b12-00009 .
Lee JG, Cho HY, Park SW, Seo MK, Kim YH. Effects of olanzapine on brain-derived neurotrophic factor gene promoter activity in SH-SY5Y neuroblastoma cells. Prog Neuropsychopharmacol Biol Psychiatry. 2010;34:1001–6. https://doi.org/10.1016/j.pnpbp.2010.05.013 .
Goldie BJ, Barnett MM, Cairns MJ. BDNF and the maturation of posttranscriptional regulatory networks in human SH-SY5Y neuroblast differentiation. Front Cell Neurosci. 2014;8:325. https://doi.org/10.3389/fncel.2014.00325 .
Svoboda J, Stankova A, Entlerova M, Stuchlik A. Acute administration of MK-801 in an animal model of psychosis in rats interferes with cognitively demanding forms of behavioral flexibility on a rotating arena. Front Behav Neurosci. 2015;9:75. https://doi.org/10.3389/fnbeh.2015.00075 .
Zhu D, Zhang J, Wu J, Li G, Yao W, Hao J, et al. Paliperidone protects SH-SY5Y cells against MK-801-induced neuronal damage through inhibition of Ca(2+) influx and regulation of SIRT1/miR-134 signal pathway. Mol Neurobiol. 2016;53:2498–509. https://doi.org/10.1007/s12035-015-9217-z .
Unal G, Dokumaci AH, Ozkartal CS, Yerer MB, Aricioglu F. Famotidine has a neuroprotective effect on MK-801 induced toxicity via the Akt/GSK-3β/β-catenin signaling pathway in the SH-SY5Y cell line. Chem Biol Interact. 2019;314:108823. https://doi.org/10.1016/j.cbi.2019.108823 .
Mellios N, Sur M. The emerging role of microRNAs in schizophrenia and autism spectrum disorders. Front Psychiatry. 2012;3:39. https://doi.org/10.3389/fpsyt.2012.00039 .
Ryskalin L, Limanaqi F, Frati A, Busceti CL, Fornai F. mTOR-related brain dysfunctions in neuropsychiatric disorders. Int J Mol Sci. 2018;19:2226. https://doi.org/10.3390/ijms19082226 .
Kruse AO, Bustillo JR. Glutamatergic dysfunction in schizophrenia. Transl Psychiatry. 2022;12:500. https://doi.org/10.1038/s41398-022-02253-w .
Eastwood SL, Walker M, Hyde TM, Kleinman JE, Harrison PJ. The DISC1 Ser704Cys substitution affects centrosomal localization of its binding partner PCM1 in glia in human brain. Hum Mol Genet. 2010;19:2487–96. https://doi.org/10.1093/hmg/ddq130 .
Piñero-Martos E, Ortega-Vila B, Pol-Fuster J, Cisneros-Barroso E, Ruiz-Guerra L, Medina-Dols A, et al. Disrupted in schizophrenia 1 (DISC1) is a constituent of the mammalian mitochondrial contact site and cristae organizing system (MICOS) complex, and is essential for oxidative phosphorylation. Hum Mol Genet. 2016;25:4157–69. https://doi.org/10.1093/hmg/ddw250 .
Ramos A, Rodríguez-Seoane C, Rosa I, Gorroño-Etxebarria I, Alonso J, Veiga S, et al. Proteomic studies reveal disrupted in schizophrenia 1 as a player in both neurodevelopment and synaptic function. Int J Mol Sci. 2018;20:119. https://doi.org/10.3390/ijms20010119 .
Trossbach SV, Bader V, Hecher L, Pum ME, Masoud ST, Prikulis I, et al. Misassembly of full-length Disrupted-in-Schizophrenia 1 protein is linked to altered dopamine homeostasis and behavioral deficits. Mol Psychiatry. 2016;21:1561–72. https://doi.org/10.1038/mp.2015.194 .
Pertile RA, Cui X, Eyles DW. Vitamin D signaling and the differentiation of developing dopamine systems. Neuroscience. 2016;333:193–203. https://doi.org/10.1016/j.neuroscience.2016.07.020 .
Sei Y, Li Z, Song J, Ren-Patterson R, Tunbridge EM, Iizuka Y, et al. Epistatic and functional interactions of catechol-o-methyltransferase (COMT) and AKT1 on neuregulin1-ErbB signaling in cell models. PLoS ONE. 2010;5:e10789. https://doi.org/10.1371/journal.pone.0010789 .
Wu S, Wang P, Tao R, Yang P, Yu X, Li Y, et al. Schizophrenia associated microRNA 148b 3p regulates COMT and PRSS16 expression by targeting the ZNF804A gene in human neuroblastoma cells. Mol Med Rep. 2020;22:1429–39. https://doi.org/10.3892/mmr.2020.11230 .
Khavari B, Mahmoudi E, Geaghan MP, Cairns MJ. Oxidative stress impact on the transcriptome of differentiating neuroblastoma cells: implication for psychiatric disorders. Int J Mol Sci. 2020;21:9182. https://doi.org/10.3390/ijms21239182 .
Larouche A, Berube P, Sarret P, Grignon S. Subacute H2O2, but not poly(IC), upregulates dopamine D2 receptors in retinoic acid differentiated SH-SY5Y neuroblastoma. Synapse. 2008;62:70–3. https://doi.org/10.1002/syn.20458 .
Deslauriers J, Lefrançois M, Larouche A, Sarret P, Grignon S. Antipsychotic-induced DRD2 upregulation and its prevention by α-lipoic acid in SH-SY5Y neuroblastoma cells. Synapse. 2011;65:321–31. https://doi.org/10.1002/syn.20851 .
Sato T, Okumura F, Kano S, Kondo T, Ariga T, Hatakeyama S. TRIM32 promotes neural differentiation through retinoic acid receptor-mediated transcription. J Cell Sci. 2011;124:3492–502. https://doi.org/10.1242/jcs.088799 .
Yap MY, Lo YL, Talbot K, Ong WY. Oxidative stress reduces levels of dysbindin-1A via its PEST domain. Neurochem Int. 2014;79:65–9. https://doi.org/10.1016/j.neuint.2014.10.001 .
Jäkel S, Dimou L. Glial cells and their function in the adult brain: a journey through the history of their ablation. Front Cell Neurosci. 2017;11:24. https://doi.org/10.3389/fncel.2017.00024 .
Silver J, Schwab ME, Popovich PG. Central nervous system regenerative failure: role of oligodendrocytes, astrocytes, and microglia. Cold Spring Harb Perspect Biol. 2014;7:a020602. https://doi.org/10.1101/cshperspect.a020602 .
Almeida PGC, Nani JV, Oses JP, Brietzke E, Hayashi MAF. Neuroinflammation and glial cell activation in mental disorders. Brain Behav Immun Health. 2019;2:100034. https://doi.org/10.1016/j.bbih.2019.100034 .
Nani JV, Almeida PGC, Noto C, Bressan RA, Brietzke E, Hayashi MAF. Unraveiling the correlation among neurodevelopmental and inflammatory biomarkers in patients with chronic schizophrenia. Nord J Psychiatry. 2022;76:559–64. https://doi.org/10.1080/08039488.2021.2023217 .
Gadelha A, Yonamine CM, Nering M, Rizzo LB, Noto C, Cogo-Moreira H, et al. Angiotensin converting enzyme activity is positively associated with IL-17a levels in patients with schizophrenia. Psychiatry Res. 2015;229:702–7. https://doi.org/10.1016/j.psychres.2015.08.018 .
Hartmann SM, Heider J, Wüst R, Fallgatter AJ, Volkmer H. Microglia-neuron interactions in schizophrenia. Front Cell Neurosci. 2024;18:1345349. https://doi.org/10.3389/fncel.2024.1345349 .
Goldsmith DR, Rapaport MH. Inflammation and negative symptoms of schizophrenia: implications for reward processing and motivational deficits. Front Psychiatry. 2020;11:46. https://doi.org/10.3389/fpsyt.2020.00046 .
De Vries GH, Boullerne AI. Glial cell lines: an overview. Neurochem Res. 2010;35:1978–2000. https://doi.org/10.1007/s11064-010-0318-9 .
Blasi E, Barluzzi R, Bocchini V, Mazzolla R, Bistoni F. Immortalization of murine microglial cells by a v-raf/v-myc carrying retrovirus. J Neuroimmunol. 1990;27:229–37. https://doi.org/10.1016/0165-5728(90)90073-v .
Horvath RJ, Nutile-McMenemy N, Alkaitis MS, Deleo JA. Differential migration, LPS-induced cytokine, chemokine, and NO expression in immortalized BV-2 and HAPI cell lines and primary microglial cultures. J Neurochem. 2008;107:557–69. https://doi.org/10.1111/j.1471-4159.2008.05633.x .
Sarapultsev A, Gusev E, Komelkova M, Utepova I, Luo S, Hu D. JAK-STAT signaling in inflammation and stress-related diseases: implications for therapeutic interventions. Mol Biomed. 2023;4:40. https://doi.org/10.1186/s43556-023-00151-1 .
Long Y, Wang Y, Shen Y, Huang J, Li Y, Wu R, et al. Minocycline and antipsychotics inhibit inflammatory responses in BV-2 microglia activated by LPS via regulating the MAPKs/ JAK-STAT signaling pathway. BMC Psychiatry. 2023;23:514. https://doi.org/10.1186/s12888-023-05014-1 .
Zheng LT, Hwang J, Ock J, Lee MG, Lee WH, Suk K. The antipsychotic spiperone attenuates inflammatory response in cultured microglia via the reduction of proinflammatory cytokine expression and nitric oxide production. J Neurochem. 2008;107:1225–35. https://doi.org/10.1111/j.1471-4159.2008.05675.x .
Racki V, Marcelic M, Stimac I, Petric D, Kucic N. Effects of haloperidol, risperidone, and aripiprazole on the immunometabolic properties of BV-2 microglial cells. Int J Mol Sci. 2021;22:4399. https://doi.org/10.3390/ijms22094399 .
Ng BG, Xu G, Chandy N, Steyermark J, Shinde DN, Radtke K, et al. Biallelic mutations in FUT8 cause a congenital disorder of glycosylation with defective fucosylation. Am J Hum Genet. 2018;102:188–95. https://doi.org/10.1016/j.ajhg.2017.12.009 .
Fukuda T, Hashimoto H, Okayasu N, Kameyama A, Onogi H, Nakagawasai O, et al. Alpha1,6-fucosyltransferase-deficient mice exhibit multiple behavioral abnormalities associated with a schizophrenia-like phenotype: importance of the balance between the dopamine and serotonin systems. J Biol Chem. 2011;286:18434–43. https://doi.org/10.1074/jbc.M110.172536 .
Lu X, Zhang D, Shoji H, Duan C, Zhang G, Isaji T, et al. Deficiency of α1,6-fucosyltransferase promotes neuroinflammation by increasing the sensitivity of glial cells to inflammatory mediators. Biochim Biophys Acta Gen Subj. 2019;1863:598–608. https://doi.org/10.1016/j.bbagen.2018.12.008 .
Takahashi K, Yamanaka S. Induction of pluripotent stem cells from mouse embryonic and adult fibroblast cultures by defined factors. Cell. 2006;126:663–76. https://doi.org/10.1016/j.cell.2006.07.024 .
McKinney CE. Using induced pluripotent stem cells derived neurons to model brain diseases. Neural Regen Res. 2017;12:1062–7. https://doi.org/10.4103/1673-5374.211180 .
Heider J, Vogel S, Volkmer H, Breitmeyer R. Human iPSC-derived glia as a tool for neuropsychiatric research and drug development. Int J Mol Sci. 2021;22:10254. https://doi.org/10.3390/ijms221910254 .
Page SC, Sripathy SR, Farinelli F, Ye Z, Wang Y, Hiler DJ, et al. Electrophysiological measures from human iPSC-derived neurons are associated with schizophrenia clinical status and predict individual cognitive performance. Proc Natl Acad Sci USA. 2022;119:e2109395119. https://doi.org/10.1073/pnas.2109395119 .
Hribkova H, Svoboda O, Bartecku E, Zelinkova J, Horinkova J, Lacinova L, et al. Clozapine reverses dysfunction of glutamatergic neurons derived from clozapine-responsive schizophrenia patients. Front Cell Neurosci. 2022;16:830757. https://doi.org/10.3389/fncel.2022.830757 .
Brennand KJ, Simone A, Jou J, Gelboin-Burkhart C, Tran N, Sangar S, et al. Modelling schizophrenia using human induced pluripotent stem cells. Nature. 2011;473:221–5. https://doi.org/10.1038/nature09915 .
Hook V, Brennand KJ, Kim Y, Toneff T, Funkelstein L, Lee KC. Human iPSC neurons display activity-dependent neurotransmitter secretion: aberrant catecholamine levels in schizophrenia neurons. Stem Cell Rep. 2014;3:531–8. https://doi.org/10.1016/j.stemcr.2014.08.001 .
Zuccoli GS, Nascimento JM, Moraes-Vieira PM, Rehen SK, Martins-de-Souza D. Mitochondrial, cell cycle control and neuritogenesis alterations in an iPSC-based neurodevelopmental model for schizophrenia. Eur Arch Psychiatry Clin Neurosci. 2023. https://doi.org/10.1007/s00406-023-01605-x .
Tay SH, Winanto, Khong ZJ, Koh YH, Ng SY. Generation of cortical, dopaminergic, motor, and sensory neurons from human pluripotent stem cells. Methods Mol Biol. 2022;2549:359–77. https://doi.org/10.1007/7651_2021_399 .
Ni P, Noh H, Park GH, Shao Z, Guan Y, Park JM, et al. iPSC-derived homogeneous populations of developing schizophrenia cortical interneurons have compromised mitochondrial function. Mol Psychiatry. 2020;25:3103–4. https://doi.org/10.1038/s41380-019-0423-3 .
Kathuria A, Lopez-Lengowski K, Watmuff B, McPhie D, Cohen BM, Karmacharya R. Synaptic deficits in iPSC-derived cortical interneurons in schizophrenia are mediated by NLGN2 and rescued by N-acetylcysteine. Transl Psychiatry. 2019;9:321. https://doi.org/10.1038/s41398-019-0660-x .
Park JM, Liu D, Park GH, Noh H, Ni P, Yin C, et al. Migratory cortical interneuron-specific transcriptome abnormalities in schizophrenia. J Psychiatr Res. 2021;137:111–6. https://doi.org/10.1016/j.jpsychires.2021.02.054 .
Shao Z, Noh H, Bin Kim W, Ni P, Nguyen C, Cote SE, et al. Dysregulated protocadherin-pathway activity as an intrinsic defect in induced pluripotent stem cell-derived cortical interneurons from subjects with schizophrenia. Nat Neurosci. 2019;22:229–42. https://doi.org/10.1038/s41593-018-0313-z .
Farrelly LA, Zheng S, Schrode N, Topol A, Bhanu NV, Bastle RM, et al. Chromatin profiling in human neurons reveals aberrant roles for histone acetylation and BET family proteins in schizophrenia. Nat Commun. 2022;13:2195. https://doi.org/10.1038/s41467-022-29922-0 .
Nascimento JM, Saia-Cereda VM, Zuccoli GS, Reis-de-Oliveira G, Carregari VC, Smith BJ, et al. Proteomic signatures of schizophrenia-sourced iPSC-derived neural cells and brain organoids are similar to patients’ postmortem brains. Cell Biosci. 2022;12:189. https://doi.org/10.1186/s13578-022-00928-x .
Hoffman GE, Hartley BJ, Flaherty E, Ladran I, Gochman P, Ruderfer DM, et al. Transcriptional signatures of schizophrenia in hiPSC-derived NPCs and neurons are concordant with post-mortem adult brains. Nat Commun. 2017;8:2225. https://doi.org/10.1038/s41467-017-02330-5 .
Lin M, Pedrosa E, Hrabovsky A, Chen J, Puliafito BR, Gilbert SR, et al. Integrative transcriptome network analysis of iPSC-derived neurons from schizophrenia and schizoaffective disorder patients with 22q11.2 deletion. BMC Syst Biol. 2016;10:105. https://doi.org/10.1186/s12918-016-0366-0 .
Li J, Ryan SK, Deboer E, Cook K, Fitzgerald S, Lachman HM, et al. Mitochondrial deficits in human iPSC-derived neurons from patients with 22q11.2 deletion syndrome and schizophrenia. Transl Psychiatry. 2019;9:302. https://doi.org/10.1038/s41398-019-0643-y .
Kim NS, Wen Z, Liu J, Zhou Y, Guo Z, Xu C, et al. Pharmacological rescue in patient iPSC and mouse models with a rare DISC1 mutation. Nat Commun. 2021;12:1398. https://doi.org/10.1038/s41467-021-21713-3 .
Kikuchi M, Nakazawa T, Kinoshita M, Yamamori H, Yasuda Y, Fujimoto M, et al. Methylation analysis in monozygotic twins with treatment-resistant schizophrenia and discordant responses to clozapine. Front Psychiatry. 2021;12:734606. https://doi.org/10.3389/fpsyt.2021.734606 .
Kathuria A, Lopez-Lengowski K, Roffman JL, Karmacharya R. Distinct effects of interleukin-6 and interferon-γ on differentiating human cortical neurons. Brain Behav Immun. 2022;103:97–108. https://doi.org/10.1016/j.bbi.2022.04.007 .
Guennewig B, Bitar M, Obiorah I, Hanks J, O’Brien EA, Kaczorowski DC, et al. THC exposure of human iPSC neurons impacts genes associated with neuropsychiatric disorders. Transl Psychiatry. 2018;8:89. https://doi.org/10.1038/s41398-018-0137-3 .
Trindade P, Nascimento JM, Casas BS, Monteverde T, Gasparotto J, Ribeiro CT, et al. Induced pluripotent stem cell-derived astrocytes from patients with schizophrenia exhibit an inflammatory phenotype that affects vascularization. Mol Psychiatry. 2023;28:871–82. https://doi.org/10.1038/s41380-022-01830-1 .
Koskuvi M, Lehtonen Š, Trontti K, Keuters M, Wu YC, Koivisto H, et al. Contribution of astrocytes to familial risk and clinical manifestation of schizophrenia. Glia. 2022;70:650–60. https://doi.org/10.1002/glia.24131 .
Couch ACM, Solomon S, Duarte RRR, Marrocu A, Sun Y, Sichlinger L, et al. Acute IL-6 exposure triggers canonical IL6Ra signaling in hiPSC microglia, but not neural progenitor cells. Brain Behav Immun. 2023;110:43–59. https://doi.org/10.1016/j.bbi.2023.02.007 .
Qian X, Song H, Ming GL. Brain organoids: advances, applications and challenges. Development. 2019;146:dev166074. https://doi.org/10.1242/dev.166074 .
Trujillo CA, Muotri AR. Brain organoids and the study of neurodevelopment. Trends Mol Med. 2018;24:982–90. https://doi.org/10.1016/j.molmed.2018.09.005 .
Trujillo CA, Gao R, Negraes PD, Gu J, Buchanan J, Preissl S, et al. Complex oscillatory waves emerging from cortical organoids model early human brain network development. Cell Stem Cell. 2019;25:558–69.e7. https://doi.org/10.1016/j.stem.2019.08.002 .
Tan HY, Cho H, Lee LP. Human mini-brain models. Nat Biomed Eng. 2021;5:11–25. https://doi.org/10.1038/s41551-020-00643-3 .
Bagley JA, Reumann D, Bian S, Lévi-Strauss J, Knoblich JA. Fused cerebral organoids model interactions between brain regions. Nat Methods. 2017;14:743–51. https://doi.org/10.1038/nmeth.4304 .
Kathuria A, Lopez-Lengowski K, Jagtap SS, McPhie D, Perlis RH, Cohen BM, et al. Transcriptomic landscape and functional characterization of induced pluripotent stem cell-derived cerebral organoids in schizophrenia. JAMA Psychiatry. 2020;77:745–54. https://doi.org/10.1001/jamapsychiatry.2020.0196 .
Benson CA, Powell HR, Liput M, Dinham S, Freedman DA, Ignatowski TA, et al. Immune factor, TNFα, disrupts human brain organoid development similar to schizophrenia-schizophrenia increases developmental vulnerability to TNFα. Front Cell Neurosci. 2020;14:233. https://doi.org/10.3389/fncel.2020.00233 .
Notaras M, Lodhi A, Dündar F, Collier P, Sayles NM, Tilgner H, et al. Schizophrenia is defined by cell-specific neuropathology and multiple neurodevelopmental mechanisms in patient-derived cerebral organoids. Mol Psychiatry. 2022;27:1416–34. https://doi.org/10.1038/s41380-021-01316-6 .
Notaras M, Lodhi A, Fang H, Greening D, Colak D. The proteomic architecture of schizophrenia iPSC-derived cerebral organoids reveals alterations in GWAS and neuronal development factors. Transl Psychiatry. 2021;11:541. https://doi.org/10.1038/s41398-021-01664-5 .
Bradshaw NJ, Hayashi MA. NDE1 and NDEL1 from genes to (mal)functions: parallel but distinct roles impacting on neurodevelopmental disorders and psychiatric illness. Cell Mol Life Sci. 2017;74:1191–210. https://doi.org/10.1007/s00018-016-2395-7 .
Dal Mas C, Nani JV, Noto C, Yonamine CM, da Cunha GR, Mansur RB, et al. Ndel1NDEL1 oligopeptidase activity as a potential biomarker of early stages of schizophrenia. Schizophr Res. 2019;208:202–8. https://doi.org/10.1016/j.schres.2019.02.021 .
Gadelha A, Machado MF, Yonamine CM, Sato JR, Juliano MA, Oliveira V, et al. Plasma Ndel1NDEL1 enzyme activity is reduced in patients with schizophrenia—a potential biomarker? J Psychiatr Res. 2013;47:657–63. https://doi.org/10.1016/j.jpsychires.2013.01.009 .
Ye F, Kang E, Yu C, Qian X, Jacob F, Yu C, et al. DISC1 regulates neurogenesis via modulating kinetochore attachment of Ndel1NDEL1/Nde1 during mitosis. Neuron. 2017;96:1041–-54.e5. https://doi.org/10.1016/j.neuron.2017.10.010 .
Papes F, Camargo AP, de Souza JS, Carvalho VMA, Szeto RA, LaMontagne E, et al. Transcription Factor 4 loss-of-function is associated with deficits in progenitor proliferation and cortical neuron content. Nat Commun. 2022;13:2387. https://doi.org/10.1038/s41467-022-29942-w .
Goo BS, Mun DJ, Kim S, Nhung TTM, Lee SB, Woo Y, et al. Schizophrenia-associated mitotic arrest deficient-1 (MAD1) regulates the polarity of migrating neurons in the developing neocortex. Mol Psychiatry. 2023;28:856–70. https://doi.org/10.1038/s41380-022-01856-5 .
Robinson NB, Krieger K, Khan FM, Huffman W, Chang M, Naik A, et al. The current state of animal models in research: a review. Int J Surg. 2019;72:9–13. https://doi.org/10.1016/j.ijsu.2019.10.015 .
Suo S, Ishiura S, Van Tol HH. Dopamine receptors in C. elegans . Eur J Pharmacol. 2004;500:159–66. https://doi.org/10.1016/j.ejphar.2004.07.021 .
Furukubo-Tokunaga K. Modeling schizophrenia in flies. Prog Brain Res. 2009;179:107–15. https://doi.org/10.1016/S0079-6123(09)17912-8 .
Beauchamp A, Yee Y, Darwin BC, Raznahan A, Mars RB, Lerch JP. Whole-brain comparison of rodent and human brains using spatial transcriptomics. Elife. 2022;11:e79418. https://doi.org/10.7554/eLife.79418 .
Semple BD, Blomgren K, Gimlin K, Ferriero DM, Noble-Haeusslein LJ. Brain development in rodents and humans: Identifying benchmarks of maturation and vulnerability to injury across species. Prog Neurobiol. 2013;106-107:1–16. https://doi.org/10.1016/j.pneurobio.2013.04.001 .
Nani JV, Rodríguez B, Cruz FC, Hayashi MAF. Animal models in psychiatric disorder studies. Animal models in medicine and biology. IntechOpen. 2020. https://doi.org/10.5772/intechopen.89034 .
Nagy S, Maurer GW, Hentze JL, Rose M, Werge TM, Rewitz K. AMPK signaling linked to the schizophrenia-associated 1q21.1 deletion is required for neuronal and sleep maintenance. PLoS Genet. 2018;14:e1007623. https://doi.org/10.1371/journal.pgen.1007623 .
Mullin AP, Sadanandappa MK, Ma W, Dickman DK, VijayRaghavan K, Ramaswami M, et al. Gene dosage in the dysbindin schizophrenia susceptibility network differentially affect synaptic function and plasticity. J Neurosci. 2015;35:325–38. https://doi.org/10.1523/JNEUROSCI.3542-14.2015 .
Hidalgo S, Castro C, Zárate RV, Valderrama BP, Hodge JJL, Campusano JM. The behavioral and neurochemical characterization of a Drosophila dysbindin mutant supports the contribution of serotonin to schizophrenia negative symptoms. Neurochem Int. 2020;138:104753. https://doi.org/10.1016/j.neuint.2020.104753 .
Lu B, Shao L, Feng S, Wang T, Zhong Y. The β-alanyl-monoamine synthase ebony is regulated by schizophrenia susceptibility gene dysbindin in Drosophila. Sci China Life Sci. 2014;57:46–51. https://doi.org/10.1007/s11427-013-4595-9 .
Sawamura N, Ando T, Maruyama Y, Fujimuro M, Mochizuki H, Honjo K, et al. Nuclear DISC1 regulates CRE-mediated gene transcription and sleep homeostasis in the fruit fly. Mol Psychiatry. 2008;13:1138–48. https://doi.org/10.1038/mp.2008.101 .
Shao L, Lu B, Wen Z, Teng S, Wang L, Zhao Y, et al. Disrupted-in-Schizophrenia-1 (DISC1) protein disturbs neural function in multiple disease-risk pathways. Hum Mol Genet. 2017;26:2634–48. https://doi.org/10.1093/hmg/ddx147 .
Ferguson L, Petty A, Rohrscheib C, Troup M, Kirszenblat L, Eyles DW, et al. Transient dysregulation of dopamine signaling in a developing drosophila arousal circuit permanently impairs behavioral responsiveness in adults. Front Psychiatry. 2017;8:22. https://doi.org/10.3389/fpsyt.2017.00022 .
Moulin TC, Stojanovic T, Rajesh RP, Pareek T, Donzelli L, Williams MJ, et al. Effects of transient administration of the NMDA receptor antagonist MK-801 in drosophila melanogaster activity, sleep, and negative geotaxis. Biomedicines. 2023;11:192. https://doi.org/10.3390/biomedicines11010192 .
Navrotskaya V, Wnorowski A, Turski W, Oxenkrug G. Effect of kynurenic acid on pupae viability of drosophila melanogaster cinnabar and cardinal eye color mutants with altered tryptophan-kynurenine metabolism. Neurotox Res. 2018;34:324–31. https://doi.org/10.1007/s12640-018-9891-5 .
Christensen EL, Beasley A, Radchuk J, Mielko ZE, Preston E, Stuckett S, et al. ngn-1/neurogenin activates transcription of multiple terminal selector transcription factors in the Caenorhabditis elegans nervous system. G3. 2020;10:1949–62. https://doi.org/10.1534/g3.120.401126 .
Chen SY, Huang PH, Cheng HJ. Disrupted-in-Schizophrenia 1-mediated axon guidance involves TRIO-RAC-PAK small GTPase pathway signaling. Proc Natl Acad Sci USA. 2011;108:5861–6. https://doi.org/10.1073/pnas.1018128108 .
Monte GG, Nani JV, de Almeida Campos MR, Dal Mas C, Marins LAN, Martins LG, et al. Impact of nuclear distribution element genes in the typical and atypical antipsychotics effects on nematode Caenorhabditis elegans : Putative animal model for studying the pathways correlated to schizophrenia. Prog Neuropsychopharmacol Biol Psychiatry. 2019;92:19–30. https://doi.org/10.1016/j.pnpbp.2018.12.010 .
Pedrini M, Cao B, Nani JVS, Cerqueira RO, Mansur RB, Tasic L, et al. Advances and challenges in development of precision psychiatry through clinical metabolomics on mood and psychotic disorders. Prog Neuropsychopharmacol Biol Psychiatry. 2019;93:182–8. https://doi.org/10.1016/j.pnpbp.2019.03.010 .
Molina JD, Avila S, Rubio G, López-Muñoz F. Metabolomic connections between schizophrenia, antipsychotic drugs and metabolic syndrome: a variety of players. Curr Pharm Des. 2021;27:4049–61. https://doi.org/10.2174/1381612827666210804110139 .
Rodríguez B, Nani JV, Almeida PGC, Brietzke E, Lee RS, Hayashi MAF. Neuropeptides and oligopeptidases in schizophrenia. Neurosci Biobehav Rev. 2020;108:679–93. https://doi.org/10.1016/j.neubiorev.2019.11.024 .
Campeiro JD, Nani JV, Monte GG, Almeida PGC, Mori MA, Hayashi MAF. Regulation of monoamine levels by typical and atypical antipsychotics in Caenorhabditis elegans mutant for nuclear distribution element genes. Neurochem Int. 2021;147:105047. https://doi.org/10.1016/j.neuint.2021.105047 .
Karmacharya R, Lynn SK, Demarco S, Ortiz A, Wang X, Lundy MY, et al. Behavioral effects of clozapine: involvement of trace amine pathways in C. elegans and M. musculus . Brain Res. 2011;1393:91–9. https://doi.org/10.1016/j.brainres.2011.04.010 .
Osuna-Luque J, Rodríguez-Ramos Á, Gámez-Del-Estal MDM, Ruiz-Rubio M. Behavioral mechanisms that depend on dopamine and serotonin in Caenorhabditis elegans interact with the antipsychotics risperidone and aripiprazole. J Exp Neurosci. 2018;12:1179069518798628. https://doi.org/10.1177/1179069518798628 .
Refai O, Blakely RD. Blockade and reversal of swimming-induced paralysis in C. elegans by the antipsychotic and D2-type dopamine receptor antagonist azaperone. Neurochem Int. 2019;123:59–68. https://doi.org/10.1016/j.neuint.2018.05.013 .
Maior RS, Nishijo H, Caixeta FV. Editorial: Non-human primate models of psychiatric disorders. Front Behav Neurosci. 2021;15:774064. https://doi.org/10.3389/fnbeh.2021.774064 .
Fedigan LM. Ethical issues faced by field primatologists: asking the relevant questions. Am J Primatol. 2010;72:754–71. https://doi.org/10.1002/ajp.20814 .
Campbell PD, Granato M. Zebrafish as a tool to study schizophrenia-associated copy number variants. Dis Model Mech. 2020;13:dmm043877. https://doi.org/10.1242/dmm.043877 .
Carlsson A, Lindqvist M. Effect of chlorpromazine or haloperidol on formation of 3methoxytyramine and normetanephrine in mouse brain. Acta Pharmacol Toxicol. 1963;20:140–4. https://doi.org/10.1111/j.1600-0773.1963.tb01730.x .
McCutcheon RA, Abi-Dargham A, Howes OD. Schizophrenia, dopamine and the striatum: from biology to symptoms. Trends Neurosci. 2019;42:205–20. https://doi.org/10.1016/j.tins.2018.12.004 .
Canetta S, Kellendonk C. Can we use mice to study schizophrenia? Philos Trans R Soc Lond B Biol Sci. 2018;373:20170032. https://doi.org/10.1098/rstb.2017.0032 .
Wang P, Li M, Zhao A, Ma J. Application of animal experimental models in the research of schizophrenia. Am J Med Genet B Neuropsychiatr Genet. 2021;186:209–27. https://doi.org/10.1002/ajmg.b.32863 .
Winship IR, Dursun SM, Baker GB, Balista PA, Kandratavicius L, Maia-de-Oliveira JP, et al. An overview of animal models related to schizophrenia. Can J Psychiatry. 2019;64:5–17. https://doi.org/10.1177/0706743718773728 .
Sato K. Why is prepulse inhibition disrupted in schizophrenia? Med Hypotheses. 2020;143:109901. https://doi.org/10.1016/j.mehy.2020.109901 .
Nikiforuk A. Assessment of cognitive functions in animal models of schizophrenia. Pharmacol Rep. 2018;70:639–49. https://doi.org/10.1016/j.pharep.2018.01.009 .
Jones CA, Watson DJ, Fone KC. Animal models of schizophrenia. Br J Pharmacol. 2011;164:1162–94. https://doi.org/10.1111/j.1476-5381.2011.01386.x .
van den Buuse M. Modeling the positive symptoms of schizophrenia in genetically modified mice: pharmacology and methodology aspects. Schizophr Bull. 2010;36:246–70. https://doi.org/10.1093/schbul/sbp132 .
Blot K, Bai J, Otani S. The effect of non-competitive NMDA receptor antagonist MK-801 on neuronal activity in rodent prefrontal cortex: an animal model for cognitive symptoms of schizophrenia. J Physiol Paris. 2013;107:448–51. https://doi.org/10.1016/j.jphysparis.2013.04.003 .
Cadinu D, Grayson B, Podda G, Harte MK, Doostdar N, Neill JC. NMDA receptor antagonist rodent models for cognition in schizophrenia and identification of novel drug treatments, an update. Neuropharmacology. 2018;142:41–62. https://doi.org/10.1016/j.neuropharm.2017.11.045 .
Featherstone RE, Kapur S, Fletcher PJ. The amphetamine-induced sensitized state as a model of schizophrenia. Prog Neuropsychopharmacol Biol Psychiatry. 2007;31:1556–71. https://doi.org/10.1016/j.pnpbp.2007.08.025 .
Weidenauer A, Bauer M, Sauerzopf U, Bartova L, Praschak-Rieder N, Sitte HH, et al. Making sense of: sensitization in schizophrenia. Int J Neuropsychopharmacol. 2016;20:1–10. https://doi.org/10.1093/ijnp/pyw081 .
Peleg-Raibstein D, Knuesel I, Feldon J. Amphetamine sensitization in rats as an animal model of schizophrenia. Behav Brain Res. 2008;191:190–201. https://doi.org/10.1016/j.bbr.2008.03.037 .
Wearne TA, Mirzaei M, Franklin JL, Goodchild AK, Haynes PA, Cornish JL. Methamphetamine-induced sensitization is associated with alterations to the proteome of the prefrontal cortex: implications for the maintenance of psychotic disorders. J Proteome Res. 2015;14:397–410. https://doi.org/10.1021/pr500719f .
Abekawa T, Ito K, Nakagawa S, Nakato Y, Koyama T. Effects of aripiprazole and haloperidol on progression to schizophrenia-like behavioural abnormalities and apoptosis in rodents. Schizophr Res. 2011;125:77–87. https://doi.org/10.1016/j.schres.2010.08.011 .
Herrera AS, Casanova JP, Gatica RI, Escobar F, Fuentealba JA. Clozapine pre-treatment has a protracted hypolocomotor effect on the induction and expression of amphetamine sensitization. Prog Neuropsychopharmacol Biol Psychiatry. 2013;47:1–6. https://doi.org/10.1016/j.pnpbp.2013.07.023 .
Almey A, Arena L, Oliel J, Shams WM, Hafez N, Mancinelli C, et al. Interactions between estradiol and haloperidol on perseveration and reversal learning in amphetamine-sensitized female rats. Horm Behav. 2017;89:113–20. https://doi.org/10.1016/j.yhbeh.2016.12.010 .
Jaaro-Peled H. Gene models of schizophrenia: DISC1 mouse models. Prog Brain Res. 2009;179:75–86. https://doi.org/10.1016/S0079-6123(09)17909-8 .
Nani JV, Fonseca MC, Engi SA, Perillo MG, Dias CS, Gazarini ML, et al. Decreased nuclear distribution nudE-like 1 enzyme activity in an animal model with dysfunctional disrupted-in-schizophrenia 1 signaling featuring aberrant neurodevelopment and amphetamine-supersensitivity. J Psychopharmacol. 2020;34:467–77. https://doi.org/10.1177/0269881119897562 .
Nason MW Jr, Adhikari A, Bozinoski M, Gordon JA, Role LW. Disrupted activity in the hippocampal-accumbens circuit of type III neuregulin 1 mutant mice. Neuropsychopharmacology. 2011;36:488–96. https://doi.org/10.1038/npp.2010.180 .
Schneider S, Götz K, Birchmeier C, Schwegler H, Roskoden T. Neuregulin-1 mutant mice indicate motor and sensory deficits, indeed few references for schizophrenia endophenotype model. Behav Brain Res. 2017;322:177–85. https://doi.org/10.1016/j.bbr.2017.01.022 .
Tomiyama K, O’Tuathaigh CM, O’Sullivan GJ, Kinsella A, Lai D, Harvey RP, et al. Phenotype of spontaneous orofacial dyskinesia in neuregulin-1 ‘knockout’ mice. Prog Neuropsychopharmacol Biol Psychiatry. 2009;33:330–3. https://doi.org/10.1016/j.pnpbp.2008.12.010 .
Benedetti A, Molent C, Barcik W, Papaleo F. Social behavior in 16p11.2 and 22q11.2 copy number variations: insights from mice and humans. Genes Brain Behav. 2022;21:e12787. https://doi.org/10.1111/gbb.12787 .
Stark KL, Xu B, Bagchi A, Lai WS, Liu H, Hsu R, et al. Altered brain microRNA biogenesis contributes to phenotypic deficits in a 22q11-deletion mouse model. Nat Genet. 2008;40:751–60. https://doi.org/10.1038/ng.138 .
Horev G, Ellegood J, Lerch JP, Son YE, Muthuswamy L, Vogel H, et al. Dosage-dependent phenotypes in models of 16p11.2 lesions found in autism. Proc Natl Acad Sci USA. 2011;108:17076–81. https://doi.org/10.1073/pnas.1114042108 .
Kimber WL, Hsieh P, Hirotsune S, Yuva-Paylor L, Sutherland HF, Chen A, et al. Deletion of 150 kb in the minimal DiGeorge/velocardiofacial syndrome critical region in mouse. Hum Mol Genet. 1999;8:2229–37. https://doi.org/10.1093/hmg/8.12.2229 .
Estes ML, McAllister AK. Maternal immune activation: implications for neuropsychiatric disorders. Science. 2016;353:772–7. https://doi.org/10.1126/science.aag3194 .
Haddad FL, Patel SV, Schmid S. Maternal immune activation by poly I:C as a preclinical model for neurodevelopmental disorders: a focus on autism and schizophrenia. Neurosci Biobehav Rev. 2020;113:546–67. https://doi.org/10.1016/j.neubiorev.2020.04.012 .
Guerrin CGJ, Doorduin J, Sommer IE, de Vries EFJ. The dual hit hypothesis of schizophrenia: evidence from animal models. Neurosci Biobehav Rev. 2021;131:1150–68. https://doi.org/10.1016/j.neubiorev.2021.10.025 .
Uzuneser TC, Speidel J, Kogias G, Wang AL, de Souza Silva MA, Huston JP, et al. Disrupted-in-schizophrenia 1 (DISC1) overexpression and juvenile immune activation cause sex-specific schizophrenia-related psychopathology in rats. Front Psychiatry. 2019;10:222. https://doi.org/10.3389/fpsyt.2019.00222 .
Feigenson KA, Kusnecov AW, Silverstein SM. Inflammation and the two-hit hypothesis of schizophrenia. Neurosci Biobehav Rev. 2014;38:72–93. https://doi.org/10.1016/j.neubiorev.2013.11.006 .
Giovanoli S, Engler H, Engler A, Richetto J, Feldon J, Riva MA, et al. Preventive effects of minocycline in a neurodevelopmental two-hit model with relevance to schizophrenia. Transl Psychiatry. 2016;6:e772. https://doi.org/10.1038/tp.2016.38 .
Liu R, Meng X, Yu X, Wang G, Dong Z, Zhou Z, et al. From 2D to 3D co-culture systems: a review of co-culture models to study the neural cells interaction. Int J Mol Sci. 2022;23:13116. https://doi.org/10.3390/ijms232113116 .
Dixon TA, Muotri AR. Advancing preclinical models of psychiatric disorders with human brain organoid cultures. Mol Psychiatry. 2023;28:83–95. https://doi.org/10.1038/s41380-022-01708-2 .
Mateos-Aparicio P, Bello SA, Rodríguez-Moreno A. Challenges in physiological phenotyping of hiPSC-derived neurons: from 2D cultures to 3D brain organoids. Front Cell Dev Biol. 2020;8:797. https://doi.org/10.3389/fcell.2020.00797 .
Ardhanareeswaran K, Mariani J, Coppola G, Abyzov A, Vaccarino FM. Human induced pluripotent stem cells for modelling neurodevelopmental disorders. Nat Rev Neurol. 2017;13:265–78. https://doi.org/10.1038/nrneurol.2017.45 .
Volpato V, Webber C. Addressing variability in iPSC-derived models of human disease: guidelines to promote reproducibility. Dis Model Mech. 2020;13:dmm042317. https://doi.org/10.1242/dmm.042317 .
Birey F, Andersen J, Makinson CD, Islam S, Wei W, Huber N, et al. Assembly of functionally integrated human forebrain spheroids. Nature. 2017;545:54–9. https://doi.org/10.1038/nature22330 .
Pham MT, Pollock KM, Rose MD, Cary WA, Stewart HR, Zhou P, et al. Generation of human vascularized brain organoids. Neuroreport. 2018;29:588–93. https://doi.org/10.1097/WNR.0000000000001014 .
Sloan SA, Andersen J, Pașca AM, Birey F, Pașca SP. Generation and assembly of human brain region-specific three-dimensional cultures. Nat Protoc. 2018;13:2062–85. https://doi.org/10.1038/s41596-018-0032-7 .
Jeziorski J, Brandt R, Evans JH, Campana W, Kalichman M, Thompson E, et al. Brain organoids, consciousness, ethics and moral status. Semin Cell Dev Biol. 2023;144:97–102. https://doi.org/10.1016/j.semcdb.2022.03.020 .
Dwyer DS. Crossing the worm-brain barrier by using Caenorhabditis elegans to explore fundamentals of human psychiatric illness. Mol Neuropsychiatry. 2018;3:170–9. https://doi.org/10.1159/000485423 .
van Alphen B, van Swinderen B. Drosophila strategies to study psychiatric disorders. Brain Res Bull. 2013;92:1–11. https://doi.org/10.1016/j.brainresbull.2011.09.007 .
Cannarozzi G, Schneider A, Gonnet G. A phylogenomic study of human, dog, and mouse. PLoS Comput Biol. 2007;3:e2. https://doi.org/10.1371/journal.pcbi.0030002 .
Forrest AD, Coto CA, Siegel SJ. Animal models of psychosis: current state and future directions. Curr Behav Neurosci Rep. 2014;1:100–16. https://doi.org/10.1007/s40473-014-0013-2 .
Białoń M, Wąsik A. Advantages and limitations of animal schizophrenia models. Int J Mol Sci. 2022;23:5968. https://doi.org/10.3390/ijms23115968 .
Liang SG, Greenwood TA. The impact of clinical heterogeneity in schizophrenia on genomic analyses. Schizophr Res. 2015;161:490–5. https://doi.org/10.1016/j.schres.2014.11.019 .
Chandra A, Miller BJ, Goldsmith DR. Predictors of successful anti-inflammatory drug trials in patients with schizophrenia: a meta-regression and critical commentary. Brain Behav Immun. 2023;114:154–62. https://doi.org/10.1016/j.bbi.2023.08.001 .
Khandaker GM, Cousins L, Deakin J, Lennox BR, Yolken R, Jones PB. Inflammation and immunity in schizophrenia: implications for pathophysiology and treatment. Lancet Psychiatry. 2015;2:258–70. https://doi.org/10.1016/S2215-0366(14)00122-9 .
Borbély E, Scheich B, Helyes Z. Neuropeptides in learning and memory. Neuropeptides. 2013;47:439–50. https://doi.org/10.1016/j.npep.2013.10.012 .
Perez-Bonilla P, Santiago-Colon K, Leinninger GM. Lateral hypothalamic area neuropeptides modulate ventral tegmental area dopamine neurons and feeding. Physiol Behav. 2020;223:112986. https://doi.org/10.1016/j.physbeh.2020.112986 .
Griebel G. Neuropeptide receptor ligands for the treatment of schizophrenia: focus on neurotensin and tachykinins. Curr Pharm Des. 2015;21:3807–12. https://doi.org/10.2174/1381612821666150605105859 .
LaCrosse AL, Olive MF. Neuropeptide systems and schizophrenia. CNS Neurol Disord Drug Targets. 2013;12:619–32. https://doi.org/10.2174/1871527311312050010 .
Harrow M, Jobe TH, Tong L. Long-term effectiveness of antipsychotics. Psychol Med. 2023;53:1129–33. https://doi.org/10.1017/S0033291721001732 .
Telias M. Neural differentiation protocols: how to choose the correct approach. Neural Regen Res. 2023;18:1273–4. https://doi.org/10.4103/1673-5374.360171 .
Martino M, Magioncalda P. A three-dimensional model of neural activity and phenomenal-behavioral patterns. Mol Psychiatry. 2024. https://doi.org/10.1038/s41380-023-02356-w .
Howes OD, Onwordi EC. The synaptic hypothesis of schizophrenia version III: a master mechanism. Mol Psychiatry. 2023;28:1843–56. https://doi.org/10.1038/s41380-023-02043-w .
Ye H, Fussenegger M. Optogenetic Medicine: Synthetic Therapeutic Solutions Precision-Guided by Light. Cold Spring Harb Perspect Med. 2019;9:a034371. https://doi.org/10.1101/cshperspect.a034371 .
Qiao C, Li D, Guo Y, Liu C, Jiang T, Dai Q, et al. Evaluation and development of deep neural networks for image super-resolution in optical microscopy. Nat Methods. 2021;18:194–202. https://doi.org/10.1038/s41592-020-01048-5 .
Lopez-Lengowski K, Kathuria A, Gerlovin K, Karmacharya R. Co-culturing microglia and cortical neurons differentiated from human induced pluripotent stem cells. J Vis Exp. 2021. https://doi.org/10.3791/62480 .
Tan SY, Feng X, Cheng LKW, Wu AR. Vascularized human brain organoid on-chip. Lab Chip. 2023;23:2693–709. https://doi.org/10.1039/d2lc01109c .
Cheng C, Chen W, Jin H, Chen X. A Review of Single-Cell RNA-Seq Annotation, Integration, and Cell-Cell Communication. Cells. 2023;12:1970. https://doi.org/10.3390/cells12151970 .
Wu Y, Zhang CY, Wang L, Li Y, Xiao X. Genetic insights of schizophrenia via single cell RNA-sequencing analyses. Schizophr Bull. 2023;49:914–22. https://doi.org/10.1093/schbul/sbad002 .
Chilla GS, Yeow LY, Chew QH, Sim K, Prakash KNB. Machine learning classification of schizophrenia patients and healthy controls using diverse neuroanatomical markers and Ensemble methods. Sci Rep. 2022;12:2755. https://doi.org/10.1038/s41598-022-06651-4 .
Santos Febles E, Ontivero Ortega M, Valdés Sosa M, Sahli H. Machine Learning Techniques for the Diagnosis of Schizophrenia Based on Event-Related Potentials. Front Neuroinform. 2022;16:893788. https://doi.org/10.3389/fninf.2022.893788 .
Montazeri M, Montazeri M, Bahaadinbeigy K, Montazeri M, Afraz A. Application of machine learning methods in predicting schizophrenia and bipolar disorders: a systematic review. Health Sci Rep. 2022;6:e962. https://doi.org/10.1002/hsr2.962 .
Hauser TU, Skvortsova V, De Choudhury M, Koutsouleris N. The promise of a model-based psychiatry: building computational models of mental ill health. Lancet Digit Health. 2022;4:e816–28. https://doi.org/10.1016/S2589-7500(22)00152-2 .
Lally J, Watkins R, Nash S, Shetty H, Gardner-Sood P, Smith S, et al. The representativeness of participants with severe mental illness in a psychosocial clinical trial. Front Psychiatry. 2018;9:654. https://doi.org/10.3389/fpsyt.2018.00654 .
Huxley P, Krayer A, Poole R, Prendergast L, Aryal S, Warner R. Schizophrenia outcomes in the 21st century: a systematic review. Brain Behav. 2021;11:e02172. https://doi.org/10.1002/brb3.2172 .
Levy N. The use of animal as models: ethical considerations. Int J Stroke. 2012;7:440–2. https://doi.org/10.1111/j.1747-4949.2012.00772.x .
Qureshi MNI, Oh J, Cho D, Jo HJ, Lee B. Multimodal discrimination of schizophrenia using hybrid weighted feature concatenation of brain functional connectivity and anatomical features with an extreme learning machine. Front Neuroinform. 2017;11:59. https://doi.org/10.3389/fninf.2017.00059 .
Download references
Acknowledgements
All figures were created with BioRender.com. We would like to thank the anonymous reviewers for their constructive and helpful comments, which have significantly improved the quality and clarity of our manuscript.
This work was supported by FAPESP (Fundação de Amparo à Pesquisa do Estado de São Paulo) [Nos. 2022/00527-8; 2020/01107-7; 2019/13112-8; 2019/08287-3; 2017/02413-1; 2014/50891-1 (INCT 2014 - TRANSLATIONAL MEDICINE)], CAPES and CNPq. MAFH is also a recipient of a fellowship from CNPq [39337/2016-0]. JVN is recipient of a Fellowship from FAPESP Nos. 2022/03297-3 and 2019/09207-3).
Author information
Authors and affiliations.
Department of Pharmacology, Escola Paulista de Medicina (EPM), Universidade Federal de São Paulo (UNIFESP), São Paulo, SP, Brazil
João V. Nani & Mirian A. F. Hayashi
National Institute for Translational Medicine (INCT-TM, CNPq/FAPESP/CAPES), Ribeirão Preto, Brazil
Department of Pediatrics and Department of Molecular and Cellular Medicine, University of California, San Diego, La Jolla, CA, USA
Alysson R. Muotri
You can also search for this author in PubMed Google Scholar
Contributions
JVN was responsible for the literature review, writing, and figure preparation. JVN, MAFH, and ARM contributed equally to the conceptualization, editing, and revision of the paper.
Corresponding authors
Correspondence to João V. Nani or Mirian A. F. Hayashi .
Ethics declarations
Competing interests.
ARM is a co-founder and has an equity interest in TISMOO, a company dedicated to genetic analysis and human brain organogenesis, focusing on therapeutic applications customized for autism spectrum disorders and other neurological disorders origin genetics. The terms of this arrangement have been reviewed and approved by the University of California, San Diego, following its conflict-of-interest policies.
Additional information
Publisher’s note Springer Nature remains neutral with regard to jurisdictional claims in published maps and institutional affiliations.
Rights and permissions
Springer Nature or its licensor (e.g. a society or other partner) holds exclusive rights to this article under a publishing agreement with the author(s) or other rightsholder(s); author self-archiving of the accepted manuscript version of this article is solely governed by the terms of such publishing agreement and applicable law.
Reprints and permissions
About this article
Cite this article.
Nani, J.V., Muotri, A.R. & Hayashi, M.A.F. Peering into the mind: unraveling schizophrenia’s secrets using models. Mol Psychiatry (2024). https://doi.org/10.1038/s41380-024-02728-w
Download citation
Received : 23 August 2023
Revised : 21 August 2024
Accepted : 27 August 2024
Published : 08 September 2024
DOI : https://doi.org/10.1038/s41380-024-02728-w
Share this article
Anyone you share the following link with will be able to read this content:
Sorry, a shareable link is not currently available for this article.
Provided by the Springer Nature SharedIt content-sharing initiative
Quick links
- Explore articles by subject
- Guide to authors
- Editorial policies


IMAGES
COMMENTS
Transmission. The role of the Huanan Seafood Wholesale Market in propagating disease is unclear. Many initial COVID-19 cases were linked to this market suggesting that SARS-CoV-2 was transmitted from animals to humans .However, a genomic study has provided evidence that the virus was introduced from another, yet unknown location, into the market where it spread more rapidly, although human-to ...
Abstract. In early December 2019, an outbreak of coronavirus disease 2019 (COVID-19), caused by a novel severe acute respiratory syndrome coronavirus 2 (SARS-CoV-2), occurred in Wuhan City, Hubei Province, China. On January 30, 2020 the World Health Organization declared the outbreak as a Public Health Emergency of International Concern.
The spread of the "Severe Acute Respiratory Coronavirus 2" (SARS-CoV-2), the causal agent of COVID-19, was characterized as a pandemic by the World Health Organization (WHO) in March 2020 and has triggered an international public health emergency [].The numbers of confirmed cases and deaths due to COVID-19 are rapidly escalating, counting in millions [], causing massive economic strain ...
The Origins of Covid-19 — Why It Matters (and ...
A Review of Coronavirus Disease-2019 (COVID-19) - PMC
These papers highlight valuable research into the biology of coronavirus infection, its detection, treatment as well as into vaccine development and the epidemiology of the disease. Browse all Top ...
Long-term effectiveness of COVID-19 vaccines against ...
Coronavirus disease (COVID-19): a scoping review - PMC
COVID-19 impact on research, lessons learned from ...
Introduction. The COVID-19 pandemic upended many normal practices around the conduct of research and development (R&D); the extent of disruption is revealed across measures of scientific research output [1-3].This paper revisits the extent to which patterns of international collaboration in coronavirus research during the COVID-19 pandemic depart from 'normal' times.
Global research on coronavirus disease (COVID-19)
Download MP3. In the final Coronapod of 2020, we dive into the scientific literature to reflect on the COVID-19 pandemic. Researchers have discovered so much about SARS-CoV-2 - information that ...
Coronavirus (Covid-19) | NEJM
There have been around 96,000 reported cases of coronavirus disease 2019 (COVID-2019) and 3300 reported deaths to date (05/03/2020). The disease is transmitted by inhalation or contact with infected droplets and the incubation period ranges from 2 to 14 d. The symptoms are usually fever, cough, sore throat, breathlessness, fatigue, malaise ...
Community‐based studies in five countries show consistent strong benefits from early rollouts of COVID‐19 vaccines. By the beginning of June 2021, almost 11% of the world's population had received at least one dose of a coronavirus disease 2019 (COVID‐19) vaccine. 1 This represents an extraordinary scientific and logistic achievement — in 18 months, researchers, manufacturers and ...
The COVID-19 outbreak has posed an unprecedented challenge to humanity and science. On the one side, public and private incentives have been put in place to promptly allocate resources toward research areas strictly related to the COVID-19 emergency. However, research in many fields not directly related to the pandemic has been displaced. In this paper, we assess the impact of COVID-19 on ...
More than 50 long-term effects of COVID-19: a systematic ...
Coronavirus (COVID-19) Research Highlights
Recent studies have attempted to analyze the changes in self-rated health (SRH) during the coronavirus disease 2019 (COVID-19) pandemic. However, the results have been inconsistent. Notably, SRH is subjective, and responses may vary across and within countries because of sociocultural differences. Thus, we aimed to examine whether the interaction effects between the COVID-19 pandemic and ...
Ferroptosis is a new type of programmed cell death. Although ferroptosis has been studied in various aspects, there has been no visual analysis of ferroptosis in coronavirus disease 2019 (COVID-19) to date. It is still a global health concern of the COVID-19 pandemic worldwide, three years after its outbreak. Yet the emergence of the mutant strain Omicron has caused a fourth wave of infections ...
This paper is one of the few to examine how people who have lived through both COVID-19 and AIDS understand these pandemics in relation to each other. Data were collected in Uganda, and we found that the AIDS epidemic proved to be a key reference point for people in explaining why COVID-19 was perceived as so worrisome.
"Other COVID-19 antibodies have been rendered ineffective as SARS-CoV-2 has evolved over the past several years," says Dr. Ippolito, an Associate Professor. "Our new study suggests the virus is less likely to escape this treatment because SC27 targets and attaches to multiple parts of the virus's spike protein, including sections that ...
Research on Covid-19 had surged in the early days with an unprecedented surge in the publications on that specific topic. ... as the COVID-19 disease is primarily a respiratory disease. ... Salimi A., Diab N., Smith L. Bibliometric analysis of early COVID-19 research: the top 50 cited papers. Inf Disp. 2020; 13:1-5. doi: 10.1177 ...
Characteristics of SARS-CoV-2 and COVID-19
The COVID-19 pandemic was triggered by the SARS-CoV-2 virus which caused multiple ill-health conditions in infected individuals. There were many cases that culminated in death. Chest X-ray images became a proven method for spotting thoracic ailments. The resultant availability of huge public datasets of chest X-ray images has great potential in deep learning for lung ailment detection. This ...
Almas Eftekhari, a 40-year-old Lyme disease advocate, shrugged off her first, super-mild bout of Covid in August of 2023. But a couple of months later, she crashed with symptoms that moved around ...
COVID-19 pandemic has severely impacted the crude, stock market, gold and metals and almost all areas of the global market [1]. Large research laboratories and corporate houses are working with a high speed to develop medicines and vaccines for the prevention and treatment of this dreaded disease. To deal with these current health management ...
Long COVID: major findings, mechanisms and ...
The eleventh of these papers sought to estimate the seroprevalence of COVID-19 in a cohort of general practitioners working in Catania, Italy, after the first wave of COVID-19, which was low (3%) . The last paper was a survey by the WHO Special Programme for Research and Training in Tropical Disease (TDR) and collaborators of health workers ...
Incorporating individuals with varying genetic backgrounds, disease subtypes, and clinical characteristics captures SCZ's heterogeneity, unveiling factors influencing disease presentation and ...