- Skip to secondary menu
- Skip to main content
- Skip to primary sidebar
Statistics By Jim
Making statistics intuitive

Null Hypothesis: Definition, Rejecting & Examples
By Jim Frost 6 Comments
What is a Null Hypothesis?
The null hypothesis in statistics states that there is no difference between groups or no relationship between variables. It is one of two mutually exclusive hypotheses about a population in a hypothesis test.

- Null Hypothesis H 0 : No effect exists in the population.
- Alternative Hypothesis H A : The effect exists in the population.
In every study or experiment, researchers assess an effect or relationship. This effect can be the effectiveness of a new drug, building material, or other intervention that has benefits. There is a benefit or connection that the researchers hope to identify. Unfortunately, no effect may exist. In statistics, we call this lack of an effect the null hypothesis. Researchers assume that this notion of no effect is correct until they have enough evidence to suggest otherwise, similar to how a trial presumes innocence.
In this context, the analysts don’t necessarily believe the null hypothesis is correct. In fact, they typically want to reject it because that leads to more exciting finds about an effect or relationship. The new vaccine works!
You can think of it as the default theory that requires sufficiently strong evidence to reject. Like a prosecutor, researchers must collect sufficient evidence to overturn the presumption of no effect. Investigators must work hard to set up a study and a data collection system to obtain evidence that can reject the null hypothesis.
Related post : What is an Effect in Statistics?
Null Hypothesis Examples
Null hypotheses start as research questions that the investigator rephrases as a statement indicating there is no effect or relationship.
Does the vaccine prevent infections? | The vaccine does not affect the infection rate. |
Does the new additive increase product strength? | The additive does not affect mean product strength. |
Does the exercise intervention increase bone mineral density? | The intervention does not affect bone mineral density. |
As screen time increases, does test performance decrease? | There is no relationship between screen time and test performance. |
After reading these examples, you might think they’re a bit boring and pointless. However, the key is to remember that the null hypothesis defines the condition that the researchers need to discredit before suggesting an effect exists.
Let’s see how you reject the null hypothesis and get to those more exciting findings!
When to Reject the Null Hypothesis
So, you want to reject the null hypothesis, but how and when can you do that? To start, you’ll need to perform a statistical test on your data. The following is an overview of performing a study that uses a hypothesis test.
The first step is to devise a research question and the appropriate null hypothesis. After that, the investigators need to formulate an experimental design and data collection procedures that will allow them to gather data that can answer the research question. Then they collect the data. For more information about designing a scientific study that uses statistics, read my post 5 Steps for Conducting Studies with Statistics .
After data collection is complete, statistics and hypothesis testing enter the picture. Hypothesis testing takes your sample data and evaluates how consistent they are with the null hypothesis. The p-value is a crucial part of the statistical results because it quantifies how strongly the sample data contradict the null hypothesis.
When the sample data provide sufficient evidence, you can reject the null hypothesis. In a hypothesis test, this process involves comparing the p-value to your significance level .
Rejecting the Null Hypothesis
Reject the null hypothesis when the p-value is less than or equal to your significance level. Your sample data favor the alternative hypothesis, which suggests that the effect exists in the population. For a mnemonic device, remember—when the p-value is low, the null must go!
When you can reject the null hypothesis, your results are statistically significant. Learn more about Statistical Significance: Definition & Meaning .
Failing to Reject the Null Hypothesis
Conversely, when the p-value is greater than your significance level, you fail to reject the null hypothesis. The sample data provides insufficient data to conclude that the effect exists in the population. When the p-value is high, the null must fly!
Note that failing to reject the null is not the same as proving it. For more information about the difference, read my post about Failing to Reject the Null .
That’s a very general look at the process. But I hope you can see how the path to more exciting findings depends on being able to rule out the less exciting null hypothesis that states there’s nothing to see here!
Let’s move on to learning how to write the null hypothesis for different types of effects, relationships, and tests.
Related posts : How Hypothesis Tests Work and Interpreting P-values
How to Write a Null Hypothesis
The null hypothesis varies by the type of statistic and hypothesis test. Remember that inferential statistics use samples to draw conclusions about populations. Consequently, when you write a null hypothesis, it must make a claim about the relevant population parameter . Further, that claim usually indicates that the effect does not exist in the population. Below are typical examples of writing a null hypothesis for various parameters and hypothesis tests.
Related posts : Descriptive vs. Inferential Statistics and Populations, Parameters, and Samples in Inferential Statistics
Group Means
T-tests and ANOVA assess the differences between group means. For these tests, the null hypothesis states that there is no difference between group means in the population. In other words, the experimental conditions that define the groups do not affect the mean outcome. Mu (µ) is the population parameter for the mean, and you’ll need to include it in the statement for this type of study.
For example, an experiment compares the mean bone density changes for a new osteoporosis medication. The control group does not receive the medicine, while the treatment group does. The null states that the mean bone density changes for the control and treatment groups are equal.
- Null Hypothesis H 0 : Group means are equal in the population: µ 1 = µ 2 , or µ 1 – µ 2 = 0
- Alternative Hypothesis H A : Group means are not equal in the population: µ 1 ≠ µ 2 , or µ 1 – µ 2 ≠ 0.
Group Proportions
Proportions tests assess the differences between group proportions. For these tests, the null hypothesis states that there is no difference between group proportions. Again, the experimental conditions did not affect the proportion of events in the groups. P is the population proportion parameter that you’ll need to include.
For example, a vaccine experiment compares the infection rate in the treatment group to the control group. The treatment group receives the vaccine, while the control group does not. The null states that the infection rates for the control and treatment groups are equal.
- Null Hypothesis H 0 : Group proportions are equal in the population: p 1 = p 2 .
- Alternative Hypothesis H A : Group proportions are not equal in the population: p 1 ≠ p 2 .
Correlation and Regression Coefficients
Some studies assess the relationship between two continuous variables rather than differences between groups.
In these studies, analysts often use either correlation or regression analysis . For these tests, the null states that there is no relationship between the variables. Specifically, it says that the correlation or regression coefficient is zero. As one variable increases, there is no tendency for the other variable to increase or decrease. Rho (ρ) is the population correlation parameter and beta (β) is the regression coefficient parameter.
For example, a study assesses the relationship between screen time and test performance. The null states that there is no correlation between this pair of variables. As screen time increases, test performance does not tend to increase or decrease.
- Null Hypothesis H 0 : The correlation in the population is zero: ρ = 0.
- Alternative Hypothesis H A : The correlation in the population is not zero: ρ ≠ 0.
For all these cases, the analysts define the hypotheses before the study. After collecting the data, they perform a hypothesis test to determine whether they can reject the null hypothesis.
The preceding examples are all for two-tailed hypothesis tests. To learn about one-tailed tests and how to write a null hypothesis for them, read my post One-Tailed vs. Two-Tailed Tests .
Related post : Understanding Correlation
Neyman, J; Pearson, E. S. (January 1, 1933). On the Problem of the most Efficient Tests of Statistical Hypotheses . Philosophical Transactions of the Royal Society A . 231 (694–706): 289–337.
Share this:

Reader Interactions
January 11, 2024 at 2:57 pm
Thanks for the reply.
January 10, 2024 at 1:23 pm
Hi Jim, In your comment you state that equivalence test null and alternate hypotheses are reversed. For hypothesis tests of data fits to a probability distribution, the null hypothesis is that the probability distribution fits the data. Is this correct?
January 10, 2024 at 2:15 pm
Those two separate things, equivalence testing and normality tests. But, yes, you’re correct for both.
Hypotheses are switched for equivalence testing. You need to “work” (i.e., collect a large sample of good quality data) to be able to reject the null that the groups are different to be able to conclude they’re the same.
With typical hypothesis tests, if you have low quality data and a low sample size, you’ll fail to reject the null that they’re the same, concluding they’re equivalent. But that’s more a statement about the low quality and small sample size than anything to do with the groups being equal.
So, equivalence testing make you work to obtain a finding that the groups are the same (at least within some amount you define as a trivial difference).
For normality testing, and other distribution tests, the null states that the data follow the distribution (normal or whatever). If you reject the null, you have sufficient evidence to conclude that your sample data don’t follow the probability distribution. That’s a rare case where you hope to fail to reject the null. And it suffers from the problem I describe above where you might fail to reject the null simply because you have a small sample size. In that case, you’d conclude the data follow the probability distribution but it’s more that you don’t have enough data for the test to register the deviation. In this scenario, if you had a larger sample size, you’d reject the null and conclude it doesn’t follow that distribution.
I don’t know of any equivalence testing type approach for distribution fit tests where you’d need to work to show the data follow a distribution, although I haven’t looked for one either!
February 20, 2022 at 9:26 pm
Is a null hypothesis regularly (always) stated in the negative? “there is no” or “does not”
February 23, 2022 at 9:21 pm
Typically, the null hypothesis includes an equal sign. The null hypothesis states that the population parameter equals a particular value. That value is usually one that represents no effect. In the case of a one-sided hypothesis test, the null still contains an equal sign but it’s “greater than or equal to” or “less than or equal to.” If you wanted to translate the null hypothesis from its native mathematical expression, you could use the expression “there is no effect.” But the mathematical form more specifically states what it’s testing.
It’s the alternative hypothesis that typically contains does not equal.
There are some exceptions. For example, in an equivalence test where the researchers want to show that two things are equal, the null hypothesis states that they’re not equal.
In short, the null hypothesis states the condition that the researchers hope to reject. They need to work hard to set up an experiment and data collection that’ll gather enough evidence to be able to reject the null condition.
February 15, 2022 at 9:32 am
Dear sir I always read your notes on Research methods.. Kindly tell is there any available Book on all these..wonderfull Urgent
Comments and Questions Cancel reply
Have a thesis expert improve your writing
Check your thesis for plagiarism in 10 minutes, generate your apa citations for free.
- Knowledge Base
- Null and Alternative Hypotheses | Definitions & Examples
Null and Alternative Hypotheses | Definitions & Examples
Published on 5 October 2022 by Shaun Turney . Revised on 6 December 2022.
The null and alternative hypotheses are two competing claims that researchers weigh evidence for and against using a statistical test :
- Null hypothesis (H 0 ): There’s no effect in the population .
- Alternative hypothesis (H A ): There’s an effect in the population.
The effect is usually the effect of the independent variable on the dependent variable .
Table of contents
Answering your research question with hypotheses, what is a null hypothesis, what is an alternative hypothesis, differences between null and alternative hypotheses, how to write null and alternative hypotheses, frequently asked questions about null and alternative hypotheses.
The null and alternative hypotheses offer competing answers to your research question . When the research question asks “Does the independent variable affect the dependent variable?”, the null hypothesis (H 0 ) answers “No, there’s no effect in the population.” On the other hand, the alternative hypothesis (H A ) answers “Yes, there is an effect in the population.”
The null and alternative are always claims about the population. That’s because the goal of hypothesis testing is to make inferences about a population based on a sample . Often, we infer whether there’s an effect in the population by looking at differences between groups or relationships between variables in the sample.
You can use a statistical test to decide whether the evidence favors the null or alternative hypothesis. Each type of statistical test comes with a specific way of phrasing the null and alternative hypothesis. However, the hypotheses can also be phrased in a general way that applies to any test.
The null hypothesis is the claim that there’s no effect in the population.
If the sample provides enough evidence against the claim that there’s no effect in the population ( p ≤ α), then we can reject the null hypothesis . Otherwise, we fail to reject the null hypothesis.
Although “fail to reject” may sound awkward, it’s the only wording that statisticians accept. Be careful not to say you “prove” or “accept” the null hypothesis.
Null hypotheses often include phrases such as “no effect”, “no difference”, or “no relationship”. When written in mathematical terms, they always include an equality (usually =, but sometimes ≥ or ≤).
Examples of null hypotheses
The table below gives examples of research questions and null hypotheses. There’s always more than one way to answer a research question, but these null hypotheses can help you get started.
( ) | ||
Does tooth flossing affect the number of cavities? | Tooth flossing has on the number of cavities. | test: The mean number of cavities per person does not differ between the flossing group (µ ) and the non-flossing group (µ ) in the population; µ = µ . |
Does the amount of text highlighted in the textbook affect exam scores? | The amount of text highlighted in the textbook has on exam scores. | : There is no relationship between the amount of text highlighted and exam scores in the population; β = 0. |
Does daily meditation decrease the incidence of depression? | Daily meditation the incidence of depression.* | test: The proportion of people with depression in the daily-meditation group ( ) is greater than or equal to the no-meditation group ( ) in the population; ≥ . |
*Note that some researchers prefer to always write the null hypothesis in terms of “no effect” and “=”. It would be fine to say that daily meditation has no effect on the incidence of depression and p 1 = p 2 .
The alternative hypothesis (H A ) is the other answer to your research question . It claims that there’s an effect in the population.
Often, your alternative hypothesis is the same as your research hypothesis. In other words, it’s the claim that you expect or hope will be true.
The alternative hypothesis is the complement to the null hypothesis. Null and alternative hypotheses are exhaustive, meaning that together they cover every possible outcome. They are also mutually exclusive, meaning that only one can be true at a time.
Alternative hypotheses often include phrases such as “an effect”, “a difference”, or “a relationship”. When alternative hypotheses are written in mathematical terms, they always include an inequality (usually ≠, but sometimes > or <). As with null hypotheses, there are many acceptable ways to phrase an alternative hypothesis.
Examples of alternative hypotheses
The table below gives examples of research questions and alternative hypotheses to help you get started with formulating your own.
Does tooth flossing affect the number of cavities? | Tooth flossing has an on the number of cavities. | test: The mean number of cavities per person differs between the flossing group (µ ) and the non-flossing group (µ ) in the population; µ ≠ µ . |
Does the amount of text highlighted in a textbook affect exam scores? | The amount of text highlighted in the textbook has an on exam scores. | : There is a relationship between the amount of text highlighted and exam scores in the population; β ≠ 0. |
Does daily meditation decrease the incidence of depression? | Daily meditation the incidence of depression. | test: The proportion of people with depression in the daily-meditation group ( ) is less than the no-meditation group ( ) in the population; < . |
Null and alternative hypotheses are similar in some ways:
- They’re both answers to the research question
- They both make claims about the population
- They’re both evaluated by statistical tests.
However, there are important differences between the two types of hypotheses, summarized in the following table.
A claim that there is in the population. | A claim that there is in the population. | |
| ||
Equality symbol (=, ≥, or ≤) | Inequality symbol (≠, <, or >) | |
Rejected | Supported | |
Failed to reject | Not supported |
To help you write your hypotheses, you can use the template sentences below. If you know which statistical test you’re going to use, you can use the test-specific template sentences. Otherwise, you can use the general template sentences.
The only thing you need to know to use these general template sentences are your dependent and independent variables. To write your research question, null hypothesis, and alternative hypothesis, fill in the following sentences with your variables:
Does independent variable affect dependent variable ?
- Null hypothesis (H 0 ): Independent variable does not affect dependent variable .
- Alternative hypothesis (H A ): Independent variable affects dependent variable .
Test-specific
Once you know the statistical test you’ll be using, you can write your hypotheses in a more precise and mathematical way specific to the test you chose. The table below provides template sentences for common statistical tests.
( ) | ||
test
with two groups | The mean dependent variable does not differ between group 1 (µ ) and group 2 (µ ) in the population; µ = µ . | The mean dependent variable differs between group 1 (µ ) and group 2 (µ ) in the population; µ ≠ µ . |
with three groups | The mean dependent variable does not differ between group 1 (µ ), group 2 (µ ), and group 3 (µ ) in the population; µ = µ = µ . | The mean dependent variable of group 1 (µ ), group 2 (µ ), and group 3 (µ ) are not all equal in the population. |
There is no correlation between independent variable and dependent variable in the population; ρ = 0. | There is a correlation between independent variable and dependent variable in the population; ρ ≠ 0. | |
There is no relationship between independent variable and dependent variable in the population; β = 0. | There is a relationship between independent variable and dependent variable in the population; β ≠ 0. | |
Two-proportions test | The dependent variable expressed as a proportion does not differ between group 1 ( ) and group 2 ( ) in the population; = . | The dependent variable expressed as a proportion differs between group 1 ( ) and group 2 ( ) in the population; ≠ . |
Note: The template sentences above assume that you’re performing one-tailed tests . One-tailed tests are appropriate for most studies.
The null hypothesis is often abbreviated as H 0 . When the null hypothesis is written using mathematical symbols, it always includes an equality symbol (usually =, but sometimes ≥ or ≤).
The alternative hypothesis is often abbreviated as H a or H 1 . When the alternative hypothesis is written using mathematical symbols, it always includes an inequality symbol (usually ≠, but sometimes < or >).
A research hypothesis is your proposed answer to your research question. The research hypothesis usually includes an explanation (‘ x affects y because …’).
A statistical hypothesis, on the other hand, is a mathematical statement about a population parameter. Statistical hypotheses always come in pairs: the null and alternative hypotheses. In a well-designed study , the statistical hypotheses correspond logically to the research hypothesis.
Cite this Scribbr article
If you want to cite this source, you can copy and paste the citation or click the ‘Cite this Scribbr article’ button to automatically add the citation to our free Reference Generator.
Turney, S. (2022, December 06). Null and Alternative Hypotheses | Definitions & Examples. Scribbr. Retrieved 3 September 2024, from https://www.scribbr.co.uk/stats/null-and-alternative-hypothesis/
Is this article helpful?
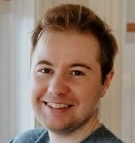
Shaun Turney
Other students also liked, levels of measurement: nominal, ordinal, interval, ratio, the standard normal distribution | calculator, examples & uses, types of variables in research | definitions & examples.
What is The Null Hypothesis & When Do You Reject The Null Hypothesis
Julia Simkus
Editor at Simply Psychology
BA (Hons) Psychology, Princeton University
Julia Simkus is a graduate of Princeton University with a Bachelor of Arts in Psychology. She is currently studying for a Master's Degree in Counseling for Mental Health and Wellness in September 2023. Julia's research has been published in peer reviewed journals.
Learn about our Editorial Process
Saul McLeod, PhD
Editor-in-Chief for Simply Psychology
BSc (Hons) Psychology, MRes, PhD, University of Manchester
Saul McLeod, PhD., is a qualified psychology teacher with over 18 years of experience in further and higher education. He has been published in peer-reviewed journals, including the Journal of Clinical Psychology.
Olivia Guy-Evans, MSc
Associate Editor for Simply Psychology
BSc (Hons) Psychology, MSc Psychology of Education
Olivia Guy-Evans is a writer and associate editor for Simply Psychology. She has previously worked in healthcare and educational sectors.
On This Page:
A null hypothesis is a statistical concept suggesting no significant difference or relationship between measured variables. It’s the default assumption unless empirical evidence proves otherwise.
The null hypothesis states no relationship exists between the two variables being studied (i.e., one variable does not affect the other).
The null hypothesis is the statement that a researcher or an investigator wants to disprove.
Testing the null hypothesis can tell you whether your results are due to the effects of manipulating the dependent variable or due to random chance.
How to Write a Null Hypothesis
Null hypotheses (H0) start as research questions that the investigator rephrases as statements indicating no effect or relationship between the independent and dependent variables.
It is a default position that your research aims to challenge or confirm.
For example, if studying the impact of exercise on weight loss, your null hypothesis might be:
There is no significant difference in weight loss between individuals who exercise daily and those who do not.
Examples of Null Hypotheses
Research Question | Null Hypothesis |
---|---|
Do teenagers use cell phones more than adults? | Teenagers and adults use cell phones the same amount. |
Do tomato plants exhibit a higher rate of growth when planted in compost rather than in soil? | Tomato plants show no difference in growth rates when planted in compost rather than soil. |
Does daily meditation decrease the incidence of depression? | Daily meditation does not decrease the incidence of depression. |
Does daily exercise increase test performance? | There is no relationship between daily exercise time and test performance. |
Does the new vaccine prevent infections? | The vaccine does not affect the infection rate. |
Does flossing your teeth affect the number of cavities? | Flossing your teeth has no effect on the number of cavities. |
When Do We Reject The Null Hypothesis?
We reject the null hypothesis when the data provide strong enough evidence to conclude that it is likely incorrect. This often occurs when the p-value (probability of observing the data given the null hypothesis is true) is below a predetermined significance level.
If the collected data does not meet the expectation of the null hypothesis, a researcher can conclude that the data lacks sufficient evidence to back up the null hypothesis, and thus the null hypothesis is rejected.
Rejecting the null hypothesis means that a relationship does exist between a set of variables and the effect is statistically significant ( p > 0.05).
If the data collected from the random sample is not statistically significance , then the null hypothesis will be accepted, and the researchers can conclude that there is no relationship between the variables.
You need to perform a statistical test on your data in order to evaluate how consistent it is with the null hypothesis. A p-value is one statistical measurement used to validate a hypothesis against observed data.
Calculating the p-value is a critical part of null-hypothesis significance testing because it quantifies how strongly the sample data contradicts the null hypothesis.
The level of statistical significance is often expressed as a p -value between 0 and 1. The smaller the p-value, the stronger the evidence that you should reject the null hypothesis.
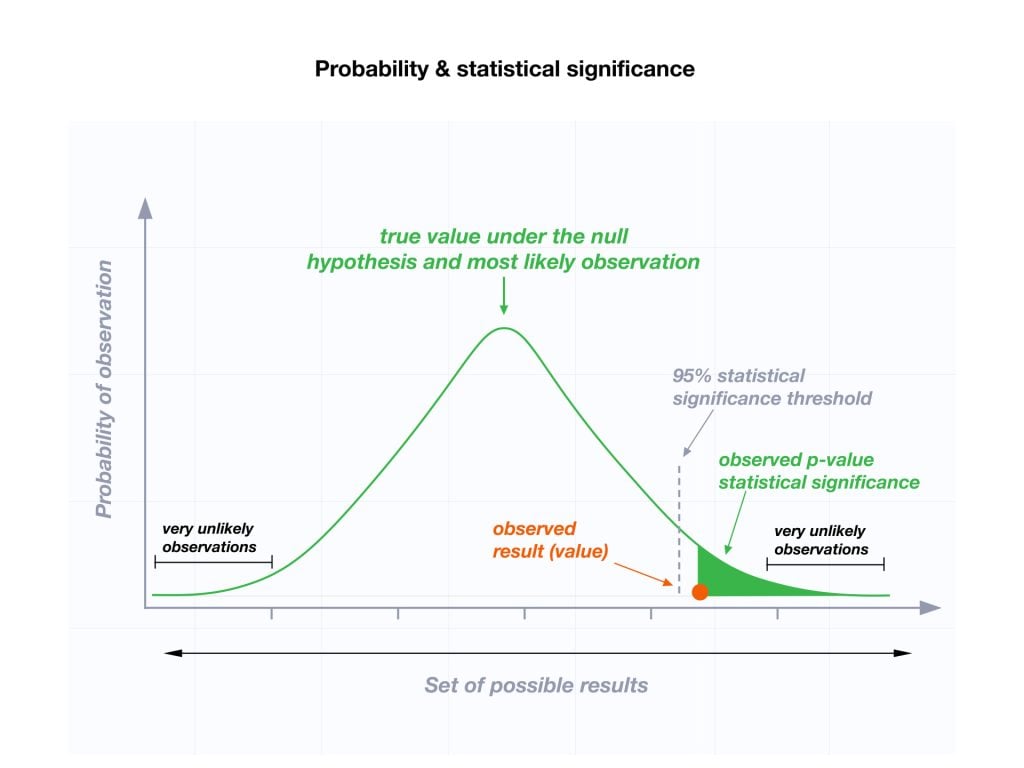
Usually, a researcher uses a confidence level of 95% or 99% (p-value of 0.05 or 0.01) as general guidelines to decide if you should reject or keep the null.
When your p-value is less than or equal to your significance level, you reject the null hypothesis.
In other words, smaller p-values are taken as stronger evidence against the null hypothesis. Conversely, when the p-value is greater than your significance level, you fail to reject the null hypothesis.
In this case, the sample data provides insufficient data to conclude that the effect exists in the population.
Because you can never know with complete certainty whether there is an effect in the population, your inferences about a population will sometimes be incorrect.
When you incorrectly reject the null hypothesis, it’s called a type I error. When you incorrectly fail to reject it, it’s called a type II error.
Why Do We Never Accept The Null Hypothesis?
The reason we do not say “accept the null” is because we are always assuming the null hypothesis is true and then conducting a study to see if there is evidence against it. And, even if we don’t find evidence against it, a null hypothesis is not accepted.
A lack of evidence only means that you haven’t proven that something exists. It does not prove that something doesn’t exist.
It is risky to conclude that the null hypothesis is true merely because we did not find evidence to reject it. It is always possible that researchers elsewhere have disproved the null hypothesis, so we cannot accept it as true, but instead, we state that we failed to reject the null.
One can either reject the null hypothesis, or fail to reject it, but can never accept it.
Why Do We Use The Null Hypothesis?
We can never prove with 100% certainty that a hypothesis is true; We can only collect evidence that supports a theory. However, testing a hypothesis can set the stage for rejecting or accepting this hypothesis within a certain confidence level.
The null hypothesis is useful because it can tell us whether the results of our study are due to random chance or the manipulation of a variable (with a certain level of confidence).
A null hypothesis is rejected if the measured data is significantly unlikely to have occurred and a null hypothesis is accepted if the observed outcome is consistent with the position held by the null hypothesis.
Rejecting the null hypothesis sets the stage for further experimentation to see if a relationship between two variables exists.
Hypothesis testing is a critical part of the scientific method as it helps decide whether the results of a research study support a particular theory about a given population. Hypothesis testing is a systematic way of backing up researchers’ predictions with statistical analysis.
It helps provide sufficient statistical evidence that either favors or rejects a certain hypothesis about the population parameter.
Purpose of a Null Hypothesis
- The primary purpose of the null hypothesis is to disprove an assumption.
- Whether rejected or accepted, the null hypothesis can help further progress a theory in many scientific cases.
- A null hypothesis can be used to ascertain how consistent the outcomes of multiple studies are.
Do you always need both a Null Hypothesis and an Alternative Hypothesis?
The null (H0) and alternative (Ha or H1) hypotheses are two competing claims that describe the effect of the independent variable on the dependent variable. They are mutually exclusive, which means that only one of the two hypotheses can be true.
While the null hypothesis states that there is no effect in the population, an alternative hypothesis states that there is statistical significance between two variables.
The goal of hypothesis testing is to make inferences about a population based on a sample. In order to undertake hypothesis testing, you must express your research hypothesis as a null and alternative hypothesis. Both hypotheses are required to cover every possible outcome of the study.
What is the difference between a null hypothesis and an alternative hypothesis?
The alternative hypothesis is the complement to the null hypothesis. The null hypothesis states that there is no effect or no relationship between variables, while the alternative hypothesis claims that there is an effect or relationship in the population.
It is the claim that you expect or hope will be true. The null hypothesis and the alternative hypothesis are always mutually exclusive, meaning that only one can be true at a time.
What are some problems with the null hypothesis?
One major problem with the null hypothesis is that researchers typically will assume that accepting the null is a failure of the experiment. However, accepting or rejecting any hypothesis is a positive result. Even if the null is not refuted, the researchers will still learn something new.
Why can a null hypothesis not be accepted?
We can either reject or fail to reject a null hypothesis, but never accept it. If your test fails to detect an effect, this is not proof that the effect doesn’t exist. It just means that your sample did not have enough evidence to conclude that it exists.
We can’t accept a null hypothesis because a lack of evidence does not prove something that does not exist. Instead, we fail to reject it.
Failing to reject the null indicates that the sample did not provide sufficient enough evidence to conclude that an effect exists.
If the p-value is greater than the significance level, then you fail to reject the null hypothesis.
Is a null hypothesis directional or non-directional?
A hypothesis test can either contain an alternative directional hypothesis or a non-directional alternative hypothesis. A directional hypothesis is one that contains the less than (“<“) or greater than (“>”) sign.
A nondirectional hypothesis contains the not equal sign (“≠”). However, a null hypothesis is neither directional nor non-directional.
A null hypothesis is a prediction that there will be no change, relationship, or difference between two variables.
The directional hypothesis or nondirectional hypothesis would then be considered alternative hypotheses to the null hypothesis.
Gill, J. (1999). The insignificance of null hypothesis significance testing. Political research quarterly , 52 (3), 647-674.
Krueger, J. (2001). Null hypothesis significance testing: On the survival of a flawed method. American Psychologist , 56 (1), 16.
Masson, M. E. (2011). A tutorial on a practical Bayesian alternative to null-hypothesis significance testing. Behavior research methods , 43 , 679-690.
Nickerson, R. S. (2000). Null hypothesis significance testing: a review of an old and continuing controversy. Psychological methods , 5 (2), 241.
Rozeboom, W. W. (1960). The fallacy of the null-hypothesis significance test. Psychological bulletin , 57 (5), 416.

Null Hypothesis
- Reference work entry
- First Online: 01 January 2020
- pp 3267–3270
- Cite this reference work entry
- Tom Booth 3 ,
- Alex Doumas 3 &
- Aja Louise Murray 4
34 Accesses
In formal hypothesis testing, the null hypothesis ( H 0 ) is the hypothesis assumed to be true in the population and which gives rise to the sampling distribution of the test statistic in question (Hays 1994 ). The critical feature of the null hypothesis across hypothesis testing frameworks is that it is stated with enough precision that it can be tested.
Introduction
A hypothesis is a statement or explanation about the nature or causes of some phenomena of interest. In the process of scientific study, we can distinguish two forms of hypotheses. A research hypothesis poses the question of interest, and if well stated, will include the variables under study and the expected relationship between them. A statistical hypothesis translates the research hypothesis into a mathematically precise, statistically testable statement concerning the assumed value of a parameter of interest in the population. The null hypothesis is an example of a statistical hypothesis.
In order to test these...
This is a preview of subscription content, log in via an institution to check access.
Access this chapter
Subscribe and save.
- Get 10 units per month
- Download Article/Chapter or eBook
- 1 Unit = 1 Article or 1 Chapter
- Cancel anytime
- Available as PDF
- Read on any device
- Instant download
- Own it forever
- Available as EPUB and PDF
- Durable hardcover edition
- Dispatched in 3 to 5 business days
- Free shipping worldwide - see info
Tax calculation will be finalised at checkout
Purchases are for personal use only
Institutional subscriptions
Fisher, R. (1925). Statistical methods for research workers (1st ed.). Edinburgh: Oliver and Boyd.
Google Scholar
Gigerenzer, G. (2004). Mindless statistics. The Journal of Socio-Economics, 33 , 587–606.
Article Google Scholar
Hays, W. L. (1994). Statistics (5th ed.). Belmont: Wadsworth.
Neyman, J., & Pearson, E. S. (1933). On the problem of the most efficient tests of statistical hypotheses. Philosophical Transactions of the Royal Society of London, Series A, 231 , 289–337.
Szucs, D., & Ioannidis, J. P. A. (2016). When null hypothesis significance testing is unsuitable for research: A reassessment. bioRxiv . https://doi.org/10.1101/095570 .
Download references
Author information
Authors and affiliations.
Department of Psychology, University of Edinburgh, Edinburgh, UK
Tom Booth & Alex Doumas
Violence Research Centre, Institute of Criminology, University of Cambridge, Cambridge, UK
Aja Louise Murray
You can also search for this author in PubMed Google Scholar
Corresponding author
Correspondence to Tom Booth .
Editor information
Editors and affiliations.
Oakland University, Rochester, MI, USA
Virgil Zeigler-Hill
Todd K. Shackelford
Section Editor information
Humboldt University, Germany, Berlin, Germany
Matthias Ziegler
Rights and permissions
Reprints and permissions
Copyright information
© 2020 Springer Nature Switzerland AG
About this entry
Cite this entry.
Booth, T., Doumas, A., Murray, A.L. (2020). Null Hypothesis. In: Zeigler-Hill, V., Shackelford, T.K. (eds) Encyclopedia of Personality and Individual Differences. Springer, Cham. https://doi.org/10.1007/978-3-319-24612-3_1335
Download citation
DOI : https://doi.org/10.1007/978-3-319-24612-3_1335
Published : 22 April 2020
Publisher Name : Springer, Cham
Print ISBN : 978-3-319-24610-9
Online ISBN : 978-3-319-24612-3
eBook Packages : Behavioral Science and Psychology Reference Module Humanities and Social Sciences Reference Module Business, Economics and Social Sciences
Share this entry
Anyone you share the following link with will be able to read this content:
Sorry, a shareable link is not currently available for this article.
Provided by the Springer Nature SharedIt content-sharing initiative
- Publish with us
Policies and ethics
- Find a journal
- Track your research
Null Hypothesis Definition and Examples
PM Images / Getty Images
- Chemical Laws
- Periodic Table
- Projects & Experiments
- Scientific Method
- Biochemistry
- Physical Chemistry
- Medical Chemistry
- Chemistry In Everyday Life
- Famous Chemists
- Activities for Kids
- Abbreviations & Acronyms
- Weather & Climate
- Ph.D., Biomedical Sciences, University of Tennessee at Knoxville
- B.A., Physics and Mathematics, Hastings College
In a scientific experiment, the null hypothesis is the proposition that there is no effect or no relationship between phenomena or populations. If the null hypothesis is true, any observed difference in phenomena or populations would be due to sampling error (random chance) or experimental error. The null hypothesis is useful because it can be tested and found to be false, which then implies that there is a relationship between the observed data. It may be easier to think of it as a nullifiable hypothesis or one that the researcher seeks to nullify. The null hypothesis is also known as the H 0, or no-difference hypothesis.
The alternate hypothesis, H A or H 1 , proposes that observations are influenced by a non-random factor. In an experiment, the alternate hypothesis suggests that the experimental or independent variable has an effect on the dependent variable .
How to State a Null Hypothesis
There are two ways to state a null hypothesis. One is to state it as a declarative sentence, and the other is to present it as a mathematical statement.
For example, say a researcher suspects that exercise is correlated to weight loss, assuming diet remains unchanged. The average length of time to achieve a certain amount of weight loss is six weeks when a person works out five times a week. The researcher wants to test whether weight loss takes longer to occur if the number of workouts is reduced to three times a week.
The first step to writing the null hypothesis is to find the (alternate) hypothesis. In a word problem like this, you're looking for what you expect to be the outcome of the experiment. In this case, the hypothesis is "I expect weight loss to take longer than six weeks."
This can be written mathematically as: H 1 : μ > 6
In this example, μ is the average.
Now, the null hypothesis is what you expect if this hypothesis does not happen. In this case, if weight loss isn't achieved in greater than six weeks, then it must occur at a time equal to or less than six weeks. This can be written mathematically as:
H 0 : μ ≤ 6
The other way to state the null hypothesis is to make no assumption about the outcome of the experiment. In this case, the null hypothesis is simply that the treatment or change will have no effect on the outcome of the experiment. For this example, it would be that reducing the number of workouts would not affect the time needed to achieve weight loss:
H 0 : μ = 6
Null Hypothesis Examples
"Hyperactivity is unrelated to eating sugar " is an example of a null hypothesis. If the hypothesis is tested and found to be false, using statistics, then a connection between hyperactivity and sugar ingestion may be indicated. A significance test is the most common statistical test used to establish confidence in a null hypothesis.
Another example of a null hypothesis is "Plant growth rate is unaffected by the presence of cadmium in the soil ." A researcher could test the hypothesis by measuring the growth rate of plants grown in a medium lacking cadmium, compared with the growth rate of plants grown in mediums containing different amounts of cadmium. Disproving the null hypothesis would set the groundwork for further research into the effects of different concentrations of the element in soil.
Why Test a Null Hypothesis?
You may be wondering why you would want to test a hypothesis just to find it false. Why not just test an alternate hypothesis and find it true? The short answer is that it is part of the scientific method. In science, propositions are not explicitly "proven." Rather, science uses math to determine the probability that a statement is true or false. It turns out it's much easier to disprove a hypothesis than to positively prove one. Also, while the null hypothesis may be simply stated, there's a good chance the alternate hypothesis is incorrect.
For example, if your null hypothesis is that plant growth is unaffected by duration of sunlight, you could state the alternate hypothesis in several different ways. Some of these statements might be incorrect. You could say plants are harmed by more than 12 hours of sunlight or that plants need at least three hours of sunlight, etc. There are clear exceptions to those alternate hypotheses, so if you test the wrong plants, you could reach the wrong conclusion. The null hypothesis is a general statement that can be used to develop an alternate hypothesis, which may or may not be correct.
- Kelvin Temperature Scale Definition
- Independent Variable Definition and Examples
- Theory Definition in Science
- Hypothesis Definition (Science)
- de Broglie Equation Definition
- Law of Combining Volumes Definition
- Chemical Definition
- Pure Substance Definition in Chemistry
- Acid Definition and Examples
- Extensive Property Definition (Chemistry)
- Radiation Definition and Examples
- Valence Definition in Chemistry
- Atomic Solid Definition
- Weak Base Definition and Examples
- Oxidation Definition and Example in Chemistry
- Definition of Binary Compound
Null Hypothesis Definition and Examples, How to State
What is the null hypothesis, how to state the null hypothesis, null hypothesis overview.

Why is it Called the “Null”?
The word “null” in this context means that it’s a commonly accepted fact that researchers work to nullify . It doesn’t mean that the statement is null (i.e. amounts to nothing) itself! (Perhaps the term should be called the “nullifiable hypothesis” as that might cause less confusion).
Why Do I need to Test it? Why not just prove an alternate one?
The short answer is, as a scientist, you are required to ; It’s part of the scientific process. Science uses a battery of processes to prove or disprove theories, making sure than any new hypothesis has no flaws. Including both a null and an alternate hypothesis is one safeguard to ensure your research isn’t flawed. Not including the null hypothesis in your research is considered very bad practice by the scientific community. If you set out to prove an alternate hypothesis without considering it, you are likely setting yourself up for failure. At a minimum, your experiment will likely not be taken seriously.
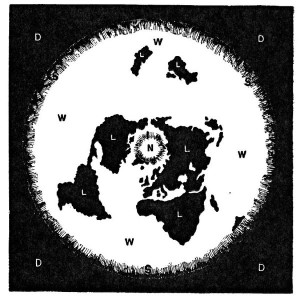
- Null hypothesis : H 0 : The world is flat.
- Alternate hypothesis: The world is round.
Several scientists, including Copernicus , set out to disprove the null hypothesis. This eventually led to the rejection of the null and the acceptance of the alternate. Most people accepted it — the ones that didn’t created the Flat Earth Society !. What would have happened if Copernicus had not disproved the it and merely proved the alternate? No one would have listened to him. In order to change people’s thinking, he first had to prove that their thinking was wrong .
How to State the Null Hypothesis from a Word Problem
You’ll be asked to convert a word problem into a hypothesis statement in statistics that will include a null hypothesis and an alternate hypothesis . Breaking your problem into a few small steps makes these problems much easier to handle.
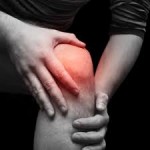
Step 2: Convert the hypothesis to math . Remember that the average is sometimes written as μ.
H 1 : μ > 8.2
Broken down into (somewhat) English, that’s H 1 (The hypothesis): μ (the average) > (is greater than) 8.2
Step 3: State what will happen if the hypothesis doesn’t come true. If the recovery time isn’t greater than 8.2 weeks, there are only two possibilities, that the recovery time is equal to 8.2 weeks or less than 8.2 weeks.
H 0 : μ ≤ 8.2
Broken down again into English, that’s H 0 (The null hypothesis): μ (the average) ≤ (is less than or equal to) 8.2
How to State the Null Hypothesis: Part Two
But what if the researcher doesn’t have any idea what will happen.
Example Problem: A researcher is studying the effects of radical exercise program on knee surgery patients. There is a good chance the therapy will improve recovery time, but there’s also the possibility it will make it worse. Average recovery times for knee surgery patients is 8.2 weeks.
Step 1: State what will happen if the experiment doesn’t make any difference. That’s the null hypothesis–that nothing will happen. In this experiment, if nothing happens, then the recovery time will stay at 8.2 weeks.
H 0 : μ = 8.2
Broken down into English, that’s H 0 (The null hypothesis): μ (the average) = (is equal to) 8.2
Step 2: Figure out the alternate hypothesis . The alternate hypothesis is the opposite of the null hypothesis. In other words, what happens if our experiment makes a difference?
H 1 : μ ≠ 8.2
In English again, that’s H 1 (The alternate hypothesis): μ (the average) ≠ (is not equal to) 8.2
That’s How to State the Null Hypothesis!
Check out our Youtube channel for more stats tips!
Gonick, L. (1993). The Cartoon Guide to Statistics . HarperPerennial. Kotz, S.; et al., eds. (2006), Encyclopedia of Statistical Sciences , Wiley.
9.1 Null and Alternative Hypotheses
The actual test begins by considering two hypotheses . They are called the null hypothesis and the alternative hypothesis . These hypotheses contain opposing viewpoints.
H 0 , the — null hypothesis: a statement of no difference between sample means or proportions or no difference between a sample mean or proportion and a population mean or proportion. In other words, the difference equals 0.
H a —, the alternative hypothesis: a claim about the population that is contradictory to H 0 and what we conclude when we reject H 0 .
Since the null and alternative hypotheses are contradictory, you must examine evidence to decide if you have enough evidence to reject the null hypothesis or not. The evidence is in the form of sample data.
After you have determined which hypothesis the sample supports, you make a decision. There are two options for a decision. They are reject H 0 if the sample information favors the alternative hypothesis or do not reject H 0 or decline to reject H 0 if the sample information is insufficient to reject the null hypothesis.
Mathematical Symbols Used in H 0 and H a :
equal (=) | not equal (≠) greater than (>) less than (<) |
greater than or equal to (≥) | less than (<) |
less than or equal to (≤) | more than (>) |
H 0 always has a symbol with an equal in it. H a never has a symbol with an equal in it. The choice of symbol depends on the wording of the hypothesis test. However, be aware that many researchers use = in the null hypothesis, even with > or < as the symbol in the alternative hypothesis. This practice is acceptable because we only make the decision to reject or not reject the null hypothesis.
Example 9.1
H 0 : No more than 30 percent of the registered voters in Santa Clara County voted in the primary election. p ≤ 30 H a : More than 30 percent of the registered voters in Santa Clara County voted in the primary election. p > 30
A medical trial is conducted to test whether or not a new medicine reduces cholesterol by 25 percent. State the null and alternative hypotheses.
Example 9.2
We want to test whether the mean GPA of students in American colleges is different from 2.0 (out of 4.0). The null and alternative hypotheses are the following: H 0 : μ = 2.0 H a : μ ≠ 2.0
We want to test whether the mean height of eighth graders is 66 inches. State the null and alternative hypotheses. Fill in the correct symbol (=, ≠, ≥, <, ≤, >) for the null and alternative hypotheses.
- H 0 : μ __ 66
- H a : μ __ 66
Example 9.3
We want to test if college students take fewer than five years to graduate from college, on the average. The null and alternative hypotheses are the following: H 0 : μ ≥ 5 H a : μ < 5
We want to test if it takes fewer than 45 minutes to teach a lesson plan. State the null and alternative hypotheses. Fill in the correct symbol ( =, ≠, ≥, <, ≤, >) for the null and alternative hypotheses.
- H 0 : μ __ 45
- H a : μ __ 45
Example 9.4
An article on school standards stated that about half of all students in France, Germany, and Israel take advanced placement exams and a third of the students pass. The same article stated that 6.6 percent of U.S. students take advanced placement exams and 4.4 percent pass. Test if the percentage of U.S. students who take advanced placement exams is more than 6.6 percent. State the null and alternative hypotheses. H 0 : p ≤ 0.066 H a : p > 0.066
On a state driver’s test, about 40 percent pass the test on the first try. We want to test if more than 40 percent pass on the first try. Fill in the correct symbol (=, ≠, ≥, <, ≤, >) for the null and alternative hypotheses.
- H 0 : p __ 0.40
- H a : p __ 0.40
Collaborative Exercise
Bring to class a newspaper, some news magazines, and some internet articles. In groups, find articles from which your group can write null and alternative hypotheses. Discuss your hypotheses with the rest of the class.
This book may not be used in the training of large language models or otherwise be ingested into large language models or generative AI offerings without OpenStax's permission.
Want to cite, share, or modify this book? This book uses the Creative Commons Attribution License and you must attribute Texas Education Agency (TEA). The original material is available at: https://www.texasgateway.org/book/tea-statistics . Changes were made to the original material, including updates to art, structure, and other content updates.
Access for free at https://openstax.org/books/statistics/pages/1-introduction
- Authors: Barbara Illowsky, Susan Dean
- Publisher/website: OpenStax
- Book title: Statistics
- Publication date: Mar 27, 2020
- Location: Houston, Texas
- Book URL: https://openstax.org/books/statistics/pages/1-introduction
- Section URL: https://openstax.org/books/statistics/pages/9-1-null-and-alternative-hypotheses
© Apr 16, 2024 Texas Education Agency (TEA). The OpenStax name, OpenStax logo, OpenStax book covers, OpenStax CNX name, and OpenStax CNX logo are not subject to the Creative Commons license and may not be reproduced without the prior and express written consent of Rice University.

Want to create or adapt books like this? Learn more about how Pressbooks supports open publishing practices.
13.1 Understanding Null Hypothesis Testing
Learning objectives.
- Explain the purpose of null hypothesis testing, including the role of sampling error.
- Describe the basic logic of null hypothesis testing.
- Describe the role of relationship strength and sample size in determining statistical significance and make reasonable judgments about statistical significance based on these two factors.
The Purpose of Null Hypothesis Testing
As we have seen, psychological research typically involves measuring one or more variables for a sample and computing descriptive statistics for that sample. In general, however, the researcher’s goal is not to draw conclusions about that sample but to draw conclusions about the population that the sample was selected from. Thus researchers must use sample statistics to draw conclusions about the corresponding values in the population. These corresponding values in the population are called parameters . Imagine, for example, that a researcher measures the number of depressive symptoms exhibited by each of 50 clinically depressed adults and computes the mean number of symptoms. The researcher probably wants to use this sample statistic (the mean number of symptoms for the sample) to draw conclusions about the corresponding population parameter (the mean number of symptoms for clinically depressed adults).
Unfortunately, sample statistics are not perfect estimates of their corresponding population parameters. This is because there is a certain amount of random variability in any statistic from sample to sample. The mean number of depressive symptoms might be 8.73 in one sample of clinically depressed adults, 6.45 in a second sample, and 9.44 in a third—even though these samples are selected randomly from the same population. Similarly, the correlation (Pearson’s r ) between two variables might be +.24 in one sample, −.04 in a second sample, and +.15 in a third—again, even though these samples are selected randomly from the same population. This random variability in a statistic from sample to sample is called sampling error . (Note that the term error here refers to random variability and does not imply that anyone has made a mistake. No one “commits a sampling error.”)
One implication of this is that when there is a statistical relationship in a sample, it is not always clear that there is a statistical relationship in the population. A small difference between two group means in a sample might indicate that there is a small difference between the two group means in the population. But it could also be that there is no difference between the means in the population and that the difference in the sample is just a matter of sampling error. Similarly, a Pearson’s r value of −.29 in a sample might mean that there is a negative relationship in the population. But it could also be that there is no relationship in the population and that the relationship in the sample is just a matter of sampling error.
In fact, any statistical relationship in a sample can be interpreted in two ways:
- There is a relationship in the population, and the relationship in the sample reflects this.
- There is no relationship in the population, and the relationship in the sample reflects only sampling error.
The purpose of null hypothesis testing is simply to help researchers decide between these two interpretations.
The Logic of Null Hypothesis Testing
Null hypothesis testing is a formal approach to deciding between two interpretations of a statistical relationship in a sample. One interpretation is called the null hypothesis (often symbolized H 0 and read as “H-naught”). This is the idea that there is no relationship in the population and that the relationship in the sample reflects only sampling error. Informally, the null hypothesis is that the sample relationship “occurred by chance.” The other interpretation is called the alternative hypothesis (often symbolized as H 1 ). This is the idea that there is a relationship in the population and that the relationship in the sample reflects this relationship in the population.
Again, every statistical relationship in a sample can be interpreted in either of these two ways: It might have occurred by chance, or it might reflect a relationship in the population. So researchers need a way to decide between them. Although there are many specific null hypothesis testing techniques, they are all based on the same general logic. The steps are as follows:
- Assume for the moment that the null hypothesis is true. There is no relationship between the variables in the population.
- Determine how likely the sample relationship would be if the null hypothesis were true.
- If the sample relationship would be extremely unlikely, then reject the null hypothesis in favor of the alternative hypothesis. If it would not be extremely unlikely, then retain the null hypothesis .
Following this logic, we can begin to understand why Mehl and his colleagues concluded that there is no difference in talkativeness between women and men in the population. In essence, they asked the following question: “If there were no difference in the population, how likely is it that we would find a small difference of d = 0.06 in our sample?” Their answer to this question was that this sample relationship would be fairly likely if the null hypothesis were true. Therefore, they retained the null hypothesis—concluding that there is no evidence of a sex difference in the population. We can also see why Kanner and his colleagues concluded that there is a correlation between hassles and symptoms in the population. They asked, “If the null hypothesis were true, how likely is it that we would find a strong correlation of +.60 in our sample?” Their answer to this question was that this sample relationship would be fairly unlikely if the null hypothesis were true. Therefore, they rejected the null hypothesis in favor of the alternative hypothesis—concluding that there is a positive correlation between these variables in the population.
A crucial step in null hypothesis testing is finding the likelihood of the sample result if the null hypothesis were true. This probability is called the p value . A low p value means that the sample result would be unlikely if the null hypothesis were true and leads to the rejection of the null hypothesis. A high p value means that the sample result would be likely if the null hypothesis were true and leads to the retention of the null hypothesis. But how low must the p value be before the sample result is considered unlikely enough to reject the null hypothesis? In null hypothesis testing, this criterion is called α (alpha) and is almost always set to .05. If there is less than a 5% chance of a result as extreme as the sample result if the null hypothesis were true, then the null hypothesis is rejected. When this happens, the result is said to be statistically significant . If there is greater than a 5% chance of a result as extreme as the sample result when the null hypothesis is true, then the null hypothesis is retained. This does not necessarily mean that the researcher accepts the null hypothesis as true—only that there is not currently enough evidence to conclude that it is true. Researchers often use the expression “fail to reject the null hypothesis” rather than “retain the null hypothesis,” but they never use the expression “accept the null hypothesis.”
The Misunderstood p Value
The p value is one of the most misunderstood quantities in psychological research (Cohen, 1994). Even professional researchers misinterpret it, and it is not unusual for such misinterpretations to appear in statistics textbooks!
The most common misinterpretation is that the p value is the probability that the null hypothesis is true—that the sample result occurred by chance. For example, a misguided researcher might say that because the p value is .02, there is only a 2% chance that the result is due to chance and a 98% chance that it reflects a real relationship in the population. But this is incorrect . The p value is really the probability of a result at least as extreme as the sample result if the null hypothesis were true. So a p value of .02 means that if the null hypothesis were true, a sample result this extreme would occur only 2% of the time.
You can avoid this misunderstanding by remembering that the p value is not the probability that any particular hypothesis is true or false. Instead, it is the probability of obtaining the sample result if the null hypothesis were true.
Role of Sample Size and Relationship Strength
Recall that null hypothesis testing involves answering the question, “If the null hypothesis were true, what is the probability of a sample result as extreme as this one?” In other words, “What is the p value?” It can be helpful to see that the answer to this question depends on just two considerations: the strength of the relationship and the size of the sample. Specifically, the stronger the sample relationship and the larger the sample, the less likely the result would be if the null hypothesis were true. That is, the lower the p value. This should make sense. Imagine a study in which a sample of 500 women is compared with a sample of 500 men in terms of some psychological characteristic, and Cohen’s d is a strong 0.50. If there were really no sex difference in the population, then a result this strong based on such a large sample should seem highly unlikely. Now imagine a similar study in which a sample of three women is compared with a sample of three men, and Cohen’s d is a weak 0.10. If there were no sex difference in the population, then a relationship this weak based on such a small sample should seem likely. And this is precisely why the null hypothesis would be rejected in the first example and retained in the second.
Of course, sometimes the result can be weak and the sample large, or the result can be strong and the sample small. In these cases, the two considerations trade off against each other so that a weak result can be statistically significant if the sample is large enough and a strong relationship can be statistically significant even if the sample is small. Table 13.1 “How Relationship Strength and Sample Size Combine to Determine Whether a Result Is Statistically Significant” shows roughly how relationship strength and sample size combine to determine whether a sample result is statistically significant. The columns of the table represent the three levels of relationship strength: weak, medium, and strong. The rows represent four sample sizes that can be considered small, medium, large, and extra large in the context of psychological research. Thus each cell in the table represents a combination of relationship strength and sample size. If a cell contains the word Yes , then this combination would be statistically significant for both Cohen’s d and Pearson’s r . If it contains the word No , then it would not be statistically significant for either. There is one cell where the decision for d and r would be different and another where it might be different depending on some additional considerations, which are discussed in Section 13.2 “Some Basic Null Hypothesis Tests”
Table 13.1 How Relationship Strength and Sample Size Combine to Determine Whether a Result Is Statistically Significant
Relationship strength | |||
---|---|---|---|
Sample Size | Weak | Medium | Strong |
Small ( = 20) | No | No | = Maybe = Yes |
Medium ( = 50) | No | Yes | Yes |
Large ( = 100) | = Yes = No | Yes | Yes |
Extra large ( = 500) | Yes | Yes | Yes |
Although Table 13.1 “How Relationship Strength and Sample Size Combine to Determine Whether a Result Is Statistically Significant” provides only a rough guideline, it shows very clearly that weak relationships based on medium or small samples are never statistically significant and that strong relationships based on medium or larger samples are always statistically significant. If you keep this in mind, you will often know whether a result is statistically significant based on the descriptive statistics alone. It is extremely useful to be able to develop this kind of intuitive judgment. One reason is that it allows you to develop expectations about how your formal null hypothesis tests are going to come out, which in turn allows you to detect problems in your analyses. For example, if your sample relationship is strong and your sample is medium, then you would expect to reject the null hypothesis. If for some reason your formal null hypothesis test indicates otherwise, then you need to double-check your computations and interpretations. A second reason is that the ability to make this kind of intuitive judgment is an indication that you understand the basic logic of this approach in addition to being able to do the computations.
Statistical Significance Versus Practical Significance
Table 13.1 “How Relationship Strength and Sample Size Combine to Determine Whether a Result Is Statistically Significant” illustrates another extremely important point. A statistically significant result is not necessarily a strong one. Even a very weak result can be statistically significant if it is based on a large enough sample. This is closely related to Janet Shibley Hyde’s argument about sex differences (Hyde, 2007). The differences between women and men in mathematical problem solving and leadership ability are statistically significant. But the word significant can cause people to interpret these differences as strong and important—perhaps even important enough to influence the college courses they take or even who they vote for. As we have seen, however, these statistically significant differences are actually quite weak—perhaps even “trivial.”
This is why it is important to distinguish between the statistical significance of a result and the practical significance of that result. Practical significance refers to the importance or usefulness of the result in some real-world context. Many sex differences are statistically significant—and may even be interesting for purely scientific reasons—but they are not practically significant. In clinical practice, this same concept is often referred to as “clinical significance.” For example, a study on a new treatment for social phobia might show that it produces a statistically significant positive effect. Yet this effect still might not be strong enough to justify the time, effort, and other costs of putting it into practice—especially if easier and cheaper treatments that work almost as well already exist. Although statistically significant, this result would be said to lack practical or clinical significance.
Key Takeaways
- Null hypothesis testing is a formal approach to deciding whether a statistical relationship in a sample reflects a real relationship in the population or is just due to chance.
- The logic of null hypothesis testing involves assuming that the null hypothesis is true, finding how likely the sample result would be if this assumption were correct, and then making a decision. If the sample result would be unlikely if the null hypothesis were true, then it is rejected in favor of the alternative hypothesis. If it would not be unlikely, then the null hypothesis is retained.
- The probability of obtaining the sample result if the null hypothesis were true (the p value) is based on two considerations: relationship strength and sample size. Reasonable judgments about whether a sample relationship is statistically significant can often be made by quickly considering these two factors.
- Statistical significance is not the same as relationship strength or importance. Even weak relationships can be statistically significant if the sample size is large enough. It is important to consider relationship strength and the practical significance of a result in addition to its statistical significance.
- Discussion: Imagine a study showing that people who eat more broccoli tend to be happier. Explain for someone who knows nothing about statistics why the researchers would conduct a null hypothesis test.
Practice: Use Table 13.1 “How Relationship Strength and Sample Size Combine to Determine Whether a Result Is Statistically Significant” to decide whether each of the following results is statistically significant.
- The correlation between two variables is r = −.78 based on a sample size of 137.
- The mean score on a psychological characteristic for women is 25 ( SD = 5) and the mean score for men is 24 ( SD = 5). There were 12 women and 10 men in this study.
- In a memory experiment, the mean number of items recalled by the 40 participants in Condition A was 0.50 standard deviations greater than the mean number recalled by the 40 participants in Condition B.
- In another memory experiment, the mean scores for participants in Condition A and Condition B came out exactly the same!
- A student finds a correlation of r = .04 between the number of units the students in his research methods class are taking and the students’ level of stress.
Cohen, J. (1994). The world is round: p < .05. American Psychologist, 49 , 997–1003.
Hyde, J. S. (2007). New directions in the study of gender similarities and differences. Current Directions in Psychological Science , 16 , 259–263.
Research Methods in Psychology Copyright © 2016 by University of Minnesota is licensed under a Creative Commons Attribution-NonCommercial-ShareAlike 4.0 International License , except where otherwise noted.

Step-by-step guide to hypothesis testing in statistics
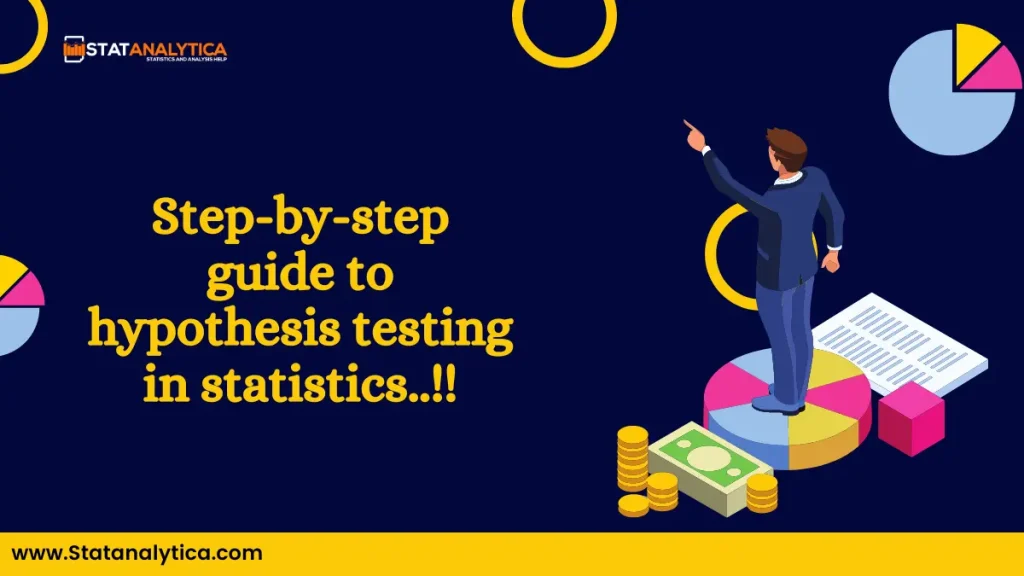
Hypothesis testing in statistics helps us use data to make informed decisions. It starts with an assumption or guess about a group or population—something we believe might be true. We then collect sample data to check if there is enough evidence to support or reject that guess. This method is useful in many fields, like science, business, and healthcare, where decisions need to be based on facts.
Learning how to do hypothesis testing in statistics step-by-step can help you better understand data and make smarter choices, even when things are uncertain. This guide will take you through each step, from creating your hypothesis to making sense of the results, so you can see how it works in practical situations.
What is Hypothesis Testing?
Table of Contents
Hypothesis testing is a method for determining whether data supports a certain idea or assumption about a larger group. It starts by making a guess, like an average or a proportion, and then uses a small sample of data to see if that guess seems true or not.
For example, if a company wants to know if its new product is more popular than its old one, it can use hypothesis testing. They start with a statement like “The new product is not more popular than the old one” (this is the null hypothesis) and compare it with “The new product is more popular” (this is the alternative hypothesis). Then, they look at customer feedback to see if there’s enough evidence to reject the first statement and support the second one.
Simply put, hypothesis testing is a way to use data to help make decisions and understand what the data is really telling us, even when we don’t have all the answers.
Importance Of Hypothesis Testing In Decision-Making And Data Analysis
Hypothesis testing is important because it helps us make smart choices and understand data better. Here’s why it’s useful:
- Reduces Guesswork : It helps us see if our guesses or ideas are likely correct, even when we don’t have all the details.
- Uses Real Data : Instead of just guessing, it checks if our ideas match up with real data, which makes our decisions more reliable.
- Avoids Errors : It helps us avoid mistakes by carefully checking if our ideas are right so we don’t make costly errors.
- Shows What to Do Next : It tells us if our ideas work or not, helping us decide whether to keep, change, or drop something. For example, a company might test a new ad and decide what to do based on the results.
- Confirms Research Findings : It makes sure that research results are accurate and not just random chance so that we can trust the findings.
Here’s a simple guide to understanding hypothesis testing, with an example:
1. Set Up Your Hypotheses
Explanation: Start by defining two statements:
- Null Hypothesis (H0): This is the idea that there is no change or effect. It’s what you assume is true.
- Alternative Hypothesis (H1): This is what you want to test. It suggests there is a change or effect.
Example: Suppose a company says their new batteries last an average of 500 hours. To check this:
- Null Hypothesis (H0): The average battery life is 500 hours.
- Alternative Hypothesis (H1): The average battery life is not 500 hours.
2. Choose the Test
Explanation: Pick a statistical test that fits your data and your hypotheses. Different tests are used for various kinds of data.
Example: Since you’re comparing the average battery life, you use a one-sample t-test .
3. Set the Significance Level
Explanation: Decide how much risk you’re willing to take if you make a wrong decision. This is called the significance level, often set at 0.05 or 5%.
Example: You choose a significance level of 0.05, meaning you’re okay with a 5% chance of being wrong.
4. Gather and Analyze Data
Explanation: Collect your data and perform the test. Calculate the test statistic to see how far your sample result is from what you assumed.
Example: You test 30 batteries and find they last an average of 485 hours. You then calculate how this average compares to the claimed 500 hours using the t-test.
5. Find the p-Value
Explanation: The p-value tells you the probability of getting a result as extreme as yours if the null hypothesis is true.
Example: You find a p-value of 0.0001. This means there’s a very small chance (0.01%) of getting an average battery life of 485 hours or less if the true average is 500 hours.
6. Make Your Decision
Explanation: Compare the p-value to your significance level. If the p-value is smaller, you reject the null hypothesis. If it’s larger, you do not reject it.
Example: Since 0.0001 is much less than 0.05, you reject the null hypothesis. This means the data suggests the average battery life is different from 500 hours.
7. Report Your Findings
Explanation: Summarize what the results mean. State whether you rejected the null hypothesis and what that implies.
Example: You conclude that the average battery life is likely different from 500 hours. This suggests the company’s claim might not be accurate.
Hypothesis testing is a way to use data to check if your guesses or assumptions are likely true. By following these steps—setting up your hypotheses, choosing the right test, deciding on a significance level, analyzing your data, finding the p-value, making a decision, and reporting results—you can determine if your data supports or challenges your initial idea.
Understanding Hypothesis Testing: A Simple Explanation
Hypothesis testing is a way to use data to make decisions. Here’s a straightforward guide:
1. What is the Null and Alternative Hypotheses?
- Null Hypothesis (H0): This is your starting assumption. It says that nothing has changed or that there is no effect. It’s what you assume to be true until your data shows otherwise. Example: If a company says their batteries last 500 hours, the null hypothesis is: “The average battery life is 500 hours.” This means you think the claim is correct unless you find evidence to prove otherwise.
- Alternative Hypothesis (H1): This is what you want to find out. It suggests that there is an effect or a difference. It’s what you are testing to see if it might be true. Example: To test the company’s claim, you might say: “The average battery life is not 500 hours.” This means you think the average battery life might be different from what the company says.
2. One-Tailed vs. Two-Tailed Tests
- One-Tailed Test: This test checks for an effect in only one direction. You use it when you’re only interested in finding out if something is either more or less than a specific value. Example: If you think the battery lasts longer than 500 hours, you would use a one-tailed test to see if the battery life is significantly more than 500 hours.
- Two-Tailed Test: This test checks for an effect in both directions. Use this when you want to see if something is different from a specific value, whether it’s more or less. Example: If you want to see if the battery life is different from 500 hours, whether it’s more or less, you would use a two-tailed test. This checks for any significant difference, regardless of the direction.
3. Common Misunderstandings
- Clarification: Hypothesis testing doesn’t prove that the null hypothesis is true. It just helps you decide if you should reject it. If there isn’t enough evidence against it, you don’t reject it, but that doesn’t mean it’s definitely true.
- Clarification: A small p-value shows that your data is unlikely if the null hypothesis is true. It suggests that the alternative hypothesis might be right, but it doesn’t prove the null hypothesis is false.
- Clarification: The significance level (alpha) is a set threshold, like 0.05, that helps you decide how much risk you’re willing to take for making a wrong decision. It should be chosen carefully, not randomly.
- Clarification: Hypothesis testing helps you make decisions based on data, but it doesn’t guarantee your results are correct. The quality of your data and the right choice of test affect how reliable your results are.
Benefits and Limitations of Hypothesis Testing
- Clear Decisions: Hypothesis testing helps you make clear decisions based on data. It shows whether the evidence supports or goes against your initial idea.
- Objective Analysis: It relies on data rather than personal opinions, so your decisions are based on facts rather than feelings.
- Concrete Numbers: You get specific numbers, like p-values, to understand how strong the evidence is against your idea.
- Control Risk: You can set a risk level (alpha level) to manage the chance of making an error, which helps avoid incorrect conclusions.
- Widely Used: It can be used in many areas, from science and business to social studies and engineering, making it a versatile tool.
Limitations
- Sample Size Matters: The results can be affected by the size of the sample. Small samples might give unreliable results, while large samples might find differences that aren’t meaningful in real life.
- Risk of Misinterpretation: A small p-value means the results are unlikely if the null hypothesis is true, but it doesn’t show how important the effect is.
- Needs Assumptions: Hypothesis testing requires certain conditions, like data being normally distributed . If these aren’t met, the results might not be accurate.
- Simple Decisions: It often results in a basic yes or no decision without giving detailed information about the size or impact of the effect.
- Can Be Misused: Sometimes, people misuse hypothesis testing, tweaking data to get a desired result or focusing only on whether the result is statistically significant.
- No Absolute Proof: Hypothesis testing doesn’t prove that your hypothesis is true. It only helps you decide if there’s enough evidence to reject the null hypothesis, so the conclusions are based on likelihood, not certainty.
Final Thoughts
Hypothesis testing helps you make decisions based on data. It involves setting up your initial idea, picking a significance level, doing the test, and looking at the results. By following these steps, you can make sure your conclusions are based on solid information, not just guesses.
This approach lets you see if the evidence supports or contradicts your initial idea, helping you make better decisions. But remember that hypothesis testing isn’t perfect. Things like sample size and assumptions can affect the results, so it’s important to be aware of these limitations.
In simple terms, using a step-by-step guide for hypothesis testing is a great way to better understand your data. Follow the steps carefully and keep in mind the method’s limits.
What is the difference between one-tailed and two-tailed tests?
A one-tailed test assesses the probability of the observed data in one direction (either greater than or less than a certain value). In contrast, a two-tailed test looks at both directions (greater than and less than) to detect any significant deviation from the null hypothesis.

How do you choose the appropriate test for hypothesis testing?
The choice of test depends on the type of data you have and the hypotheses you are testing. Common tests include t-tests, chi-square tests, and ANOVA. You get more details about ANOVA, you may read Complete Details on What is ANOVA in Statistics ? It’s important to match the test to the data characteristics and the research question.
What is the role of sample size in hypothesis testing?
Sample size affects the reliability of hypothesis testing. Larger samples provide more reliable estimates and can detect smaller effects, while smaller samples may lead to less accurate results and reduced power.
Can hypothesis testing prove that a hypothesis is true?
Hypothesis testing cannot prove that a hypothesis is true. It can only provide evidence to support or reject the null hypothesis. A result can indicate whether the data is consistent with the null hypothesis or not, but it does not prove the alternative hypothesis with certainty.
Related Posts
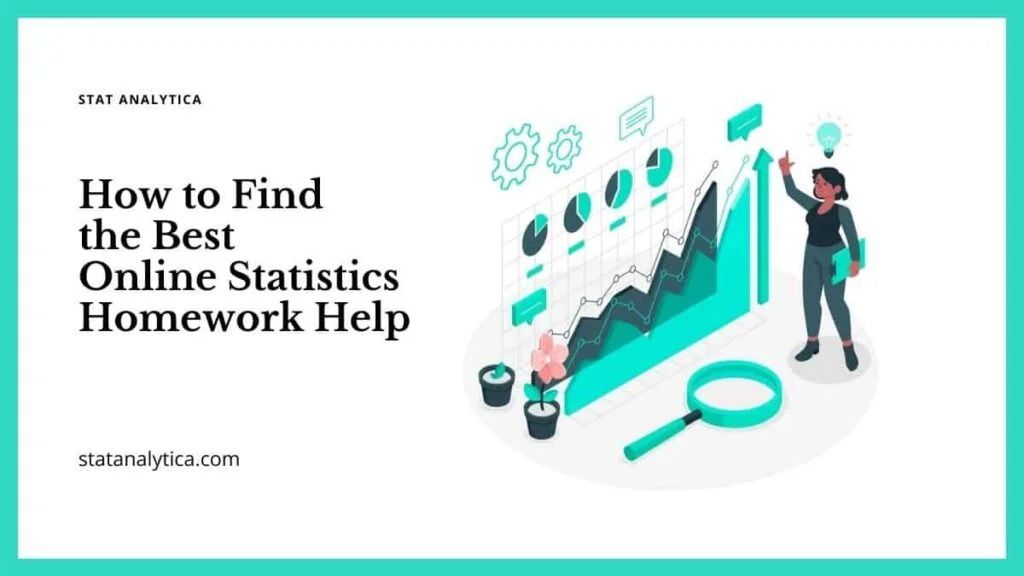
How to Find the Best Online Statistics Homework Help
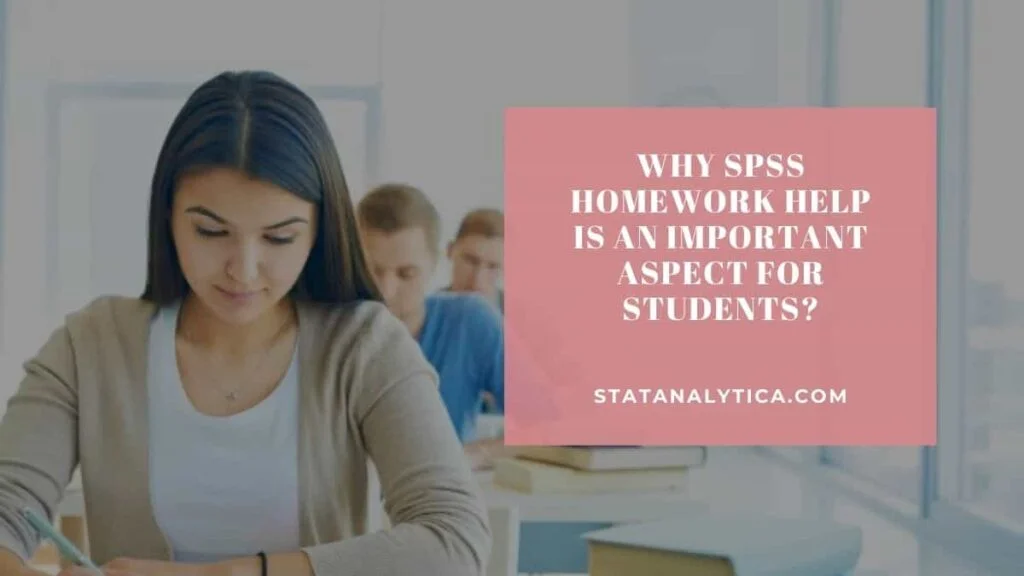
Why SPSS Homework Help Is An Important aspect for Students?
Leave a comment cancel reply.
Your email address will not be published. Required fields are marked *

What is Null Hypothesis? What Is Its Importance in Research?
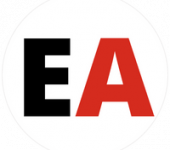
Scientists begin their research with a hypothesis that a relationship of some kind exists between variables. The null hypothesis is the opposite stating that no such relationship exists. Null hypothesis may seem unexciting, but it is a very important aspect of research. In this article, we discuss what null hypothesis is, how to make use of it, and why you should use it to improve your statistical analyses.
What is the Null Hypothesis?
The null hypothesis can be tested using statistical analysis and is often written as H 0 (read as “H-naught”). Once you determine how likely the sample relationship would be if the H 0 were true, you can run your analysis. Researchers use a significance test to determine the likelihood that the results supporting the H 0 are not due to chance.
The null hypothesis is not the same as an alternative hypothesis. An alternative hypothesis states, that there is a relationship between two variables, while H 0 posits the opposite. Let us consider the following example.
A researcher wants to discover the relationship between exercise frequency and appetite. She asks:
Q: Does increased exercise frequency lead to increased appetite? Alternative hypothesis: Increased exercise frequency leads to increased appetite. H 0 assumes that there is no relationship between the two variables: Increased exercise frequency does not lead to increased appetite.
Let us look at another example of how to state the null hypothesis:
Q: Does insufficient sleep lead to an increased risk of heart attack among men over age 50? H 0 : The amount of sleep men over age 50 get does not increase their risk of heart attack.
Why is Null Hypothesis Important?
Many scientists often neglect null hypothesis in their testing. As shown in the above examples, H 0 is often assumed to be the opposite of the hypothesis being tested. However, it is good practice to include H 0 and ensure it is carefully worded. To understand why, let us return to our previous example. In this case,
Alternative hypothesis: Getting too little sleep leads to an increased risk of heart attack among men over age 50.
H 0 : The amount of sleep men over age 50 get has no effect on their risk of heart attack.
Note that this H 0 is different than the one in our first example. What if we were to conduct this experiment and find that neither H 0 nor the alternative hypothesis was supported? The experiment would be considered invalid . Take our original H 0 in this case, “the amount of sleep men over age 50 get, does not increase their risk of heart attack”. If this H 0 is found to be untrue, and so is the alternative, we can still consider a third hypothesis. Perhaps getting insufficient sleep actually decreases the risk of a heart attack among men over age 50. Because we have tested H 0 , we have more information that we would not have if we had neglected it.
Do I Really Need to Test It?
The biggest problem with the null hypothesis is that many scientists see accepting it as a failure of the experiment. They consider that they have not proven anything of value. However, as we have learned from the replication crisis , negative results are just as important as positive ones. While they may seem less appealing to publishers, they can tell the scientific community important information about correlations that do or do not exist. In this way, they can drive science forward and prevent the wastage of resources.
Do you test for the null hypothesis? Why or why not? Let us know your thoughts in the comments below.

The following null hypotheses were formulated for this study: Ho1. There are no significant differences in the factors that influence urban gardening when respondents are grouped according to age, sex, household size, social status and average combined monthly income.
Rate this article Cancel Reply
Your email address will not be published.
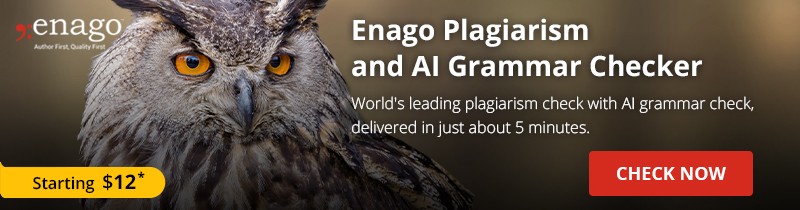
Enago Academy's Most Popular Articles
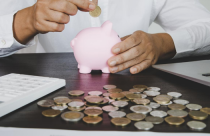
- Trending Now
- Upcoming Webinars
- Webinar Mobile App
Mastering Research Funding: A step-by-step guide to finding and winning grants
Identifying relevant funding opportunities Importance of eligibility criteria Understanding the funder’s perspective Crafting a strong…
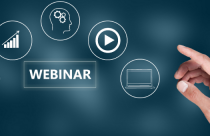
- Career Corner
Academic Webinars: Transforming knowledge dissemination in the digital age
Digitization has transformed several areas of our lives, including the teaching and learning process. During…
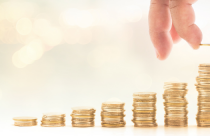
- Manuscripts & Grants
- Reporting Research
Mastering Research Grant Writing in 2024: Navigating new policies and funder demands
Entering the world of grants and government funding can leave you confused; especially when trying…
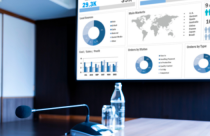
How to Create a Poster That Stands Out: Tips for a smooth poster presentation
It was the conference season. Judy was excited to present her first poster! She had…
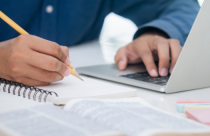
Academic Essay Writing Made Simple: 4 types and tips
The pen is mightier than the sword, they say, and nowhere is this more evident…
Recognizing the Signs: A guide to overcoming academic burnout
Intersectionality in Academia: Dealing with diverse perspectives

Sign-up to read more
Subscribe for free to get unrestricted access to all our resources on research writing and academic publishing including:
- 2000+ blog articles
- 50+ Webinars
- 10+ Expert podcasts
- 50+ Infographics
- 10+ Checklists
- Research Guides
We hate spam too. We promise to protect your privacy and never spam you.
- Industry News
- Publishing Research
- AI in Academia
- Promoting Research
- Diversity and Inclusion
- Infographics
- Expert Video Library
- Other Resources
- Enago Learn
- Upcoming & On-Demand Webinars
- Peer Review Week 2024
- Open Access Week 2023
- Conference Videos
- Enago Report
- Journal Finder
- Enago Plagiarism & AI Grammar Check
- Editing Services
- Publication Support Services
- Research Impact
- Translation Services
- Publication solutions
- AI-Based Solutions
- Thought Leadership
- Call for Articles
- Call for Speakers
- Author Training
- Edit Profile
I am looking for Editing/ Proofreading services for my manuscript Tentative date of next journal submission:
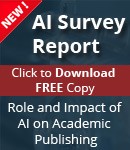
In your opinion, what is the most effective way to improve integrity in the peer review process?

- Science Notes Posts
- Contact Science Notes
- Todd Helmenstine Biography
- Anne Helmenstine Biography
- Free Printable Periodic Tables (PDF and PNG)
- Periodic Table Wallpapers
- Interactive Periodic Table
- Periodic Table Posters
- Science Experiments for Kids
- How to Grow Crystals
- Chemistry Projects
- Fire and Flames Projects
- Holiday Science
- Chemistry Problems With Answers
- Physics Problems
- Unit Conversion Example Problems
- Chemistry Worksheets
- Biology Worksheets
- Periodic Table Worksheets
- Physical Science Worksheets
- Science Lab Worksheets
- My Amazon Books
Null Hypothesis Examples

The null hypothesis (H 0 ) is the hypothesis that states there is no statistical difference between two sample sets. In other words, it assumes the independent variable does not have an effect on the dependent variable in a scientific experiment .
The null hypothesis is the most powerful type of hypothesis in the scientific method because it’s the easiest one to test with a high confidence level using statistics. If the null hypothesis is accepted, then it’s evidence any observed differences between two experiment groups are due to random chance. If the null hypothesis is rejected, then it’s strong evidence there is a true difference between test sets or that the independent variable affects the dependent variable.
- The null hypothesis is a nullifiable hypothesis. A researcher seeks to reject it because this result strongly indicates observed differences are real and not just due to chance.
- The null hypothesis may be accepted or rejected, but not proven. There is always a level of confidence in the outcome.
What Is the Null Hypothesis?
The null hypothesis is written as H 0 , which is read as H-zero, H-nought, or H-null. It is associated with another hypothesis, called the alternate or alternative hypothesis H A or H 1 . When the null hypothesis and alternate hypothesis are written mathematically, they cover all possible outcomes of an experiment.
An experimenter tests the null hypothesis with a statistical analysis called a significance test. The significance test determines the likelihood that the results of the test are not due to chance. Usually, a researcher uses a confidence level of 95% or 99% (p-value of 0.05 or 0.01). But, even if the confidence in the test is high, there is always a small chance the outcome is incorrect. This means you can’t prove a null hypothesis. It’s also a good reason why it’s important to repeat experiments.
Exact and Inexact Null Hypothesis
The most common type of null hypothesis assumes no difference between two samples or groups or no measurable effect of a treatment. This is the exact hypothesis . If you’re asked to state a null hypothesis for a science class, this is the one to write. It is the easiest type of hypothesis to test and is the only one accepted for certain types of analysis. Examples include:
There is no difference between two groups H 0 : μ 1 = μ 2 (where H 0 = the null hypothesis, μ 1 = the mean of population 1, and μ 2 = the mean of population 2)
Both groups have value of 100 (or any number or quality) H 0 : μ = 100
However, sometimes a researcher may test an inexact hypothesis . This type of hypothesis specifies ranges or intervals. Examples include:
Recovery time from a treatment is the same or worse than a placebo: H 0 : μ ≥ placebo time
There is a 5% or less difference between two groups: H 0 : 95 ≤ μ ≤ 105
An inexact hypothesis offers “directionality” about a phenomenon. For example, an exact hypothesis can indicate whether or not a treatment has an effect, while an inexact hypothesis can tell whether an effect is positive of negative. However, an inexact hypothesis may be harder to test and some scientists and statisticians disagree about whether it’s a true null hypothesis .
How to State the Null Hypothesis
To state the null hypothesis, first state what you expect the experiment to show. Then, rephrase the statement in a form that assumes there is no relationship between the variables or that a treatment has no effect.
Example: A researcher tests whether a new drug speeds recovery time from a certain disease. The average recovery time without treatment is 3 weeks.
- State the goal of the experiment: “I hope the average recovery time with the new drug will be less than 3 weeks.”
- Rephrase the hypothesis to assume the treatment has no effect: “If the drug doesn’t shorten recovery time, then the average time will be 3 weeks or longer.” Mathematically: H 0 : μ ≥ 3
This null hypothesis (inexact hypothesis) covers both the scenario in which the drug has no effect and the one in which the drugs makes the recovery time longer. The alternate hypothesis is that average recovery time will be less than three weeks:
H A : μ < 3
Of course, the researcher could test the no-effect hypothesis (exact null hypothesis): H 0 : μ = 3
The danger of testing this hypothesis is that rejecting it only implies the drug affected recovery time (not whether it made it better or worse). This is because the alternate hypothesis is:
H A : μ ≠ 3 (which includes μ <3 and μ >3)
Even though the no-effect null hypothesis yields less information, it’s used because it’s easier to test using statistics. Basically, testing whether something is unchanged/changed is easier than trying to quantify the nature of the change.
Remember, a researcher hopes to reject the null hypothesis because this supports the alternate hypothesis. Also, be sure the null and alternate hypothesis cover all outcomes. Finally, remember a simple true/false, equal/unequal, yes/no exact hypothesis is easier to test than a more complex inexact hypothesis.
Does chewing willow bark relieve pain? | Pain relief is the same compared with a . (exact) Pain relief after chewing willow bark is the same or worse versus taking a placebo. (inexact) | Pain relief is different compared with a placebo. (exact) Pain relief is better compared to a placebo. (inexact) |
Do cats care about the shape of their food? | Cats show no food preference based on shape. (exact) | Cat show a food preference based on shape. (exact) |
Do teens use mobile devices more than adults? | Teens and adults use mobile devices the same amount. (exact) Teens use mobile devices less than or equal to adults. (inexact) | Teens and adults used mobile devices different amounts. (exact) Teens use mobile devices more than adults. (inexact) |
Does the color of light influence plant growth? | The color of light has no effect on plant growth. (exact) | The color of light affects plant growth. (exact) |
- Adèr, H. J.; Mellenbergh, G. J. & Hand, D. J. (2007). Advising on Research Methods: A Consultant’s Companion . Huizen, The Netherlands: Johannes van Kessel Publishing. ISBN 978-90-79418-01-5 .
- Cox, D. R. (2006). Principles of Statistical Inference . Cambridge University Press. ISBN 978-0-521-68567-2 .
- Everitt, Brian (1998). The Cambridge Dictionary of Statistics . Cambridge, UK New York: Cambridge University Press. ISBN 978-0521593465.
- Weiss, Neil A. (1999). Introductory Statistics (5th ed.). ISBN 9780201598773.
Related Posts

Want to create or adapt books like this? Learn more about how Pressbooks supports open publishing practices.
Inferential Statistics
Learning Objectives
- Explain the purpose of null hypothesis testing, including the role of sampling error.
- Describe the basic logic of null hypothesis testing.
- Describe the role of relationship strength and sample size in determining statistical significance and make reasonable judgments about statistical significance based on these two factors.
The Purpose of Null Hypothesis Testing
As we have seen, psychological research typically involves measuring one or more variables in a sample and computing descriptive summary data (e.g., means, correlation coefficients) for those variables. These descriptive data for the sample are called statistics . In general, however, the researcher’s goal is not to draw conclusions about that sample but to draw conclusions about the population that the sample was selected from. Thus researchers must use sample statistics to draw conclusions about the corresponding values in the population. These corresponding values in the population are called parameters . Imagine, for example, that a researcher measures the number of depressive symptoms exhibited by each of 50 adults with clinical depression and computes the mean number of symptoms. The researcher probably wants to use this sample statistic (the mean number of symptoms for the sample) to draw conclusions about the corresponding population parameter (the mean number of symptoms for adults with clinical depression).
Unfortunately, sample statistics are not perfect estimates of their corresponding population parameters. This is because there is a certain amount of random variability in any statistic from sample to sample. The mean number of depressive symptoms might be 8.73 in one sample of adults with clinical depression, 6.45 in a second sample, and 9.44 in a third—even though these samples are selected randomly from the same population. Similarly, the correlation (Pearson’s r ) between two variables might be +.24 in one sample, −.04 in a second sample, and +.15 in a third—again, even though these samples are selected randomly from the same population. This random variability in a statistic from sample to sample is called sampling error . (Note that the term error here refers to random variability and does not imply that anyone has made a mistake. No one “commits a sampling error.”)
One implication of this is that when there is a statistical relationship in a sample, it is not always clear that there is a statistical relationship in the population. A small difference between two group means in a sample might indicate that there is a small difference between the two group means in the population. But it could also be that there is no difference between the means in the population and that the difference in the sample is just a matter of sampling error. Similarly, a Pearson’s r value of −.29 in a sample might mean that there is a negative relationship in the population. But it could also be that there is no relationship in the population and that the relationship in the sample is just a matter of sampling error.
In fact, any statistical relationship in a sample can be interpreted in two ways:
- There is a relationship in the population, and the relationship in the sample reflects this.
- There is no relationship in the population, and the relationship in the sample reflects only sampling error.
The purpose of null hypothesis testing is simply to help researchers decide between these two interpretations.
The Logic of Null Hypothesis Testing
Null hypothesis testing (often called null hypothesis significance testing or NHST) is a formal approach to deciding between two interpretations of a statistical relationship in a sample. One interpretation is called the null hypothesis (often symbolized H 0 and read as “H-zero”). This is the idea that there is no relationship in the population and that the relationship in the sample reflects only sampling error. Informally, the null hypothesis is that the sample relationship “occurred by chance.” The other interpretation is called the alternative hypothesis (often symbolized as H 1 ). This is the idea that there is a relationship in the population and that the relationship in the sample reflects this relationship in the population.
Again, every statistical relationship in a sample can be interpreted in either of these two ways: It might have occurred by chance, or it might reflect a relationship in the population. So researchers need a way to decide between them. Although there are many specific null hypothesis testing techniques, they are all based on the same general logic. The steps are as follows:
- Assume for the moment that the null hypothesis is true. There is no relationship between the variables in the population.
- Determine how likely the sample relationship would be if the null hypothesis were true.
- If the sample relationship would be extremely unlikely, then reject the null hypothesis in favor of the alternative hypothesis. If it would not be extremely unlikely, then retain the null hypothesis .
Following this logic, we can begin to understand why Mehl and his colleagues concluded that there is no difference in talkativeness between women and men in the population. In essence, they asked the following question: “If there were no difference in the population, how likely is it that we would find a small difference of d = 0.06 in our sample?” Their answer to this question was that this sample relationship would be fairly likely if the null hypothesis were true. Therefore, they retained the null hypothesis—concluding that there is no evidence of a sex difference in the population. We can also see why Kanner and his colleagues concluded that there is a correlation between hassles and symptoms in the population. They asked, “If the null hypothesis were true, how likely is it that we would find a strong correlation of +.60 in our sample?” Their answer to this question was that this sample relationship would be fairly unlikely if the null hypothesis were true. Therefore, they rejected the null hypothesis in favor of the alternative hypothesis—concluding that there is a positive correlation between these variables in the population.
A crucial step in null hypothesis testing is finding the probability of the sample result or a more extreme result if the null hypothesis were true (Lakens, 2017). [1] This probability is called the p value . A low p value means that the sample or more extreme result would be unlikely if the null hypothesis were true and leads to the rejection of the null hypothesis. A p value that is not low means that the sample or more extreme result would be likely if the null hypothesis were true and leads to the retention of the null hypothesis. But how low must the p value criterion be before the sample result is considered unlikely enough to reject the null hypothesis? In null hypothesis testing, this criterion is called α (alpha) and is almost always set to .05. If there is a 5% chance or less of a result at least as extreme as the sample result if the null hypothesis were true, then the null hypothesis is rejected. When this happens, the result is said to be statistically significant . If there is greater than a 5% chance of a result as extreme as the sample result when the null hypothesis is true, then the null hypothesis is retained. This does not necessarily mean that the researcher accepts the null hypothesis as true—only that there is not currently enough evidence to reject it. Researchers often use the expression “fail to reject the null hypothesis” rather than “retain the null hypothesis,” but they never use the expression “accept the null hypothesis.”
The Misunderstood p Value
The p value is one of the most misunderstood quantities in psychological research (Cohen, 1994) [2] . Even professional researchers misinterpret it, and it is not unusual for such misinterpretations to appear in statistics textbooks!
The most common misinterpretation is that the p value is the probability that the null hypothesis is true—that the sample result occurred by chance. For example, a misguided researcher might say that because the p value is .02, there is only a 2% chance that the result is due to chance and a 98% chance that it reflects a real relationship in the population. But this is incorrect . The p value is really the probability of a result at least as extreme as the sample result if the null hypothesis were true. So a p value of .02 means that if the null hypothesis were true, a sample result this extreme would occur only 2% of the time.
You can avoid this misunderstanding by remembering that the p value is not the probability that any particular hypothesis is true or false. Instead, it is the probability of obtaining the sample result if the null hypothesis were true.
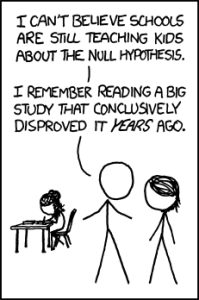
Role of Sample Size and Relationship Strength
Recall that null hypothesis testing involves answering the question, “If the null hypothesis were true, what is the probability of a sample result as extreme as this one?” In other words, “What is the p value?” It can be helpful to see that the answer to this question depends on just two considerations: the strength of the relationship and the size of the sample. Specifically, the stronger the sample relationship and the larger the sample, the less likely the result would be if the null hypothesis were true. That is, the lower the p value. This should make sense. Imagine a study in which a sample of 500 women is compared with a sample of 500 men in terms of some psychological characteristic, and Cohen’s d is a strong 0.50. If there were really no sex difference in the population, then a result this strong based on such a large sample should seem highly unlikely. Now imagine a similar study in which a sample of three women is compared with a sample of three men, and Cohen’s d is a weak 0.10. If there were no sex difference in the population, then a relationship this weak based on such a small sample should seem likely. And this is precisely why the null hypothesis would be rejected in the first example and retained in the second.
Of course, sometimes the result can be weak and the sample large, or the result can be strong and the sample small. In these cases, the two considerations trade off against each other so that a weak result can be statistically significant if the sample is large enough and a strong relationship can be statistically significant even if the sample is small. Table 13.1 shows roughly how relationship strength and sample size combine to determine whether a sample result is statistically significant. The columns of the table represent the three levels of relationship strength: weak, medium, and strong. The rows represent four sample sizes that can be considered small, medium, large, and extra large in the context of psychological research. Thus each cell in the table represents a combination of relationship strength and sample size. If a cell contains the word Yes , then this combination would be statistically significant for both Cohen’s d and Pearson’s r . If it contains the word No , then it would not be statistically significant for either. There is one cell where the decision for d and r would be different and another where it might be different depending on some additional considerations, which are discussed in Section 13.2 “Some Basic Null Hypothesis Tests”
Sample Size | Weak | Medium | Strong |
Small ( = 20) | No | No | = Maybe = Yes |
Medium ( = 50) | No | Yes | Yes |
Large ( = 100) | = Yes = No | Yes | Yes |
Extra large ( = 500) | Yes | Yes | Yes |
Although Table 13.1 provides only a rough guideline, it shows very clearly that weak relationships based on medium or small samples are never statistically significant and that strong relationships based on medium or larger samples are always statistically significant. If you keep this lesson in mind, you will often know whether a result is statistically significant based on the descriptive statistics alone. It is extremely useful to be able to develop this kind of intuitive judgment. One reason is that it allows you to develop expectations about how your formal null hypothesis tests are going to come out, which in turn allows you to detect problems in your analyses. For example, if your sample relationship is strong and your sample is medium, then you would expect to reject the null hypothesis. If for some reason your formal null hypothesis test indicates otherwise, then you need to double-check your computations and interpretations. A second reason is that the ability to make this kind of intuitive judgment is an indication that you understand the basic logic of this approach in addition to being able to do the computations.
Statistical Significance Versus Practical Significance
Table 13.1 illustrates another extremely important point. A statistically significant result is not necessarily a strong one. Even a very weak result can be statistically significant if it is based on a large enough sample. This is closely related to Janet Shibley Hyde’s argument about sex differences (Hyde, 2007) [3] . The differences between women and men in mathematical problem solving and leadership ability are statistically significant. But the word significant can cause people to interpret these differences as strong and important—perhaps even important enough to influence the college courses they take or even who they vote for. As we have seen, however, these statistically significant differences are actually quite weak—perhaps even “trivial.”
This is why it is important to distinguish between the statistical significance of a result and the practical significance of that result. Practical significance refers to the importance or usefulness of the result in some real-world context. Many sex differences are statistically significant—and may even be interesting for purely scientific reasons—but they are not practically significant. In clinical practice, this same concept is often referred to as “clinical significance.” For example, a study on a new treatment for social phobia might show that it produces a statistically significant positive effect. Yet this effect still might not be strong enough to justify the time, effort, and other costs of putting it into practice—especially if easier and cheaper treatments that work almost as well already exist. Although statistically significant, this result would be said to lack practical or clinical significance.
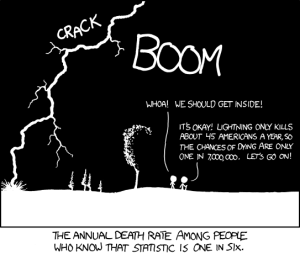
Image Description
“Null Hypothesis” long description: A comic depicting a man and a woman talking in the foreground. In the background is a child working at a desk. The man says to the woman, “I can’t believe schools are still teaching kids about the null hypothesis. I remember reading a big study that conclusively disproved it years ago.” [Return to “Null Hypothesis”]
“Conditional Risk” long description: A comic depicting two hikers beside a tree during a thunderstorm. A bolt of lightning goes “crack” in the dark sky as thunder booms. One of the hikers says, “Whoa! We should get inside!” The other hiker says, “It’s okay! Lightning only kills about 45 Americans a year, so the chances of dying are only one in 7,000,000. Let’s go on!” The comic’s caption says, “The annual death rate among people who know that statistic is one in six.” [Return to “Conditional Risk”]
Media Attributions
- Null Hypothesis by XKCD CC BY-NC (Attribution NonCommercial)
- Conditional Risk by XKCD CC BY-NC (Attribution NonCommercial)
- Lakens, D. (2017, December 25). About p -values: Understanding common misconceptions. [Blog post] Retrieved from https://correlaid.org/en/blog/understand-p-values/ ↵
- Cohen, J. (1994). The world is round: p < .05. American Psychologist, 49 , 997–1003. ↵
- Hyde, J. S. (2007). New directions in the study of gender similarities and differences. Current Directions in Psychological Science, 16 , 259–263. ↵
Descriptive data that involves measuring one or more variables in a sample and computing descriptive summary data (e.g., means, correlation coefficients) for those variables.
Corresponding values in the population.
The random variability in a statistic from sample to sample.
A formal approach to deciding between two interpretations of a statistical relationship in a sample.
The idea that there is no relationship in the population and that the relationship in the sample reflects only sampling error (often symbolized H0 and read as “H-zero”).
An alternative to the null hypothesis (often symbolized as H1), this hypothesis proposes that there is a relationship in the population and that the relationship in the sample reflects this relationship in the population.
A decision made by researchers using null hypothesis testing which occurs when the sample relationship would be extremely unlikely.
A decision made by researchers in null hypothesis testing which occurs when the sample relationship would not be extremely unlikely.
The probability of obtaining the sample result or a more extreme result if the null hypothesis were true.
The criterion that shows how low a p-value should be before the sample result is considered unlikely enough to reject the null hypothesis (Usually set to .05).
An effect that is unlikely due to random chance and therefore likely represents a real effect in the population.
Refers to the importance or usefulness of the result in some real-world context.
Research Methods in Psychology Copyright © 2019 by Rajiv S. Jhangiani, I-Chant A. Chiang, Carrie Cuttler, & Dana C. Leighton is licensed under a Creative Commons Attribution-NonCommercial-ShareAlike 4.0 International License , except where otherwise noted.
Share This Book
- PRO Courses Guides New Tech Help Pro Expert Videos About wikiHow Pro Upgrade Sign In
- EDIT Edit this Article
- EXPLORE Tech Help Pro About Us Random Article Quizzes Request a New Article Community Dashboard This Or That Game Happiness Hub Popular Categories Arts and Entertainment Artwork Books Movies Computers and Electronics Computers Phone Skills Technology Hacks Health Men's Health Mental Health Women's Health Relationships Dating Love Relationship Issues Hobbies and Crafts Crafts Drawing Games Education & Communication Communication Skills Personal Development Studying Personal Care and Style Fashion Hair Care Personal Hygiene Youth Personal Care School Stuff Dating All Categories Arts and Entertainment Finance and Business Home and Garden Relationship Quizzes Cars & Other Vehicles Food and Entertaining Personal Care and Style Sports and Fitness Computers and Electronics Health Pets and Animals Travel Education & Communication Hobbies and Crafts Philosophy and Religion Work World Family Life Holidays and Traditions Relationships Youth
- Browse Articles
- Learn Something New
- Quizzes Hot
- Happiness Hub
- This Or That Game
- Train Your Brain
- Explore More
- Support wikiHow
- About wikiHow
- Log in / Sign up
- Education and Communications
- College University and Postgraduate
- Academic Writing
Writing Null Hypotheses in Research and Statistics
Last Updated: September 2, 2024 Fact Checked
This article was co-authored by Joseph Quinones and by wikiHow staff writer, Jennifer Mueller, JD . Joseph Quinones is a Physics Teacher working at South Bronx Community Charter High School. Joseph specializes in astronomy and astrophysics and is interested in science education and science outreach, currently practicing ways to make physics accessible to more students with the goal of bringing more students of color into the STEM fields. He has experience working on Astrophysics research projects at the Museum of Natural History (AMNH). Joseph recieved his Bachelor's degree in Physics from Lehman College and his Masters in Physics Education from City College of New York (CCNY). He is also a member of a network called New York City Men Teach. There are 7 references cited in this article, which can be found at the bottom of the page. This article has been fact-checked, ensuring the accuracy of any cited facts and confirming the authority of its sources. This article has been viewed 29,836 times.
Are you working on a research project and struggling with how to write a null hypothesis? Well, you've come to the right place! Keep reading to learn everything you need to know about the null hypothesis, including a review of what it is, how it relates to your research question and your alternative hypothesis, as well as how to use it in different types of studies.
Things You Should Know
- Write a research null hypothesis as a statement that the studied variables have no relationship to each other, or that there's no difference between 2 groups.
- Adjust the format of your null hypothesis to match the statistical method you used to test it, such as using "mean" if you're comparing the mean between 2 groups.
What is a null hypothesis?

- Research hypothesis: States in plain language that there's no relationship between the 2 variables or there's no difference between the 2 groups being studied.
- Statistical hypothesis: States the predicted outcome of statistical analysis through a mathematical equation related to the statistical method you're using.
Examples of Null Hypotheses

Null Hypothesis vs. Alternative Hypothesis

- For example, your alternative hypothesis could state a positive correlation between 2 variables while your null hypothesis states there's no relationship. If there's a negative correlation, then both hypotheses are false.

- You need additional data or evidence to show that your alternative hypothesis is correct—proving the null hypothesis false is just the first step.
- In smaller studies, sometimes it's enough to show that there's some relationship and your hypothesis could be correct—you can leave the additional proof as an open question for other researchers to tackle.
How do I test a null hypothesis?

- Group means: Compare the mean of the variable in your sample with the mean of the variable in the general population. [6] X Research source
- Group proportions: Compare the proportion of the variable in your sample with the proportion of the variable in the general population. [7] X Research source
- Correlation: Correlation analysis looks at the relationship between 2 variables—specifically, whether they tend to happen together. [8] X Research source
- Regression: Regression analysis reveals the correlation between 2 variables while also controlling for the effect of other, interrelated variables. [9] X Research source
Templates for Null Hypotheses

- Research null hypothesis: There is no difference in the mean [dependent variable] between [group 1] and [group 2].
- Research null hypothesis: The proportion of [dependent variable] in [group 1] and [group 2] is the same.
- Research null hypothesis: There is no correlation between [independent variable] and [dependent variable] in the population.
- Research null hypothesis: There is no relationship between [independent variable] and [dependent variable] in the population.
Expert Q&A

You Might Also Like

Expert Interview

Thanks for reading our article! If you’d like to learn more about physics, check out our in-depth interview with Joseph Quinones .
- ↑ https://online.stat.psu.edu/stat100/lesson/10/10.1
- ↑ https://online.stat.psu.edu/stat501/lesson/2/2.12
- ↑ https://support.minitab.com/en-us/minitab/21/help-and-how-to/statistics/basic-statistics/supporting-topics/basics/null-and-alternative-hypotheses/
- ↑ https://www.ncbi.nlm.nih.gov/pmc/articles/PMC5635437/
- ↑ https://online.stat.psu.edu/statprogram/reviews/statistical-concepts/hypothesis-testing
- ↑ https://education.arcus.chop.edu/null-hypothesis-testing/
- ↑ https://sphweb.bumc.bu.edu/otlt/mph-modules/bs/bs704_hypothesistest-means-proportions/bs704_hypothesistest-means-proportions_print.html
About This Article

- Send fan mail to authors
Reader Success Stories

Dec 3, 2022
Did this article help you?

Featured Articles

Trending Articles

Watch Articles

- Terms of Use
- Privacy Policy
- Do Not Sell or Share My Info
- Not Selling Info
Don’t miss out! Sign up for
wikiHow’s newsletter
Frequently asked questions
What are null and alternative hypotheses.
Null and alternative hypotheses are used in statistical hypothesis testing . The null hypothesis of a test always predicts no effect or no relationship between variables, while the alternative hypothesis states your research prediction of an effect or relationship.
Frequently asked questions: Statistics
As the degrees of freedom increase, Student’s t distribution becomes less leptokurtic , meaning that the probability of extreme values decreases. The distribution becomes more and more similar to a standard normal distribution .
The three categories of kurtosis are:
- Mesokurtosis : An excess kurtosis of 0. Normal distributions are mesokurtic.
- Platykurtosis : A negative excess kurtosis. Platykurtic distributions are thin-tailed, meaning that they have few outliers .
- Leptokurtosis : A positive excess kurtosis. Leptokurtic distributions are fat-tailed, meaning that they have many outliers.
Probability distributions belong to two broad categories: discrete probability distributions and continuous probability distributions . Within each category, there are many types of probability distributions.
Probability is the relative frequency over an infinite number of trials.
For example, the probability of a coin landing on heads is .5, meaning that if you flip the coin an infinite number of times, it will land on heads half the time.
Since doing something an infinite number of times is impossible, relative frequency is often used as an estimate of probability. If you flip a coin 1000 times and get 507 heads, the relative frequency, .507, is a good estimate of the probability.
Categorical variables can be described by a frequency distribution. Quantitative variables can also be described by a frequency distribution, but first they need to be grouped into interval classes .
A histogram is an effective way to tell if a frequency distribution appears to have a normal distribution .
Plot a histogram and look at the shape of the bars. If the bars roughly follow a symmetrical bell or hill shape, like the example below, then the distribution is approximately normally distributed.
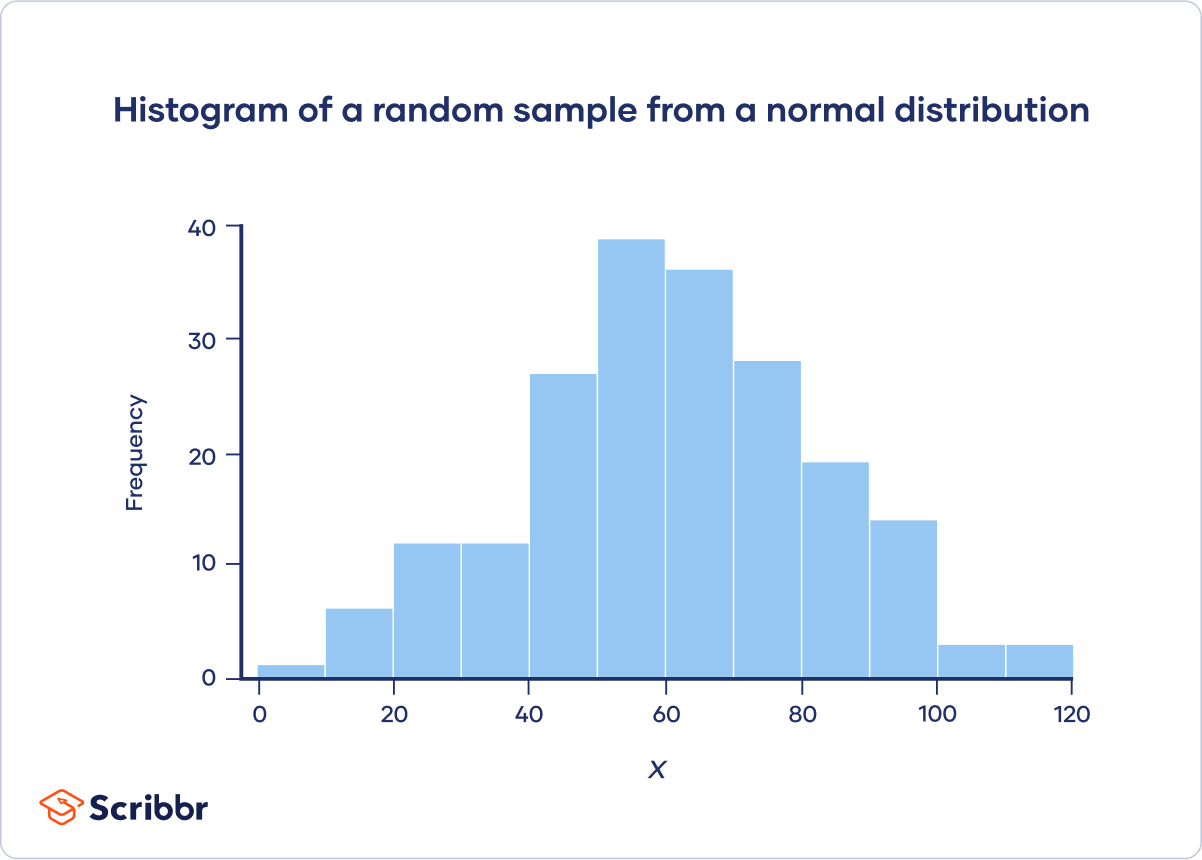
You can use the CHISQ.INV.RT() function to find a chi-square critical value in Excel.
For example, to calculate the chi-square critical value for a test with df = 22 and α = .05, click any blank cell and type:
=CHISQ.INV.RT(0.05,22)
You can use the qchisq() function to find a chi-square critical value in R.
For example, to calculate the chi-square critical value for a test with df = 22 and α = .05:
qchisq(p = .05, df = 22, lower.tail = FALSE)
You can use the chisq.test() function to perform a chi-square test of independence in R. Give the contingency table as a matrix for the “x” argument. For example:
m = matrix(data = c(89, 84, 86, 9, 8, 24), nrow = 3, ncol = 2)
chisq.test(x = m)
You can use the CHISQ.TEST() function to perform a chi-square test of independence in Excel. It takes two arguments, CHISQ.TEST(observed_range, expected_range), and returns the p value.
Chi-square goodness of fit tests are often used in genetics. One common application is to check if two genes are linked (i.e., if the assortment is independent). When genes are linked, the allele inherited for one gene affects the allele inherited for another gene.
Suppose that you want to know if the genes for pea texture (R = round, r = wrinkled) and color (Y = yellow, y = green) are linked. You perform a dihybrid cross between two heterozygous ( RY / ry ) pea plants. The hypotheses you’re testing with your experiment are:
- This would suggest that the genes are unlinked.
- This would suggest that the genes are linked.
You observe 100 peas:
- 78 round and yellow peas
- 6 round and green peas
- 4 wrinkled and yellow peas
- 12 wrinkled and green peas
Step 1: Calculate the expected frequencies
To calculate the expected values, you can make a Punnett square. If the two genes are unlinked, the probability of each genotypic combination is equal.
RRYY | RrYy | RRYy | RrYY | |
RrYy | rryy | Rryy | rrYy | |
RRYy | Rryy | RRyy | RrYy | |
RrYY | rrYy | RrYy | rrYY |
The expected phenotypic ratios are therefore 9 round and yellow: 3 round and green: 3 wrinkled and yellow: 1 wrinkled and green.
From this, you can calculate the expected phenotypic frequencies for 100 peas:
Round and yellow | 78 | 100 * (9/16) = 56.25 |
Round and green | 6 | 100 * (3/16) = 18.75 |
Wrinkled and yellow | 4 | 100 * (3/16) = 18.75 |
Wrinkled and green | 12 | 100 * (1/16) = 6.21 |
Step 2: Calculate chi-square
− | − | ||||
Round and yellow | 78 | 56.25 | 21.75 | 473.06 | 8.41 |
Round and green | 6 | 18.75 | −12.75 | 162.56 | 8.67 |
Wrinkled and yellow | 4 | 18.75 | −14.75 | 217.56 | 11.6 |
Wrinkled and green | 12 | 6.21 | 5.79 | 33.52 | 5.4 |
Χ 2 = 8.41 + 8.67 + 11.6 + 5.4 = 34.08
Step 3: Find the critical chi-square value
Since there are four groups (round and yellow, round and green, wrinkled and yellow, wrinkled and green), there are three degrees of freedom .
For a test of significance at α = .05 and df = 3, the Χ 2 critical value is 7.82.
Step 4: Compare the chi-square value to the critical value
Χ 2 = 34.08
Critical value = 7.82
The Χ 2 value is greater than the critical value .
Step 5: Decide whether the reject the null hypothesis
The Χ 2 value is greater than the critical value, so we reject the null hypothesis that the population of offspring have an equal probability of inheriting all possible genotypic combinations. There is a significant difference between the observed and expected genotypic frequencies ( p < .05).
The data supports the alternative hypothesis that the offspring do not have an equal probability of inheriting all possible genotypic combinations, which suggests that the genes are linked
You can use the chisq.test() function to perform a chi-square goodness of fit test in R. Give the observed values in the “x” argument, give the expected values in the “p” argument, and set “rescale.p” to true. For example:
chisq.test(x = c(22,30,23), p = c(25,25,25), rescale.p = TRUE)
You can use the CHISQ.TEST() function to perform a chi-square goodness of fit test in Excel. It takes two arguments, CHISQ.TEST(observed_range, expected_range), and returns the p value .
Both correlations and chi-square tests can test for relationships between two variables. However, a correlation is used when you have two quantitative variables and a chi-square test of independence is used when you have two categorical variables.
Both chi-square tests and t tests can test for differences between two groups. However, a t test is used when you have a dependent quantitative variable and an independent categorical variable (with two groups). A chi-square test of independence is used when you have two categorical variables.
The two main chi-square tests are the chi-square goodness of fit test and the chi-square test of independence .
A chi-square distribution is a continuous probability distribution . The shape of a chi-square distribution depends on its degrees of freedom , k . The mean of a chi-square distribution is equal to its degrees of freedom ( k ) and the variance is 2 k . The range is 0 to ∞.
As the degrees of freedom ( k ) increases, the chi-square distribution goes from a downward curve to a hump shape. As the degrees of freedom increases further, the hump goes from being strongly right-skewed to being approximately normal.
To find the quartiles of a probability distribution, you can use the distribution’s quantile function.
You can use the quantile() function to find quartiles in R. If your data is called “data”, then “quantile(data, prob=c(.25,.5,.75), type=1)” will return the three quartiles.
You can use the QUARTILE() function to find quartiles in Excel. If your data is in column A, then click any blank cell and type “=QUARTILE(A:A,1)” for the first quartile, “=QUARTILE(A:A,2)” for the second quartile, and “=QUARTILE(A:A,3)” for the third quartile.
You can use the PEARSON() function to calculate the Pearson correlation coefficient in Excel. If your variables are in columns A and B, then click any blank cell and type “PEARSON(A:A,B:B)”.
There is no function to directly test the significance of the correlation.
You can use the cor() function to calculate the Pearson correlation coefficient in R. To test the significance of the correlation, you can use the cor.test() function.
You should use the Pearson correlation coefficient when (1) the relationship is linear and (2) both variables are quantitative and (3) normally distributed and (4) have no outliers.
The Pearson correlation coefficient ( r ) is the most common way of measuring a linear correlation. It is a number between –1 and 1 that measures the strength and direction of the relationship between two variables.
This table summarizes the most important differences between normal distributions and Poisson distributions :
Characteristic | Normal | Poisson |
---|---|---|
Continuous | ||
Mean (µ) and standard deviation (σ) | Lambda (λ) | |
Shape | Bell-shaped | Depends on λ |
Symmetrical | Asymmetrical (right-skewed). As λ increases, the asymmetry decreases. | |
Range | −∞ to ∞ | 0 to ∞ |
When the mean of a Poisson distribution is large (>10), it can be approximated by a normal distribution.
In the Poisson distribution formula, lambda (λ) is the mean number of events within a given interval of time or space. For example, λ = 0.748 floods per year.
The e in the Poisson distribution formula stands for the number 2.718. This number is called Euler’s constant. You can simply substitute e with 2.718 when you’re calculating a Poisson probability. Euler’s constant is a very useful number and is especially important in calculus.
The three types of skewness are:
- Right skew (also called positive skew ) . A right-skewed distribution is longer on the right side of its peak than on its left.
- Left skew (also called negative skew). A left-skewed distribution is longer on the left side of its peak than on its right.
- Zero skew. It is symmetrical and its left and right sides are mirror images.
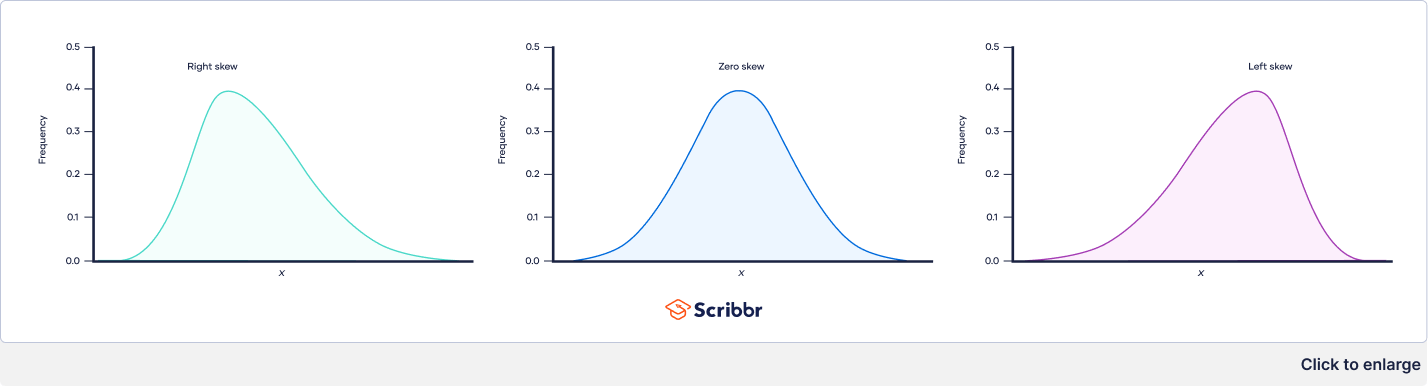
Skewness and kurtosis are both important measures of a distribution’s shape.
- Skewness measures the asymmetry of a distribution.
- Kurtosis measures the heaviness of a distribution’s tails relative to a normal distribution .
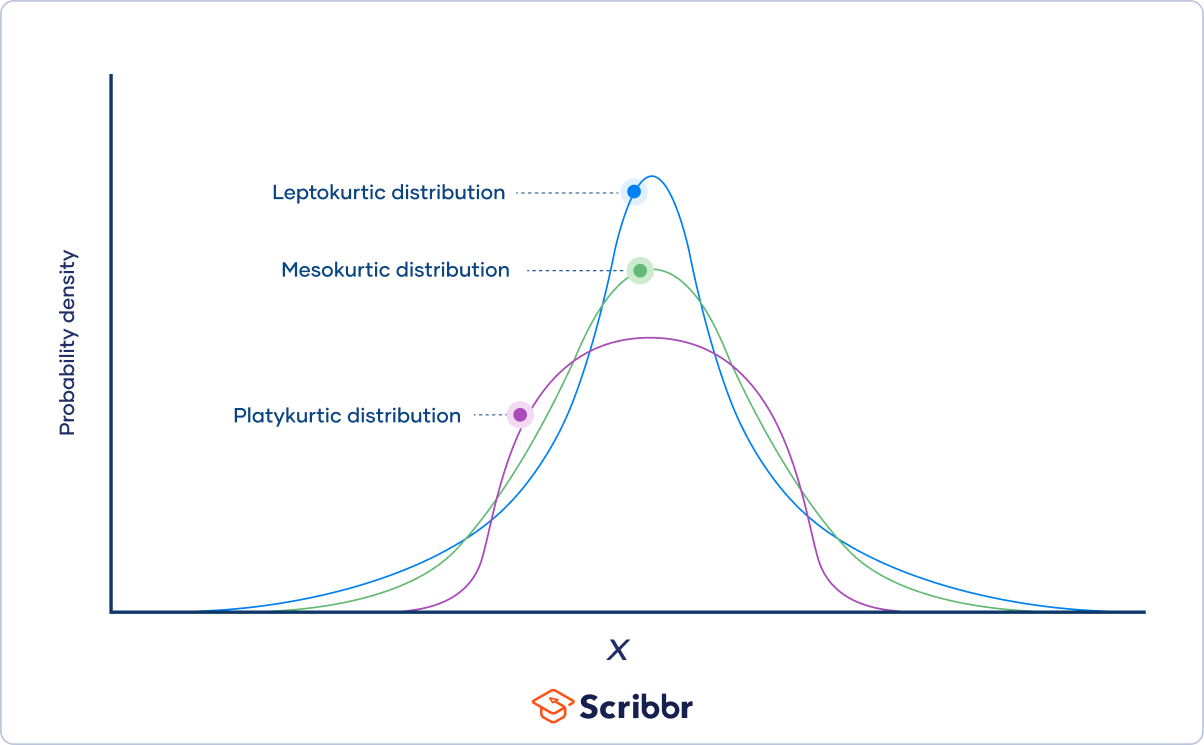
A research hypothesis is your proposed answer to your research question. The research hypothesis usually includes an explanation (“ x affects y because …”).
A statistical hypothesis, on the other hand, is a mathematical statement about a population parameter. Statistical hypotheses always come in pairs: the null and alternative hypotheses . In a well-designed study , the statistical hypotheses correspond logically to the research hypothesis.
The alternative hypothesis is often abbreviated as H a or H 1 . When the alternative hypothesis is written using mathematical symbols, it always includes an inequality symbol (usually ≠, but sometimes < or >).
The null hypothesis is often abbreviated as H 0 . When the null hypothesis is written using mathematical symbols, it always includes an equality symbol (usually =, but sometimes ≥ or ≤).
The t distribution was first described by statistician William Sealy Gosset under the pseudonym “Student.”
To calculate a confidence interval of a mean using the critical value of t , follow these four steps:
- Choose the significance level based on your desired confidence level. The most common confidence level is 95%, which corresponds to α = .05 in the two-tailed t table .
- Find the critical value of t in the two-tailed t table.
- Multiply the critical value of t by s / √ n .
- Add this value to the mean to calculate the upper limit of the confidence interval, and subtract this value from the mean to calculate the lower limit.
To test a hypothesis using the critical value of t , follow these four steps:
- Calculate the t value for your sample.
- Find the critical value of t in the t table .
- Determine if the (absolute) t value is greater than the critical value of t .
- Reject the null hypothesis if the sample’s t value is greater than the critical value of t . Otherwise, don’t reject the null hypothesis .
You can use the T.INV() function to find the critical value of t for one-tailed tests in Excel, and you can use the T.INV.2T() function for two-tailed tests.
You can use the qt() function to find the critical value of t in R. The function gives the critical value of t for the one-tailed test. If you want the critical value of t for a two-tailed test, divide the significance level by two.
You can use the RSQ() function to calculate R² in Excel. If your dependent variable is in column A and your independent variable is in column B, then click any blank cell and type “RSQ(A:A,B:B)”.
You can use the summary() function to view the R² of a linear model in R. You will see the “R-squared” near the bottom of the output.
There are two formulas you can use to calculate the coefficient of determination (R²) of a simple linear regression .

The coefficient of determination (R²) is a number between 0 and 1 that measures how well a statistical model predicts an outcome. You can interpret the R² as the proportion of variation in the dependent variable that is predicted by the statistical model.
There are three main types of missing data .
Missing completely at random (MCAR) data are randomly distributed across the variable and unrelated to other variables .
Missing at random (MAR) data are not randomly distributed but they are accounted for by other observed variables.
Missing not at random (MNAR) data systematically differ from the observed values.
To tidy up your missing data , your options usually include accepting, removing, or recreating the missing data.
- Acceptance: You leave your data as is
- Listwise or pairwise deletion: You delete all cases (participants) with missing data from analyses
- Imputation: You use other data to fill in the missing data
Missing data are important because, depending on the type, they can sometimes bias your results. This means your results may not be generalizable outside of your study because your data come from an unrepresentative sample .
Missing data , or missing values, occur when you don’t have data stored for certain variables or participants.
In any dataset, there’s usually some missing data. In quantitative research , missing values appear as blank cells in your spreadsheet.
There are two steps to calculating the geometric mean :
- Multiply all values together to get their product.
- Find the n th root of the product ( n is the number of values).
Before calculating the geometric mean, note that:
- The geometric mean can only be found for positive values.
- If any value in the data set is zero, the geometric mean is zero.
The arithmetic mean is the most commonly used type of mean and is often referred to simply as “the mean.” While the arithmetic mean is based on adding and dividing values, the geometric mean multiplies and finds the root of values.
Even though the geometric mean is a less common measure of central tendency , it’s more accurate than the arithmetic mean for percentage change and positively skewed data. The geometric mean is often reported for financial indices and population growth rates.
The geometric mean is an average that multiplies all values and finds a root of the number. For a dataset with n numbers, you find the n th root of their product.
Outliers are extreme values that differ from most values in the dataset. You find outliers at the extreme ends of your dataset.
It’s best to remove outliers only when you have a sound reason for doing so.
Some outliers represent natural variations in the population , and they should be left as is in your dataset. These are called true outliers.
Other outliers are problematic and should be removed because they represent measurement errors , data entry or processing errors, or poor sampling.
You can choose from four main ways to detect outliers :
- Sorting your values from low to high and checking minimum and maximum values
- Visualizing your data with a box plot and looking for outliers
- Using the interquartile range to create fences for your data
- Using statistical procedures to identify extreme values
Outliers can have a big impact on your statistical analyses and skew the results of any hypothesis test if they are inaccurate.
These extreme values can impact your statistical power as well, making it hard to detect a true effect if there is one.
No, the steepness or slope of the line isn’t related to the correlation coefficient value. The correlation coefficient only tells you how closely your data fit on a line, so two datasets with the same correlation coefficient can have very different slopes.
To find the slope of the line, you’ll need to perform a regression analysis .
Correlation coefficients always range between -1 and 1.
The sign of the coefficient tells you the direction of the relationship: a positive value means the variables change together in the same direction, while a negative value means they change together in opposite directions.
The absolute value of a number is equal to the number without its sign. The absolute value of a correlation coefficient tells you the magnitude of the correlation: the greater the absolute value, the stronger the correlation.
These are the assumptions your data must meet if you want to use Pearson’s r :
- Both variables are on an interval or ratio level of measurement
- Data from both variables follow normal distributions
- Your data have no outliers
- Your data is from a random or representative sample
- You expect a linear relationship between the two variables
A correlation coefficient is a single number that describes the strength and direction of the relationship between your variables.
Different types of correlation coefficients might be appropriate for your data based on their levels of measurement and distributions . The Pearson product-moment correlation coefficient (Pearson’s r ) is commonly used to assess a linear relationship between two quantitative variables.
There are various ways to improve power:
- Increase the potential effect size by manipulating your independent variable more strongly,
- Increase sample size,
- Increase the significance level (alpha),
- Reduce measurement error by increasing the precision and accuracy of your measurement devices and procedures,
- Use a one-tailed test instead of a two-tailed test for t tests and z tests.
A power analysis is a calculation that helps you determine a minimum sample size for your study. It’s made up of four main components. If you know or have estimates for any three of these, you can calculate the fourth component.
- Statistical power : the likelihood that a test will detect an effect of a certain size if there is one, usually set at 80% or higher.
- Sample size : the minimum number of observations needed to observe an effect of a certain size with a given power level.
- Significance level (alpha) : the maximum risk of rejecting a true null hypothesis that you are willing to take, usually set at 5%.
- Expected effect size : a standardized way of expressing the magnitude of the expected result of your study, usually based on similar studies or a pilot study.
Statistical analysis is the main method for analyzing quantitative research data . It uses probabilities and models to test predictions about a population from sample data.
The risk of making a Type II error is inversely related to the statistical power of a test. Power is the extent to which a test can correctly detect a real effect when there is one.
To (indirectly) reduce the risk of a Type II error, you can increase the sample size or the significance level to increase statistical power.
The risk of making a Type I error is the significance level (or alpha) that you choose. That’s a value that you set at the beginning of your study to assess the statistical probability of obtaining your results ( p value ).
The significance level is usually set at 0.05 or 5%. This means that your results only have a 5% chance of occurring, or less, if the null hypothesis is actually true.
To reduce the Type I error probability, you can set a lower significance level.
In statistics, a Type I error means rejecting the null hypothesis when it’s actually true, while a Type II error means failing to reject the null hypothesis when it’s actually false.
In statistics, power refers to the likelihood of a hypothesis test detecting a true effect if there is one. A statistically powerful test is more likely to reject a false negative (a Type II error).
If you don’t ensure enough power in your study, you may not be able to detect a statistically significant result even when it has practical significance. Your study might not have the ability to answer your research question.
While statistical significance shows that an effect exists in a study, practical significance shows that the effect is large enough to be meaningful in the real world.
Statistical significance is denoted by p -values whereas practical significance is represented by effect sizes .
There are dozens of measures of effect sizes . The most common effect sizes are Cohen’s d and Pearson’s r . Cohen’s d measures the size of the difference between two groups while Pearson’s r measures the strength of the relationship between two variables .
Effect size tells you how meaningful the relationship between variables or the difference between groups is.
A large effect size means that a research finding has practical significance, while a small effect size indicates limited practical applications.
Using descriptive and inferential statistics , you can make two types of estimates about the population : point estimates and interval estimates.
- A point estimate is a single value estimate of a parameter . For instance, a sample mean is a point estimate of a population mean.
- An interval estimate gives you a range of values where the parameter is expected to lie. A confidence interval is the most common type of interval estimate.
Both types of estimates are important for gathering a clear idea of where a parameter is likely to lie.
Standard error and standard deviation are both measures of variability . The standard deviation reflects variability within a sample, while the standard error estimates the variability across samples of a population.
The standard error of the mean , or simply standard error , indicates how different the population mean is likely to be from a sample mean. It tells you how much the sample mean would vary if you were to repeat a study using new samples from within a single population.
To figure out whether a given number is a parameter or a statistic , ask yourself the following:
- Does the number describe a whole, complete population where every member can be reached for data collection ?
- Is it possible to collect data for this number from every member of the population in a reasonable time frame?
If the answer is yes to both questions, the number is likely to be a parameter. For small populations, data can be collected from the whole population and summarized in parameters.
If the answer is no to either of the questions, then the number is more likely to be a statistic.
The arithmetic mean is the most commonly used mean. It’s often simply called the mean or the average. But there are some other types of means you can calculate depending on your research purposes:
- Weighted mean: some values contribute more to the mean than others.
- Geometric mean : values are multiplied rather than summed up.
- Harmonic mean: reciprocals of values are used instead of the values themselves.
You can find the mean , or average, of a data set in two simple steps:
- Find the sum of the values by adding them all up.
- Divide the sum by the number of values in the data set.
This method is the same whether you are dealing with sample or population data or positive or negative numbers.
The median is the most informative measure of central tendency for skewed distributions or distributions with outliers. For example, the median is often used as a measure of central tendency for income distributions, which are generally highly skewed.
Because the median only uses one or two values, it’s unaffected by extreme outliers or non-symmetric distributions of scores. In contrast, the mean and mode can vary in skewed distributions.
To find the median , first order your data. Then calculate the middle position based on n , the number of values in your data set.

A data set can often have no mode, one mode or more than one mode – it all depends on how many different values repeat most frequently.
Your data can be:
- without any mode
- unimodal, with one mode,
- bimodal, with two modes,
- trimodal, with three modes, or
- multimodal, with four or more modes.
To find the mode :
- If your data is numerical or quantitative, order the values from low to high.
- If it is categorical, sort the values by group, in any order.
Then you simply need to identify the most frequently occurring value.
The interquartile range is the best measure of variability for skewed distributions or data sets with outliers. Because it’s based on values that come from the middle half of the distribution, it’s unlikely to be influenced by outliers .
The two most common methods for calculating interquartile range are the exclusive and inclusive methods.
The exclusive method excludes the median when identifying Q1 and Q3, while the inclusive method includes the median as a value in the data set in identifying the quartiles.
For each of these methods, you’ll need different procedures for finding the median, Q1 and Q3 depending on whether your sample size is even- or odd-numbered. The exclusive method works best for even-numbered sample sizes, while the inclusive method is often used with odd-numbered sample sizes.
While the range gives you the spread of the whole data set, the interquartile range gives you the spread of the middle half of a data set.
Homoscedasticity, or homogeneity of variances, is an assumption of equal or similar variances in different groups being compared.
This is an important assumption of parametric statistical tests because they are sensitive to any dissimilarities. Uneven variances in samples result in biased and skewed test results.
Statistical tests such as variance tests or the analysis of variance (ANOVA) use sample variance to assess group differences of populations. They use the variances of the samples to assess whether the populations they come from significantly differ from each other.
Variance is the average squared deviations from the mean, while standard deviation is the square root of this number. Both measures reflect variability in a distribution, but their units differ:
- Standard deviation is expressed in the same units as the original values (e.g., minutes or meters).
- Variance is expressed in much larger units (e.g., meters squared).
Although the units of variance are harder to intuitively understand, variance is important in statistical tests .
The empirical rule, or the 68-95-99.7 rule, tells you where most of the values lie in a normal distribution :
- Around 68% of values are within 1 standard deviation of the mean.
- Around 95% of values are within 2 standard deviations of the mean.
- Around 99.7% of values are within 3 standard deviations of the mean.
The empirical rule is a quick way to get an overview of your data and check for any outliers or extreme values that don’t follow this pattern.
In a normal distribution , data are symmetrically distributed with no skew. Most values cluster around a central region, with values tapering off as they go further away from the center.
The measures of central tendency (mean, mode, and median) are exactly the same in a normal distribution.
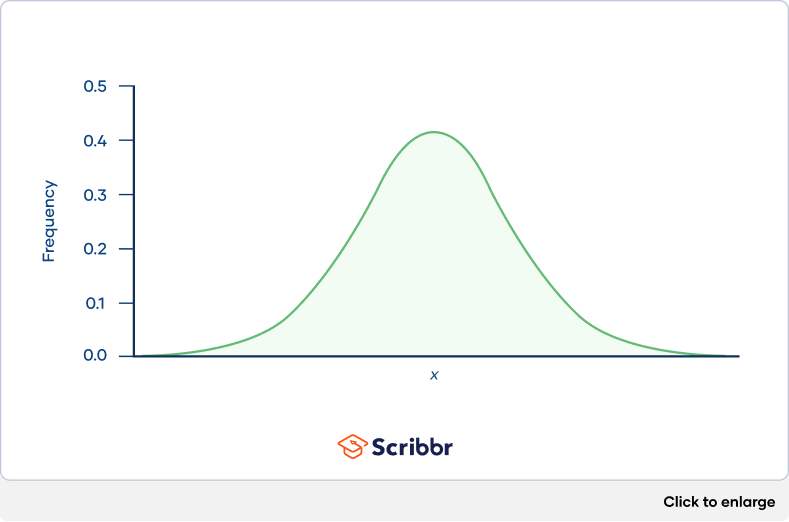
The standard deviation is the average amount of variability in your data set. It tells you, on average, how far each score lies from the mean .
In normal distributions, a high standard deviation means that values are generally far from the mean, while a low standard deviation indicates that values are clustered close to the mean.
No. Because the range formula subtracts the lowest number from the highest number, the range is always zero or a positive number.
In statistics, the range is the spread of your data from the lowest to the highest value in the distribution. It is the simplest measure of variability .
While central tendency tells you where most of your data points lie, variability summarizes how far apart your points from each other.
Data sets can have the same central tendency but different levels of variability or vice versa . Together, they give you a complete picture of your data.
Variability is most commonly measured with the following descriptive statistics :
- Range : the difference between the highest and lowest values
- Interquartile range : the range of the middle half of a distribution
- Standard deviation : average distance from the mean
- Variance : average of squared distances from the mean
Variability tells you how far apart points lie from each other and from the center of a distribution or a data set.
Variability is also referred to as spread, scatter or dispersion.
While interval and ratio data can both be categorized, ranked, and have equal spacing between adjacent values, only ratio scales have a true zero.
For example, temperature in Celsius or Fahrenheit is at an interval scale because zero is not the lowest possible temperature. In the Kelvin scale, a ratio scale, zero represents a total lack of thermal energy.
A critical value is the value of the test statistic which defines the upper and lower bounds of a confidence interval , or which defines the threshold of statistical significance in a statistical test. It describes how far from the mean of the distribution you have to go to cover a certain amount of the total variation in the data (i.e. 90%, 95%, 99%).
If you are constructing a 95% confidence interval and are using a threshold of statistical significance of p = 0.05, then your critical value will be identical in both cases.
The t -distribution gives more probability to observations in the tails of the distribution than the standard normal distribution (a.k.a. the z -distribution).
In this way, the t -distribution is more conservative than the standard normal distribution: to reach the same level of confidence or statistical significance , you will need to include a wider range of the data.
A t -score (a.k.a. a t -value) is equivalent to the number of standard deviations away from the mean of the t -distribution .
The t -score is the test statistic used in t -tests and regression tests. It can also be used to describe how far from the mean an observation is when the data follow a t -distribution.
The t -distribution is a way of describing a set of observations where most observations fall close to the mean , and the rest of the observations make up the tails on either side. It is a type of normal distribution used for smaller sample sizes, where the variance in the data is unknown.
The t -distribution forms a bell curve when plotted on a graph. It can be described mathematically using the mean and the standard deviation .
In statistics, ordinal and nominal variables are both considered categorical variables .
Even though ordinal data can sometimes be numerical, not all mathematical operations can be performed on them.
Ordinal data has two characteristics:
- The data can be classified into different categories within a variable.
- The categories have a natural ranked order.
However, unlike with interval data, the distances between the categories are uneven or unknown.
Nominal and ordinal are two of the four levels of measurement . Nominal level data can only be classified, while ordinal level data can be classified and ordered.
Nominal data is data that can be labelled or classified into mutually exclusive categories within a variable. These categories cannot be ordered in a meaningful way.
For example, for the nominal variable of preferred mode of transportation, you may have the categories of car, bus, train, tram or bicycle.
If your confidence interval for a difference between groups includes zero, that means that if you run your experiment again you have a good chance of finding no difference between groups.
If your confidence interval for a correlation or regression includes zero, that means that if you run your experiment again there is a good chance of finding no correlation in your data.
In both of these cases, you will also find a high p -value when you run your statistical test, meaning that your results could have occurred under the null hypothesis of no relationship between variables or no difference between groups.
If you want to calculate a confidence interval around the mean of data that is not normally distributed , you have two choices:
- Find a distribution that matches the shape of your data and use that distribution to calculate the confidence interval.
- Perform a transformation on your data to make it fit a normal distribution, and then find the confidence interval for the transformed data.
The standard normal distribution , also called the z -distribution, is a special normal distribution where the mean is 0 and the standard deviation is 1.
Any normal distribution can be converted into the standard normal distribution by turning the individual values into z -scores. In a z -distribution, z -scores tell you how many standard deviations away from the mean each value lies.
The z -score and t -score (aka z -value and t -value) show how many standard deviations away from the mean of the distribution you are, assuming your data follow a z -distribution or a t -distribution .
These scores are used in statistical tests to show how far from the mean of the predicted distribution your statistical estimate is. If your test produces a z -score of 2.5, this means that your estimate is 2.5 standard deviations from the predicted mean.
The predicted mean and distribution of your estimate are generated by the null hypothesis of the statistical test you are using. The more standard deviations away from the predicted mean your estimate is, the less likely it is that the estimate could have occurred under the null hypothesis .
To calculate the confidence interval , you need to know:
- The point estimate you are constructing the confidence interval for
- The critical values for the test statistic
- The standard deviation of the sample
- The sample size
Then you can plug these components into the confidence interval formula that corresponds to your data. The formula depends on the type of estimate (e.g. a mean or a proportion) and on the distribution of your data.
The confidence level is the percentage of times you expect to get close to the same estimate if you run your experiment again or resample the population in the same way.
The confidence interval consists of the upper and lower bounds of the estimate you expect to find at a given level of confidence.
For example, if you are estimating a 95% confidence interval around the mean proportion of female babies born every year based on a random sample of babies, you might find an upper bound of 0.56 and a lower bound of 0.48. These are the upper and lower bounds of the confidence interval. The confidence level is 95%.
The mean is the most frequently used measure of central tendency because it uses all values in the data set to give you an average.
For data from skewed distributions, the median is better than the mean because it isn’t influenced by extremely large values.
The mode is the only measure you can use for nominal or categorical data that can’t be ordered.
The measures of central tendency you can use depends on the level of measurement of your data.
- For a nominal level, you can only use the mode to find the most frequent value.
- For an ordinal level or ranked data, you can also use the median to find the value in the middle of your data set.
- For interval or ratio levels, in addition to the mode and median, you can use the mean to find the average value.
Measures of central tendency help you find the middle, or the average, of a data set.
The 3 most common measures of central tendency are the mean, median and mode.
- The mode is the most frequent value.
- The median is the middle number in an ordered data set.
- The mean is the sum of all values divided by the total number of values.
Some variables have fixed levels. For example, gender and ethnicity are always nominal level data because they cannot be ranked.
However, for other variables, you can choose the level of measurement . For example, income is a variable that can be recorded on an ordinal or a ratio scale:
- At an ordinal level , you could create 5 income groupings and code the incomes that fall within them from 1–5.
- At a ratio level , you would record exact numbers for income.
If you have a choice, the ratio level is always preferable because you can analyze data in more ways. The higher the level of measurement, the more precise your data is.
The level at which you measure a variable determines how you can analyze your data.
Depending on the level of measurement , you can perform different descriptive statistics to get an overall summary of your data and inferential statistics to see if your results support or refute your hypothesis .
Levels of measurement tell you how precisely variables are recorded. There are 4 levels of measurement, which can be ranked from low to high:
- Nominal : the data can only be categorized.
- Ordinal : the data can be categorized and ranked.
- Interval : the data can be categorized and ranked, and evenly spaced.
- Ratio : the data can be categorized, ranked, evenly spaced and has a natural zero.
No. The p -value only tells you how likely the data you have observed is to have occurred under the null hypothesis .
If the p -value is below your threshold of significance (typically p < 0.05), then you can reject the null hypothesis, but this does not necessarily mean that your alternative hypothesis is true.
The alpha value, or the threshold for statistical significance , is arbitrary – which value you use depends on your field of study.
In most cases, researchers use an alpha of 0.05, which means that there is a less than 5% chance that the data being tested could have occurred under the null hypothesis.
P -values are usually automatically calculated by the program you use to perform your statistical test. They can also be estimated using p -value tables for the relevant test statistic .
P -values are calculated from the null distribution of the test statistic. They tell you how often a test statistic is expected to occur under the null hypothesis of the statistical test, based on where it falls in the null distribution.
If the test statistic is far from the mean of the null distribution, then the p -value will be small, showing that the test statistic is not likely to have occurred under the null hypothesis.
A p -value , or probability value, is a number describing how likely it is that your data would have occurred under the null hypothesis of your statistical test .
The test statistic you use will be determined by the statistical test.
You can choose the right statistical test by looking at what type of data you have collected and what type of relationship you want to test.
The test statistic will change based on the number of observations in your data, how variable your observations are, and how strong the underlying patterns in the data are.
For example, if one data set has higher variability while another has lower variability, the first data set will produce a test statistic closer to the null hypothesis , even if the true correlation between two variables is the same in either data set.
The formula for the test statistic depends on the statistical test being used.
Generally, the test statistic is calculated as the pattern in your data (i.e. the correlation between variables or difference between groups) divided by the variance in the data (i.e. the standard deviation ).
- Univariate statistics summarize only one variable at a time.
- Bivariate statistics compare two variables .
- Multivariate statistics compare more than two variables .
The 3 main types of descriptive statistics concern the frequency distribution, central tendency, and variability of a dataset.
- Distribution refers to the frequencies of different responses.
- Measures of central tendency give you the average for each response.
- Measures of variability show you the spread or dispersion of your dataset.
Descriptive statistics summarize the characteristics of a data set. Inferential statistics allow you to test a hypothesis or assess whether your data is generalizable to the broader population.
In statistics, model selection is a process researchers use to compare the relative value of different statistical models and determine which one is the best fit for the observed data.
The Akaike information criterion is one of the most common methods of model selection. AIC weights the ability of the model to predict the observed data against the number of parameters the model requires to reach that level of precision.
AIC model selection can help researchers find a model that explains the observed variation in their data while avoiding overfitting.
In statistics, a model is the collection of one or more independent variables and their predicted interactions that researchers use to try to explain variation in their dependent variable.
You can test a model using a statistical test . To compare how well different models fit your data, you can use Akaike’s information criterion for model selection.
The Akaike information criterion is calculated from the maximum log-likelihood of the model and the number of parameters (K) used to reach that likelihood. The AIC function is 2K – 2(log-likelihood) .
Lower AIC values indicate a better-fit model, and a model with a delta-AIC (the difference between the two AIC values being compared) of more than -2 is considered significantly better than the model it is being compared to.
The Akaike information criterion is a mathematical test used to evaluate how well a model fits the data it is meant to describe. It penalizes models which use more independent variables (parameters) as a way to avoid over-fitting.
AIC is most often used to compare the relative goodness-of-fit among different models under consideration and to then choose the model that best fits the data.
A factorial ANOVA is any ANOVA that uses more than one categorical independent variable . A two-way ANOVA is a type of factorial ANOVA.
Some examples of factorial ANOVAs include:
- Testing the combined effects of vaccination (vaccinated or not vaccinated) and health status (healthy or pre-existing condition) on the rate of flu infection in a population.
- Testing the effects of marital status (married, single, divorced, widowed), job status (employed, self-employed, unemployed, retired), and family history (no family history, some family history) on the incidence of depression in a population.
- Testing the effects of feed type (type A, B, or C) and barn crowding (not crowded, somewhat crowded, very crowded) on the final weight of chickens in a commercial farming operation.
In ANOVA, the null hypothesis is that there is no difference among group means. If any group differs significantly from the overall group mean, then the ANOVA will report a statistically significant result.
Significant differences among group means are calculated using the F statistic, which is the ratio of the mean sum of squares (the variance explained by the independent variable) to the mean square error (the variance left over).
If the F statistic is higher than the critical value (the value of F that corresponds with your alpha value, usually 0.05), then the difference among groups is deemed statistically significant.
The only difference between one-way and two-way ANOVA is the number of independent variables . A one-way ANOVA has one independent variable, while a two-way ANOVA has two.
- One-way ANOVA : Testing the relationship between shoe brand (Nike, Adidas, Saucony, Hoka) and race finish times in a marathon.
- Two-way ANOVA : Testing the relationship between shoe brand (Nike, Adidas, Saucony, Hoka), runner age group (junior, senior, master’s), and race finishing times in a marathon.
All ANOVAs are designed to test for differences among three or more groups. If you are only testing for a difference between two groups, use a t-test instead.
Multiple linear regression is a regression model that estimates the relationship between a quantitative dependent variable and two or more independent variables using a straight line.
Linear regression most often uses mean-square error (MSE) to calculate the error of the model. MSE is calculated by:
- measuring the distance of the observed y-values from the predicted y-values at each value of x;
- squaring each of these distances;
- calculating the mean of each of the squared distances.
Linear regression fits a line to the data by finding the regression coefficient that results in the smallest MSE.
Simple linear regression is a regression model that estimates the relationship between one independent variable and one dependent variable using a straight line. Both variables should be quantitative.
For example, the relationship between temperature and the expansion of mercury in a thermometer can be modeled using a straight line: as temperature increases, the mercury expands. This linear relationship is so certain that we can use mercury thermometers to measure temperature.
A regression model is a statistical model that estimates the relationship between one dependent variable and one or more independent variables using a line (or a plane in the case of two or more independent variables).
A regression model can be used when the dependent variable is quantitative, except in the case of logistic regression, where the dependent variable is binary.
A t-test should not be used to measure differences among more than two groups, because the error structure for a t-test will underestimate the actual error when many groups are being compared.
If you want to compare the means of several groups at once, it’s best to use another statistical test such as ANOVA or a post-hoc test.
A one-sample t-test is used to compare a single population to a standard value (for example, to determine whether the average lifespan of a specific town is different from the country average).
A paired t-test is used to compare a single population before and after some experimental intervention or at two different points in time (for example, measuring student performance on a test before and after being taught the material).
A t-test measures the difference in group means divided by the pooled standard error of the two group means.
In this way, it calculates a number (the t-value) illustrating the magnitude of the difference between the two group means being compared, and estimates the likelihood that this difference exists purely by chance (p-value).
Your choice of t-test depends on whether you are studying one group or two groups, and whether you care about the direction of the difference in group means.
If you are studying one group, use a paired t-test to compare the group mean over time or after an intervention, or use a one-sample t-test to compare the group mean to a standard value. If you are studying two groups, use a two-sample t-test .
If you want to know only whether a difference exists, use a two-tailed test . If you want to know if one group mean is greater or less than the other, use a left-tailed or right-tailed one-tailed test .
A t-test is a statistical test that compares the means of two samples . It is used in hypothesis testing , with a null hypothesis that the difference in group means is zero and an alternate hypothesis that the difference in group means is different from zero.
Statistical significance is a term used by researchers to state that it is unlikely their observations could have occurred under the null hypothesis of a statistical test . Significance is usually denoted by a p -value , or probability value.
Statistical significance is arbitrary – it depends on the threshold, or alpha value, chosen by the researcher. The most common threshold is p < 0.05, which means that the data is likely to occur less than 5% of the time under the null hypothesis .
When the p -value falls below the chosen alpha value, then we say the result of the test is statistically significant.
A test statistic is a number calculated by a statistical test . It describes how far your observed data is from the null hypothesis of no relationship between variables or no difference among sample groups.
The test statistic tells you how different two or more groups are from the overall population mean , or how different a linear slope is from the slope predicted by a null hypothesis . Different test statistics are used in different statistical tests.
Statistical tests commonly assume that:
- the data are normally distributed
- the groups that are being compared have similar variance
- the data are independent
If your data does not meet these assumptions you might still be able to use a nonparametric statistical test , which have fewer requirements but also make weaker inferences.
Ask our team
Want to contact us directly? No problem. We are always here for you.
- Email [email protected]
- Start live chat
- Call +1 (510) 822-8066
- WhatsApp +31 20 261 6040
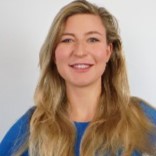
Our team helps students graduate by offering:
- A world-class citation generator
- Plagiarism Checker software powered by Turnitin
- Innovative Citation Checker software
- Professional proofreading services
- Over 300 helpful articles about academic writing, citing sources, plagiarism, and more
Scribbr specializes in editing study-related documents . We proofread:
- PhD dissertations
- Research proposals
- Personal statements
- Admission essays
- Motivation letters
- Reflection papers
- Journal articles
- Capstone projects
Scribbr’s Plagiarism Checker is powered by elements of Turnitin’s Similarity Checker , namely the plagiarism detection software and the Internet Archive and Premium Scholarly Publications content databases .
The add-on AI detector is powered by Scribbr’s proprietary software.
The Scribbr Citation Generator is developed using the open-source Citation Style Language (CSL) project and Frank Bennett’s citeproc-js . It’s the same technology used by dozens of other popular citation tools, including Mendeley and Zotero.
You can find all the citation styles and locales used in the Scribbr Citation Generator in our publicly accessible repository on Github .
- Neuroscience
Reevaluating the Neural Noise Hypothesis in Dyslexia: Insights from EEG and 7T MRS Biomarkers
Agnieszka glica, katarzyna wasilewska, julia jurkowska, jarosław żygierewicz, bartosz kossowski.
- Katarzyna Jednoróg author has email address
- Laboratory of Language Neurobiology, Nencki Institute of Experimental Biology, Polish Academy of Sciences, Pasteur 3 Street, 02-093 Warsaw, Poland
- Faculty of Physics, University of Warsaw, Pasteur 5 Street, 02-093 Warsaw, Poland
- Laboratory of Brain Imaging, Nencki Institute of Experimental Biology, Polish Academy of Sciences, Pasteur 3 Street, 02-093 Warsaw, Poland
- https://doi.org/ 10.7554/eLife.99920.1
- Open access
- Copyright information
The neural noise hypothesis of dyslexia posits an imbalance between excitatory and inhibitory (E/I) brain activity as an underlying mechanism of reading difficulties. This study provides the first direct test of this hypothesis using both indirect EEG power spectrum measures in 120 Polish adolescents and young adults (60 with dyslexia, 60 controls) and direct glutamate (Glu) and gamma-aminobutyric acid (GABA) concentrations from magnetic resonance spectroscopy (MRS) at 7T MRI scanner in half of the sample. Our results, supported by Bayesian statistics, show no evidence of E/I balance differences between groups, challenging the hypothesis that cortical hyperexcitability underlies dyslexia. These findings suggest alternative mechanisms must be explored and highlight the need for further research into the E/I balance and its role in neurodevelopmental disorders.
eLife assessment
The authors combined neurophysiological (electroencephalography [EEG]) and neurochemical (magnetic resonance spectroscopy [MRS]) measures to empirically evaluate the neural noise hypothesis of developmental dyslexia. Their results are solid , supported by consistent findings from the two complementary methodologies and Bayesian statistics. Additional analyses, particularly on the neurochemical measures, are necessary to further substantiate the results. This study is useful for understanding the neural mechanisms of dyslexia and neural development in general.
- https://doi.org/ 10.7554/eLife.99920.1.sa3
- Read the peer reviews
- About eLife assessments
Introduction
According to the neural noise hypothesis of dyslexia, reading difficulties stem from an imbalance between excitatory and inhibitory (E/I) neural activity ( Hancock et al., 2017 ). The hypothesis predicts increased cortical excitation leading to more variable and less synchronous neural firing. This instability supposedly results in disrupted sensory representations and impedes phonological awareness and multisensory integration skills, crucial for learning to read ( Hancock et al., 2017 ). Yet, studies testing this hypothesis are lacking.
The non-invasive measurement of the E/I balance can be derived through assessment of glutamate (Glu) and gamma-aminobutyric acid (GABA) neurotransmitters concentration via magnetic resonance spectroscopy (MRS) ( Finkelman et al., 2022 ) or through global, indirect estimations from the electroencephalography (EEG) signal ( Ahmad et al., 2022 ).
Direct measurements of Glu and GABA yielded conflicting findings. Higher Glu concentrations in the midline occipital cortex correlated with poorer reading performance in children ( Del Tufo et al., 2018 ; Pugh et al., 2014 ), while elevated Glu levels in the anterior cingulate cortex (ACC) corresponded to greater phonological skills ( Lebel et al., 2016 ). Elevated GABA in the left inferior frontal gyrus was linked to reduced verbal fluency in adults ( Nakai and Okanoya, 2016 ), and increased GABA in the midline occipital cortex in children was associated with slower reaction times in a linguistic task ( Del Tufo et al., 2018 ). However, notable null findings exist regarding dyslexia status and Glu levels in the ACC among children ( Horowitz-Kraus et al., 2018 ) as well as Glu and GABA levels in the visual and temporo-parietal cortices in both children and adults ( Kossowski et al., 2019 ).
Both beta (∼13-28 Hz) and gamma (> 30 Hz) oscillations may serve as E/I balance indicators ( Ahmad et al., 2022 ), as greater GABA-ergic activity has been associated with greater beta power ( Jensen et al., 2005 ; Porjesz et al., 2002 ) and gamma power or peak frequency ( Brunel and Wang, 2003 ; Chen et al., 2017 ). Resting-state analyses often reported nonsignificant beta power associations with dyslexia ( Babiloni et al., 2012 ; Fraga González et al., 2018 ; Xue et al., 2020 ), however, one study indicated lower beta power in dyslexic compared to control boys ( Fein et al., 1986 ). Mixed results were also observed during tasks. One study found decreased beta power in the dyslexic group ( Spironelli et al., 2008 ), while the other increased beta power relative to the control group ( Rippon and Brunswick, 2000 ). Insignificant relationship between resting gamma power and dyslexia was reported ( Babiloni et al., 2012 ; Lasnick et al., 2023 ). When analyzing auditory steady-state responses, the dyslexic group had a lower gamma peak frequency, while no significant differences in gamma power were observed ( Rufener and Zaehle, 2021 ). Essentially, the majority of studies in dyslexia examining gamma frequencies evaluated cortical entrainment to auditory stimuli ( Lehongre et al., 2011 ; Marchesotti et al., 2020 ; Van Hirtum et al., 2019 ). Therefore, the results from these tasks do not provide direct evidence of differences in either gamma power or peak frequency between the dyslexic and control groups.
The EEG signal comprises both oscillatory, periodic activity, and aperiodic activity, characterized by a gradual decrease in power as frequencies rise (1/f signal) ( Donoghue et al., 2020 ). Recently recognized as a biomarker of E/I balance, a lower exponent of signal decay (flatter slope) indicates a greater dominance of excitation over inhibition in the brain, as shown by the simulation models of local field potentials, ratio of AMPA/GABA a synapses in the rat hippocampus ( Gao et al., 2017 ) and recordings under propofol or ketamine in macaques and humans ( Gao et al., 2017 ; Waschke et al., 2021 ). However, there are also pharmacological studies providing mixed results ( Colombo et al., 2019 ; Salvatore et al., 2024 ). Nonetheless, the 1/f signal has shown associations with various conditions putatively characterized by changes in E/I balance, such as early development in infancy ( Schaworonkow and Voytek, 2021 ), healthy aging ( Voytek et al., 2015 ) and neurodevelopmental disorders like ADHD ( Ostlund et al., 2021 ), autism spectrum disorder ( Manyukhina et al., 2022 ) or schizophrenia ( Molina et al., 2020 ). Despite its potential relevance, the evaluation of the 1/f signal in dyslexia remains limited to one study, revealing flatter slopes among dyslexic compared to control participants at rest ( Turri et al., 2023 ), thereby lending support to the notion of neural noise in dyslexia.
Here, we examined both indirect (1/f signal, beta, and gamma oscillations during both rest and a spoken language task) and direct (Glu and GABA) biomarkers of E/I balance in participants with dyslexia and age-matched controls. The neural noise hypothesis predicts flatter slopes of 1/f signal, decreased beta and gamma power, and higher Glu concentrations in the dyslexic group. Furthermore, we tested the relationships between different E/I measures. Flatter slopes of 1/f signal should be related to higher Glu level, while enhanced beta and gamma power to increased GABA level.
No evidence for group differences in the EEG E/I biomarkers
We recruited 120 Polish adolescents and young adults – 60 with dyslexia diagnosis and 60 controls matched in sex, age, and family socio-economic status. The dyslexic group scored lower in all reading and reading-related tasks and higher in the Polish version of the Adult Reading History Questionnaire (ARHQ-PL) ( Bogdanowicz et al., 2015 ),where a higher score indicates a higher risk of dyslexia (see Table S1 in the Supplementary Material). Although all participants were within the intellectual norm, the dyslexic group scored lower on the IQ scale (including nonverbal subscale only) than the control group. However, the Bayesian statistics did not provide evidence for the difference between groups in the nonverbal IQ.
We analyzed the aperiodic (exponent and offset) components of the EEG signal at rest and during a spoken language task, where participants listened to a sentence and had to indicate its veracity. Due to a technical error, the signal from one person (a female from the dyslexic group) was not recorded during most of the language task and was excluded from the analyses. Hence, the results are provided for 119 participants – 59 in the dyslexic and 60 in the control group.
First, aperiodic parameter values were averaged across all electrodes and compared between groups (dyslexic, control) and conditions (resting state, language task) using a 2×2 repeated measures ANOVA. Age negatively correlated both with the exponent ( r = -.27, p = .003, BF 10 = 7.96) and offset ( r = -.40, p < .001, BF 10 = 3174.29) in line with previous investigations ( Cellier et al., 2021 ; McSweeney et al., 2021 ; Schaworonkow and Voytek, 2021 ; Voytek et al., 2015 ), therefore we included age as a covariate. Post-hoc tests are reported with Bonferroni corrected p -values.
For the mean exponent, we found a significant effect of age ( F (1,116) = 8.90, p = .003, η 2 p = .071, BF incl = 10.47), while the effects of condition ( F (1,116) = 2.32, p = .131, η 2 p = .020, BF incl = 0.39) and group ( F (1,116) = 0.08, p = .779, η 2 p = .001, BF incl = 0.40) were not significant and Bayes Factor did not provide evidence for either inclusion or exclusion. Interaction between group and condition ( F (1,116) = 0.16, p = .689, η 2 p = .001, BF incl = 0.21) was not significant and Bayes Factor indicated against including it in the model.
For the mean offset, we found significant effects of age ( F (1,116) = 22.57, p < .001, η 2 p = .163, BF incl = 1762.19) and condition ( F (1,116) = 23.04, p < .001, η 2 p = .166, BF incl > 10000) with post-hoc comparison indicating that the offset was lower in the resting state condition ( M = -10.80, SD = 0.21) than in the language task ( M = -10.67, SD = 0.26, p corr < .001). The effect of group ( F (1,116) = 0.00, p = .964, η 2 p = .000, BF incl = 0.54) was not significant while Bayes Factor did not provide evidence for either inclusion or exclusion. Interaction between group and condition was not significant ( F (1,116) = 0.07, p = .795, η 2 p = .001, BF incl = 0.22) and Bayes Factor indicated against including it in the model.
Next, we restricted analyses to language regions and averaged exponent and offset values from the frontal electrodes corresponding to the left (F7, FT7, FC5) and right inferior frontal gyrus (F8, FT8, FC6), as well as temporal electrodes, corresponding to the left (T7, TP7, TP9) and right superior temporal sulcus, STS (T8, TP8, TP10)( Giacometti et al., 2014 )( Scrivener and Reader, 2022 ). A 2×2×2×2 (group, condition, hemisphere, region) repeated measures ANOVA with age as a covariate was applied. Power spectra from the left STS at rest and during the language task are presented in Figure 1A and C , while the results for the exponent, offset, and beta power are presented in Figure 1B and D .

Overview of the main results obtained in the study. (A) Power spectral densities averaged across 3 electrodes (T7, TP7, TP9) corresponding to the left superior temporal sulcus (STS) separately for dyslexic (DYS) and control (CON) groups at rest and (C) during the language task. (B) Plots illustrating results for the exponent, offset, and the beta power from the left STS electrodes at rest and (D ) during the language task. (E) Group results (CON > DYS) from the fMRI localizer task for words compared to the control stimuli (p < .05 FWE cluster threshold) and overlap of the MRS voxel placement across participants. (F) MRS spectra separately for DYS and CON groups. (G) Plots illustrating results for the Glu, GABA, Glu/GABA ratio and the Glu/GABA imbalance. (H ) Semi-partial correlation between offset at rest (left STS electrodes) and Glu controlling for age and gray matter volume (GMV).
For the exponent, there were significant effects of age ( F (1,116) = 14.00, p < .001, η 2 p = .108, BF incl = 11.46) and condition F (1,116) = 4.06, p = .046, η 2 p = .034, BF incl = 1.88), however, Bayesian statistics did not provide evidence for either including or excluding the condition factor. Furthermore, post-hoc comparisons did not reveal significant differences between the exponent at rest ( M = 1.51, SD = 0.17) and during the language task ( M = 1.51, SD = 0.18, p corr = .546). There was also a significant interaction between region and group, although Bayes Factor indicated against including it in the model ( F (1,116) = 4.44, p = .037, η 2 p = .037, BF incl = 0.25). Post-hoc comparisons indicated that the exponent was higher in the frontal than in the temporal region both in the dyslexic ( M frontal = 1.54, SD frontal = 0.15, M temporal = 1.49, SD temporal = 0.18, p corr < .001) and in the control group ( M frontal = 1.54, SD frontal = 0.17, M temporal = 1.46, SD temporal = 0.20, p corr < .001). The difference between groups was not significant either in the frontal ( p corr = .858) or temporal region ( p corr = .441). The effects of region ( F (1,116) = 1.17, p = .282, η 2 p = .010, BF incl > 10000) and hemisphere ( F (1,116) = 1.17, p = .282, η 2 p = .010, BF incl = 12.48) were not significant, although Bayesian statistics indicated in favor of including them in the model. Furthermore, the interactions between condition and group ( F (1,116) = 0.18, p = .673, η 2 p = .002, BF incl = 3.70), and between region, hemisphere, and condition ( F (1,116) = 0.11, p = .747, η 2 p = .001, BF incl = 7.83) were not significant, however Bayesian statistics indicated in favor of including these interactions in the model. The effect of group ( F (1,116) = 0.12, p = .733, η 2 p = .001, BF incl = 1.19) was not significant, while Bayesian statistics did not provide evidence for either inclusion or exclusion. Any other interactions were not significant and Bayes Factor indicated against including them in the model.
In the case of offset, there were significant effects of condition ( F (1,116) = 20.88, p < .001, η 2 p = .153, BF incl > 10000) and region ( F (1,116) = 6.18, p = .014, η 2 p = .051, BF incl > 10000). For the main effect of condition, post-hoc comparison indicated that the offset was lower in the resting state condition ( M = -10.88, SD = 0.33) than in the language task ( M = -10.76, SD = 0.38, p corr < .001), while for the main effect of region, post-hoc comparison indicated that the offset was lower in the temporal ( M = -10.94, SD = 0.37) as compared to the frontal region ( M = -10.69, SD = 0.34, p corr < .001). There was also a significant effect of age ( F (1,116) = 20.84, p < .001, η 2 p = .152, BF incl = 0.23) and interaction between condition and hemisphere, ( F (1,116) = 4.35, p = .039, η 2 p = .036, BF incl = 0.21), although Bayes Factor indicated against including these factors in the model. Post-hoc comparisons for the condition*hemisphere interaction indicated that the offset was lower in the resting state condition than in the language task both in the left ( M rest = -10.85, SD rest = 0.34, M task = -10.73, SD task = 0.40, p corr < .001) and in the right hemisphere ( M rest = -10.91, SD rest = 0.31, M task = -10.79, SD task = 0.37, p corr < .001) and that the offset was lower in the right as compared to the left hemisphere both at rest ( p corr < .001) and during the language task ( p corr < .001). The interactions between region and condition ( F (1,116) = 1.76, p = .187, η 2 p = .015, BF incl > 10000), hemisphere and group ( F (1,116) = 1.58, p = .211, η 2 p = .013, BF incl = 1595.18), region and group ( F (1,116) = 0.27, p = .605, η 2 p = .002, BF incl = 9.32), as well as between region, condition, and group ( F (1,116) = 0.21, p = .651, η 2 p = .002, BF incl = 2867.18) were not significant, although Bayesian statistics indicated in favor of including them in the model. The effect of group ( F (1,116) = 0.18, p = .673, η 2 p = .002, BF incl < 0.00001) was not significant and Bayesian statistics indicated against including it in the model. Any other interactions were not significant and Bayesian statistics indicated against including them in the model or did not provide evidence for either inclusion or exclusion.
Then, we analyzed the aperiodic-adjusted brain oscillations. Since the algorithm did not find the gamma peak (30-43 Hz) above the aperiodic component in the majority of participants, we report the results only for the beta (14-30 Hz) power. We performed a similar regional analysis as for the exponent and offset with a 2×2×2×2 (group, condition, hemisphere, region) repeated measures ANOVA. However, we did not include age as a covariate, as it did not correlate with any of the periodic measures. The sample size was 117 (DYS n = 57, CON n = 60) since in 2 participants the algorithm did not find the beta peak above the aperiodic component in the left frontal electrodes during the task.
The analysis revealed a significant effect of condition ( F (1,115) = 8.58, p = .004, η 2 p = .069, BF incl = 5.82) with post-hoc comparison indicating that the beta power was greater during the language task ( M = 0.53, SD = 0.22) than at rest ( M = 0.50, SD = 0.19, p corr = .004). There were also significant effects of region ( F (1,115) = 10.98, p = .001, η 2 p = .087, BF incl = 23.71), and hemisphere ( F (1,115) = 12.08, p < .001, η 2 p = .095, BF incl = 23.91). For the main effect of region, post-hoc comparisons indicated that the beta power was greater in the temporal ( M = 0.52, SD = 0.21) as compared to the frontal region ( M = 0.50, SD = 0.19, p corr = .001), while for the main effect of hemisphere, post-hoc comparisons indicated that the beta power was greater in the right ( M = 0.52, SD = 0.20) than in the left hemisphere ( M = 0.51, SD = 0.20, p corr < .001). There was a significant interaction between condition and region ( F (1,115) = 12.68, p < .001, η 2 p = .099, BF incl = 55.26) with greater beta power during the language task as compared to rest significant in the temporal ( M rest = 0.50, SD rest = 0.20, M task = 0.55, SD task = 0.24, p corr < .001), while not in the frontal region ( M rest = 0.49, SD rest = 0.18, M task = 0.51, SD task = 0.22, p corr = .077). Also, greater beta power in the temporal as compared to the frontal region was significant during the language task ( p corr < .001), while not at rest ( p corr = .283). The effect of group ( F (1,115) = 0.05, p = .817, η 2 p = .000, BF incl < 0.00001) was not significant and Bayes Factor indicated against including it in the model. Any other interactions were not significant and Bayesian statistics indicated against including them in the model or did not provide evidence for either inclusion or exclusion.
Additionally, building upon previous findings which demonstrated differences in dyslexia in aperiodic and periodic components within the parieto-occipital region ( Turri et al., 2023 ), we have included analyses for the same cluster of electrodes in the Supplementary Material. However, in this region, we also did not find evidence for group differences either in the exponent, offset or beta power.
No evidence for group differences in Glu and GABA concentrations in the left STS
In total, 59 out of 120 participants underwent MRS session at 7T MRI scanner - 29 from the dyslexic group (13 females, 16 males) and 30 from the control group (14 females, 16 males). The MRS voxel was placed in the left STS, in a region showing highest activation for both visual and auditory words (compared to control stimuli) localized individually in each participant, based on an fMRI task (see Figure 1E for overlap of the MRS voxel placement across participants and Figure 1F for MRS spectra). We decided to analyze the neurometabolites’ levels derived from the left STS, as this region is consistently related to functional and structural differences in dyslexia across languages ( Yan et al., 2021 ).
Due to insufficient magnetic homogeneity or interruption of the study by the participants, 5 participants from the dyslexic group had to be excluded. We excluded further 4 participants due to poor quality of the obtained spectra thus the results for Glu are reported for 50 participants - 21 in the dyslexic (12 females, 9 males) and 29 in the control group (13 females, 16 males). In the case of GABA, we additionally excluded 3 participants based on the Cramér-Rao Lower Bounds (CRLB) > 20%. Therefore, the results for GABA, Glu/GABA ratio and Glu/GABA imbalance are reported for 47 participants - 20 in the dyslexic (12 females, 8 males) and 27 in the control group (11 females, 16 males). Demographic and behavioral characteristics for the subsample of 47 participants are provided in the Table S2.
For each metabolite, we performed a separate univariate ANCOVA with the effect of group being tested and voxel’s gray matter volume (GMV) as a covariate (see Figure 1G ). For the Glu analysis, we also included age as a covariate, due to negative correlation between variables ( r = -.35, p = .014, BF 10 = 3.41). The analysis revealed significant effect of GMV ( F (1,46) = 8.18, p = .006, η 2 p = .151, BF incl = 12.54), while the effects of age ( F (1,46) = 3.01, p = .090, η 2 p = .061, BF incl = 1.15) and group ( F (1,46) = 1.94, p = .170, 1 = .040, BF incl = 0.63) were not significant and Bayes Factor did not provide evidence for either inclusion or exclusion.
Conversely, GABA did not correlate with age ( r = -.11, p = .481, BF 10 = 0.23), thus age was not included as a covariate. The analysis revealed a significant effect of GMV ( F (1,44) = 4.39, p = .042, η 2 p = .091, BF incl = 1.64), however Bayes Factor did not provide evidence for either inclusion or exclusion. The effect of group was not significant ( F (1,44) = 0.49, p = .490, η 2 p = .011, BF incl = 0.35) although Bayesian statistics did not provide evidence for either inclusion or exclusion.
Also, Glu/GABA ratio did not correlate with age ( r = -.05, p = .744, BF 10 = 0.19), therefore age was not included as a covariate. The results indicated that the effect of GMV was not significant ( F (1,44) = 0.95, p = .335, η 2 p = .021, BF incl = 0.43) while Bayes Factor did not provide evidence for either inclusion or exclusion. The effect of group was not significant ( F (1,44) = 0.01, p = .933, η 2 p = .000, BF incl = 0.29) and Bayes Factor indicated against including it in the model.
Following a recent study examining developmental changes in both EEG and MRS E/I biomarkers ( McKeon et al., 2024 ), we calculated an additional measure of Glu/GABA imbalance, computed as the absolute residual value from the linear regression of Glu predicted by GABA with greater values indicating greater Glu/GABA imbalance. Alike the previous work ( McKeon et al., 2024 ), we took the square root of this value to ensure a normal distribution of the data. This measure did not correlate with age ( r = -.05, p = .719, BF 10 = 0.19); thus, age was not included as a covariate. The results indicated that the effect of GMV was not significant ( F (1,44) = 0.63, p = .430, η 2 p = .014, BF incl = 0.37) while Bayes Factor did not provide evidence for either inclusion or exclusion. The effect of group was not significant ( F (1,44) = 0.74, p = .396, η 2 p = .016, BF incl = 0.39) although Bayesian statistics did not provide evidence for either inclusion or exclusion.
Correspondence between Glu and GABA concentrations and EEG E/I biomarkers is limited
Next, we investigated correlations between Glu and GABA concentrations in the left STS and EEG markers of E/I balance. Semi-partial correlations were performed ( Table 1 ) to control for confounding variables - for Glu the effects of age and GMV were regressed, for GABA, Glu/GABA ratio and Glu/GABA imbalance the effect of GMV was regressed, while for exponents and offsets the effect of age was regressed. For zero-order correlations between variables see Table S3.

Semi-partial Correlations Between Direct and Indirect Markers of Excitatory-Inhibitory Balance. For Glu the Effects of Age and Gray Matter Volume (GMV) Were Regressed, for GABA, Glu/GABA Ratio and Glu/GABA Imbalance the Effect of GMV was Regressed, While for Exponents and Offsets the Effect of Age was Regressed
Glu negatively correlated with offset in the left STS both at rest ( r = -.38, p = .007, BF 10 = 6.28; Figure 1H ) and during the language task ( r = -.37, p = .009, BF 10 = 5.05), while any other correlations between Glu and EEG markers were not significant and Bayesian statistics indicated in favor of null hypothesis or provided absence of evidence for either hypothesis. Furthermore, Glu/GABA imbalance positively correlated with exponent at rest both averaged across all electrodes ( r = .29, p = .048, BF 10 = 1.21), as well as in the left STS electrodes ( r = .35, p = .017, BF 10 = 2.87) although Bayes Factor provided absence of evidence for either alternative or null hypothesis. Conversely, GABA and Glu/GABA ratio were not significantly correlated with any of the EEG markers and Bayesian statistics indicated in favor of null hypothesis or provided absence of evidence for either hypothesis.
Testing the paths from neural noise to reading
The neural noise hypothesis of dyslexia predicts impact of the neural noise on reading through the impairment of 1) phonological awareness, 2) lexical access and generalization and 3) multisensory integration ( Hancock et al., 2017 ). Therefore, we analyzed correlations between these variables, reading skills and direct and indirect markers of E/I balance. For the composite score of phonological awareness, we averaged z-scores from phoneme deletion, phoneme and syllable spoonerisms tasks. For the composite score of lexical access and generalization we averaged z-scores from objects, colors, letters and digits subtests from rapid automatized naming (RAN) task, while for the composite score of reading we averaged z-scores from words and pseudowords read per minute, and text reading time in reading comprehension task. The outcomes from the RAN and reading comprehension task have been transformed from raw time scores to items/time scores in order to provide the same direction of relationships for all z-scored measures, with greater values indicating better skills. For the multisensory integration score we used results from the redundant target effect task reported in our previous work ( Glica et al., 2024 ), with greater values indicating a greater magnitude of multisensory integration.
Age positively correlated with multisensory integration ( r = .38, p < .001, BF 10 = 87.98), composite scores of reading ( r = .22, p = .014, BF 10 = 2.24) and phonological awareness ( r = .21, p = .021, BF 10 = 1.59), while not with the composite score of RAN ( r = .13, p = .151, BF 10 = 0.32). Hence, we regressed the effect of age from multisensory integration, reading and phonological awareness scores and performed semi-partial correlations ( Table 2 , for zero-order correlations see Table S4).

Semi-partial Correlations Between Reading, Phonological Awareness, Rapid Automatized Naming, Multisensory Integration and Markers of Excitatory-Inhibitory Balance. For Reading, Phonological Awareness and Multisensory Integration the Effect of Age was Regressed, for Glu the Effects of Age and Gray Matter Volume (GMV) Were Regressed, for GABA, Glu/GABA Ratio and Glu/GABA Imbalance the Effect of GMV was Regressed, While for Exponents and Offsets the Effect of Age was Regressed
Phonological awareness positively correlated with offset in the left STS at rest ( r = .18, p = .049, BF 10 = 0.77) and with beta power in the left STS both at rest ( r = .23, p = .011, BF 10 = 2.73; Figure 2A ) and during the language task ( r = .23, p = .011, BF 10 = 2.84; Figure 2B ), although Bayes Factor provided absence of evidence for either alternative or null hypothesis. Furthermore, multisensory integration positively correlated with GABA concentration ( r = .31, p = .034, BF 10 = 1.62) and negatively with Glu/GABA ratio ( r = -.32, p = .029, BF 10 = 1.84), although Bayes Factor provided absence of evidence for either alternative or null hypothesis. Any other correlations between reading skills and E/I balance markers were not significant and Bayesian statistics indicated in favor of null hypothesis or provided absence of evidence for either hypothesis.

Associations between beta power, phonological awareness and reading. (A) Semi-partial correlation between phonological awareness controlling for age and beta power (in the left STS electrodes) at rest and (B) during the language task. (C) Partial correlation between phonological awareness and reading controlling for age. (D) Mediation analysis results. Unstandardized b regression coefficients are presented. Age was included in the analysis as a covariate. 95% CI - 95% confidence intervals. left STS - values averaged across 3 electrodes corresponding to the left superior temporal sulcus (T7, TP7, TP9).
Given that beta power correlated with phonological awareness, and considering the prediction that neural noise impedes reading by affecting phonological awareness — we examined this relationship through a mediation model. Since phonological awareness correlated with beta power in the left STS both at rest and during language task, the outcomes from these two conditions were averaged prior to the mediation analysis. Macro PROCESS v4.2 ( Hayes, 2017 ) on IBM SPSS Statistics v29 with model 4 (simple mediation) with 5000 Bootstrap samples to assess the significance of indirect effect was employed. Since age correlated both with phonological awareness and reading, we also included age as a covariate.
The results indicated that both effects of beta power in the left STS ( b = .96, t (116) = 2.71, p = .008, BF incl = 7.53) and age ( b = .06, t (116) = 2.55, p = .012, BF incl = 5.98) on phonological awareness were significant. The effect of phonological awareness on reading was also significant ( b = .69, t (115) = 8.16, p < .001, BF incl > 10000), while the effects of beta power ( b = -.42, t (115) = -1.25, p = .213, BF incl = 0.52) and age ( b = .03, t (115) = 1.18, p = .241, BF incl = 0.49) on reading were not significant when controlling for phonological awareness. Finally, the indirect effect of beta power on reading through phonological awareness was significant ( b = .66, SE = .24, 95% CI = [.24, 1.18]), while the total effect of beta power was not significant ( b = .24, t (116) = 0.61, p = .546, BF incl = 0.41). The results from the mediation analysis are presented in Figure 2D .
Although similar mediation analysis could have been conducted for the Glu/GABA ratio, multisensory integration, and reading based on the correlations between these variables, we did not test this model due to the small sample size (47 participants), which resulted in insufficient statistical power.
The current study aimed to validate the neural noise hypothesis of dyslexia ( Hancock et al., 2017 ) utilizing E/I balance biomarkers from EEG power spectra and ultra-high-field MRS. Contrary to its predictions, we did not observe differences either in 1/f slope, beta power, or Glu and GABA concentrations in participants with dyslexia. Relations between E/I balance biomarkers were limited to significant correlations between Glu and the offset when controlling for age, and between Glu/GABA imbalance and the exponent.
In terms of indirect markers, our study found no evidence of group differences in the aperiodic components of the EEG signal. In most of the models, we did not find evidence for either including or excluding the effect of the group when Bayesian statistics were evaluated. The only exception was the regional analysis for the offset, where results indicated against including the group factor in the model. These findings diverge from previous research on an Italian cohort, which reported decreased exponent and offset in the dyslexic group at rest, specifically within the parieto-occipital region, but not the frontal region ( Turri et al., 2023 ). Despite our study involving twice the number of participants and utilizing a longer acquisition time, we observed no group differences, even in the same cluster of electrodes (refer to Supplementary Material). The participants in both studies were of similar ages. The only methodological difference – EEG acquisition with eyes open in our study versus both eyes-open and eyes-closed in the work by Turri and colleagues (2023) – cannot fully account for the overall lack of group differences observed. The diverging study outcomes highlight the importance of considering potential inflation of effect sizes in studies with smaller samples.
Although a lower exponent of the EEG power spectrum has been associated with other neurodevelopmental disorders, such as ADHD ( Ostlund et al., 2021 ) or ASD (but only in children with IQ below average) ( Manyukhina et al., 2022 ), our study suggests that this is not the case for dyslexia. Considering the frequent comorbidity of dyslexia and ADHD ( Germanò et al., 2010 ; Langer et al., 2019 ), increased neural noise could serve as a common underlying mechanism for both disorders. However, our specific exclusion of participants with a comorbid ADHD diagnosis indicates that the EEG spectral exponent cannot serve as a neurobiological marker for dyslexia in isolation. No information regarding such exclusion criteria was provided in the study by Turri et al. (2023) ; thus, potential comorbidity with ADHD may explain the positive findings related to dyslexia reported therein.
Regarding the aperiodic-adjusted oscillatory EEG activity, Bayesian statistics for beta power, indicated in favor of excluding the group factor from the model. Non-significant group differences in beta power at rest have been previously reported in studies that did not account for aperiodic components ( Babiloni et al., 2012 ; Fraga González et al., 2018 ; Xue et al., 2020 ). This again contrasts with the study by Turri et al. (2023) , which observed lower aperiodic-adjusted beta power (at 15-25 Hz) in the dyslexic group. Concerning beta power during task, our results also contrast with previous studies which showed either reduced ( Spironelli et al., 2008 ) or increased ( Rippon and Brunswick, 2000 ) beta activity in participants with dyslexia. Nevertheless, since both of these studies employed phonological tasks and involved children’s samples, their relevance to our work is limited.
In terms of direct neurometabolite concentrations derived from the MRS, we found no evidence for group differences in either Glu, GABA or Glu/GABA imbalance in the language-sensitive left STS. Conversely, the Bayes Factor suggested against including the group factor in the model for the Glu/GABA ratio. While no previous study has localized the MRS voxel based on the individual activation levels, nonsignificant group differences in Glu and GABA concentrations within the temporo-parietal and visual cortices have been reported in both children and adults ( Kossowski et al., 2019 ), as well as in the ACC in children ( Horowitz-Kraus et al., 2018 ). Although our MRS sample size was half that of the EEG sample, previous research reporting group differences in Glu concentrations involved an even smaller dyslexic cohort (10 participants with dyslexia and 45 typical readers in Pugh et al., 2014 ). Consistent with earlier studies that identified group differences in Glu and GABA concentrations ( Del Tufo et al., 2018 ; Pugh et al., 2014 ) we reported neurometabolite levels relative to total creatine (tCr), indicating that the absence of corresponding results cannot be ascribed to reference differences. Notably, our analysis of the fMRI localizer task revealed greater activation in the control group as compared to the dyslexic group within the left STS for words than control stimuli (see Figure 1E and the Supplementary Material) in line with previous observations ( Blau et al., 2009 ; Dębska et al., 2021 ; Yan et al., 2021 ).
Irrespective of dyslexia status, we found negative correlations between age and exponent and offset, consistent with previous research ( Cellier et al., 2021 ; McSweeney et al., 2021 ; Schaworonkow and Voytek, 2021 ; Voytek et al., 2015 ) and providing further evidence for maturational changes in the aperiodic components (indicative of increased E/I ratio). At the same time, in line with previous MRS works ( Kossowski et al., 2019 ; Marsman et al., 2013 ), we observed a negative correlation between age and Glu concentrations. This suggests a contrasting pattern to EEG results, indicating a decrease in neuronal excitation with age. We also found a condition-dependent change in offset, with a lower offset observed at rest than during the language task. The offset value represents the uniform shift in power across frequencies ( Donoghue et al., 2020 ), with a higher offset linked to increased neuronal spiking rates ( Manning et al., 2009 ). Change in offset between conditions is consistent with observed increased alpha and beta power during the task, indicating elevated activity in both broadband (offset) and narrowband (alpha and beta oscillations) frequency ranges during the language task.
In regard to relationships between EEG and MRS E/I balance biomarkers, we observed a negative correlation between the offset in the left STS (both at rest and during the task) and Glu levels, after controlling for age and GMV. This correlation was not observed in zero-order correlations (see Supplementary Material). Contrary to our predictions, informed by previous studies linking the exponent to E/I ratio ( Colombo et al., 2019 ; Gao et al., 2017 ; Waschke et al., 2021 ), we found the correlation with Glu levels to involve the offset rather than the exponent. This outcome was unexpected, as none of the referenced studies reported results for the offset. However, given the strong correlation between the exponent and offset observed in our study ( r = .68, p < .001, BF 10 > 10000 and r = .72, p < .001, BF 10 > 10000 at rest and during the task respectively) it is conceivable that similar association might be identified for the offset if it were analyzed.
Nevertheless, previous studies examining relationships between EEG and MRS E/I balance biomarkers ( McKeon et al., 2024 ; van Bueren et al., 2023 ) did not identify a similar negative association between Glu and the offset. Instead, one study noted a positive correlation between the Glu/GABA ratio and the exponent ( van Bueren et al., 2023 ), which was significant in the intraparietal sulcus but not in the middle frontal gyrus. This finding presents counterintuitive evidence, suggesting that an increased E/I balance, as indicated by MRS, is associated with a higher aperiodic exponent, considered indicative of decreased E/I balance. In line with this pattern, another study discovered a positive relationship between the exponent and Glu levels in the dorsolateral prefrontal cortex ( McKeon et al., 2024 ). Furthermore, they observed a positive correlation between the exponent and the Glu/GABA imbalance measure, calculated as the absolute residual value of a linear relationship between Glu and GABA ( McKeon et al., 2024 ), a finding replicated in the current work. This implies that a higher spectral exponent might not be directly linked to MRS-derived Glu or GABA levels, but rather to a greater disproportion (in either direction) between these neurotransmitters. These findings, alongside the contrasting relationships between EEG and MRS biomarkers and age, suggest that these methods may reflect distinct biological mechanisms of E/I balance.
Evidence regarding associations between neurotransmitters levels and oscillatory activity also remains mixed. One study found a positive correlation between gamma peak frequency and GABA concentration in the visual cortex ( Muthukumaraswamy et al., 2009 ), a finding later challenged by a study with a larger sample ( Cousijn et al., 2014 ). Similarly, a different study noted a positive correlation between GABA in the left STS and gamma power ( Balz et al., 2016 ), another study, found non-significant relation between these measures ( Wyss et al., 2017 ). Moreover, in a simultaneous EEG and MRS study, an event-related increase in Glu following visual stimulation was found to correlate with greater gamma power ( Lally et al., 2014 ). We could not investigate such associations, as the algorithm failed to identify a gamma peak above the aperiodic component for the majority of participants. Also, contrary to previous findings showing associations between GABA in the motor and sensorimotor cortices and beta power ( Cheng et al., 2017 ; Gaetz et al., 2011 ) or beta peak frequency ( Baumgarten et al., 2016 ), we observed no correlation between Glu or GABA levels and beta power. However, these studies placed MRS voxels in motor regions which are typically linked to movement-related beta activity ( Baker et al., 1999 ; Rubino et al., 2006 ; Sanes and Donoghue, 1993 ) and did not adjust beta power for aperiodic components, making direct comparisons with our findings limited.
Finally, we examined pathways posited by the neural noise hypothesis of dyslexia, through which increased neural noise may impact reading: phonological awareness, lexical access and generalization, and multisensory integration ( Hancock et al., 2017 ). Phonological awareness was positively correlated with the offset in the left STS at rest, and with beta power in the left STS, both at rest and during the task. Additionally, multisensory integration showed correlations with GABA and the Glu/GABA ratio. Since the Bayes Factor did not provide conclusive evidence supporting either the alternative or null hypothesis, these associations appear rather weak. Nonetheless, given the hypothesis’s prediction of a causal link between these variables, we further examined a mediation model involving beta power, phonological awareness, and reading skills. The results suggested a positive indirect effect of beta power on reading via phonological awareness, whereas both the direct (controlling for phonological awareness and age) and total effects (without controlling for phonological awareness) were not significant. This finding is noteworthy, considering that participants with dyslexia exhibited reduced phonological awareness and reading skills, despite no observed differences in beta power. Given the cross-sectional nature of our study, further longitudinal research is necessary to confirm the causal relation among these variables. The effects of GABA and the Glu/GABA ratio on reading, mediated by multisensory integration, warrant further investigation. Additionally, considering our finding that only males with dyslexia showed deficits in multisensory integration ( Glica et al., 2024 ), sex should be considered as a potential moderating factor in future analyses. We did not test this model here due to the smaller sample size for GABA measurements.
Our findings suggest that the neural noise hypothesis, as proposed by Hancock and colleagues (2017) , does not fully explain the reading difficulties observed in dyslexia. Despite the innovative use of both EEG and MRS biomarkers to assess excitatory-inhibitory (E/I) balance, neither method provided evidence supporting an E/I imbalance in dyslexic individuals. Importantly, our study focused on adolescents and young adults, and the EEG recordings were conducted during rest and a spoken language task. These factors may limit the generalizability of our results. Future research should include younger populations and incorporate a broader array of tasks, such as reading and phonological processing, to provide a more comprehensive evaluation of the E/I balance hypothesis. Additionally, our findings are consistent with another study by Tan et al. (2022) which found no evidence for increased variability (’noise’) in behavioral and fMRI response patterns in dyslexia. Together, these results highlight the need to explore alternative neural mechanisms underlying dyslexia and suggest that cortical hyperexcitability may not be the primary cause of reading difficulties.
In conclusion, while our study challenges the neural noise hypothesis as a sole explanatory framework for dyslexia, it also underscores the complexity of the disorder and the necessity for multifaceted research approaches. By refining our understanding of the neural underpinnings of dyslexia, we can better inform future studies and develop more effective interventions for those affected by this condition.
Materials and methods
Participants.
A total of 120 Polish participants aged between 15.09 and 24.95 years ( M = 19.47, SD = 3.06) took part in the study. This included 60 individuals with a clinical diagnosis of dyslexia performed by the psychological and pedagogical counseling centers (28 females and 32 males) and 60 control participants without a history of reading difficulties (28 females and 32 males). All participants were right-handed, born at term, without any reported neurological/psychiatric diagnosis and treatment (including ADHD), without hearing impairment, with normal or corrected-to-normal vision, and IQ higher than 80 as assessed by the Polish version of the Abbreviated Battery of the Stanford-Binet Intelligence Scale-Fifth Edition (SB5) ( Roid et al., 2017 ).
The study was approved by the institutional review board at the University of Warsaw, Poland (reference number 2N/02/2021). All participants (or their parents in the case of underaged participants) provided written informed consent and received monetary remuneration for taking part in the study.
Reading and Reading-Related Tasks
Participants’ reading skills were assessed by multiple paper-pencil tasks described in detail in our previous work ( Glica et al., 2024 ). Briefly, we evaluated words and pseudowords read in one minute ( Szczerbiński and Pelc-Pękała, 2013 ), rapid automatized naming ( Fecenec et al., 2013 ), and reading comprehension speed. We also assessed phonological awareness by a phoneme deletion task ( Szczerbiński and Pelc-Pękała, 2013 ) and spoonerisms tasks ( Bogdanowicz et al., 2016 ), as well as orthographic awareness (Awramiuk and Krasowicz-Kupis, 2013). Furthermore, we evaluated non-verbal perception speed ( Ciechanowicz and Stańczak, 2006 ) and short-term and working memory by forward and backward conditions from the Digit Span subtest from the WAIS-R ( Wechsler, 1981 ). We also assessed participants’ multisensory audiovisual integration by a redundant target effect task, which results have been reported in our previous work ( Glica et al., 2024 ).
Electroencephalography Acquisition and Procedure
EEG was recorded from 62 scalp and 2 ear electrodes using the Brain Products system (actiCHamp Plus, Brain Products GmbH, Gilching, Germany). Data were recorded in BrainVision Recorder Software (Vers. 1.22.0002, Brain Products GmbH, Gilching, Germany) with a 500 Hz sampling rate. Electrodes were positioned in line with the extended 10-20 system. Electrode Cz served as an online reference, while the Fpz as a ground electrode. All electrodes’ impedances were kept below 10 kΩ. Participants sat in a chair with their heads on a chin-rest in a dark, sound-attenuated, and electrically shielded room while the EEG was recorded during both a 5-minute eyes-open resting state and the spoken language comprehension task. The paradigm was prepared in the Presentation software (Version 20.1, Neurobehavioral Systems, Inc., Berkeley, CA, www.neurobs.com ).
During rest, participants were instructed to relax and fixate their eyes on a white cross presented centrally on a black background. After 5 minutes, the spoken language comprehension task automatically started. The task consisted of 3 to 5 word-long sentences recorded in a speech synthesizer which were presented binaurally through sound-isolating earphones. After hearing a sentence, participants were asked to indicate whether the sentence was true or false by pressing a corresponding button. In total, there were 256 sentences – 128 true (e.g., “Plants need water”) and 128 false (e.g., “Dogs can fly”).
Sentences were presented in a random order in two blocks of 128 trials. At the beginning of each trial, a white fixation cross was presented centrally on a black background for 500 ms, then a blank screen appeared for either 500, 600, 700, or 800 ms (durations set randomly and equiprobably) followed by an auditory sentence presentation. The length of sentences ranged between 1.17 and 2.78 seconds and was balanced between true ( M = 1.82 seconds, SD = 0.29) and false sentences ( M = 1.82 seconds, SD = 0.32; t (254) = -0.21, p = .835; BF 10 = 0.14). After a sentence presentation, a blank screen was displayed for 1000 ms before starting the next trial. To reduce participants’ fatigue, a 1-minute break between two blocks of trials was introduced, and it took approximately 15 minutes to complete the task.
fMRI Acquisition and Procedure
MRI data were acquired using Siemens 3T Trio system with a 32-channel head coil. Structural data were acquired using whole brain 3D T1-weighted image (MP_RAGE, TI = 1100 ms, GRAPPA parallel imaging with acceleration factor PE = 2, voxel resolution = 1mm 3 , dimensions = 256×256×176). Functional data were acquired using whole-brain echo planar imaging sequence (TE = 30ms, TR = 1410 ms, flip angle FA = 90°, FOV = 212 mm, matrix size = 92×92, 60 axial slices 2.3mm thick, 2.3×2.3 mm in-plane resolution, multiband acceleration factor = 3). Due to a technical issue, data from two participants were acquired with a 12-channel coil (see Supplementary Material).
The fMRI task served as a localizer for later MRS voxel placement in language-sensitive left STS. The task was prepared using Presentation software (Version 20.1, Neurobehavioral Systems, Inc., Berkeley, CA, www.neurobs.com ) and consisted of three runs, each lasting 5 minutes and 9 seconds. Two runs involved the presentation of visual stimuli, while the third run of auditory stimuli. In each run, stimuli were presented in 12 blocks, with 14 stimuli per block. In visual runs, there were four blocks from each category: 1) 3 to 4 letters-long words, 2) the same words presented as a false font string (BACS font) ( Vidal et al., 2017 ), and 3) strings of 3 to 4-long consonants. Similarly, in the auditory run, there were four blocks from each category: 1) words recorded in a speech synthesizer, 2) the same words presented backward, and 3) consonant strings recorded in a speech synthesizer. Stimuli within each block were presented for 800 ms with a 400 ms break in between. The duration of each block was 16.8 seconds. Between blocks, a fixation cross was displayed for 8 seconds. Participants performed a 1-back task to maintain focus. The blocks were presented in a pseudorandom order and each block included 2 to 3 repeated stimuli.
MRS Acquisition and Procedure
The GE 7T system with a 32-channel coil was utilized. Structural data were acquired using whole brain 3D T1-weighted image (3D-SPGR BRAVO, TI = 450ms, TE = 2.6ms, TR = 6.6ms, flip angle = 12 deg, bandwidth = ±32.5kHz, ARC acceleration factor PE = 2, voxel resolution = 1mm, dimensions = 256 x 256 x 180). MRS spectra with 320 averages were acquired from the left STS using single-voxel spectroscopy semiLaser sequence ( Deelchand et al., 2021 ) (voxel size = 15 x 15 x 15 mm, TE = 28ms, TR = 4000ms, 4096 data points, water suppressed using VAPOR). Eight averages with unsuppressed water as a reference were collected.
To localize left STS, T1-weighted images from fMRI and MRS sessions were coregistered and fMRI peak coordinates were used as a center of voxel volume for MRS. Voxels were then adjusted to include only the brain tissue. During the acquisition, participants took part in a simple orthographic task.
Statistical Analyses
The continuous EEG signal was preprocessed in the EEGLAB ( Delorme and Makeig, 2004 ). The data were filtered between 0.5 and 45 Hz (Butterworth filter, 4th order) and re-referenced to the average of both ear electrodes. The data recorded during the break between blocks, as well as bad channels, were manually rejected. The number of rejected channels ranged between 0 and 4 ( M = 0.19, SD = 0.63). Next, independent component analysis (ICA) was applied. Components were automatically labeled by ICLabel ( Pion-Tonachini et al., 2019 ), and those classified with 50-100% source probability as eye blinks, muscle activity, heart activity, channel noise, and line noise, or with 0-50% source probability as brain activity, were excluded. Components labeled as “other” were visually inspected, and those identified as eye blinks and muscle activity were also rejected. The number of rejected components ranged between 11 and 46 ( M = 28.43, SD = 7.26). Previously rejected bad channels were interpolated using the nearest neighbor spline ( Perrin et al., 1989 , 1987 ).
The preprocessed data were divided into a 5-minute resting-state signal and a signal recorded during a spoken language comprehension task using MNE ( Gramfort, 2013 ) and custom Python scripts. The signal from the task was cut up based on the event markers indicating the beginning and end of a sentence. Only trials with correct responses given between 0 and 1000 ms after the end of a sentence were included. The signals recorded during every trial were further multiplied by the Tukey window with α = 0.01 in order to normalize signal amplitudes at the beginning and end of every trial. This allowed a smooth concatenation of signals recorded during task trials, resulting in a continuous signal derived only when participants were listening to the sentences.
The continuous signal from the resting state and the language task was epoched into 2-second-long segments. An automatic rejection criterion of +/-200 μV was applied to exclude epochs with excessive amplitudes. The number of epochs retained in the analysis ranged between 140–150 ( M = 149.66, SD = 1.20) in the resting state condition and between 102–226 ( M = 178.24, SD = 28.94) in the spoken language comprehension task.
Power spectral density (PSD) for 0.5-45 Hz in 0.5 Hz increments was calculated for every artifact-free epoch using Welch’s method for 2-second-long data segments windowed with a Hamming window with no overlap. The estimated PSDs were averaged for each participant and each channel separately for the resting state condition and the language task. Aperiodic and periodic (oscillatory) components were parameterized using the FOOOF method ( Donoghue et al., 2020 ). For each PSD, we extracted parameters for the 1-43 Hz frequency range using the following settings: peak_width_limits = [1, 12], max_n_peaks = infinite, peak_threshold = 2.0, mean_peak_height = 0.0, aperiodic_mode = ‘fixed’. Apart from broad-band aperiodic parameters (exponent and offset), we also extracted power, bandwidth, and the center frequency parameters for the theta (4-7 Hz), alpha (7-14 Hz), beta (14-30 Hz) and gamma (30-43 Hz) bands. Since in the majority of participants, the algorithm did not find the peak above the aperiodic component in theta and gamma bands, we calculated the results only for the alpha and beta bands. The results for other periodic parameters than the beta power are reported in Supplementary Material.
Apart from the frequentist statistics, we also performed Bayesian statistics using JASP ( JASP Team, 2023 ). For Bayesian repeated measures ANOVA, we reported the Bayes Factor for the inclusion of a given effect (BF incl ) with the ’across matched model’ option, as suggested by Keysers and colleagues (2020) , calculated as a likelihood ratio of models with a presence of a specific factor to equivalent models differing only in the absence of the specific factor. For Bayesian t -tests and correlations, we reported the BF 10 value, indicating the ratio of the likelihood of an alternative hypothesis to a null hypothesis. We considered BF incl/10 > 3 and BF incl/10 < 1/3 as evidence for alternative and null hypotheses respectively, while 1/3 < BF incl/10 < 3 as the absence of evidence ( Keysers et al., 2020 ).
MRS voxel localization in the native space
The data were analyzed using Statistical Parametric Mapping (SPM12, Wellcome Trust Centre for Neuroimaging, London, UK) run on MATLAB R2020b (The MathWorks Inc., Natick, MA, USA). First, all functional images were realigned to the participant’s mean. Then, T1-weighted images were coregistered to functional images for each subject. Finally, fMRI data were smoothed with a 6mm isotropic Gaussian kernel.
In each subject, the left STS was localized in the native space as a cluster in the middle and posterior left superior temporal sulcus, exhibiting higher activation for visual words versus false font strings and auditory words versus backward words (logical AND conjunction) at p < .01 uncorrected. For 6 participants, the threshold was lowered to p < .05 uncorrected, while for another 6 participants, the contrast from the auditory run was changed to auditory words versus fixation cross due to a lack of activation for other contrasts.
In the Supplementary Material, we also performed the group-level analysis of the fMRI data (Tables S5-S7 and Figure S1).
MRS data were analyzed using fsl-mrs version 2.0.7 ( Clarke et al., 2021 ). Data stored in pfile format were converted into NIfTI-MRS using spec2nii tool. We then used the fsl_mrs_preproc function to automatically perform coil combination, frequency and phase alignment, bad average removal, combination of spectra, eddy current correction, shifting frequency to reference peak and phase correction.
To obtain information about the percentage of WM, GM and CSF in the voxel we used the svs_segmentation with results of fsl_anat as an input. Voxel segmentation was performed on structural images from a 3T scanner, coregistered to 7T structural images in SPM12. Next, quantitative fitting was performed using fsl_mrs function. As a basis set, we utilized a collection of 27 metabolite spectra simulated using FID-A ( Simpson et al., 2017 ) and a script tailored for our experiment. We supplemented this with synthetic macromolecule spectra provided by fsl_mrs . Signals acquired with unsuppressed water served as water reference.
Spectra underwent quantitative assessment and visual inspection and those with linewidth higher than 20Hz, %CRLB higher than 20%, and poor fit to the model were excluded from the analysis (see Table S8 in the Supplementary Material for a detailed checklist). Glu and GABA concentrations were expressed as a ratio to total-creatine (tCr; Creatine + Phosphocreatine).
Data Availability Statement
Behavioral data, raw and preprocessed EEG data, 2 nd level fMRI data, preprocessed MRS data and Python script for the analysis of preprocessed EEG data can be found at OSF: https://osf.io/4e7ps/
Acknowledgements
This study was supported by the National Science Centre grant (2019/35/B/HS6/01763) awarded to Katarzyna Jednoróg.
We gratefully acknowledge valuable discussions with Ralph Noeske from GE Healthcare for his support in setting up the protocol for an ultra-high field MR spectroscopy and sharing the set-up for basis set simulation in FID-A.
- Buitelaar J
- dos Santos FP
- Verschure PFMJ
- McAlonan G.
- Krasowicz-Kupis G
- Albertini G
- Roa Romero Y
- Ittermann B
- Senkowski D
- Baumgarten TJ
- Oeltzschner G
- Hoogenboom N
- Wittsack H-J
- Schnitzler A
- van Atteveldt N
- Bogdanowicz KM
- Bogdanowicz M
- Sajewicz-Radtke U
- Karpińska E
- Łockiewicz M
- Ciechanowicz A
- Napolitani M
- Gosseries O
- Casarotto S
- Brichant J-F
- Massimini M
- Chieregato A
- Harrison PJ
- Dzięgiel-Fivet G
- Łuniewska M
- Grabowska A
- Deelchand DK
- Berrington A
- Seraji-Bozorgzad N
- Del Tufo SN
- Fulbright RK
- Peterson EJ
- Sebastian P
- Jaworowska A
- Yingling CD
- Johnstone J
- Davenport L
- Finkelman T
- Furman-Haran E
- Fraga González G
- van der Molen MJW
- de Geus EJC
- van der Molen MW.
- Roberts TPL
- Giacometti P
- Wasilewska K
- Kossowski B
- Żygierewicz J
- Horowitz-Kraus T
- Ermentrout B
- Wagenmakers E-J
- Bogorodzki P
- Roberts M V.
- Haenschel C
- Lasnick OHM
- MacMaster FP
- Villiermet N
- Manyukhina VO
- Prokofyev AO
- Obukhova TS
- Schneiderman JF
- Altukhov DI
- Stroganova TA
- Orekhova E V
- Marchesotti S
- Donoghue JP
- van den Heuvel MP
- Hilleke E. HP
- Hetherington H
- McSweeney M
- Swerdlow NR
- Muthukumaraswamy SD
- Swettenham JB
- Karalunas SL
- Echallier JF
- Pion-Tonachini L
- Kreutz-Delgado K
- Edenberg HJ
- Chorlian DB
- O’Connor SJ
- Rohrbaugh J
- Schuckit MA
- Hesselbrock V
- Conneally PM
- Tischfield JA
- Begleiter H
- Grigorenko EL
- Seidenberg MS
- Brunswick N
- Hatsopoulos NG
- Salvatore S V.
- Zorumski CF
- Mennerick S
- Schaworonkow N
- Scrivener CL
- Hennessy TJ
- Spironelli C
- Penolazzi B
- Szczerbiński M
- Pelc-Pękała O
- van Bueren NER
- van der Ven SHG
- Cohen Kadosh R.
- Van Hirtum T
- Ghesquière P
- Tempesta ZR
- Achermann R
Article and author information
Katarzyna jednoróg, for correspondence:, version history.
- Sent for peer review : June 11, 2024
- Preprint posted : June 12, 2024
- Reviewed Preprint version 1 : September 5, 2024
© 2024, Glica et al.
This article is distributed under the terms of the Creative Commons Attribution License , which permits unrestricted use and redistribution provided that the original author and source are credited.
Views, downloads and citations are aggregated across all versions of this paper published by eLife.
Be the first to read new articles from eLife
13.1 Understanding Null Hypothesis Testing
Learning objectives.
- Explain the purpose of null hypothesis testing, including the role of sampling error.
- Describe the basic logic of null hypothesis testing.
- Describe the role of relationship strength and sample size in determining statistical significance and make reasonable judgments about statistical significance based on these two factors.
The Purpose of Null Hypothesis Testing
As we have seen, psychological research typically involves measuring one or more variables in a sample and computing descriptive statistics for that sample. In general, however, the researcher’s goal is not to draw conclusions about that sample but to draw conclusions about the population that the sample was selected from. Thus researchers must use sample statistics to draw conclusions about the corresponding values in the population. These corresponding values in the population are called parameters . Imagine, for example, that a researcher measures the number of depressive symptoms exhibited by each of 50 adults with clinical depression and computes the mean number of symptoms. The researcher probably wants to use this sample statistic (the mean number of symptoms for the sample) to draw conclusions about the corresponding population parameter (the mean number of symptoms for adults with clinical depression).
Unfortunately, sample statistics are not perfect estimates of their corresponding population parameters. This is because there is a certain amount of random variability in any statistic from sample to sample. The mean number of depressive symptoms might be 8.73 in one sample of adults with clinical depression, 6.45 in a second sample, and 9.44 in a third—even though these samples are selected randomly from the same population. Similarly, the correlation (Pearson’s r ) between two variables might be +.24 in one sample, −.04 in a second sample, and +.15 in a third—again, even though these samples are selected randomly from the same population. This random variability in a statistic from sample to sample is called sampling error . (Note that the term error here refers to random variability and does not imply that anyone has made a mistake. No one “commits a sampling error.”)
One implication of this is that when there is a statistical relationship in a sample, it is not always clear that there is a statistical relationship in the population. A small difference between two group means in a sample might indicate that there is a small difference between the two group means in the population. But it could also be that there is no difference between the means in the population and that the difference in the sample is just a matter of sampling error. Similarly, a Pearson’s r value of −.29 in a sample might mean that there is a negative relationship in the population. But it could also be that there is no relationship in the population and that the relationship in the sample is just a matter of sampling error.
In fact, any statistical relationship in a sample can be interpreted in two ways:
- There is a relationship in the population, and the relationship in the sample reflects this.
- There is no relationship in the population, and the relationship in the sample reflects only sampling error.
The purpose of null hypothesis testing is simply to help researchers decide between these two interpretations.
The Logic of Null Hypothesis Testing
Null hypothesis testing is a formal approach to deciding between two interpretations of a statistical relationship in a sample. One interpretation is called the null hypothesis (often symbolized H 0 and read as “H-naught”). This is the idea that there is no relationship in the population and that the relationship in the sample reflects only sampling error. Informally, the null hypothesis is that the sample relationship “occurred by chance.” The other interpretation is called the alternative hypothesis (often symbolized as H 1 ). This is the idea that there is a relationship in the population and that the relationship in the sample reflects this relationship in the population.
Again, every statistical relationship in a sample can be interpreted in either of these two ways: It might have occurred by chance, or it might reflect a relationship in the population. So researchers need a way to decide between them. Although there are many specific null hypothesis testing techniques, they are all based on the same general logic. The steps are as follows:
- Assume for the moment that the null hypothesis is true. There is no relationship between the variables in the population.
- Determine how likely the sample relationship would be if the null hypothesis were true.
- If the sample relationship would be extremely unlikely, then reject the null hypothesis in favor of the alternative hypothesis. If it would not be extremely unlikely, then retain the null hypothesis .
Following this logic, we can begin to understand why Mehl and his colleagues concluded that there is no difference in talkativeness between women and men in the population. In essence, they asked the following question: “If there were no difference in the population, how likely is it that we would find a small difference of d = 0.06 in our sample?” Their answer to this question was that this sample relationship would be fairly likely if the null hypothesis were true. Therefore, they retained the null hypothesis—concluding that there is no evidence of a sex difference in the population. We can also see why Kanner and his colleagues concluded that there is a correlation between hassles and symptoms in the population. They asked, “If the null hypothesis were true, how likely is it that we would find a strong correlation of +.60 in our sample?” Their answer to this question was that this sample relationship would be fairly unlikely if the null hypothesis were true. Therefore, they rejected the null hypothesis in favor of the alternative hypothesis—concluding that there is a positive correlation between these variables in the population.
A crucial step in null hypothesis testing is finding the likelihood of the sample result if the null hypothesis were true. This probability is called the p value . A low p value means that the sample result would be unlikely if the null hypothesis were true and leads to the rejection of the null hypothesis. A p value that is not low means that the sample result would be likely if the null hypothesis were true and leads to the retention of the null hypothesis. But how low must the p value be before the sample result is considered unlikely enough to reject the null hypothesis? In null hypothesis testing, this criterion is called α (alpha) and is almost always set to .05. If there is a 5% chance or less of a result as extreme as the sample result if the null hypothesis were true, then the null hypothesis is rejected. When this happens, the result is said to be statistically significant . If there is greater than a 5% chance of a result as extreme as the sample result when the null hypothesis is true, then the null hypothesis is retained. This does not necessarily mean that the researcher accepts the null hypothesis as true—only that there is not currently enough evidence to reject it. Researchers often use the expression “fail to reject the null hypothesis” rather than “retain the null hypothesis,” but they never use the expression “accept the null hypothesis.”
The Misunderstood p Value
The p value is one of the most misunderstood quantities in psychological research (Cohen, 1994) [1] . Even professional researchers misinterpret it, and it is not unusual for such misinterpretations to appear in statistics textbooks!
The most common misinterpretation is that the p value is the probability that the null hypothesis is true—that the sample result occurred by chance. For example, a misguided researcher might say that because the p value is .02, there is only a 2% chance that the result is due to chance and a 98% chance that it reflects a real relationship in the population. But this is incorrect . The p value is really the probability of a result at least as extreme as the sample result if the null hypothesis were true. So a p value of .02 means that if the null hypothesis were true, a sample result this extreme would occur only 2% of the time.
You can avoid this misunderstanding by remembering that the p value is not the probability that any particular hypothesis is true or false. Instead, it is the probability of obtaining the sample result if the null hypothesis were true.

“Null Hypothesis” retrieved from http://imgs.xkcd.com/comics/null_hypothesis.png (CC-BY-NC 2.5)
Role of Sample Size and Relationship Strength
Recall that null hypothesis testing involves answering the question, “If the null hypothesis were true, what is the probability of a sample result as extreme as this one?” In other words, “What is the p value?” It can be helpful to see that the answer to this question depends on just two considerations: the strength of the relationship and the size of the sample. Specifically, the stronger the sample relationship and the larger the sample, the less likely the result would be if the null hypothesis were true. That is, the lower the p value. This should make sense. Imagine a study in which a sample of 500 women is compared with a sample of 500 men in terms of some psychological characteristic, and Cohen’s d is a strong 0.50. If there were really no sex difference in the population, then a result this strong based on such a large sample should seem highly unlikely. Now imagine a similar study in which a sample of three women is compared with a sample of three men, and Cohen’s d is a weak 0.10. If there were no sex difference in the population, then a relationship this weak based on such a small sample should seem likely. And this is precisely why the null hypothesis would be rejected in the first example and retained in the second.
Of course, sometimes the result can be weak and the sample large, or the result can be strong and the sample small. In these cases, the two considerations trade off against each other so that a weak result can be statistically significant if the sample is large enough and a strong relationship can be statistically significant even if the sample is small. Table 13.1 shows roughly how relationship strength and sample size combine to determine whether a sample result is statistically significant. The columns of the table represent the three levels of relationship strength: weak, medium, and strong. The rows represent four sample sizes that can be considered small, medium, large, and extra large in the context of psychological research. Thus each cell in the table represents a combination of relationship strength and sample size. If a cell contains the word Yes , then this combination would be statistically significant for both Cohen’s d and Pearson’s r . If it contains the word No , then it would not be statistically significant for either. There is one cell where the decision for d and r would be different and another where it might be different depending on some additional considerations, which are discussed in Section 13.2 “Some Basic Null Hypothesis Tests”
Sample Size | Weak | Medium | Strong |
Small ( = 20) | No | No | = Maybe = Yes |
Medium ( = 50) | No | Yes | Yes |
Large ( = 100) | = Yes = No | Yes | Yes |
Extra large ( = 500) | Yes | Yes | Yes |
Although Table 13.1 provides only a rough guideline, it shows very clearly that weak relationships based on medium or small samples are never statistically significant and that strong relationships based on medium or larger samples are always statistically significant. If you keep this lesson in mind, you will often know whether a result is statistically significant based on the descriptive statistics alone. It is extremely useful to be able to develop this kind of intuitive judgment. One reason is that it allows you to develop expectations about how your formal null hypothesis tests are going to come out, which in turn allows you to detect problems in your analyses. For example, if your sample relationship is strong and your sample is medium, then you would expect to reject the null hypothesis. If for some reason your formal null hypothesis test indicates otherwise, then you need to double-check your computations and interpretations. A second reason is that the ability to make this kind of intuitive judgment is an indication that you understand the basic logic of this approach in addition to being able to do the computations.
Statistical Significance Versus Practical Significance
Table 13.1 illustrates another extremely important point. A statistically significant result is not necessarily a strong one. Even a very weak result can be statistically significant if it is based on a large enough sample. This is closely related to Janet Shibley Hyde’s argument about sex differences (Hyde, 2007) [2] . The differences between women and men in mathematical problem solving and leadership ability are statistically significant. But the word significant can cause people to interpret these differences as strong and important—perhaps even important enough to influence the college courses they take or even who they vote for. As we have seen, however, these statistically significant differences are actually quite weak—perhaps even “trivial.”
This is why it is important to distinguish between the statistical significance of a result and the practical significance of that result. Practical significance refers to the importance or usefulness of the result in some real-world context. Many sex differences are statistically significant—and may even be interesting for purely scientific reasons—but they are not practically significant. In clinical practice, this same concept is often referred to as “clinical significance.” For example, a study on a new treatment for social phobia might show that it produces a statistically significant positive effect. Yet this effect still might not be strong enough to justify the time, effort, and other costs of putting it into practice—especially if easier and cheaper treatments that work almost as well already exist. Although statistically significant, this result would be said to lack practical or clinical significance.

“Conditional Risk” retrieved from http://imgs.xkcd.com/comics/conditional_risk.png (CC-BY-NC 2.5)
Key Takeaways
- Null hypothesis testing is a formal approach to deciding whether a statistical relationship in a sample reflects a real relationship in the population or is just due to chance.
- The logic of null hypothesis testing involves assuming that the null hypothesis is true, finding how likely the sample result would be if this assumption were correct, and then making a decision. If the sample result would be unlikely if the null hypothesis were true, then it is rejected in favor of the alternative hypothesis. If it would not be unlikely, then the null hypothesis is retained.
- The probability of obtaining the sample result if the null hypothesis were true (the p value) is based on two considerations: relationship strength and sample size. Reasonable judgments about whether a sample relationship is statistically significant can often be made by quickly considering these two factors.
- Statistical significance is not the same as relationship strength or importance. Even weak relationships can be statistically significant if the sample size is large enough. It is important to consider relationship strength and the practical significance of a result in addition to its statistical significance.
- Discussion: Imagine a study showing that people who eat more broccoli tend to be happier. Explain for someone who knows nothing about statistics why the researchers would conduct a null hypothesis test.
- The correlation between two variables is r = −.78 based on a sample size of 137.
- The mean score on a psychological characteristic for women is 25 ( SD = 5) and the mean score for men is 24 ( SD = 5). There were 12 women and 10 men in this study.
- In a memory experiment, the mean number of items recalled by the 40 participants in Condition A was 0.50 standard deviations greater than the mean number recalled by the 40 participants in Condition B.
- In another memory experiment, the mean scores for participants in Condition A and Condition B came out exactly the same!
- A student finds a correlation of r = .04 between the number of units the students in his research methods class are taking and the students’ level of stress.
- Cohen, J. (1994). The world is round: p < .05. American Psychologist, 49 , 997–1003. ↵
- Hyde, J. S. (2007). New directions in the study of gender similarities and differences. Current Directions in Psychological Science, 16 , 259–263. ↵

Share This Book
- Increase Font Size
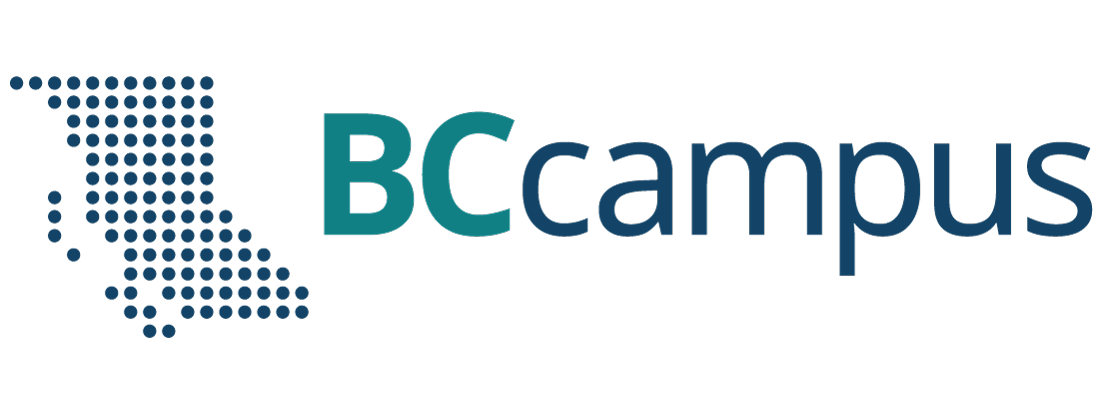
Want to create or adapt books like this? Learn more about how Pressbooks supports open publishing practices.
Chapter 13: Inferential Statistics
Understanding Null Hypothesis Testing
Learning Objectives
- Explain the purpose of null hypothesis testing, including the role of sampling error.
- Describe the basic logic of null hypothesis testing.
- Describe the role of relationship strength and sample size in determining statistical significance and make reasonable judgments about statistical significance based on these two factors.
The Purpose of Null Hypothesis Testing
As we have seen, psychological research typically involves measuring one or more variables for a sample and computing descriptive statistics for that sample. In general, however, the researcher’s goal is not to draw conclusions about that sample but to draw conclusions about the population that the sample was selected from. Thus researchers must use sample statistics to draw conclusions about the corresponding values in the population. These corresponding values in the population are called parameters . Imagine, for example, that a researcher measures the number of depressive symptoms exhibited by each of 50 clinically depressed adults and computes the mean number of symptoms. The researcher probably wants to use this sample statistic (the mean number of symptoms for the sample) to draw conclusions about the corresponding population parameter (the mean number of symptoms for clinically depressed adults).
Unfortunately, sample statistics are not perfect estimates of their corresponding population parameters. This is because there is a certain amount of random variability in any statistic from sample to sample. The mean number of depressive symptoms might be 8.73 in one sample of clinically depressed adults, 6.45 in a second sample, and 9.44 in a third—even though these samples are selected randomly from the same population. Similarly, the correlation (Pearson’s r ) between two variables might be +.24 in one sample, −.04 in a second sample, and +.15 in a third—again, even though these samples are selected randomly from the same population. This random variability in a statistic from sample to sample is called sampling error . (Note that the term error here refers to random variability and does not imply that anyone has made a mistake. No one “commits a sampling error.”)
One implication of this is that when there is a statistical relationship in a sample, it is not always clear that there is a statistical relationship in the population. A small difference between two group means in a sample might indicate that there is a small difference between the two group means in the population. But it could also be that there is no difference between the means in the population and that the difference in the sample is just a matter of sampling error. Similarly, a Pearson’s r value of −.29 in a sample might mean that there is a negative relationship in the population. But it could also be that there is no relationship in the population and that the relationship in the sample is just a matter of sampling error.
In fact, any statistical relationship in a sample can be interpreted in two ways:
- There is a relationship in the population, and the relationship in the sample reflects this.
- There is no relationship in the population, and the relationship in the sample reflects only sampling error.
The purpose of null hypothesis testing is simply to help researchers decide between these two interpretations.
The Logic of Null Hypothesis Testing
Null hypothesis testing is a formal approach to deciding between two interpretations of a statistical relationship in a sample. One interpretation is called the null hypothesis (often symbolized H 0 and read as “H-naught”). This is the idea that there is no relationship in the population and that the relationship in the sample reflects only sampling error. Informally, the null hypothesis is that the sample relationship “occurred by chance.” The other interpretation is called the alternative hypothesis (often symbolized as H 1 ). This is the idea that there is a relationship in the population and that the relationship in the sample reflects this relationship in the population.
Again, every statistical relationship in a sample can be interpreted in either of these two ways: It might have occurred by chance, or it might reflect a relationship in the population. So researchers need a way to decide between them. Although there are many specific null hypothesis testing techniques, they are all based on the same general logic. The steps are as follows:
- Assume for the moment that the null hypothesis is true. There is no relationship between the variables in the population.
- Determine how likely the sample relationship would be if the null hypothesis were true.
- If the sample relationship would be extremely unlikely, then reject the null hypothesis in favour of the alternative hypothesis. If it would not be extremely unlikely, then retain the null hypothesis .
Following this logic, we can begin to understand why Mehl and his colleagues concluded that there is no difference in talkativeness between women and men in the population. In essence, they asked the following question: “If there were no difference in the population, how likely is it that we would find a small difference of d = 0.06 in our sample?” Their answer to this question was that this sample relationship would be fairly likely if the null hypothesis were true. Therefore, they retained the null hypothesis—concluding that there is no evidence of a sex difference in the population. We can also see why Kanner and his colleagues concluded that there is a correlation between hassles and symptoms in the population. They asked, “If the null hypothesis were true, how likely is it that we would find a strong correlation of +.60 in our sample?” Their answer to this question was that this sample relationship would be fairly unlikely if the null hypothesis were true. Therefore, they rejected the null hypothesis in favour of the alternative hypothesis—concluding that there is a positive correlation between these variables in the population.
A crucial step in null hypothesis testing is finding the likelihood of the sample result if the null hypothesis were true. This probability is called the p value . A low p value means that the sample result would be unlikely if the null hypothesis were true and leads to the rejection of the null hypothesis. A high p value means that the sample result would be likely if the null hypothesis were true and leads to the retention of the null hypothesis. But how low must the p value be before the sample result is considered unlikely enough to reject the null hypothesis? In null hypothesis testing, this criterion is called α (alpha) and is almost always set to .05. If there is less than a 5% chance of a result as extreme as the sample result if the null hypothesis were true, then the null hypothesis is rejected. When this happens, the result is said to be statistically significant . If there is greater than a 5% chance of a result as extreme as the sample result when the null hypothesis is true, then the null hypothesis is retained. This does not necessarily mean that the researcher accepts the null hypothesis as true—only that there is not currently enough evidence to conclude that it is true. Researchers often use the expression “fail to reject the null hypothesis” rather than “retain the null hypothesis,” but they never use the expression “accept the null hypothesis.”
The Misunderstood p Value
The p value is one of the most misunderstood quantities in psychological research (Cohen, 1994) [1] . Even professional researchers misinterpret it, and it is not unusual for such misinterpretations to appear in statistics textbooks!
The most common misinterpretation is that the p value is the probability that the null hypothesis is true—that the sample result occurred by chance. For example, a misguided researcher might say that because the p value is .02, there is only a 2% chance that the result is due to chance and a 98% chance that it reflects a real relationship in the population. But this is incorrect . The p value is really the probability of a result at least as extreme as the sample result if the null hypothesis were true. So a p value of .02 means that if the null hypothesis were true, a sample result this extreme would occur only 2% of the time.
You can avoid this misunderstanding by remembering that the p value is not the probability that any particular hypothesis is true or false. Instead, it is the probability of obtaining the sample result if the null hypothesis were true.
Role of Sample Size and Relationship Strength
Recall that null hypothesis testing involves answering the question, “If the null hypothesis were true, what is the probability of a sample result as extreme as this one?” In other words, “What is the p value?” It can be helpful to see that the answer to this question depends on just two considerations: the strength of the relationship and the size of the sample. Specifically, the stronger the sample relationship and the larger the sample, the less likely the result would be if the null hypothesis were true. That is, the lower the p value. This should make sense. Imagine a study in which a sample of 500 women is compared with a sample of 500 men in terms of some psychological characteristic, and Cohen’s d is a strong 0.50. If there were really no sex difference in the population, then a result this strong based on such a large sample should seem highly unlikely. Now imagine a similar study in which a sample of three women is compared with a sample of three men, and Cohen’s d is a weak 0.10. If there were no sex difference in the population, then a relationship this weak based on such a small sample should seem likely. And this is precisely why the null hypothesis would be rejected in the first example and retained in the second.
Of course, sometimes the result can be weak and the sample large, or the result can be strong and the sample small. In these cases, the two considerations trade off against each other so that a weak result can be statistically significant if the sample is large enough and a strong relationship can be statistically significant even if the sample is small. Table 13.1 shows roughly how relationship strength and sample size combine to determine whether a sample result is statistically significant. The columns of the table represent the three levels of relationship strength: weak, medium, and strong. The rows represent four sample sizes that can be considered small, medium, large, and extra large in the context of psychological research. Thus each cell in the table represents a combination of relationship strength and sample size. If a cell contains the word Yes , then this combination would be statistically significant for both Cohen’s d and Pearson’s r . If it contains the word No , then it would not be statistically significant for either. There is one cell where the decision for d and r would be different and another where it might be different depending on some additional considerations, which are discussed in Section 13.2 “Some Basic Null Hypothesis Tests”
Sample Size | Weak relationship | Medium-strength relationship | Strong relationship |
---|---|---|---|
Small ( = 20) | No | No | = Maybe = Yes |
Medium ( = 50) | No | Yes | Yes |
Large ( = 100) | = Yes = No | Yes | Yes |
Extra large ( = 500) | Yes | Yes | Yes |
Although Table 13.1 provides only a rough guideline, it shows very clearly that weak relationships based on medium or small samples are never statistically significant and that strong relationships based on medium or larger samples are always statistically significant. If you keep this lesson in mind, you will often know whether a result is statistically significant based on the descriptive statistics alone. It is extremely useful to be able to develop this kind of intuitive judgment. One reason is that it allows you to develop expectations about how your formal null hypothesis tests are going to come out, which in turn allows you to detect problems in your analyses. For example, if your sample relationship is strong and your sample is medium, then you would expect to reject the null hypothesis. If for some reason your formal null hypothesis test indicates otherwise, then you need to double-check your computations and interpretations. A second reason is that the ability to make this kind of intuitive judgment is an indication that you understand the basic logic of this approach in addition to being able to do the computations.
Statistical Significance Versus Practical Significance
Table 13.1 illustrates another extremely important point. A statistically significant result is not necessarily a strong one. Even a very weak result can be statistically significant if it is based on a large enough sample. This is closely related to Janet Shibley Hyde’s argument about sex differences (Hyde, 2007) [2] . The differences between women and men in mathematical problem solving and leadership ability are statistically significant. But the word significant can cause people to interpret these differences as strong and important—perhaps even important enough to influence the college courses they take or even who they vote for. As we have seen, however, these statistically significant differences are actually quite weak—perhaps even “trivial.”
This is why it is important to distinguish between the statistical significance of a result and the practical significance of that result. Practical significance refers to the importance or usefulness of the result in some real-world context. Many sex differences are statistically significant—and may even be interesting for purely scientific reasons—but they are not practically significant. In clinical practice, this same concept is often referred to as “clinical significance.” For example, a study on a new treatment for social phobia might show that it produces a statistically significant positive effect. Yet this effect still might not be strong enough to justify the time, effort, and other costs of putting it into practice—especially if easier and cheaper treatments that work almost as well already exist. Although statistically significant, this result would be said to lack practical or clinical significance.
Key Takeaways
- Null hypothesis testing is a formal approach to deciding whether a statistical relationship in a sample reflects a real relationship in the population or is just due to chance.
- The logic of null hypothesis testing involves assuming that the null hypothesis is true, finding how likely the sample result would be if this assumption were correct, and then making a decision. If the sample result would be unlikely if the null hypothesis were true, then it is rejected in favour of the alternative hypothesis. If it would not be unlikely, then the null hypothesis is retained.
- The probability of obtaining the sample result if the null hypothesis were true (the p value) is based on two considerations: relationship strength and sample size. Reasonable judgments about whether a sample relationship is statistically significant can often be made by quickly considering these two factors.
- Statistical significance is not the same as relationship strength or importance. Even weak relationships can be statistically significant if the sample size is large enough. It is important to consider relationship strength and the practical significance of a result in addition to its statistical significance.
- Discussion: Imagine a study showing that people who eat more broccoli tend to be happier. Explain for someone who knows nothing about statistics why the researchers would conduct a null hypothesis test.
- The correlation between two variables is r = −.78 based on a sample size of 137.
- The mean score on a psychological characteristic for women is 25 ( SD = 5) and the mean score for men is 24 ( SD = 5). There were 12 women and 10 men in this study.
- In a memory experiment, the mean number of items recalled by the 40 participants in Condition A was 0.50 standard deviations greater than the mean number recalled by the 40 participants in Condition B.
- In another memory experiment, the mean scores for participants in Condition A and Condition B came out exactly the same!
- A student finds a correlation of r = .04 between the number of units the students in his research methods class are taking and the students’ level of stress.
Long Descriptions
“Null Hypothesis” long description: A comic depicting a man and a woman talking in the foreground. In the background is a child working at a desk. The man says to the woman, “I can’t believe schools are still teaching kids about the null hypothesis. I remember reading a big study that conclusively disproved it years ago.” [Return to “Null Hypothesis”]
“Conditional Risk” long description: A comic depicting two hikers beside a tree during a thunderstorm. A bolt of lightning goes “crack” in the dark sky as thunder booms. One of the hikers says, “Whoa! We should get inside!” The other hiker says, “It’s okay! Lightning only kills about 45 Americans a year, so the chances of dying are only one in 7,000,000. Let’s go on!” The comic’s caption says, “The annual death rate among people who know that statistic is one in six.” [Return to “Conditional Risk”]
Media Attributions
- Null Hypothesis by XKCD CC BY-NC (Attribution NonCommercial)
- Conditional Risk by XKCD CC BY-NC (Attribution NonCommercial)
- Cohen, J. (1994). The world is round: p < .05. American Psychologist, 49 , 997–1003. ↵
- Hyde, J. S. (2007). New directions in the study of gender similarities and differences. Current Directions in Psychological Science, 16 , 259–263. ↵
Values in a population that correspond to variables measured in a study.
The random variability in a statistic from sample to sample.
A formal approach to deciding between two interpretations of a statistical relationship in a sample.
The idea that there is no relationship in the population and that the relationship in the sample reflects only sampling error.
The idea that there is a relationship in the population and that the relationship in the sample reflects this relationship in the population.
When the relationship found in the sample would be extremely unlikely, the idea that the relationship occurred “by chance” is rejected.
When the relationship found in the sample is likely to have occurred by chance, the null hypothesis is not rejected.
The probability that, if the null hypothesis were true, the result found in the sample would occur.
How low the p value must be before the sample result is considered unlikely in null hypothesis testing.
When there is less than a 5% chance of a result as extreme as the sample result occurring and the null hypothesis is rejected.
Research Methods in Psychology - 2nd Canadian Edition Copyright © 2015 by Paul C. Price, Rajiv Jhangiani, & I-Chant A. Chiang is licensed under a Creative Commons Attribution-NonCommercial-ShareAlike 4.0 International License , except where otherwise noted.
Share This Book

IMAGES
VIDEO
COMMENTS
Null & Alternative Hypotheses | Definitions, Templates & ...
Null Hypothesis: Definition, Rejecting & Examples
The Null hypothesis \(\left(H_{O}\right)\) is a statement about the comparisons, e.g., between a sample statistic and the population, or between two treatment groups. The former is referred to as a one-tailed test whereas the latter is called a two-tailed test. The null hypothesis is typically "no statistical difference" between the ...
Null hypothesis - Wikipedia ... Null hypothesis
The null and alternative hypotheses offer competing answers to your research question. When the research question asks "Does the independent variable affect the dependent variable?", the null hypothesis (H 0) answers "No, there's no effect in the population.". On the other hand, the alternative hypothesis (H A) answers "Yes, there ...
H 0 (Null Hypothesis): Population parameter =, ≤, ≥ some value. H A (Alternative Hypothesis): Population parameter <, >, ≠ some value. Note that the null hypothesis always contains the equal sign. We interpret the hypotheses as follows: Null hypothesis: The sample data provides no evidence to support some claim being made by an individual.
A tutorial on a practical Bayesian alternative to null-hypothesis significance testing. Behavior research methods, 43, 679-690. Nickerson, R. S. (2000). Null hypothesis significance testing: a review of an old and continuing controversy. Psychological methods, 5(2), 241. Rozeboom, W. W. (1960). The fallacy of the null-hypothesis significance test.
How to Formulate a Null Hypothesis (With Examples)
This null hypothesis can be written as: H0: X¯ = μ H 0: X ¯ = μ. For most of this textbook, the null hypothesis is that the means of the two groups are similar. Much later, the null hypothesis will be that there is no relationship between the two groups. Either way, remember that a null hypothesis is always saying that nothing is different.
Definition. In formal hypothesis testing, the null hypothesis (H0) is the hypothesis assumed to be true in the population and which gives rise to the sampling distribution of the test statistic in question (Hays 1994). The critical feature of the null hypothesis across hypothesis testing frameworks is that it is stated with enough precision ...
Null Hypothesis Examples. "Hyperactivity is unrelated to eating sugar " is an example of a null hypothesis. If the hypothesis is tested and found to be false, using statistics, then a connection between hyperactivity and sugar ingestion may be indicated. A significance test is the most common statistical test used to establish confidence in a ...
Null Hypothesis Definition and Examples, How to State
6. Write a null hypothesis. If your research involves statistical hypothesis testing, you will also have to write a null hypothesis. The null hypothesis is the default position that there is no association between the variables. The null hypothesis is written as H 0, while the alternative hypothesis is H 1 or H a.
The actual test begins by considering two hypotheses.They are called the null hypothesis and the alternative hypothesis.These hypotheses contain opposing viewpoints. H 0, the —null hypothesis: a statement of no difference between sample means or proportions or no difference between a sample mean or proportion and a population mean or proportion. In other words, the difference equals 0.
The actual test begins by considering two hypotheses.They are called the null hypothesis and the alternative hypothesis.These hypotheses contain opposing viewpoints. \(H_0\): The null hypothesis: It is a statement of no difference between the variables—they are not related. This can often be considered the status quo and as a result if you cannot accept the null it requires some action.
A crucial step in null hypothesis testing is finding the likelihood of the sample result if the null hypothesis were true. This probability is called the p value. A low p value means that the sample result would be unlikely if the null hypothesis were true and leads to the rejection of the null hypothesis. A high p value means that the sample ...
Misunderstanding 2: A Small p-value Means the Null Hypothesis is False. Clarification: A small p-value shows that your data is unlikely if the null hypothesis is true. It suggests that the alternative hypothesis might be right, but it doesn't prove the null hypothesis is false. Misunderstanding 3: The Significance Level (Alpha) Can Be Chosen ...
What is Null Hypothesis? What Is Its Importance ...
An example of the null hypothesis is that light color has no effect on plant growth. The null hypothesis (H 0) is the hypothesis that states there is no statistical difference between two sample sets. In other words, it assumes the independent variable does not have an effect on the dependent variable in a scientific experiment.
The Purpose of Null Hypothesis Testing. As we have seen, psychological research typically involves measuring one or more variables in a sample and computing descriptive summary data (e.g., means, correlation coefficients) for those variables. These descriptive data for the sample are called statistics. In general, however, the researcher's ...
Write a research null hypothesis as a statement that the studied variables have no relationship to each other, or that there's no difference between 2 groups. Write a statistical null hypothesis as a mathematical equation, such as. μ 1 = μ 2 {\displaystyle \mu _ {1}=\mu _ {2}} if you're comparing group means.
Null and alternative hypotheses are used in statistical hypothesis testing. The null hypothesis of a test always predicts no effect or no relationship between variables, while the alternative hypothesis states your research prediction of an effect or relationship.
For Bayesian t-tests and correlations, we reported the BF 10 value, indicating the ratio of the likelihood of an alternative hypothesis to a null hypothesis. We considered BF incl/10 > 3 and BF incl/10 < 1/3 as evidence for alternative and null hypotheses respectively, while 1/3 < BF incl/10 < 3 as the absence of evidence (Keysers et al., 2020 ...
The Logic of Null Hypothesis Testing. Null hypothesis testing is a formal approach to deciding between two interpretations of a statistical relationship in a sample. One interpretation is called the null hypothesis (often symbolized H 0 and read as "H-naught"). This is the idea that there is no relationship in the population and that the ...
A null model was established to determine the community assembly processes of each taxon using the EcoSimR package in R (Gotelli et al., 2015). The communities were randomized 1,000 times using a SIM2 randomization algorithm (which had the best statistical properties in terms of Type I and II errors) to generate a null distribution (Gotelli ...
A crucial step in null hypothesis testing is finding the likelihood of the sample result if the null hypothesis were true. This probability is called the p value. A low p value means that the sample result would be unlikely if the null hypothesis were true and leads to the rejection of the null hypothesis. A high p value means that the sample ...