- Research Process
- Manuscript Preparation
- Manuscript Review
- Publication Process
- Publication Recognition
- Language Editing Services
- Translation Services
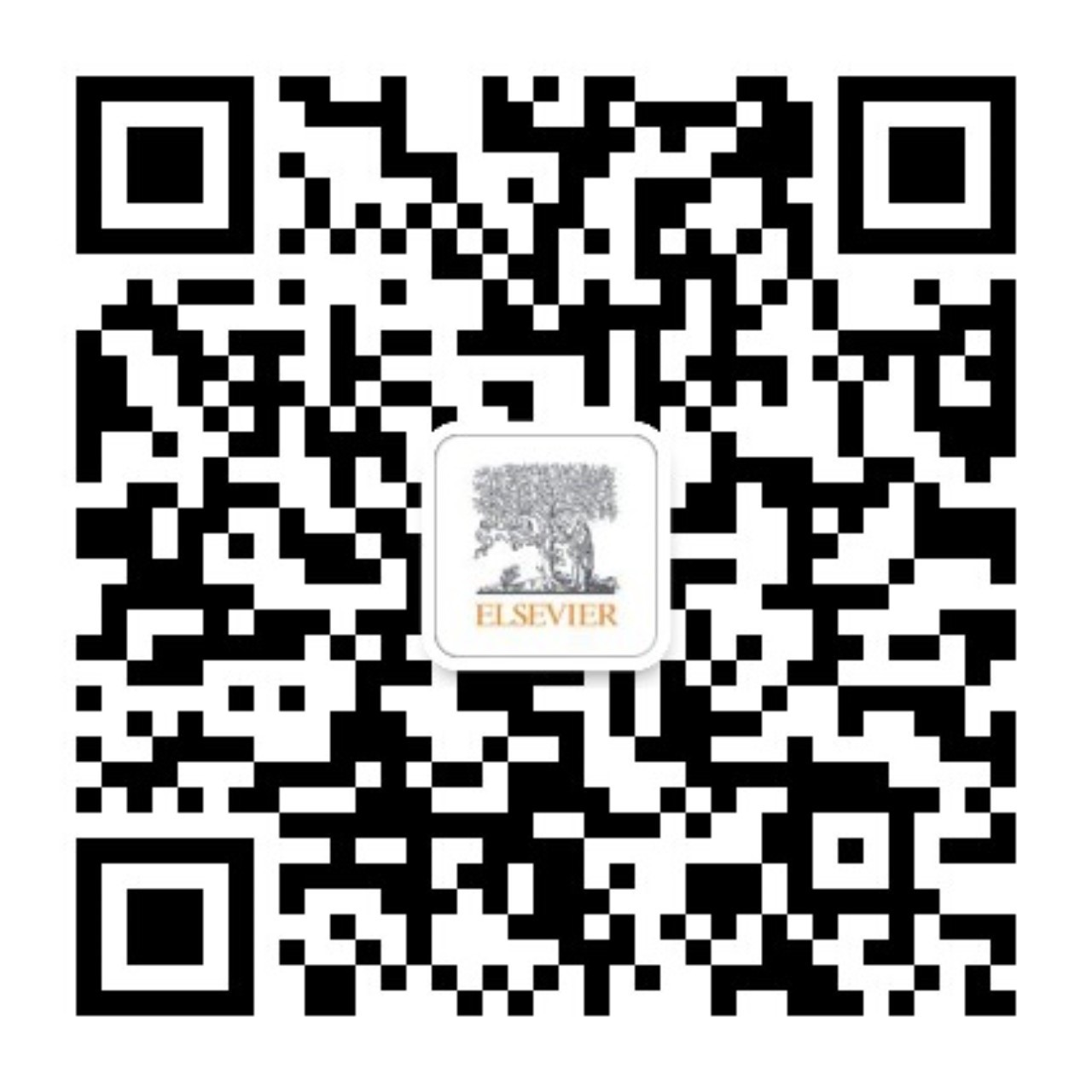

Step-by-Step Guide: How to Craft a Strong Research Hypothesis
- 4 minute read
- 418.1K views
Table of Contents
A research hypothesis is a concise statement about the expected result of an experiment or project. In many ways, a research hypothesis represents the starting point for a scientific endeavor, as it establishes a tentative assumption that is eventually substantiated or falsified, ultimately improving our certainty about the subject investigated.
To help you with this and ease the process, in this article, we discuss the purpose of research hypotheses and list the most essential qualities of a compelling hypothesis. Let’s find out!
How to Craft a Research Hypothesis
Crafting a research hypothesis begins with a comprehensive literature review to identify a knowledge gap in your field. Once you find a question or problem, come up with a possible answer or explanation, which becomes your hypothesis. Now think about the specific methods of experimentation that can prove or disprove the hypothesis, which ultimately lead to the results of the study.
Enlisted below are some standard formats in which you can formulate a hypothesis¹ :
- A hypothesis can use the if/then format when it seeks to explore the correlation between two variables in a study primarily.
Example: If administered drug X, then patients will experience reduced fatigue from cancer treatment.
- A hypothesis can adopt when X/then Y format when it primarily aims to expose a connection between two variables
Example: When workers spend a significant portion of their waking hours in sedentary work , then they experience a greater frequency of digestive problems.
- A hypothesis can also take the form of a direct statement.
Example: Drug X and drug Y reduce the risk of cognitive decline through the same chemical pathways
What are the Features of an Effective Hypothesis?
Hypotheses in research need to satisfy specific criteria to be considered scientifically rigorous. Here are the most notable qualities of a strong hypothesis:
- Testability: Ensure the hypothesis allows you to work towards observable and testable results.
- Brevity and objectivity: Present your hypothesis as a brief statement and avoid wordiness.
- Clarity and Relevance: The hypothesis should reflect a clear idea of what we know and what we expect to find out about a phenomenon and address the significant knowledge gap relevant to a field of study.
Understanding Null and Alternative Hypotheses in Research
There are two types of hypotheses used commonly in research that aid statistical analyses. These are known as the null hypothesis and the alternative hypothesis . A null hypothesis is a statement assumed to be factual in the initial phase of the study.
For example, if a researcher is testing the efficacy of a new drug, then the null hypothesis will posit that the drug has no benefits compared to an inactive control or placebo . Suppose the data collected through a drug trial leads a researcher to reject the null hypothesis. In that case, it is considered to substantiate the alternative hypothesis in the above example, that the new drug provides benefits compared to the placebo.
Let’s take a closer look at the null hypothesis and alternative hypothesis with two more examples:
Null Hypothesis:
The rate of decline in the number of species in habitat X in the last year is the same as in the last 100 years when controlled for all factors except the recent wildfires.
In the next experiment, the researcher will experimentally reject this null hypothesis in order to confirm the following alternative hypothesis :
The rate of decline in the number of species in habitat X in the last year is different from the rate of decline in the last 100 years when controlled for all factors other than the recent wildfires.
In the pair of null and alternative hypotheses stated above, a statistical comparison of the rate of species decline over a century and the preceding year will help the research experimentally test the null hypothesis, helping to draw scientifically valid conclusions about two factors—wildfires and species decline.
We also recommend that researchers pay attention to contextual echoes and connections when writing research hypotheses. Research hypotheses are often closely linked to the introduction ² , such as the context of the study, and can similarly influence the reader’s judgment of the relevance and validity of the research hypothesis.
Seasoned experts, such as professionals at Elsevier Language Services, guide authors on how to best embed a hypothesis within an article so that it communicates relevance and credibility. Contact us if you want help in ensuring readers find your hypothesis robust and unbiased.
References
- Hypotheses – The University Writing Center. (n.d.). https://writingcenter.tamu.edu/writing-speaking-guides/hypotheses
- Shaping the research question and hypothesis. (n.d.). Students. https://students.unimelb.edu.au/academic-skills/graduate-research-services/writing-thesis-sections-part-2/shaping-the-research-question-and-hypothesis
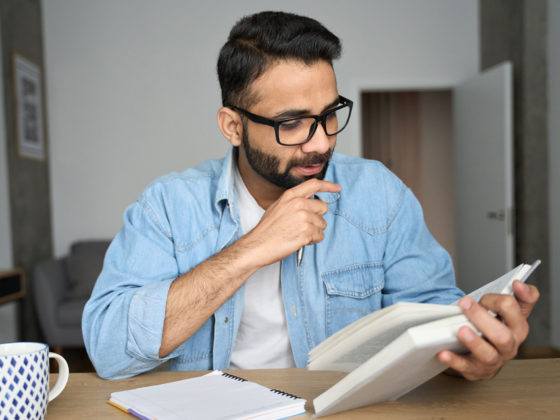
Systematic Literature Review or Literature Review?
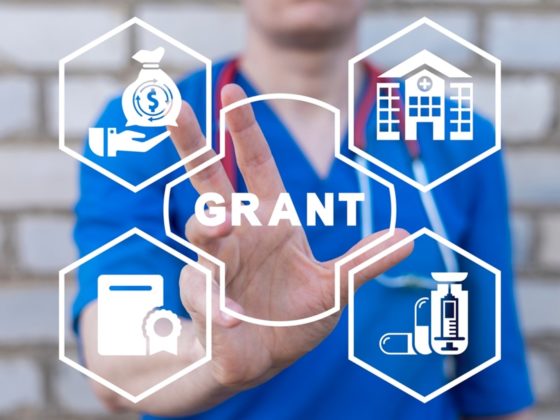
How to Write an Effective Problem Statement for Your Research Paper
You may also like.
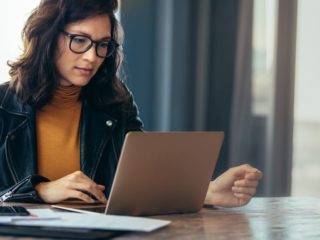
Submission 101: What format should be used for academic papers?
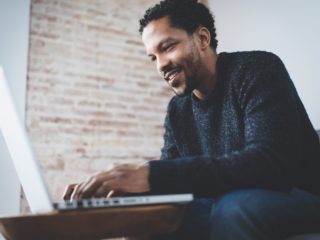

Page-Turner Articles are More Than Just Good Arguments: Be Mindful of Tone and Structure!
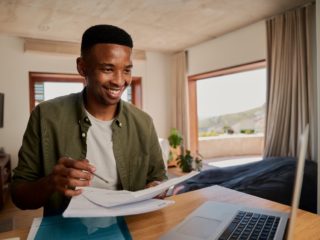
A Must-see for Researchers! How to Ensure Inclusivity in Your Scientific Writing
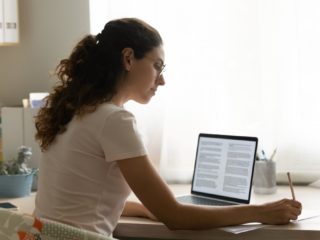
Make Hook, Line, and Sinker: The Art of Crafting Engaging Introductions
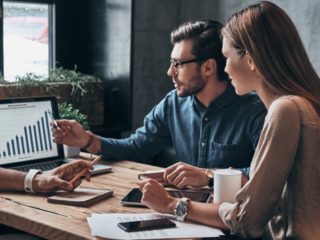
Can Describing Study Limitations Improve the Quality of Your Paper?
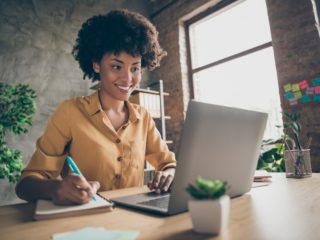
A Guide to Crafting Shorter, Impactful Sentences in Academic Writing
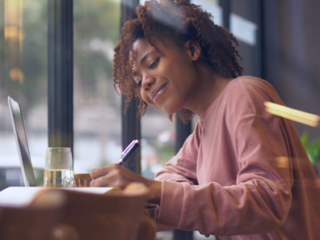
6 Steps to Write an Excellent Discussion in Your Manuscript
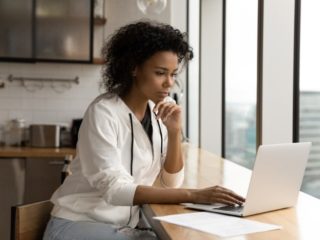
How to Write Clear and Crisp Civil Engineering Papers? Here are 5 Key Tips to Consider
Input your search keywords and press Enter.

IMAGES
VIDEO