- Bipolar Disorder
- Therapy Center
- When To See a Therapist
- Types of Therapy
- Best Online Therapy
- Best Couples Therapy
- Managing Stress
- Sleep and Dreaming
- Understanding Emotions
- Self-Improvement
- Healthy Relationships
- Student Resources
- Personality Types
- Sweepstakes
- Guided Meditations
- Verywell Mind Insights
- 2024 Verywell Mind 25
- Mental Health in the Classroom
- Editorial Process
- Meet Our Review Board
- Crisis Support

Internal Validity vs. External Validity in Research
What they tell us about the meaningfulness and trustworthiness of research
Verywell / Bailey Mariner
- Internal Validity
- External Validity
How do you determine whether a psychology study is trustworthy and meaningful? Two characteristics that can help you assess research findings are internal and external validity.
- Internal validity measures how well a study is conducted (its structure) and how accurately its results reflect the studied group.
- External validity relates to how applicable the findings are in the real world.
These two concepts help researchers gauge if the results of a research study are trustworthy and meaningful.
Conclusions are warranted
Controls extraneous variables
Eliminates alternative explanations
Focus on accuracy and strong research methods
Findings can be generalized
Outcomes apply to practical situations
Results apply to the world at large
Results can be translated into another context
What Is Internal Validity in Research?
Internal validity is the extent to which a research study establishes a trustworthy cause-and-effect relationship. This type of validity depends largely on the study's procedures and how rigorously it is performed.
Internal validity is important because once established, it makes it possible to eliminate alternative explanations for a finding. If you implement a smoking cessation program, for instance, internal validity ensures that any improvement in the subjects is due to the treatment administered and not something else.
Internal validity is not a "yes or no" concept. Instead, we consider how confident we can be with study findings based on whether the research avoids traps that may make those findings questionable. The less chance there is for "confounding," the higher the internal validity and the more confident we can be.
Confounding refers to uncontrollable variables that come into play and can confuse the outcome of a study, making us unsure of whether we can trust that we have identified the cause-and-effect relationship.
In short, you can only be confident that a study is internally valid if you can rule out alternative explanations for the findings. Three criteria are required to assume cause and effect in a research study:
- The cause preceded the effect in terms of time.
- The cause and effect vary together.
- There are no other likely explanations for the relationship observed.
Factors That Improve Internal Validity
To ensure the internal validity of a study, you want to consider aspects of the research design that will increase the likelihood that you can reject alternative hypotheses. Many factors can improve internal validity in research, including:
- Blinding : Participants—and sometimes researchers—are unaware of what intervention they are receiving (such as using a placebo on some subjects in a medication study) to avoid having this knowledge bias their perceptions and behaviors, thus impacting the study's outcome
- Experimental manipulation : Manipulating an independent variable in a study (for instance, giving smokers a cessation program) instead of just observing an association without conducting any intervention (examining the relationship between exercise and smoking behavior)
- Random selection : Choosing participants at random or in a manner in which they are representative of the population that you wish to study
- Randomization or random assignment : Randomly assigning participants to treatment and control groups, ensuring that there is no systematic bias between the research groups
- Strict study protocol : Following specific procedures during the study so as not to introduce any unintended effects; for example, doing things differently with one group of study participants than you do with another group
Internal Validity Threats
Just as there are many ways to ensure internal validity, a list of potential threats should be considered when planning a study.
- Attrition : Participants dropping out or leaving a study, which means that the results are based on a biased sample of only the people who did not choose to leave (and possibly who all have something in common, such as higher motivation)
- Confounding : A situation in which changes in an outcome variable can be thought to have resulted from some type of outside variable not measured or manipulated in the study
- Diffusion : This refers to the results of one group transferring to another through the groups interacting and talking with or observing one another; this can also lead to another issue called resentful demoralization, in which a control group tries less hard because they feel resentful over the group that they are in
- Experimenter bias : An experimenter behaving in a different way with different groups in a study, which can impact the results (and is eliminated through blinding)
- Historical events : May influence the outcome of studies that occur over a period of time, such as a change in the political leader or a natural disaster that occurs, influencing how study participants feel and act
- Instrumentation : This involves "priming" participants in a study in certain ways with the measures used, causing them to react in a way that is different than they would have otherwise reacted
- Maturation : The impact of time as a variable in a study; for example, if a study takes place over a period of time in which it is possible that participants naturally change in some way (i.e., they grew older or became tired), it may be impossible to rule out whether effects seen in the study were simply due to the impact of time
- Statistical regression : The natural effect of participants at extreme ends of a measure falling in a certain direction due to the passage of time rather than being a direct effect of an intervention
- Testing : Repeatedly testing participants using the same measures influences outcomes; for example, if you give someone the same test three times, it is likely that they will do better as they learn the test or become used to the testing process, causing them to answer differently
What Is External Validity in Research?
External validity refers to how well the outcome of a research study can be expected to apply to other settings. This is important because, if external validity is established, it means that the findings can be generalizable to similar individuals or populations.
External validity affirmatively answers the question: Do the findings apply to similar people, settings, situations, and time periods?
Population validity and ecological validity are two types of external validity. Population validity refers to whether you can generalize the research outcomes to other populations or groups. Ecological validity refers to whether a study's findings can be generalized to additional situations or settings.
Another term called transferability refers to whether results transfer to situations with similar characteristics. Transferability relates to external validity and refers to a qualitative research design.
Factors That Improve External Validity
If you want to improve the external validity of your study, there are many ways to achieve this goal. Factors that can enhance external validity include:
- Field experiments : Conducting a study outside the laboratory, in a natural setting
- Inclusion and exclusion criteria : Setting criteria as to who can be involved in the research, ensuring that the population being studied is clearly defined
- Psychological realism : Making sure participants experience the events of the study as being real by telling them a "cover story," or a different story about the aim of the study so they don't behave differently than they would in real life based on knowing what to expect or knowing the study's goal
- Replication : Conducting the study again with different samples or in different settings to see if you get the same results; when many studies have been conducted on the same topic, a meta-analysis can also be used to determine if the effect of an independent variable can be replicated, therefore making it more reliable
- Reprocessing or calibration : Using statistical methods to adjust for external validity issues, such as reweighting groups if a study had uneven groups for a particular characteristic (such as age)
External Validity Threats
External validity is threatened when a study does not take into account the interaction of variables in the real world. Threats to external validity include:
- Pre- and post-test effects : When the pre- or post-test is in some way related to the effect seen in the study, such that the cause-and-effect relationship disappears without these added tests
- Sample features : When some feature of the sample used was responsible for the effect (or partially responsible), leading to limited generalizability of the findings
- Selection bias : Also considered a threat to internal validity, selection bias describes differences between groups in a study that may relate to the independent variable—like motivation or willingness to take part in the study, or specific demographics of individuals being more likely to take part in an online survey
- Situational factors : Factors such as the time of day of the study, its location, noise, researcher characteristics, and the number of measures used may affect the generalizability of findings
While rigorous research methods can ensure internal validity, external validity may be limited by these methods.
Internal Validity vs. External Validity
Internal validity and external validity are two research concepts that share a few similarities while also having several differences.
Similarities
One of the similarities between internal validity and external validity is that both factors should be considered when designing a study. This is because both have implications in terms of whether the results of a study have meaning.
Both internal validity and external validity are not "either/or" concepts. Therefore, you always need to decide to what degree a study performs in terms of each type of validity.
Each of these concepts is also typically reported in research articles published in scholarly journals . This is so that other researchers can evaluate the study and make decisions about whether the results are useful and valid.
Differences
The essential difference between internal validity and external validity is that internal validity refers to the structure of a study (and its variables) while external validity refers to the universality of the results. But there are further differences between the two as well.
For instance, internal validity focuses on showing a difference that is due to the independent variable alone. Conversely, external validity results can be translated to the world at large.
Internal validity and external validity aren't mutually exclusive. You can have a study with good internal validity but be overall irrelevant to the real world. You could also conduct a field study that is highly relevant to the real world but doesn't have trustworthy results in terms of knowing what variables caused the outcomes.
Examples of Validity
Perhaps the best way to understand internal validity and external validity is with examples.
Internal Validity Example
An example of a study with good internal validity would be if a researcher hypothesizes that using a particular mindfulness app will reduce negative mood. To test this hypothesis, the researcher randomly assigns a sample of participants to one of two groups: those who will use the app over a defined period and those who engage in a control task.
The researcher ensures that there is no systematic bias in how participants are assigned to the groups. They do this by blinding the research assistants so they don't know which groups the subjects are in during the experiment.
A strict study protocol is also used to outline the procedures of the study. Potential confounding variables are measured along with mood , such as the participants' socioeconomic status, gender, age, and other factors. If participants drop out of the study, their characteristics are examined to make sure there is no systematic bias in terms of who stays in.
External Validity Example
An example of a study with good external validity would be if, in the above example, the participants used the mindfulness app at home rather than in the laboratory. This shows that results appear in a real-world setting.
To further ensure external validity, the researcher clearly defines the population of interest and chooses a representative sample . They might also replicate the study's results using different technological devices.
Setting up an experiment so that it has both sound internal validity and external validity involves being mindful from the start about factors that can influence each aspect of your research.
It's best to spend extra time designing a structurally sound study that has far-reaching implications rather than to quickly rush through the design phase only to discover problems later on. Only when both internal validity and external validity are high can strong conclusions be made about your results.
Andrade C. Internal, external, and ecological validity in research design, conduct, and evaluation . Indian J Psychol Med . 2018;40(5):498-499. doi:10.4103/IJPSYM.IJPSYM_334_18
San Jose State University. Internal and external validity .
Kemper CJ. Internal validity . In: Zeigler-Hill V, Shackelford TK, eds. Encyclopedia of Personality and Individual Differences . Springer International Publishing; 2017:1-3. doi:10.1007/978-3-319-28099-8_1316-1
Patino CM, Ferreira JC. Internal and external validity: can you apply research study results to your patients? J Bras Pneumol . 2018;44(3):183. doi:10.1590/S1806-37562018000000164
Matthay EC, Glymour MM. A graphical catalog of threats to validity: Linking social science with epidemiology . Epidemiology . 2020;31(3):376-384. doi:10.1097/EDE.0000000000001161
Amico KR. Percent total attrition: a poor metric for study rigor in hosted intervention designs . Am J Public Health . 2009;99(9):1567-1575. doi:10.2105/AJPH.2008.134767
Kemper CJ. External validity . In: Zeigler-Hill V, Shackelford TK, eds. Encyclopedia of Personality and Individual Differences . Springer International Publishing; 2017:1-4. doi:10.1007/978-3-319-28099-8_1303-1
Desjardins E, Kurtz J, Kranke N, Lindeza A, Richter SH. Beyond standardization: improving external validity and reproducibility in experimental evolution . BioScience. 2021;71(5):543-552. doi:10.1093/biosci/biab008
Drude NI, Martinez Gamboa L, Danziger M, Dirnagl U, Toelch U. Improving preclinical studies through replications . Elife . 2021;10:e62101. doi:10.7554/eLife.62101
Michael RS. Threats to internal & external validity: Y520 strategies for educational inquiry .
Pahus L, Burgel PR, Roche N, Paillasseur JL, Chanez P. Randomized controlled trials of pharmacological treatments to prevent COPD exacerbations: applicability to real-life patients . BMC Pulm Med . 2019;19(1):127. doi:10.1186/s12890-019-0882-y
By Arlin Cuncic, MA Arlin Cuncic, MA, is the author of The Anxiety Workbook and founder of the website About Social Anxiety. She has a Master's degree in clinical psychology.
- Skip to main content
- Skip to primary sidebar
- Skip to footer
- QuestionPro

- Solutions Industries Gaming Automotive Sports and events Education Government Travel & Hospitality Financial Services Healthcare Cannabis Technology Use Case AskWhy Communities Audience Contactless surveys Mobile LivePolls Member Experience GDPR Positive People Science 360 Feedback Surveys
- Resources Blog eBooks Survey Templates Case Studies Training Help center

Home Market Research Research Tools and Apps
External Validity: Types, Research Methods & Examples
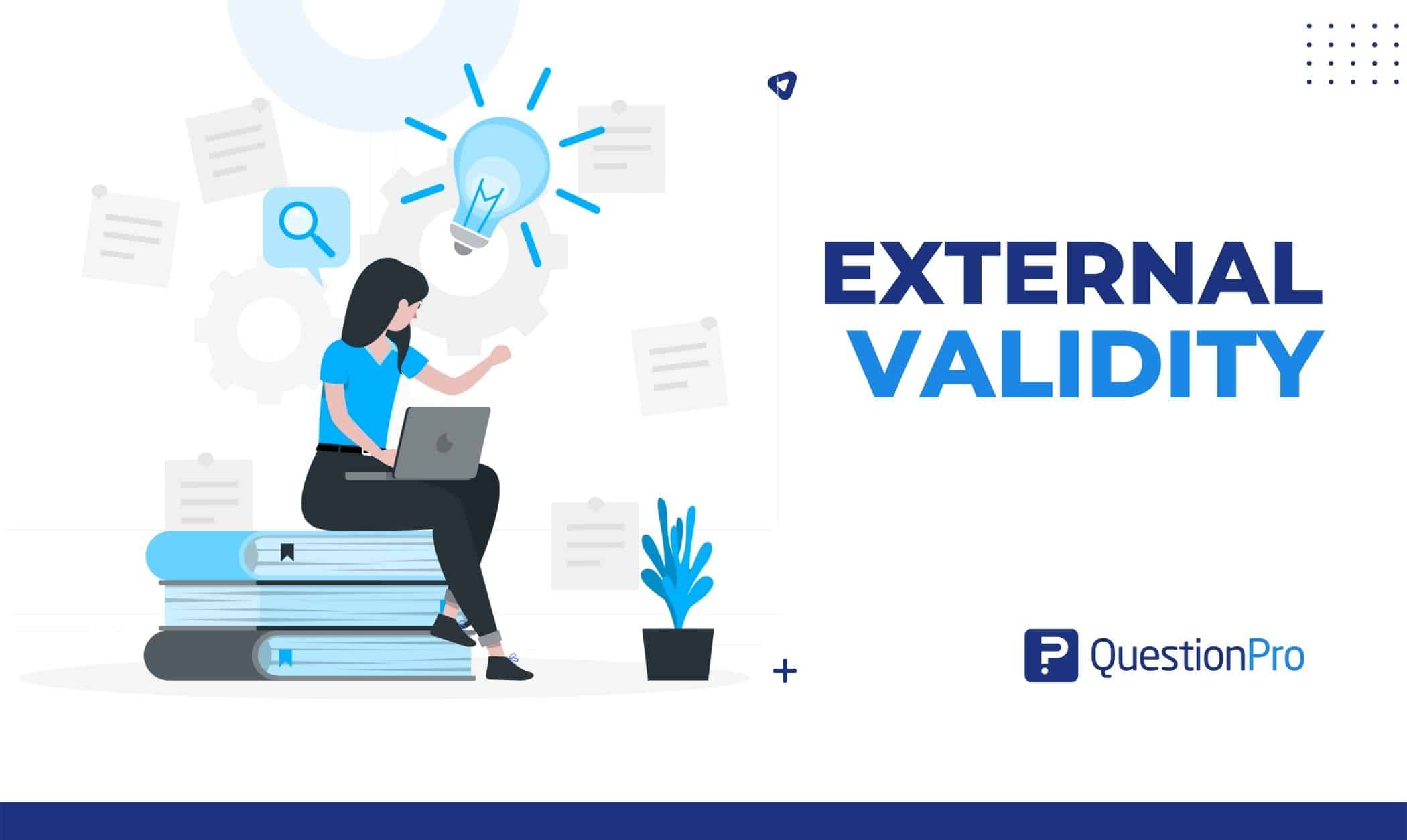
External validity is one of the main goals of researchers who want to find reliable cause-and-effect relationships in qualitative research.
When research has this validity, the results can be used with other people in different situations or places. Because without this validity, analysis can’t be generalized, and researchers can’t apply the results of studies to the real world. So, psychology research needs to be conducted outside a lab setting.
Still, sometimes they prefer to research how variables cause each other instead of being able to generalize the results.
In this article, we’ll talk about what external validity means, its types, and its research design methods.
LEARN ABOUT: Theoretical Research
What is external validity?
External validity describes how effectively the findings of an experiment may be generalized to different people, places, or times. Most scientific investigations do not intend to obtain outcomes that only apply to the few persons who participated in the study.
Instead, researchers want to be able to take the results of an experiment and use them with a larger group of people. It is a big part of what inferential statistics try to do.
For example, if you’re looking at a new drug or educational program, you don’t want to know that it works for only a few people. You want to use those results outside the experiment and beyond those participating. It is called “generalizability,” the essential part of this validity.
Types of external validity
Generally, there are three main types of this validity. We’ll discuss each one below and give examples to help you understand.
Population validity
Population validity is a kind of external validity that looks at how well the study’s results applied to a larger group of people. In this case, “population” refers to the group of people about whom a researcher is trying to conclude. On the other hand, a sample is a particular group of people who participate in the research.
If the results from the sample can apply to a larger group of people, then the study is valid for a large population.
Example: low population validity
You want to test the theory about how exercise and sleep are linked. You think that adults will sleep better when they do physical activities regularly. Your target group is adults in the United States, but your sample comprises about 300 college students.
Even though they are all adults, it might be hard to ensure the population validity in this case because the sampling model of students only represents some adults in the US.
So, your study has a limited amount of population validity, and you can only apply the results to some of the population.
Ecological validity
Ecological validity is another type of external validity that shows how well the research results can be used in different situations. In simple terms, ecological validity is about whether or not your results can be used in the real world.
So, if a study has a lot of ecological validity, the results can be used in the real world. On the other hand, low validity means that the results can’t be used outside the experiment.
Example: low ecological validity
The Milgram Experiment is a classic example of low ecological validity.
Stanley Milgram studied authority in the 1960s. He randomly chose participants and directed them to employ higher and higher voltage shocks to penalize wrong-answering actors. The study showed great obedience to authorities despite fake shock and victim behaviors.
The results of this study are revolutionary for the field of social psychology. However, it is often criticized because it has little ecological validity. Milgram’s set-up was not like real-life situations.
In the experiment, he set up a situation where the participants couldn’t avoid obeying the rules. But the reality of the issue can be very different.
Temporal validity
When figuring out external validity, time is just as important as the number of people involved and confusing factors.
The concept of temporal validity refers to how findings evolve. Particularly, this form of validity refers to how well the research results can be extended to another period.
High temporal validity means that research results can be used correctly in different times and places and that factors will be important in the future.
Imagine that you’re a psychologist, and you’re studying how people act the same.
You found out that social pressure from the majority group has a big effect on the choices of the minority. Because of this, people act similarly. Even though famous psychologist Solomon Asch did this research in the 1950s, the results can still be used in the real world today.
This study, therefore, has temporal validity even after nearly a century.
Research methods of external validity
There are a lot of methods you can do to improve the external validity of your research. Some things that can improve are given below:
Field experiments
Field experiments are like conducting research outside rather than in a controlled environment like a laboratory.
Criteria for inclusion and exclusion
Establishing criteria for who can participate in the research and ensuring that the group being examined is properly identified
Realism in psychology
If you want the participants to believe that the events that take place throughout the study are true, you should provide them with a cover story regarding the purpose of the research. So that they don’t behave any differently than they would in real life based on the fact.
Replication
Doing the study again with different samples or in a different place to see if you get the same results. When many studies have been done on the same topic, a meta-analysis can be used to see if the effect of an independent variable can be repeated to make it more reliable.
Reprocessing
It is like using statistical methods to fix problems with external validity, like reweighting groups if they were different in a certain way, such as age.
LEARN ABOUT: 12 Best Tools for Researchers
As stated in the article, the ability to replicate the results of an experiment is a key component of its external validity. Using the sampling methods the external validity can be improved in the research.
Researchers compare the results to other relevant data to determine the external validity. They can also do the research with more people from the target population. It’s hard to figure out external validity in research, but it’s important to use the results in the future.
The QuestionPro research suite is an enterprise-level research tool that can help you with your research process and surveys.
We at QuestionPro provide tools for data collection, such as our survey software, and a library of insights for any lengthy study. If you’re interested in seeing a demo or learning more, visit the Insight Hub.
LEARN MORE FREE TRIAL
MORE LIKE THIS
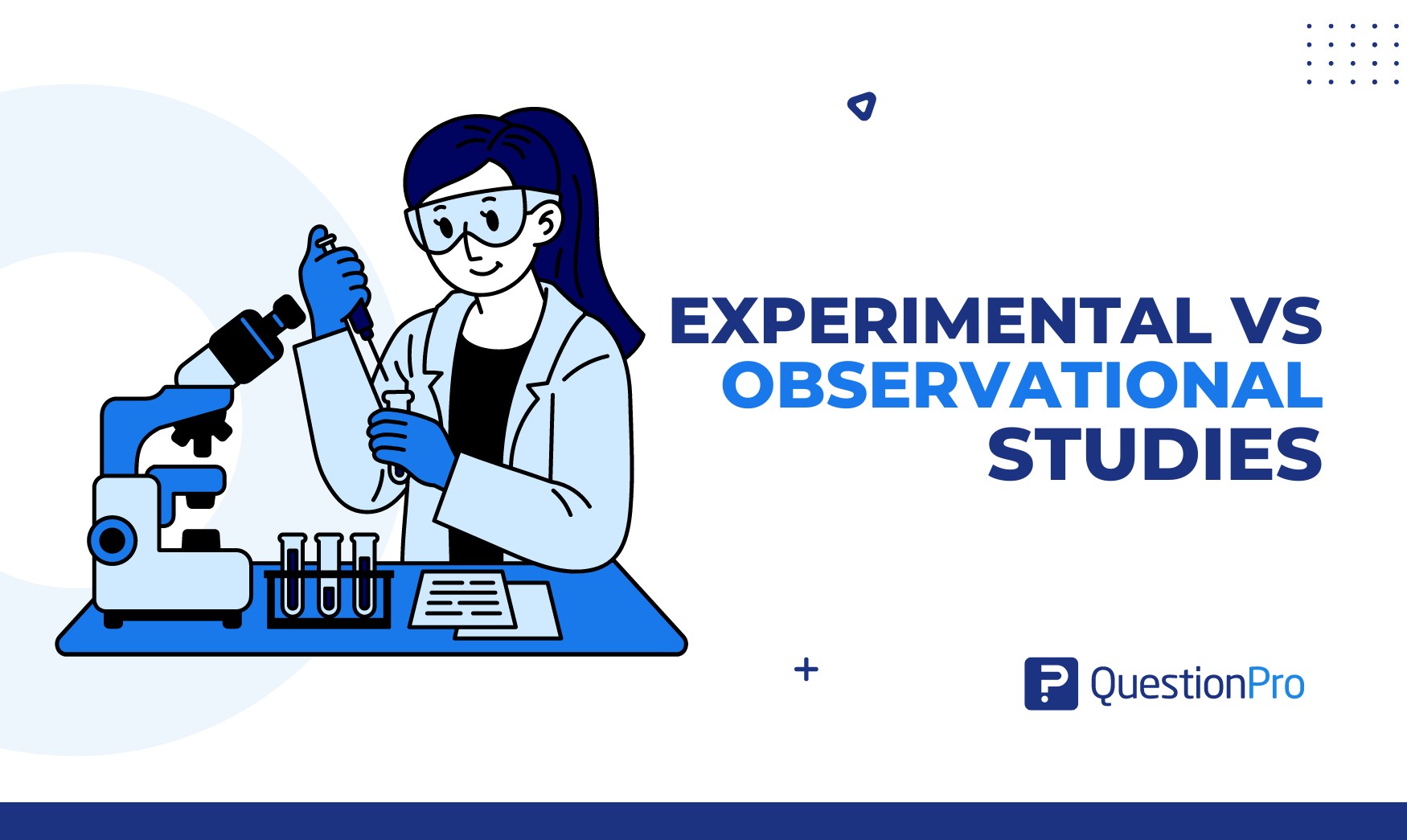
Experimental vs Observational Studies: Differences & Examples
Sep 5, 2024
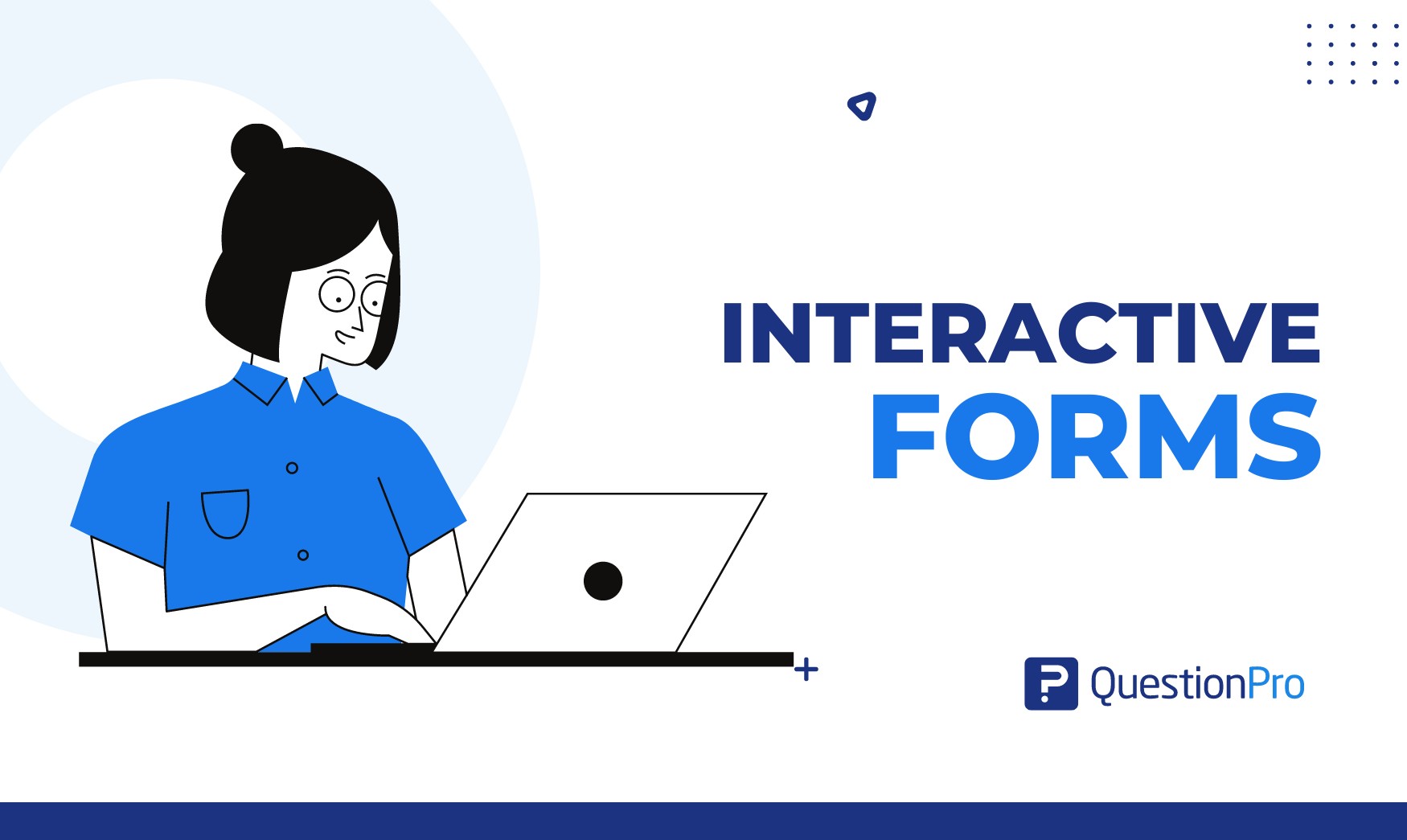
Interactive Forms: Key Features, Benefits, Uses + Design Tips
Sep 4, 2024
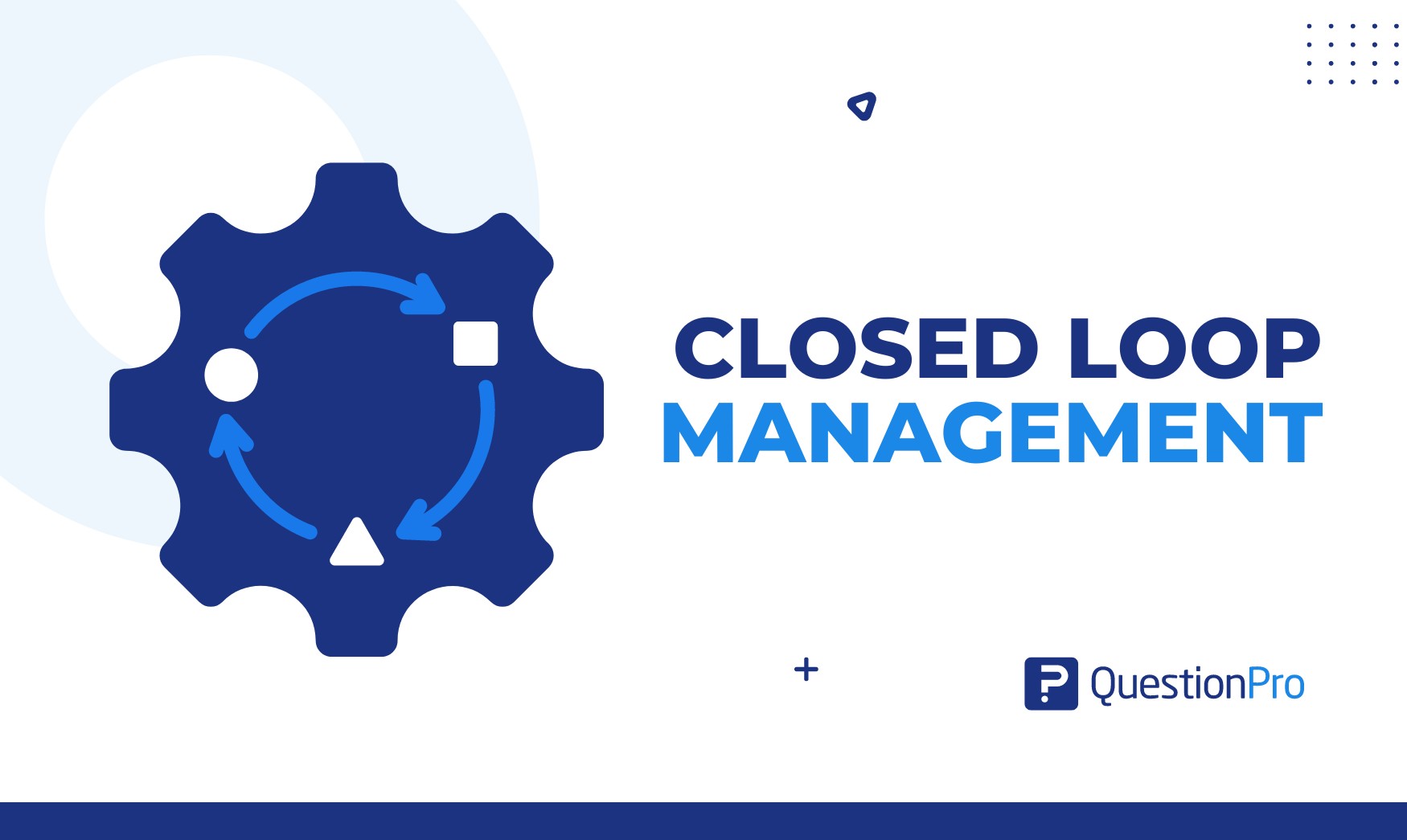
Closed-Loop Management: The Key to Customer Centricity
Sep 3, 2024
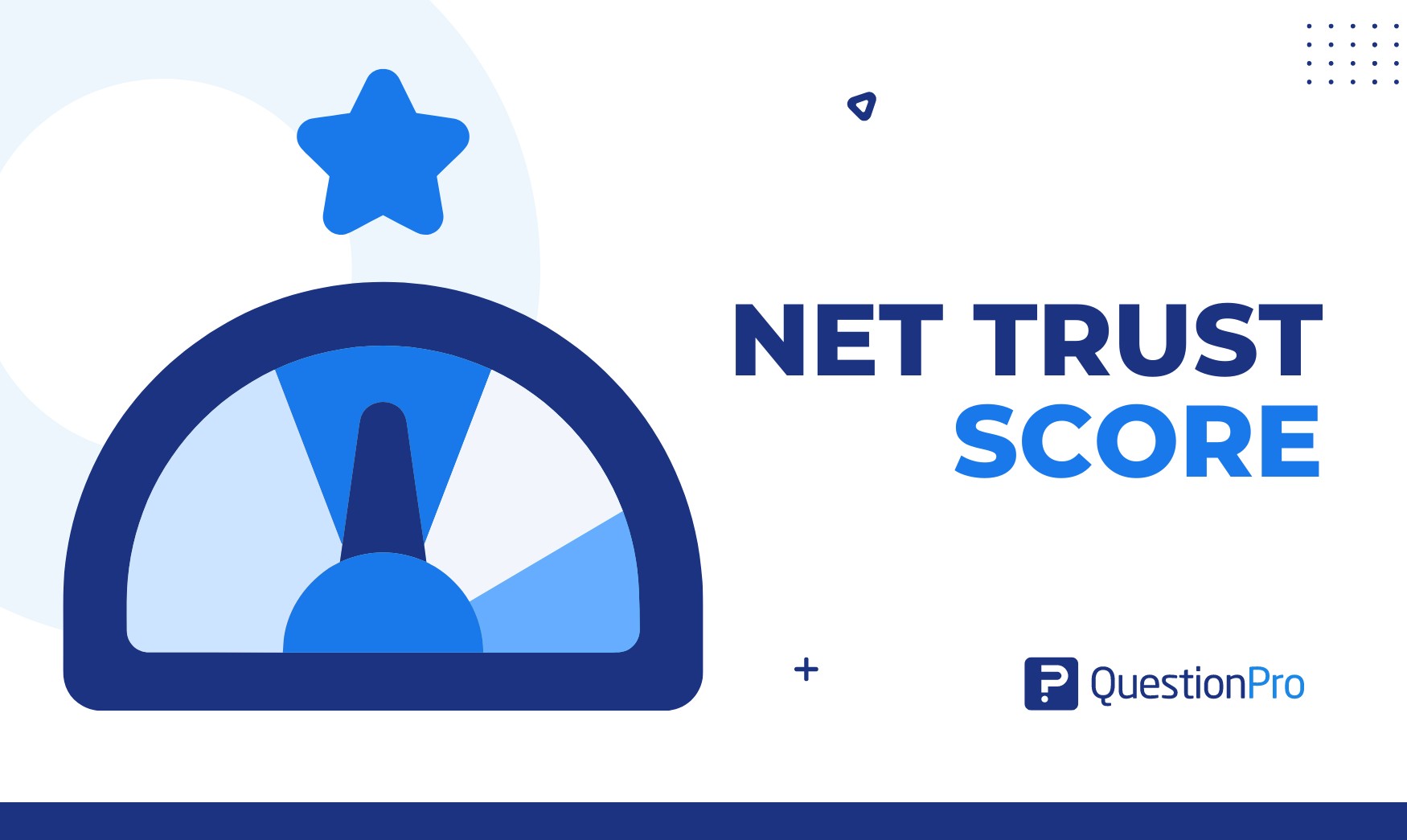
Net Trust Score: Tool for Measuring Trust in Organization
Sep 2, 2024
Other categories
- Academic Research
- Artificial Intelligence
- Assessments
- Brand Awareness
- Case Studies
- Communities
- Consumer Insights
- Customer effort score
- Customer Engagement
- Customer Experience
- Customer Loyalty
- Customer Research
- Customer Satisfaction
- Employee Benefits
- Employee Engagement
- Employee Retention
- Friday Five
- General Data Protection Regulation
- Insights Hub
- Life@QuestionPro
- Market Research
- Mobile diaries
- Mobile Surveys
- New Features
- Online Communities
- Question Types
- Questionnaire
- QuestionPro Products
- Release Notes
- Research Tools and Apps
- Revenue at Risk
- Survey Templates
- Training Tips
- Tuesday CX Thoughts (TCXT)
- Uncategorized
- What’s Coming Up
- Workforce Intelligence
External Validity
- Living reference work entry
- First Online: 10 February 2017
- Cite this living reference work entry
- Christoph J. Kemper 3
475 Accesses
External validity refers to the degree to which conclusions from experimental scientific studies can be generalized from the specific set of conditions under which the study is conducted to other populations, settings, treatments, measurements, times, and experimenters.
Introduction
The ultimate goal of experimental scientific studies is to advance our understanding of real-life processes and phenomena. In research on individual differences it is rarely feasible to design experiments that involve thousands of participants and conditions that closely resemble the real world. Researchers usually seek to study an assumed cause-effect relationship without the interference of myriads of extraneous variables in real-life settings. To this purpose, they set up an experimental situation which allows to focus on the assumed cause-effect relationship and to control potentially confounding effects of extraneous variables. As a result, an artificial situation that differs from the real...
This is a preview of subscription content, log in via an institution to check access.
Access this chapter
Institutional subscriptions
Bracht, G. H., & Glass, G. V. (1968). The external validity of experiments. American Educational Research Journal, 5 (4), 437–474.
Article Google Scholar
Campbell, D., & Stanley, J. (1963). Experimental and quasi-experimental designs for research . Chicago: Rand-McNally.
Google Scholar
Henrich, J., Heine, S. J., & Norenzayan, A. (2010). Most people are not WEIRD. Nature, 466 (7302), 29–29.
Article PubMed Google Scholar
Lynch, J. G. (1982). On the external validity of experiments in consumer research. Journal of Consumer Research, 9 (3), 225–239.
Download references
Author information
Authors and affiliations.
University of Luxembourg, Esch-sur-Alzette, Belval, Luxembourg
Christoph J. Kemper
You can also search for this author in PubMed Google Scholar
Corresponding author
Correspondence to Christoph J. Kemper .
Editor information
Editors and affiliations.
Oakland University, Rochester, USA
Virgil Zeigler-Hill
Todd K. Shackelford
Section Editor information
Humboldt University, Germany, Berlin, Germany
Matthias Ziegler
Rights and permissions
Reprints and permissions
Copyright information
© 2017 Springer International Publishing AG
About this entry
Cite this entry.
Kemper, C.J. (2017). External Validity. In: Zeigler-Hill, V., Shackelford, T. (eds) Encyclopedia of Personality and Individual Differences. Springer, Cham. https://doi.org/10.1007/978-3-319-28099-8_1303-1
Download citation
DOI : https://doi.org/10.1007/978-3-319-28099-8_1303-1
Received : 03 February 2017
Accepted : 03 February 2017
Published : 10 February 2017
Publisher Name : Springer, Cham
Print ISBN : 978-3-319-28099-8
Online ISBN : 978-3-319-28099-8
eBook Packages : Springer Reference Behavioral Science and Psychology Reference Module Humanities and Social Sciences Reference Module Business, Economics and Social Sciences
- Publish with us
Policies and ethics
- Find a journal
- Track your research
Have a language expert improve your writing
Run a free plagiarism check in 10 minutes, automatically generate references for free.
- Knowledge Base
- Methodology
- Internal vs External Validity | Understanding Differences & Examples
Internal vs External Validity | Differences & Examples
Published on 5 May 2022 by Raimo Streefkerk . Revised on 10 October 2022.
When testing cause-and-effect relationships, validity can be split up into two types: internal validity and external validity .
Table of contents
Trade-off between internal and external validity, threats to internal validity, threats to external validity, frequently asked questions about internal and external validity.
Better internal validity often comes at the expense of external validity (and vice versa). The type of study you choose reflects the priorities of your research.
A solution to this trade-off is to conduct the research first in a controlled (artificial) environment to establish the existence of a causal relationship, followed by a field experiment to analyse whether the results hold in the real world.
Prevent plagiarism, run a free check.
There are eight factors that can threaten the internal validity of your research. They are explained below using the following example:
They set up an experiment with two groups: 1) a control group of employees with fixed working hours, and 2) an experiment group with employees with flexible working hours.
Threat | Explanation | Example |
---|---|---|
History | Unanticipated events change the conditions of the study and influence the outcome. | A new (better) manager starts during the study, which improves job satisfaction. |
Maturation | The passage of time influences the dependent variable (job satisfaction). | During the six-month experiment, employees become more experienced and better at their jobs. Therefore, job satisfaction may improve. |
Testing | The pre-test (used to establish a baseline) affects the results of the post-test. | Employees feel the need to be consistent in their answers in the pre-test and post-test. |
Participant selection | Participants in the control and experimental group differ substantially and can thus not be compared. | Instead of a randomly assigning employees to one of two groups, employees can volunteer to participate in an experiment to improve job satisfaction. The experimental group now consists of more engaged (more satisfied) employees to begin with. |
Over the course of a (longer) study, participants may drop out. If the dropout is caused by the experimental treatment (as opposed to coincidence), it can threaten the internal validity. | Really dissatisfied employees quit their job during the study. The average job satisfaction will now improve, not because the ‘treatment’ worked, but because the dissatisfied employees are not included in the post-test. | |
Regression towards mean | Extreme scores on a second measurement. | Employees who score extremely low in the first job satisfaction survey probably show greater gain in job satisfaction than employees who scored average. |
Instrumentation | There is a change in how the dependent variable is measured during the study. | The in the post-test contains extra questions compared to the one used for the pre-test. |
Social interaction | Interaction between participants from different groups influences the outcome. | The group of employees with fixed working hours are resentful of the group with flexible working hours, and their job satisfaction decreases as a result. |
There are three main factors that might threaten the external validity of our study example.
Threat | Explanation | Example |
---|---|---|
Testing | Participation in the pre-test influences the reaction to the ‘treatment’. | The questionnaire about job satisfaction used in the pre-test triggers employees to start thinking more consciously about their job satisfaction. |
Participants of the study differ substantially from the population. | Employees participating in the experiment are significantly younger than employees in other departments, so the results can’t be generalised. | |
Participants change their behaviour because they know they are being studied. | The employees make an extra effort in their jobs and feel greater job satisfaction because they know they are participating in an experiment. |
There are various other threats to external validity that can apply to different kinds of experiments.
I nternal validity is the degree of confidence that the causal relationship you are testing is not influenced by other factors or variables .
External validity is the extent to which your results can be generalised to other contexts.
The validity of your experiment depends on your experimental design .
There are eight threats to internal validity : history, maturation, instrumentation, testing, selection bias , regression to the mean, social interaction, and attrition .
The two types of external validity are population validity (whether you can generalise to other groups of people) and ecological validity (whether you can generalise to other situations and settings).
There are seven threats to external validity : selection bias , history, experimenter effect, Hawthorne effect , testing effect, aptitude-treatment, and situation effect.
Experimental designs are a set of procedures that you plan in order to examine the relationship between variables that interest you.
To design a successful experiment, first identify:
- A testable hypothesis
- One or more independent variables that you will manipulate
- One or more dependent variables that you will measure
When designing the experiment, first decide:
- How your variable(s) will be manipulated
- How you will control for any potential confounding or lurking variables
- How many subjects you will include
- How you will assign treatments to your subjects
Cite this Scribbr article
If you want to cite this source, you can copy and paste the citation or click the ‘Cite this Scribbr article’ button to automatically add the citation to our free Reference Generator.
Streefkerk, R. (2022, October 10). Internal vs External Validity | Differences & Examples. Scribbr. Retrieved 3 September 2024, from https://www.scribbr.co.uk/research-methods/internal-vs-external-validity/
Is this article helpful?
Raimo Streefkerk
Have a language expert improve your writing
Run a free plagiarism check in 10 minutes, generate accurate citations for free.
- Knowledge Base
Methodology
- The 4 Types of Validity in Research | Definitions & Examples
The 4 Types of Validity in Research | Definitions & Examples
Published on September 6, 2019 by Fiona Middleton . Revised on June 22, 2023.
Validity tells you how accurately a method measures something. If a method measures what it claims to measure, and the results closely correspond to real-world values, then it can be considered valid. There are four main types of validity:
- Construct validity : Does the test measure the concept that it’s intended to measure?
- Content validity : Is the test fully representative of what it aims to measure?
- Face validity : Does the content of the test appear to be suitable to its aims?
- Criterion validity : Do the results accurately measure the concrete outcome they are designed to measure?
In quantitative research , you have to consider the reliability and validity of your methods and measurements.
Note that this article deals with types of test validity, which determine the accuracy of the actual components of a measure. If you are doing experimental research, you also need to consider internal and external validity , which deal with the experimental design and the generalizability of results.
Table of contents
Construct validity, content validity, face validity, criterion validity, other interesting articles, frequently asked questions about types of validity.
Construct validity evaluates whether a measurement tool really represents the thing we are interested in measuring. It’s central to establishing the overall validity of a method.
What is a construct?
A construct refers to a concept or characteristic that can’t be directly observed, but can be measured by observing other indicators that are associated with it.
Constructs can be characteristics of individuals, such as intelligence, obesity, job satisfaction, or depression; they can also be broader concepts applied to organizations or social groups, such as gender equality, corporate social responsibility, or freedom of speech.
There is no objective, observable entity called “depression” that we can measure directly. But based on existing psychological research and theory, we can measure depression based on a collection of symptoms and indicators, such as low self-confidence and low energy levels.
What is construct validity?
Construct validity is about ensuring that the method of measurement matches the construct you want to measure. If you develop a questionnaire to diagnose depression, you need to know: does the questionnaire really measure the construct of depression? Or is it actually measuring the respondent’s mood, self-esteem, or some other construct?
To achieve construct validity, you have to ensure that your indicators and measurements are carefully developed based on relevant existing knowledge. The questionnaire must include only relevant questions that measure known indicators of depression.
The other types of validity described below can all be considered as forms of evidence for construct validity.
Here's why students love Scribbr's proofreading services
Discover proofreading & editing
Content validity assesses whether a test is representative of all aspects of the construct.
To produce valid results, the content of a test, survey or measurement method must cover all relevant parts of the subject it aims to measure. If some aspects are missing from the measurement (or if irrelevant aspects are included), the validity is threatened and the research is likely suffering from omitted variable bias .
A mathematics teacher develops an end-of-semester algebra test for her class. The test should cover every form of algebra that was taught in the class. If some types of algebra are left out, then the results may not be an accurate indication of students’ understanding of the subject. Similarly, if she includes questions that are not related to algebra, the results are no longer a valid measure of algebra knowledge.
Face validity considers how suitable the content of a test seems to be on the surface. It’s similar to content validity, but face validity is a more informal and subjective assessment.
You create a survey to measure the regularity of people’s dietary habits. You review the survey items, which ask questions about every meal of the day and snacks eaten in between for every day of the week. On its surface, the survey seems like a good representation of what you want to test, so you consider it to have high face validity.
As face validity is a subjective measure, it’s often considered the weakest form of validity. However, it can be useful in the initial stages of developing a method.
Criterion validity evaluates how well a test can predict a concrete outcome, or how well the results of your test approximate the results of another test.
What is a criterion variable?
A criterion variable is an established and effective measurement that is widely considered valid, sometimes referred to as a “gold standard” measurement. Criterion variables can be very difficult to find.
What is criterion validity?
To evaluate criterion validity, you calculate the correlation between the results of your measurement and the results of the criterion measurement. If there is a high correlation, this gives a good indication that your test is measuring what it intends to measure.
A university professor creates a new test to measure applicants’ English writing ability. To assess how well the test really does measure students’ writing ability, she finds an existing test that is considered a valid measurement of English writing ability, and compares the results when the same group of students take both tests. If the outcomes are very similar, the new test has high criterion validity.
Prevent plagiarism. Run a free check.
If you want to know more about statistics , methodology , or research bias , make sure to check out some of our other articles with explanations and examples.
- Normal distribution
- Degrees of freedom
- Null hypothesis
- Discourse analysis
- Control groups
- Mixed methods research
- Non-probability sampling
- Quantitative research
- Ecological validity
Research bias
- Rosenthal effect
- Implicit bias
- Cognitive bias
- Selection bias
- Negativity bias
- Status quo bias
Face validity and content validity are similar in that they both evaluate how suitable the content of a test is. The difference is that face validity is subjective, and assesses content at surface level.
When a test has strong face validity, anyone would agree that the test’s questions appear to measure what they are intended to measure.
For example, looking at a 4th grade math test consisting of problems in which students have to add and multiply, most people would agree that it has strong face validity (i.e., it looks like a math test).
On the other hand, content validity evaluates how well a test represents all the aspects of a topic. Assessing content validity is more systematic and relies on expert evaluation. of each question, analyzing whether each one covers the aspects that the test was designed to cover.
A 4th grade math test would have high content validity if it covered all the skills taught in that grade. Experts(in this case, math teachers), would have to evaluate the content validity by comparing the test to the learning objectives.
Criterion validity evaluates how well a test measures the outcome it was designed to measure. An outcome can be, for example, the onset of a disease.
Criterion validity consists of two subtypes depending on the time at which the two measures (the criterion and your test) are obtained:
- Concurrent validity is a validation strategy where the the scores of a test and the criterion are obtained at the same time .
- Predictive validity is a validation strategy where the criterion variables are measured after the scores of the test.
Convergent validity and discriminant validity are both subtypes of construct validity . Together, they help you evaluate whether a test measures the concept it was designed to measure.
- Convergent validity indicates whether a test that is designed to measure a particular construct correlates with other tests that assess the same or similar construct.
- Discriminant validity indicates whether two tests that should not be highly related to each other are indeed not related. This type of validity is also called divergent validity .
You need to assess both in order to demonstrate construct validity. Neither one alone is sufficient for establishing construct validity.
The purpose of theory-testing mode is to find evidence in order to disprove, refine, or support a theory. As such, generalizability is not the aim of theory-testing mode.
Due to this, the priority of researchers in theory-testing mode is to eliminate alternative causes for relationships between variables . In other words, they prioritize internal validity over external validity , including ecological validity .
It’s often best to ask a variety of people to review your measurements. You can ask experts, such as other researchers, or laypeople, such as potential participants, to judge the face validity of tests.
While experts have a deep understanding of research methods , the people you’re studying can provide you with valuable insights you may have missed otherwise.
Cite this Scribbr article
If you want to cite this source, you can copy and paste the citation or click the “Cite this Scribbr article” button to automatically add the citation to our free Citation Generator.
Middleton, F. (2023, June 22). The 4 Types of Validity in Research | Definitions & Examples. Scribbr. Retrieved September 3, 2024, from https://www.scribbr.com/methodology/types-of-validity/
Is this article helpful?
Fiona Middleton
Other students also liked, reliability vs. validity in research | difference, types and examples, construct validity | definition, types, & examples, external validity | definition, types, threats & examples, get unlimited documents corrected.
✔ Free APA citation check included ✔ Unlimited document corrections ✔ Specialized in correcting academic texts
- A-Z Publications
Annual Review of Political Science
Volume 24, 2021, review article, open access, external validity.
- Michael G. Findley 1 , Kyosuke Kikuta 2 , and Michael Denly 1
- View Affiliations Hide Affiliations Affiliations: 1 Department of Government, University of Texas, Austin, Texas 78712, USA; email: [email protected] 2 Osaka School of International Public Policy, Osaka University, Osaka 560-0043, Japan
- Vol. 24:365-393 (Volume publication date May 2021) https://doi.org/10.1146/annurev-polisci-041719-102556
- Copyright © 2021 by Annual Reviews. This work is licensed under a Creative Commons Attribution 4.0 International License, which permits unrestricted use, distribution, and reproduction in any medium, provided the original author and source are credited. See credit lines of images or other third-party material in this article for license information
External validity captures the extent to which inferences drawn from a given study's sample apply to a broader population or other target populations. Social scientists frequently invoke external validity as an ideal, but they rarely attempt to make rigorous, credible external validity inferences. In recent years, methodologically oriented scholars have advanced a flurry of work on various components of external validity, and this article reviews and systematizes many of those insights. We first clarify the core conceptual dimensions of external validity and introduce a simple formalization that demonstrates why external validity matters so critically. We then organize disparate arguments about how to address external validity by advancing three evaluative criteria: model utility, scope plausibility, and specification credibility. We conclude with a practical aspiration that scholars supplement existing reporting standards to include routine discussion of external validity. It is our hope that these evaluation and reporting standards help rebalance scientific inquiry, such that the current obsession with causal inference is complemented with an equal interest in generalized knowledge.
Article metrics loading...
Full text loading...
Literature Cited
- Abadie A , Diamond A , Hainmueller J. 2010 . Synthetic control methods for comparative case studies: estimating the effect of California's tobacco control program. J. Am. Stat. Assoc. 105 : 493– 505 [Google Scholar]
- Acemoglu D. 2010 . Theory, general equilibrium, and political economy in development economics. J. Econ. Perspect. 24 : 17– 32 [Google Scholar]
- Allcott H. 2015 . Site selection bias in program evaluation. Q. J. Econ. 130 : 1117– 65 [Google Scholar]
- Andrews I , Oster E. 2019 . A simple approximation for evaluating external validity bias. Econ. Lett. 178 : 58– 62 [Google Scholar]
- Angrist JD , Imbens GW , Rubin DB. 1996 . Identification of causal effects using instrumental variables. J. Am. Stat. Assoc. 91 : 456– 58 [Google Scholar]
- Angrist JD , Pischke JS. 2008 . Mostly Harmless Econometrics: An Empiricist's Companion Princeton, NJ: Princeton Univ. Press [Google Scholar]
- Angrist JD , Pischke JS. 2010 . The credibility revolution in empirical economics: how better research design is taking the con out of econometrics. J. Econ. Perspect. 24 : 3– 30 [Google Scholar]
- Angrist JD , Rokkanen M. 2015 . Wanna get away? Regression discontinuity estimation of exam school effects away from the cutoff. J. Am. Stat. Assoc. 110 : 1331– 44 [Google Scholar]
- Appelbaum M , Cooper H , Kline RB , Mayo-Wilson E , Nezu AM , Rao SM. 2018 . Journal article reporting standards for quantitative research in psychology: the APA Publications and Communications Board Task Force report. Am. Psychol. 73 : 3– 25 [Google Scholar]
- Aronow PM , Samii C. 2016 . Does regression produce representative estimates of causal effects?. Am. J. Political Sci. 60 : 250– 67 [Google Scholar]
- Aronow PM , Sävje F. 2020 . The book of why: the new science of cause and effect. J. Am. Stat. Assoc. 1459 : 482– 85 [Google Scholar]
- Aronson E , Carlsmith JM. 1968 . Experimentation in social psychology. Handb. Soc. Psychol. 2 : 1– 79 [Google Scholar]
- Aronson E , Wilson TD , Akert RM. 1994 . Social Psychology: The Heart and the Mind . New York: Harper Collins [Google Scholar]
- Ashworth S , Berry CR , Mesquita EBD. 2014 . All else equal in theory and data (big or small). PS: Political Sci. Politics 48 : 89– 94 [Google Scholar]
- Banerjee A , Banerji R , Berry J , Duflo E , Kannan H et al. 2017a . From proof of concept to scalable policies: challenges and solutions, with an application. J. Econ. Perspect. 31 : 73– 102 [Google Scholar]
- Banerjee A , Chassang S , Snowberg E. 2017b . Decision theoretic approaches to experiment design and external validity. Handbook of Economic Field Experiments , Vol. 2 Duflo E, Banerjee A 141– 74 Oxford, UK: Elsevier [Google Scholar]
- Banerjee A , Duflo E , Goldberg N , Karlan D , Osei R et al. 2015 . A multifaceted program causes lasting progress for the very poor: evidence from six countries. Science 348 : 1260799 [Google Scholar]
- Bareinboim E , Pearl J. 2013 . A general algorithm for deciding transportability of experimental results. J. Causal Inference 1 : 107– 134 [Google Scholar]
- Bareinboim E , Pearl J 2016 . Causal inference and the data-fusion problem. PNAS 113 : 7345– 52 [Google Scholar]
- Bates MA , Glennerster R. 2017 . The generalizability puzzle. Stanford Soc. Innov. Rev. 201 : 50– 54 [Google Scholar]
- Berinsky AJ , Huber GA , Lenz GS. 2012 . Evaluating online labor markets for experimental research: Amazon.com's Mechanical Turk. Political Anal 20 : 351– 68 [Google Scholar]
- Berinsky AJ , Margolis MF , Sances MW. 2014 . Separating the shirkers from the workers? Making sure respondents pay attention on self-administered surveys. Am. J. Political Sci. 58 : 739– 53 [Google Scholar]
- Bertanha M , Imbens GW. 2020 . External validity in fuzzy regression discontinuity designs. J. Bus. Econ. Stat. 38 : 593– 612 [Google Scholar]
- Biglaiser G , Staats JL. 2010 . Do political institutions affect foreign direct investment? A survey of U.S. corporations in Latin America. Political Res. Q. 63 : 508– 22 [Google Scholar]
- Bisbee J , Dehejia R , Pop-Eleches C , Samii C. 2017 . Local instruments, global extrapolation: external validity of the labor supply–fertility local average treatment effect. J. Labor Econ. 35 : S99– 147 [Google Scholar]
- Bold T , Kimenyi M , Mwabu G , Ng A , Sandefur J. 2018 . Experimental evidence on scaling up education reforms in Kenya. J. Public Econ. 168 : 1– 20 [Google Scholar]
- Breskin A , Westreich D , Cole SR , Edwards JK. 2019 . Using bounds to compare the strength of exchangeability assumptions for internal and external validity. Am. J. Epidemiol. 188 : 1355– 60 [Google Scholar]
- Brunswik E. 1947 . Systematic and representative design of psychological experiments. Proceedings of the Berkeley Symposium on Mathematical Statistics and Probability 143– 202 Berkeley: Univ. Calif. Press [Google Scholar]
- Buchanan AL , Hudgens MG , Cole SR , Mollan KR , Sax PE et al. 2018 . Generalizing evidence from randomized trials using inverse probability of sampling weights. J. R. Stat. Soc. Ser. A: Stat. Soc. 181 : 1193– 209 [Google Scholar]
- Camerer C 2015 . The promise and success of lab–field generalizability in experimental economics: a critical reply to Levitt and List. Handbook of Experimental Economic Methodology GR Fréchette, A Schotter 249– 95 Oxford, UK: Oxford Univ. Press [Google Scholar]
- Campbell DT. 1957 . Factors relevant to the validity of experiments in social settings. Psychol. Bull. 54 : 297– 312 [Google Scholar]
- Campbell DT 1986 . Relabeling internal and external validity for applied social scientists. Advances in Quasi-Experimental Design and Analysis WMK Trochim pp. 67 – 77 San Francisco: Jossey-Bass [Google Scholar]
- Carroll L. 1865 . Alice's Adventures in Wonderland London: Macmillan [Google Scholar]
- Cartwright N. 1999 . The Dappled World: A Study of the Boundaries of Science Cambridge, UK: Cambridge Univ. Press [Google Scholar]
- Cartwright N. 2020 . Middle-range theory: Without it what could anyone do?. Theoria 35 : 269 – 323 [Google Scholar]
- Cartwright N , Hardie J. 2012 . Evidence-Based Policy: A Practical Guide to Doing It Better Oxford, UK: Oxford Univ. Press [Google Scholar]
- Clarke KA , Primo DM. 2012 . A Model Discipline: Political Science and the Logic of Representations Oxford, UK: Oxford Univ. Press [Google Scholar]
- Cole SR , Stuart EA. 2010 . Generalizing evidence from randomized clinical trials to target populations: the ACTG 320 Trial. Am. J. Epidemiol. 172 : 107– 15 [Google Scholar]
- Cook TD , Campbell DT 1979 . Quasi-Experimentation: Design and Analysis for Field Settings , Vol. 3 Chicago: Rand McNally [Google Scholar]
- Coppock A. 2018 . Generalizing from survey experiments conducted on Mechanical Turk: a replication approach. Political Sci. Res. Methods 7 : 613– 28 [Google Scholar]
- Coppock A , Green DP. 2015 . Assessing the correspondence between experimental results obtained in the lab and field: a review of recent social science research. Political Sci. Res. Methods 3 : 113– 31 [Google Scholar]
- Coppock A , Hill SJ , Vavreck L. 2020 . The small effects of political advertising are small regardless of context, message, sender, or receiver: evidence from 59 real-time randomized experiments. Sci. Adv . 6 : eabc4046 [Google Scholar]
- Coppock A , Leeper TJ , Mullinix KJ 2018 . The generalizability of heterogeneous treatment effect estimates across samples. PNAS 115 : 12441– 46 [Google Scholar]
- Cronbach LJ , Shapiro K. 1982 . Designing Evaluations of Educational and Social Programs San Francisco: Jossey-Bass [Google Scholar]
- Dahabreh IJ , Hayward R , Kent DM. 2016 . Using group data to treat individuals: understanding heterogeneous treatment effects in the age of precision medicine and patient-centred evidence. Int. J. Epidemiol. 45 : 2184– 93 [Google Scholar]
- Deaton A. 2010 . Instruments, randomization, and learning about development. J. Econ. Lit. 48 : 424– 55 [Google Scholar]
- Deaton A. 2019 . Randomization in the tropics revisited: a theme and eleven variations . NBER Work. Pap. 27600 [Google Scholar]
- Deaton A , Cartwright N. 2018 . Understanding and misunderstanding randomized controlled trials. Soc. Sci. Med. 210 : 2– 21 [Google Scholar]
- Dehejia R , Pop-Eleches C , Samii C. 2021 . From local to global: external validity in a fertility natural experiment. J. Bus. Econ. Stat. 39 : 217– 43 [Google Scholar]
- Druckman JN , Green DP , Kuklinski JH , Lupia A. 2006 . The growth and development of experimental research in political science. Am. Political Sci. Rev. 100 : 627– 35 [Google Scholar]
- Druckman JN , Kam CD. 2011 . Students as experimental participants. Cambridge Handbook of Experimental Political Science JN Druckman, DP Greene, JH Kuklinski, A Lupia 41– 57 New York: Cambridge Univ. Press [Google Scholar]
- Dunning T. 2012 . Natural Experiments in the Social Sciences: A Design - Based Approach Cambridge, UK: Cambridge Univ. Press [Google Scholar]
- Dunning T , Grossman G , Humphreys M , Hyde S , McIntosh C , Nellis G 2019 . . Information, Accountability, and Cumulative Learning: Lessons from Metaketa I . Cambridge, UK: Cambridge Univ. Press [Google Scholar]
- Elman C , Gerring J , Mahoney J. 2019 . The Production of Knowledge: Enhancing Progress in Social Science Cambridge, UK: Cambridge Univ. Press [Google Scholar]
- Falk A , Heckman JJ. 2009 . Lab experiments are a major source of knowledge in the social sciences. Science 326 : 535– 38 [Google Scholar]
- Falleti TG , Lynch JF. 2009 . Context and causal analysis. Comp. Political Stud. 42 : 1143– 66 [Google Scholar]
- Fey M , Ramsay KW 2011 . Uncertainty and incentives in crisis bargaining: Game-free analysis of international conflict. Am. J. Political Sci. 55 : 149– 69 [Google Scholar]
- Findley MG , Denly M , Kikuta K. 2022 . External Validity in the Social Sciences: An Integrated Approach Cambridge, UK: Cambridge Univ. Press Manuscript under contract [Google Scholar]
- Findley MG , Harris AS , Milner HV , Nielson DL. 2017a . Who controls foreign aid? Elite versus public perceptions of donor influence in aid-dependent Uganda. Int. Organ. 71 : 633– 63 [Google Scholar]
- Findley MG , Laney B , Nielson DL , Sharman JC. 2017b . External validity in parallel global field and survey experiments on anonymous incorporation. J. Politics 79 : 856– 72 [Google Scholar]
- Franco A , Malhotra N , Simonovits G , Zigerell LJ. 2017 . Developing standards for post-hoc weighting in population-based survey experiments. J. Exp. Political Sci. 4 : 161– 72 [Google Scholar]
- Gaines BJ , Kuklinski JH. 2011 . Experimental estimation of heterogeneous treatment effects related to self-selection. Am. J. Political Sci. 55 : 724– 36 [Google Scholar]
- Garcia FM , Wantchekon L. 2010 . Theory, external validity, and experimental inference: some conjectures. Ann. Am. Acad. Political Soc. Sci. 628 : 132– 47 [Google Scholar]
- Gelman A , Hill J. 2007 . Data Analysis Using Regression and Multilevel/Hierarchical Modelling Cambridge, UK: Cambridge Univ. Press [Google Scholar]
- Gerber A , Arceneaux K , Boudreau C , Dowling C , Hillygus S et al. 2014 . Reporting guidelines for experimental research: a report from the experimental research section standards committee. J. Exp. Political Sci. 1 : 81– 98 [Google Scholar]
- Gerber AS , Green DP. 2012 . Field Experiments: Design, Analysis, and Interpretation New York: WW Norton [Google Scholar]
- Gerring J. 2008 . The mechanismic worldview: thinking inside the box. Br. J. Political Sci. 38 : 161– 79 [Google Scholar]
- Gerring J. 2011 . Social Science Methodology: A Unified Framework Cambridge, UK: Cambridge Univ. Press [Google Scholar]
- Gisselquist RM. 2020 . How the cases you choose affect the answers you get, revisited. World Dev 127 : 104800 [Google Scholar]
- Goertz G. 2017 . Multimethod Research, Causal Mechanisms, and Case Studies: An Integrated Approach Princeton, NJ: Princeton Univ. Press [Google Scholar]
- Goertz G , Mahoney J. 2012 . A Tale of Two Cultures: Qualitative and Quantitative Research in the Social Sciences Princeton, NJ: Princeton Univ. Press [Google Scholar]
- Grossman G , Humphreys M , Sacramone-Lutz G. 2020 . Information technology and political engagement: mixed evidence from Uganda. J. Politics 82 : 1321– 36 [Google Scholar]
- Grzymala-Busse A. 2011 . Time will tell? Temporality and the analysis of causal mechanisms and processes. Comp. Political Stud. 44 : 1267– 97 [Google Scholar]
- Guala F. 2005 . The Methodology of Experimental Economics Cambridge, UK: Cambridge Univ. Press [Google Scholar]
- Guala F. 2010 . Extrapolation, analogy, and comparative process tracing. Philos. Sci. 77 : 1070– 82 [Google Scholar]
- Guardado J , Wantchékon L. 2018 . Do electoral handouts affect voting behavior?. Electoral Stud 53 : 139– 49 [Google Scholar]
- Hartman E , Grieve R , Ramsahai R , Sekhon J. 2015 . From sample average treatment effect to population average treatment effect on the treated: combining experimental with observational studies to estimate population treatment effects. J. R. Stat. Soc. Ser. A: Stat. Soc. 178 : 757– 78 [Google Scholar]
- Heckman JJ , Urzúa S. 2010 . Comparing IV with structural models: what simple IV can and cannot identify. J. Econ. 156 : 27– 37 [Google Scholar]
- Heckman JJ , Vytlacil E. 2001 . Policy-relevant treatment effects. Am. Econ. Rev. Pap . Proc . 91 : 107– 11 [Google Scholar]
- Heckman JJ , Vytlacil EJ. 2005 . Structural equations, treatment effects, and econometric policy evaluation. Econometrica 73 : 669– 738 [Google Scholar]
- Henrich J , Heine SJ , Norenzayan A. 2010 . The weirdest people in the world?. Behav. Brain Sci. 33 : 61– 83 [Google Scholar]
- Ho DE , Imai K , King G , Stuart EA. 2007 . Matching as nonparametric preprocessing for reducing model dependence in parametric causal inference. Political Anal 15 : 199– 236 [Google Scholar]
- Holland PW. 1986 . Statistics and causal inference. J. Am. Stat. Assoc. 81 : 945– 60 [Google Scholar]
- Hollenbach FM , Montgomery JM 2020 . Bayesian model selection, model comparison, and model averaging. SAGE Handbook of Research Methods in Political Science and International Relations L Curini, RJ Franzese 937– 60 London: SAGE [Google Scholar]
- Hotz VJ , Imbens GW , Mortimer JH. 2005 . Predicting the efficacy of future training programs using past experiences at other locations. J. Econ. 125 : 241– 70 [Google Scholar]
- Huff C , Tingley D. 2015 .. “ Who are these people?” Evaluating the demographic characteristics and political preferences of MTurk survey respondents. Res. Politics 2 : 1– 12 [Google Scholar]
- Imai K , Keele LJ , Tingley D , Yamamoto T. 2011 . Unpacking the black box of causality: learning about causal mechanisms from experimental and observational studies. Am. Political Sci. Rev. 105 : 765– 89 [Google Scholar]
- Imai K , King G , Stuart EA. 2008 . Misunderstandings between experimentalists and observationalists about causal inference. J. R. Stat. Soc. Ser. A: Stat. Soc. 171 : 481– 502 [Google Scholar]
- Imai K , Ratkovic M. 2013 . Estimating treatment effect heterogeneity in randomized program evaluation. Ann. Appl. Stat. 7 : 443– 70 [Google Scholar]
- Imbens GW. 2010 . Better LATE than nothing: some comments on Deaton (2009) and Heckman and Urzua (2009). J. Econ. Lit. 48 : 399– 423 [Google Scholar]
- Imbens GW , Angrist JD. 1994 . Identification and estimation of local average treatment effects. Econometrica 62 : 467– 75 [Google Scholar]
- Imbens GW , Rubin DB. 2015 . Causal Inference for Statistics, Social, and Biomedical Sciences: An Introduction New York: Cambridge Univ. Press [Google Scholar]
- Keane MP , Wolpin KI. 2007 . Exploring the usefulness of a nonrandom holdout sample for model validation: welfare effects on female behavior. Int. Econ. Rev. 48 : 1351– 78 [Google Scholar]
- Kern HL , Stuart EA , Hill J , Green DP. 2016 . Assessing methods for generalizing experimental impact estimates to target populations. J. Res. Educ. Eff. 9 : 103– 27 [Google Scholar]
- Kessler J , Lise V 2015 . The external validity of experiments: the misleading emphasis on quantitative effects. Handbook of Experimental Economic Methodology GR Fréchette, A Schotter 391– 406 Oxford, UK: Oxford Univ. Press [Google Scholar]
- King G , Keohane RO , Verba S. 1994 . Designing Social Inquiry: Scientific Inference in Qualitative Research Princeton, NJ: Princeton Univ. Press [Google Scholar]
- Klašnja M , Titiunik R. 2017 . The incumbency curse: weak parties, term limits, and unfulfilled accountability. Am. Political Sci. Rev. 111 : 129– 48 [Google Scholar]
- Kruskal W , Mosteller F. 1979 . Representative sampling, III: the current statistical literature. Int. Stat. Rev./Rev. Int. Stat. 47 : 245– 65 [Google Scholar]
- Leamer EE. 2010 . Tantalus on the road to asymptopia. J. Econ. Perspect. 24 : 31– 46 [Google Scholar]
- Lesko CR , Buchanan AL , Westreich D , Edwards JK , Hudgens MG , Cole SR. 2017 . Generalizing study results. Epidemiology 28 : 553– 61 [Google Scholar]
- Levitt HM , Creswell JW , Josselson R , Bamberg M , Frost DM , Suarez-Orozco C. 2018 . Journal article reporting standards for qualitative research in psychology: the APA Publications and Communications Board task force report. Am. Psychol. 73 : 26– 46 [Google Scholar]
- Lieberson S. 1985 . Making It Count: The Improvement of Social Research and Theory Berkeley: Univ. Calif. Press [Google Scholar]
- Little A , Pepinsky TB. 2021 . Learning from biased research designs. J. Politics In press. https://www.journals.uchicago.edu/doi/10.1086/710088 [Google Scholar]
- Low H , Meghir C. 2017 . The use of structural models in econometrics. J. Econ. Perspect. 31 : 33– 58 [Google Scholar]
- Lucas JW. 2003 . Theory-testing, generalization, and the problem of external validity. Sociol. Theory 21 : 236– 53 [Google Scholar]
- Mackie JL. 1965 . Causes and conditions. Am. Philos. Q. 2 : 245– 64 [Google Scholar]
- Marcellesi A. 2015 . External validity: Is there still a problem?. Philos. Sci. 82 : 1308– 17 [Google Scholar]
- McDermott R 2011 . Internal and external validity. Cambridge Handbook of Experimental Political Science , ed. JN Druckman, DP Green DP, JH Kuklinski, A Lupia 27– 40 New York: Cambridge Univ. Press [Google Scholar]
- McFadden D , Talvitie AP 1977 . Demand model estimation and validation. Urban travel demand forecasting project: phase 1 final report series, Vol. V Rep. UCB-ITS-SR-77-9 Inst. Transport. Stud., Univ. Calif Berkeley and Irvine: [Google Scholar]
- McIntyre L. 2019 . The Scientific Attitude: Defending Science from Denial, Fraud, and Pseudoscience Cambridge, MA: MIT Press [Google Scholar]
- Miratrix LW , Sekhon JS , Theodoridis AG , Campos LF. 2018 . Worth weighting? How to think about and use weights in survey experiments. Political Anal 26 : 275– 91 [Google Scholar]
- Morton RB , Williams KC. 2010 . Experimental Political Science and the Study of Causality: From Nature to the Lab Cambridge, UK: Cambridge Univ. Press [Google Scholar]
- Muller SM. 2015 . Causal interaction and external validity: obstacles to the policy relevance of randomized evaluations. World Bank Econ. Rev. 29 : S217– 25 [Google Scholar]
- Mullinix KJ , Leeper TJ , Druckman JN , Freese J. 2015 . The generalizability of survey experiments. J. Exp. Political Sci. 2 : 109– 38 [Google Scholar]
- Muralidharan K , Niehaus P. 2017 . Experimentation at scale. J. Econ. Perspect. 31 : 103– 24 [Google Scholar]
- Mutz DC. 2011 . Population-Based Survey Experiments Princeton, NJ: Princeton Univ. Press [Google Scholar]
- Nagler J , Tucker JA. 2015 . Drawing inferences and testing theories with big data. PS: Political Sci. Politics 48 : 84– 88 [Google Scholar]
- Neumayer E , Plumper T. 2017 . Robustness Tests for Quantitative Research Cambridge, UK: Cambridge Univ. Press [Google Scholar]
- Nguyen TQ , Ebnesajjad C , Cole SR , Stuart EA. 2017 . Sensitivity analysis for an unobserved moderator in RCT-to-target-population generalization of treatment effects. Ann. Appl. Stat. 11 : 225– 47 [Google Scholar]
- Olsen R , Bell S , Orr L , Stuart EA. 2013 . External validity in policy evaluations that choose sites purposively. J. Policy Anal. Manag. 32 : 107– 21 [Google Scholar]
- Pearl J. 2009 . Causality: Models, Reasoning, and Inference Cambridge, UK: Cambridge Univ. Press [Google Scholar]
- Pearl J , Bareinboim E. 2014 . External validity: from do-calculus to transportability across populations. Stat. Sci. 29 : 579– 95 [Google Scholar]
- Pearl J , Bareinboim E. 2019 . Note on “generalizability of study results. .” Epidemiology 30 : 186– 88 [Google Scholar]
- Pearl J , Mackenzie D. 2018 . The Book of Why: The New Science of Cause and Effect New York: Basic Books [Google Scholar]
- Pierson P. 2000 . Increasing returns, path dependence, and the study of politics. Am. Political Sci. Rev. 94 : 251– 67 [Google Scholar]
- Pritchett L , Sandefur J. 2013 . Context matters for size: why external validity claims and development practice don't mix Work. Pap., Cent. Glob. Dev Washington, DC: [Google Scholar]
- Pritchett L , Sandefur J. 2015 . Learning from experiments when context matters. Am. Econ. Rev. 105 : 471– 75 [Google Scholar]
- Ragin CC. 2000 . Fuzzy-Set Social Science Chicago: Univ. Chicago Press [Google Scholar]
- Ravallion M. 2012 . Fighting poverty one experiment at a time: Poor Economics: A Radical Rethinking of the Way to Fight Global Poverty : review essay. J. Econ. Lit. 50 : 103– 14 [Google Scholar]
- Rodrik D 2009 . The new development economics: We shall experiment, but how shall we learn?. What Works in Development? Thinking Big and Thinking Small J Cohen, W Easterly 24– 50 Washington, DC: Brookings Inst. Press [Google Scholar]
- Rubin DB. 2004 . Multiple Imputation for Nonresponse in Surveys New York: John Wiley & Sons [Google Scholar]
- Russell B. 1912 . On the notion of cause. Proc. Aristot . Soc . 13 : 1– 26 [Google Scholar]
- Samii C. 2016 . Causal empiricism in quantitative research. J. Politics 78 : 941– 55 [Google Scholar]
- Sartori G. 1970 . Concept misformation in comparative politics. Am. Political Sci. Rev. 64 : 1033– 53 [Google Scholar]
- Schulz K. 2015 . The rabbit-hole rabbit hole. New Yorker June 4. https://www.newyorker.com/culture/cultural-comment/the-rabbit-hole-rabbit-hole [Google Scholar]
- Sekhon JS , Titiunik R 2017 . On interpreting the regression discontinuity design as a local experiment. Regression Discontinuity Designs: Theory and Applications , Vol. 38 MD Cattaneo, JC Escanciano 1– 28 Bingley, UK: Emerald Publ. [Google Scholar]
- Shadish W , Cook TD , Campbell DT. 2002 . Experimental and Quasi-Experimental Designs for Generalized Causal Inference Boston: Houghton Mifflin [Google Scholar]
- Tipton E. 2013 . Improving generalizations from experiments using propensity score subclassification: assumptions, properties, and contexts. J. Educ. Behav. Stat. 38 : 239– 66 [Google Scholar]
- Tipton E , Hedges L , Vaden-Kiernan M , Borman G , Sullivan K , Caverly S. 2014 . Sample selection in randomized experiments: a new method using propensity score stratified sampling. J. Res. Educ. Eff. 7 : 114– 35 [Google Scholar]
- Trochim WMK , Donnelly JP. 2006 . The Research Methods Knowledge Base Cincinnati, OH: Atomic Dog. , 3rd ed.. [Google Scholar]
- van Eersel GG , Koppenol-Gonzalez GV , Reiss J. 2019 . Extrapolation of experimental results through analogical reasoning from latent classes. Philos. Sci. 86 : 219– 35 [Google Scholar]
- Vivalt E. 2020 . How much can we generalize from impact evaluations?. J. Eur. Econ. Assoc. 18 : 6 3045– 89 [Google Scholar]
- Walker HA , Cohen BP. 1985 . Scope statements: imperatives for evaluating theory. Am. Sociol. Rev. 50 : 288– 301 [Google Scholar]
- Weller N , Barnes J. 2014 . Finding Pathways: Mixed-Method Research for Studying Causal Mechanisms Cambridge, UK: Cambridge Univ. Press [Google Scholar]
- Wells GL , Windschilt PD. 1999 . Stimulus sampling and social psychology. Personal. Soc. Psychol. Bull. 25 : 1115– 25 [Google Scholar]
- Wells HG. 1905 . A Modern Utopia London: Chapman & Hall [Google Scholar]
- Westreich D , Edwards JK , Lesko CR , Cole SR , Stuart EA. 2019 . Target validity and the hierarchy of study designs. Am. J. Epidemiol. 188 : 438– 43 [Google Scholar]
- Westreich D , Edwards JK , Lesko CR , Stuart EA , Cole SR. 2017 . Transportability of trial results using inverse odds of sampling weights. Am. J. Epidemiol. 186 : 1010– 14 [Google Scholar]
- Wilke A , Humphreys M 2020 . Field experiments, theory, and external validity. SAGE Handbook of Research Methods in Political Science and International Relations L Curini, RJ Franzese 1007– 35 London: SAGE [Google Scholar]
- Wilson MC , Knutsen CH. 2020 . Geographical coverage in political science research. Perspect. Politics https://doi.org/10.1017/S1537592720002509 [Crossref] [Google Scholar]
- Wing C , Bello-Gomez RA. 2018 . Regression discontinuity and beyond: options for studying external validity in an internally valid design. Am. J. Eval. 39 : 91– 108 [Google Scholar]
- Wolpin KI. 2007 . Ex ante policy evaluation, structural estimation, and model selection. Am. Econ. Rev. 97 : 48– 52 [Google Scholar]
Data & Media loading...
- Article Type: Review Article
Most Read This Month
Most cited most cited rss feed, framing theory, discursive institutionalism: the explanatory power of ideas and discourse, historical institutionalism in comparative politics, the origins and consequences of affective polarization in the united states, political trust and trustworthiness, public attitudes toward immigration, what have we learned about the causes of corruption from ten years of cross-national empirical research, what do we know about democratization after twenty years, economic determinants of electoral outcomes, public deliberation, discursive participation, and citizen engagement: a review of the empirical literature.
Internal vs. External Validity In Psychology
Julia Simkus
Editor at Simply Psychology
BA (Hons) Psychology, Princeton University
Julia Simkus is a graduate of Princeton University with a Bachelor of Arts in Psychology. She is currently studying for a Master's Degree in Counseling for Mental Health and Wellness in September 2023. Julia's research has been published in peer reviewed journals.
Learn about our Editorial Process
Saul McLeod, PhD
Editor-in-Chief for Simply Psychology
BSc (Hons) Psychology, MRes, PhD, University of Manchester
Saul McLeod, PhD., is a qualified psychology teacher with over 18 years of experience in further and higher education. He has been published in peer-reviewed journals, including the Journal of Clinical Psychology.
Olivia Guy-Evans, MSc
Associate Editor for Simply Psychology
BSc (Hons) Psychology, MSc Psychology of Education
Olivia Guy-Evans is a writer and associate editor for Simply Psychology. She has previously worked in healthcare and educational sectors.
Internal validity centers on demonstrating clear casual relationships within the bounds of a specific study and external validity relates to demonstrating the applicability of findings beyond that original study situation or population.
Researchers have to weigh these considerations in designing methodologically rigorous and generalizable studies.
Whether conclusions about cause and effect relationships within a study are valid | The extent study results apply to contexts beyond the original study | |
Were effects observed really caused by the independent variable or did flaws in the study design/conduct lead to that result? | Can results be expected to apply to other settings, populations, times? | |
Randomization, control conditions, elimination of confounding variables | Having a sample representative of the population of interest, testing variability in contexts | |
Selection bias, attrition, history effects | Interaction effects of setting and treatment, limited participant sample | |
Use control groups, randomization, blinding, account for confounders | Draw from heterogeneous, more representative samples, replicate across ranges of contexts | |
Controlling internal validity often means more artificial research context | Broader generalizability requires flexible, real-world applicable paradigms |

Internal Validity
Internal validity refers to the degree of confidence that the causal relationship being tested exists and is trustworthy.
It tests how likely it is that your treatment caused the differences in results that you observe. Internal validity is largely determined by the study’s experimental design and methods .
Studies that have a high degree of internal validity provide strong evidence of causality, so it makes it possible to eliminate alternative explanations for a finding.
Studies with low internal validity provide weak evidence of causality. The less chance there is for confounding or extraneous variables , the higher the internal validity and the more confident we can be in our findings.
In order to assume cause and effect in a research study, the cause must precede the effect in terms of time, the cause and effect must vary together, and there must be no other explanations for the relationship observed. If these three criteria are observed, you can be confident that a study is internally valid.
An example of a study with high internal validity would be if you wanted to run an experiment to see if using a particular weight-loss pill will help people lose weight.
To test this hypothesis, you would randomly assign a sample of participants to one of two groups: those who will take the weight-loss pill and those who will take a placebo pill.
You can ensure that there is no bias in how participants are assigned to the groups by blinding the research assistants , so they don’t know which participants are in which groups during the experiment. The participants are also blinded, so they do not know whether they are receiving the intervention or not.
If participants drop out of the study, their characteristics are examined to ensure there is no systematic bias regarding who left.
It is important to have a well-thought-out research procedure to mitigate the threats to internal validity.

External Validity
External validity refers to the extent to which the results of a research study can be applied or generalized to another context.
This is important because if external validity is established, the studies’ findings can be generalized to a larger population as opposed to only the relatively few subjects who participated in the study. Unlike internal validity, external validity doesn’t assess causality or rule out confounders.
There are two types of external validity: ecological validity and population validity.
- Ecological validity refers to whether a study’s findings can be generalized to other situations or settings. A high ecological validity means that there is a high degree of similarity between the experimental setting and another setting, and thus we can be confident that the results will generalize to that other setting.
- Population validity refers to how well the experimental sample represents other populations or groups. Using random sampling techniques , such as stratified sampling or cluster sampling, significantly helps increase population validity.
An example of a study with high external validity would be if you hypothesize that practicing mindfulness two times per week will improve the mental health of those diagnosed with depression.
You recruit people who have been diagnosed with depression for at least a year and are between 18–29 years old. Choosing this representative sample with a clearly defined population of interest helps ensure external validity.
You give participants a pre-test and a post-test measuring how often they experienced symptoms of depression in the past week.
During the study, all participants were given individual mindfulness training and asked to practice mindfulness daily for 15 minutes as part of their morning routine.
You can also replicate the study’s results using different methods of mindfulness or different samples of participants.
Trade-off Between Internal and External Validity
There tends to be a negative correlation between internal and external validity in experimental research. This means that experiments that have high internal validity will likely have low external validity and vice versa.
This happens because experimental conditions that produce higher degrees of internal validity (e.g., artificial labs) tend to be highly unlikely to match real-world conditions. So, the external validity is weaker because a lab environment is much different than the real world.
On the other hand, to produce higher degrees of external validity, you want experimental conditions that match a real-world setting (e.g., observational studies ).
However, this comes at the expense of internal validity because these types of studies increase the likelihood of confounding variables and alternative explanations for differences in outcomes.
A solution to this trade-off is replication! You want to conduct the research in multiple environments and settings – first in a controlled, artificial environment to establish the existence of a causal relationship and then in a “real-world” setting to analyze if the results are generalizable.
Threats to Internal Validity
Attrition refers to the loss of study participants over time. Participants might drop out or leave the study which means that the results are based solely on a biased sample of only the people who did not choose to leave.
Differential rates of attrition between treatment and control groups can skew results by affecting the relationship between your independent and dependent variables and thus affect the internal validity of a study.
Confounders
A confounding variable is an unmeasured third variable that influences, or “confounds,” the relationship between an independent and a dependent variable by suggesting the presence of a spurious correlation.
Confounders are threats to internal validity because you can’t tell whether the predicted independent variable causes the outcome or if the confounding variable causes it.
Participant Selection Bias
This is a bias that may result from the selection or assignment of study groups in such a way that proper randomization is not achieved.
If participants are not randomly assigned to groups, the sample obtained might not be representative of the population intended to be studied. For example, some members of a population might be less likely to be included than others due to motivation, willingness to take part in the study, or demographics.
Experimenter Bias
Experimenter bias occurs when an experimenter behaves in a different way with different groups in a study, impacting the results and threatening internal validity. This can be eliminated through blinding.
Social Interaction (Diffusion)
Diffusion refers to when the treatment in research spreads within or between treatment and control groups. This can happen when there is interaction or observation among the groups.
Diffusion poses a threat to internal validity because it can lead to resentful demoralization. This is when the control group is less motivated because they feel resentful over the group that they are in.
Historical Events
Historical events might influence the outcome of studies that occur over longer periods of time. For example, changes in political leadership, natural disasters, or other unanticipated events might change the conditions of the study and influence the outcomes.
Instrumentation
Instrumentation refers to any change in the dependent variable in a study that arises from changes in the measuring instrument used. This happens when different measures are used in the pre-test and post-test phases.
Maturation refers to the impact of time on a study. If the outcomes of the study vary as a natural result of time, it might not be possible to determine whether the effects seen in the study were due to the study treatment or simply due to the impact of time.
Statistical Regression
Regression to the mean refers to the fact that if one sample of a random variable is extreme, the next sampling of the same random variable is likely going to be closer to its mean.
This is a threat to internal validity as participants at extreme ends of treatment can naturally fall in a certain direction due to the passage of time rather than being a direct effect of an intervention.
Repeated Testing
Testing your research participants repeatedly with the same measures will influence your research findings because participants will become more accustomed to the testing. Due to familiarity, or awareness of the study’s purpose, many participants might achieve better results over time.
Threats to External Validity
Sample features.
If some feature(s) of the sample used were responsible for the effect, this could lead to limited generalizability of the findings.
This is a bias that may result from the selection or assignment of study groups in such a way that proper randomization is not achieved. If participants are not randomly assigned to groups, the sample obtained might not be representative of the population intended to be studied.
For example, some members of a population might be less likely to be included than others due to motivation, willingness to take part in the study, or demographics.
Situational Factors
Factors such as the setting, time of day, location, researchers’ characteristics, noise, or the number of measures might affect the generalizability of the findings.
Aptitude-Treatment Interaction → Aptitude-Treatment Interaction to the concept that some treatments are more or less effective for particular individuals depending upon their specific abilities or characteristics.
Hawthorne Effect
The Hawthorne Effect refers to the tendency for participants to change their behaviors simply because they know they are being studied.
Experimenter Effect
Experimenter bias occurs when an experimenter behaves in a different way with different groups in a study, impacting the results and threatening the external validity.
John Henry Effect
The John Henry Effect refers to the tendency for participants in a control group to actively work harder because they know they are in an experiment and want to overcome the “disadvantage” of being in the control group.
Factors that Improve Internal Validity
Blinding refers to a practice where the participants (and sometimes the researchers) are unaware of what intervention they are receiving.
This reduces the influence of extraneous factors and minimizes bias, as any differences in outcome can thus be linked to the intervention and not to the participant’s knowledge of whether they were receiving a new treatment or not.
Random Sampling
Using random sampling to obtain a sample that represents the population that you wish to study will improve internal validity.
Random Assignment
Using random assignment to assign participants to control and treatment groups ensures that there is no systematic bias among the research groups.
Strict Study Protocol
Highly controlled experiments tend to improve internal validity. Experiments that occur in lab settings tend to have higher validity as this reduces variability from sources other than the treatment.
Experimental Manipulation
Manipulating an independent variable in a study as opposed to just observing an association without conducting an intervention improves internal validity.
Factors that Improve External Validity
Replication.
Conducting a study more than once with a different sample or in a different setting to see if the results will replicate can help improve external validity.
If multiple studies have been conducted on the same topic, a meta-analysis can be used to determine if the effect of an independent variable can be replicated, thus making it more reliable.
Replication is the strongest method to counter threats to external validity by enhancing generalizability to other settings, populations, and conditions.
Field Experiments
Conducting a study outside the laboratory, in a natural, real-world setting will improve external validity (however, this will threaten the internal validity)
Probability Sampling
Using probability sampling will counter selection bias by making sure everyone in a population has an equal chance of being selected for a study sample.
Recalibration
Recalibration is the use of statistical methods to maintain accuracy, standardization, and repeatability in measurements to assure reliable results.
Reweighting groups, if a study had uneven groups for a particular characteristic (such as age), is an example of calibration.
Inclusion and Exclusion Criteria
Setting criteria as to who can be involved in the research and who cannot be involved will ensure that the population being studied is clearly defined and that the sample is representative of the population.
Psychological Realism
Psychological realism refers to the process of making sure participants perceive the experimental manipulations as real events so as to not reveal the purpose of the study and so participants don’t behave differently than they would in real life based on knowing the study’s goal.


An official website of the United States government
The .gov means it’s official. Federal government websites often end in .gov or .mil. Before sharing sensitive information, make sure you’re on a federal government site.
The site is secure. The https:// ensures that you are connecting to the official website and that any information you provide is encrypted and transmitted securely.
- Publications
- Account settings
Preview improvements coming to the PMC website in October 2024. Learn More or Try it out now .
- Advanced Search
- Journal List
- J Bras Pneumol
- v.44(3); May-Jun 2018
Internal and external validity: can you apply research study results to your patients?
Cecilia maria patino.
1 . Methods in Epidemiologic, Clinical, and Operations Research-MECOR-program, American Thoracic Society/Asociación Latinoamericana del Tórax, Montevideo, Uruguay.
2 . Department of Preventive Medicine, Keck School of Medicine, University of Southern California, Los Angeles, CA, USA.
Juliana Carvalho Ferreira
3 . Divisão de Pneumologia, Instituto do Coração, Hospital das Clínicas, Faculdade de Medicina, Universidade de São Paulo, São Paulo (SP) Brasil.
CLINICAL SCENARIO
In a multicenter study in France, investigators conducted a randomized controlled trial to test the effect of prone vs. supine positioning ventilation on mortality among patients with early, severe ARDS. They showed that prolonged prone-positioning ventilation decreased 28-day mortality [hazard ratio (HR) = 0.39; 95% CI: 0.25-0.63]. 1
STUDY VALIDITY
The validity of a research study refers to how well the results among the study participants represent true findings among similar individuals outside the study. This concept of validity applies to all types of clinical studies, including those about prevalence, associations, interventions, and diagnosis. The validity of a research study includes two domains: internal and external validity.
Internal validity is defined as the extent to which the observed results represent the truth in the population we are studying and, thus, are not due to methodological errors. In our example, if the authors can support that the study has internal validity, they can conclude that prone positioning reduces mortality among patients with severe ARDS. The internal validity of a study can be threatened by many factors, including errors in measurement or in the selection of participants in the study, and researchers should think about and avoid these errors.
Once the internal validity of the study is established, the researcher can proceed to make a judgment regarding its external validity by asking whether the study results apply to similar patients in a different setting or not ( Figure 1 ). In the example, we would want to evaluate if the results of the clinical trial apply to ARDS patients in other ICUs. If the patients have early, severe ARDS, probably yes, but the study results may not apply to patients with mild ARDS . External validity refers to the extent to which the results of a study are generalizable to patients in our daily practice, especially for the population that the sample is thought to represent.

Lack of internal validity implies that the results of the study deviate from the truth, and, therefore, we cannot draw any conclusions; hence, if the results of a trial are not internally valid, external validity is irrelevant. 2 Lack of external validity implies that the results of the trial may not apply to patients who differ from the study population and, consequently, could lead to low adoption of the treatment tested in the trial by other clinicians.
INCREASING VALIDITY OF RESEARCH STUDIES
To increase internal validity, investigators should ensure careful study planning and adequate quality control and implementation strategies-including adequate recruitment strategies, data collection, data analysis, and sample size. External validity can be increased by using broad inclusion criteria that result in a study population that more closely resembles real-life patients, and, in the case of clinical trials, by choosing interventions that are feasible to apply. 2
Our websites may use cookies to personalize and enhance your experience. By continuing without changing your cookie settings, you agree to this collection. For more information, please see our University Websites Privacy Notice .
Neag School of Education
Educational Research Basics by Del Siegle
External validity.
Note to EPSY 5601 Students: An understanding of the difference between population and ecological validity is sufficient. Mastery of the sub categories for each is not necessary for this course.
External Validity (Generalizability) –to whom can the results of the study be applied–
There are two types of study validity: internal (more applicable with experimental research) and external. This section covers external validity.
External validity involves the extent to which the results of a study can be generalized (applied) beyond the sample. In other words, can you apply what you found in your study to other people (population validity) or settings (ecological validity). A study of fifth graders in a rural school that found one method of teaching spelling was superior to another may not be applicable with third graders (population) in an urban school (ecological).
Threats to External Validity
Population Validity the extent to which the results of a study can be generalized from the specific sample that was studied to a larger group of subjects
- the extent to which one can generalize from the study sample to a defined population– If the sample is drawn from an accessible population, rather than the target population, generalizing the research results from the accessible population to the target population is risky. 2. the extent to which personological variables interact with treatment effects– If the study is an experiment, it may be possible that different results might be found with students at different grades (a personological variable).
Ecological Validity the extent to which the results of an experiment can be generalized from the set of environmental conditions created by the researcher to other environmental conditions (settings and conditions).
- Explicit description of the experimental treatment (not sufficiently described for others to replicate) If the researcher fails to adequately describe how he or she conducted a study, it is difficult to determine whether the results are applicable to other settings.
- Multiple-treatment interference (catalyst effect) If a researcher were to apply several treatments, it is difficult to determine how well each of the treatments would work individually. It might be that only the combination of the treatments is effective.
- Hawthorne effect (attention causes differences) Subjects perform differently because they know they are being studied. “…External validity of the experiment is jeopardized because the findings might not generalize to a situation in which researchers or others who were involved in the research are not present” (Gall, Borg, & Gall, 1996, p. 475)
- Novelty and disruption effect (anything different makes a difference) A treatment may work because it is novel and the subjects respond to the uniqueness, rather than the actual treatment. The opposite may also occur, the treatment may not work because it is unique, but given time for the subjects to adjust to it, it might have worked.
- Experimenter effect (it only works with this experimenter) The treatment might have worked because of the person implementing it. Given a different person, the treatment might not work at all.
- Pretest sensitization (pretest sets the stage) A treatment might only work if a pretest is given. Because they have taken a pretest, the subjects may be more sensitive to the treatment. Had they not taken a pretest, the treatment would not have worked.
- Posttest sensitization (posttest helps treatment “fall into place”) The posttest can become a learning experience. “For example, the posttest might cause certain ideas presented during the treatment to ‘fall into place’ ” (p. 477). If the subjects had not taken a posttest, the treatment would not have worked.
- Interaction of history and treatment effec t (…to everything there is a time…) Not only should researchers be cautious about generalizing to other population, caution should be taken to generalize to a different time period. As time passes, the conditions under which treatments work change.
- Measurement of the dependent variable (maybe only works with M/C tests) A treatment may only be evident with certain types of measurements. A teaching method may produce superior results when its effectiveness is tested with an essay test, but show no differences when the effectiveness is measured with a multiple choice test.
- Interaction of time of measurement and treatment effect (it takes a while for the treatment to kick in) It may be that the treatment effect does not occur until several weeks after the end of the treatment. In this situation, a posttest at the end of the treatment would show no impact, but a posttest a month later might show an impact.
Bracht, G. H., & Glass, G. V. (1968). The external validity of experiments. American Education Research Journal, 5, 437-474. Gall, M. D., Borg, W. R., & Gall, J. P. (1996). Educational research: An introduction. White Plains, NY: Longman.
Del Siegle, Ph.D. Neag School of Education – University of Connecticut [email protected] www.delsiegle.com
Log in using your username and password
- Search More Search for this keyword Advanced search
- Latest content
- Current issue
- BMJ Journals
You are here
- Volume 23, Issue 1
- External validity, generalisability, applicability and directness: a brief primer
- Article Text
- Article info
- Citation Tools
- Rapid Responses
- Article metrics

https://doi.org/10.1136/ebmed-2017-110800
Statistics from Altmetric.com
Request permissions.
If you wish to reuse any or all of this article please use the link below which will take you to the Copyright Clearance Center’s RightsLink service. You will be able to get a quick price and instant permission to reuse the content in many different ways.

You do not have access to the full text of this article, the first page of the PDF of this article appears above.
Contributors MHM and VMM conceived the idea and drafted the manuscript. All authors critically revised the manuscript and approved it.
Competing interests None declared.
Provenance and peer review Not commissioned; externally peer reviewed.
Read the full text or download the PDF:
- Open access
- Published: 07 September 2024
Using online methods to recruit participants into mental health clinical trials: considerations and recommendations from the RE-MIND study
- Mais Iflaifel 1 ,
- Charlotte L. Hall 2 , 3 ,
- Heidi R. Green 4 , 5 ,
- Andrew Willis 6 ,
- Stefan Rennick-Egglestone 3 , 7 ,
- Edmund Juszczak 1 ,
- Mark Townsend 8 ,
- Jennifer Martin 2 , 3 &
- Kirsty Sprange ORCID: orcid.org/0000-0001-6443-7242 1
Trials volume 25 , Article number: 596 ( 2024 ) Cite this article
Metrics details
Ensuring diversity in clinical trials can be a challenge, which may be exacerbated when recruiting vulnerable populations, such as participants with mental health illness. As recruitment continues to be the major cause of trial delays, researchers are turning to online recruitment strategies, e.g. social media, to reach a wider population and reduce recruitment time and costs. There is mixed evidence for the use of online recruitment strategies; therefore, the REcruitment in Mental health trials: broadening the ‘net’, opportunities for INclusivity through online methoDs (RE-MIND) study aimed to identify evidence and provide guidance for use of online strategies in recruitment to mental health trials, with a focus on whether online strategies can enhance inclusivity. This commentary, as part of the RE-MIND study, focusses on providing recommendations for recruitment strategy selection in future research with the aim to improve trial efficiency.
A mixed-methods approach was employed involving three work packages: (I) an evidence review of a cohort of 97 recently published randomised controlled trials/feasibility or pilot studies in mental health to assess the impact of online versus offline recruitment; (II) a qualitative study investigating the experiences of n = 23 key stakeholders on use of an online recruitment approach in mental health clinical trials; (III) combining the results of WP1 and WP2 to produce recommendations on the use of an online recruitment strategy in mental health clinical trials. The findings from WP1 and 2 have been published elsewhere; this commentary represents the results of the third work package.
For external validity, clinical trial participants should reflect the populations that will ultimately receive the interventions being tested, if proven effective. To guide researchers on their options for inclusive recruitment strategies, we have developed a list of considerations and practical recommendations on how to maximise the use of online recruitment methods.
Peer Review reports
Introduction
Recruitment to clinical trials is challenging, and those in mental health research are no exception and people with mental health illness have been identified as an under-served group in health research [ 1 ]. The importance of broader representation of under-served populations in clinical trials is already well established to ensure they reflect the populations that stand to benefit from the intervention being tested [ 2 ]. The question is how do we improve recruitment when we already know that mental health service use is proportionately lower for the socioeconomically disadvantaged [ 3 ], males [ 4 ], people from ethnic minority backgrounds [ 5 ], and older participants or those living in more rural areas [ 6 ]. Traditionally, recruitment into mental health trials has been dependent upon face-to-face referrals and therefore limited to those individuals actively seeking service intervention, thus perpetuating the problem [ 7 ]. Furthermore, increasing pressures on mental health services has become an obstacle to the delivery of trials using this approach; however, technological advances are allowing researchers to be more creative and dynamic in their choice of recruitment strategies to target potential participants typically outside of services and reach wider groups of people [ 8 ]. Despite this potential, deciding upon what might be the best recruitment strategy for those living with mental health illness needs further careful consideration.
To help address, this we conducted a study, “REcruitment in Mental health trials: broadening the ‘net’, opportunities for Inclusivity through online methoDs’ (RE-MIND)” https://www.nctu.ac.uk/our-research/methodology.aspx . The objective was to explore the use of offline and online recruitment strategies with the aim of helping researchers improve recruitment reach and increase the efficiency of clinical trials of mental health interventions.
This project focussed on the recruitment strategy used to make the initial approach to potential participants, informing them about an active clinical trial. As our focus was on the initial stage in recruitment, we did not cover issues surrounding the consent process itself. Despite this, we acknowledge the importance of the methods of taking informed consent, and this should be considered when deciding on a recruitment strategy.
The RE-MIND study consisted of two work packages which have been published separately [ 9 , 10 ]. First is an evidence review of 97 recently published randomised controlled trials (RCTs) and randomised feasibility/pilot studies in mental health to assess the impact of online recruitment versus offline recruitment in clinical trials [ 9 ]. Second is a qualitative study investigating the experiences, opinions, and ideas of n = 23 key stakeholders (research staff and patients and public involvement members with experience working in mental health research) on the use of online recruitment as an approach in mental health clinical trials [ 10 ]. The findings were then triangulated [ 11 ] by researchers MI, KS, and CLH to develop draft considerations and practical recommendations which then underwent a review process by the study Advisory Group (HRG, AW, SRE, EJ, MT, and JM) who have experience in digital research, design, and delivery of online and offline RCTs and equality, diversity, and inclusion resulting in the final recommendations.
Throughout the RE-MIND study, we used the following definitions to broadly categorise offline or online recruitment. These definitions describe an overarching strategy to recruitment:
Online recruitment strategies —the use of Internet technologies such as social media advertisements, Google search engine advertisements, and other website campaigns [ 12 ].
Offline recruitment strategies —in-clinic recruitment, approaching potential participants through mail and telephone using health records and registers, media campaigns, newspaper advertisements, and input during radio and television interviews [ 12 ].
In this commentary, we present a list of considerations and practical recommendations for research teams on the use of online recruitment of participants into mental health clinical trials with the aim to improve recruitment efficiency in clinical trials of mental health interventions. It is worth noting that although the RE-MIND study focussed on mental health interventions the findings may also be beneficial in wider clinical research.
- Recommendations
Complexity of mental illness
Severity of mental health illness has previously been identified as a barrier to participation in mental health research [ 13 , 14 ]. RE-MIND reported that the type of mental health illness, its stage, participants’ feelings about their illness, and carers’ responsibilities were key factors when selecting a recruitment strategy [ 10 ]. Alongside meaningful and authentic patient and public involvement (PPI) to guide and inform the recruitment strategy, using a multi-method approach to recruitment could improve accessibility and inclusivity, by supporting the diverse and changing needs of those living with mental health illness.
Considerations
Consider any relationships between recruitment strategy and mental health symptomatology:
For example, individuals with learning disabilities, autism, anxiety, or obsessive compulsive disorder may have difficulty interfacing in public and therefore may benefit from online recruitment.
Online recruitment may, however, be a barrier for other mental health illnesses such as low mood disorders, depression, personality disorders, and psychosis where an in-person approach offers more security, contact, and support to the individual.
Consider using the stage or severity of illness to inform recruitment method:
Will the person’s diagnostic and treatment experience impact on selection of recruitment method, for example, both patients and carers may be reluctant to talk about or need more time to process a diagnosis in the early stages?
Are personal cues, such as body language, important for communicating with your participants and supporting greater engagement in a trial, for example, recognising changes in mental health state, physical discomfort, increasing tics, loss of concentration, fatigue, etc.?
Is personal contact preferable or more encouraging, for example, for building rapport and trust with the individual?
Consider whether the recruitment method selected may impact on any experience of stigma around mental health:
Providing a virtual safe space (online) may be beneficial, but the safety of this space relies on participants having secure and private access to a safe space and a device that can access the Internet.
Consider the impact of the relationship between participants living with mental illness and the research team:
Trusting relationships are deemed important for both recruitment and retention of participants living with mental ill health. Knowing that a health care provider understands an illness and can offer personalised support can be reassuring.
Will your recruitment strategy choices contribute to maintaining or building trust with this group? Online recruitment, such as social media, can be seen as distant and disengaging compared to in-person recruitment. Regular trial updates and information sharing through short videos or live ‘chats’ may help ‘humanise’ the trial on digital platforms.
Develop your recruitment approach (offline/online/mixed) by working in partnership with potential participants and members of the public that share characteristics with your target population group. You can identify PPI contributors through your local employing organisation or through professional or existing research or public contributor groups such as Sprouting Minds https://digitalyouth.ac.uk/the-digital-youth-programme/about-sprouting-minds/ . Please note that most UK National Health Service (NHS) Trusts have established PPI Groups.
Build in flexibility where possible at the protocol development stage, to ensure that participants with fluctuating symptoms can remain engaged in a safe and supported way. This may be achieved in several ways, for example, by offering a mixed recruitment strategy to allow individuals to choose how they want to participate. Alternatively, you may select an online recruitment strategy via Facebook for the initial approach to participate but then build in telephone or in-person opportunities for eligibility checks or follow-ups. It is important to ensure participants know that these options exist at the earliest opportunity.
- Inclusivity
RE-MIND identified a number of specific challenges to inclusive recruitment into mental health clinical trials. Continuing stigma surrounding mental health was a significant factor on a political, cultural, community, and individual level, underpinned by lack of education and mistrust of services and research [ 10 ]. In addition, lack of researcher skills and experience in inclusive recruitment strategies has also been found to contribute to underrepresentation in clinical research [ 15 , 16 ]. This highlights the critical role of PPI in understanding a trial population’s needs. It is also vital to educate researchers on equality and diversity, to enable co-design and selection of suitable recruitment methods to improve representation in mental health clinical trials, for example, through better implementation of the UK’s National Institute for Health Research (NIHR) INCLUDE ethnicity framework [ 1 ].
Consider the impact of the relationship between participants from marginalised groups and the research team:
Will your recruitment strategy choices contribute to maintaining or building trust with this group, for example, those living with mental health illness in rural or under-served communities may benefit from an online recruitment strategy?
Can you develop relationships with local and/or national community groups to build trust in your research? Identify community group leaders who will advocate for your research.
Do you have connections with trusted members of the community to support the building and development of relationships to facilitate inclusive recruitment? This can be in-person or online for example. through administrators of Facebook groups, libraries, or leaders of interest groups.
Do you have a PPI member with lived experience on your research team who can advocate for your research with community groups? Establishing connections through shared experience can help break down barriers of mistrust and misunderstanding.
Consider which recruitment methods your target participant populations may prefer. Living with a mental health illness can be complex due to fluctuating health status or exacerbation of symptoms:
Think about what factors may be most important to them, e.g. if they are working, parents, carers, and/or attending school, then convenience may be the main factor to target.
Consider the range of media platforms available to target people who are educationally or socioeconomically diverse.
If local IT access, e.g. poor Internet access is known in a geographical area, consider using mixed methods for recruitment to improve inclusivity.
Consider information provision and accessibility when selecting your recruitment methods:
Consider whether the methods you are using to recruit and retain participants allow for language (written and/or spoken) needs to be met, e.g., using a translation service.
Consider whether the recruitment method selected allows you to adequately communicate what you need to your participants for example:
Social media platforms such as X (previously Twitter) or use of SMS text-based services have character limitations. Could any language or phrasing lead to misinterpretation or misunderstanding
Use of clinical or diagnostic terms, phrases and labels when considering issues of stigma.
If you are using offline methods, are they accessible for people in a physical sense? E.g., people with motor/mobility needs, or visual or auditory difficulties.
If you are using online methods, are the colours, font, and imagery that you are using inclusive? E.g., alt text for images, colour blindness, colour contrast and font readability.
Work in partnership with people with lived experience and members of the public that share characteristics with your target population group. Explore the needs of both the trial team and the target population group and select methods that are effective for both parties.
Greater sensitivities and confidentiality in mental health care mean that relationships and trust are critical, which may be easier to facilitate face-to-face. However, online recruitment may offer greater flexibility and convenience for participants, for example, by supporting those who may find in-person contact challenging due to their illness. When selecting a recruitment strategy , be mindful of both advantages and drawbacks of the strategy used.
Avoid stereotypes, particularly related to age, when thinking about online methods. For example, technology as a barrier is likely reduced with each generation as well as recent necessity to engage with digital communications (e.g. smartphones, WhatsApp, Facebook, videoconferencing platforms) due to the COVID pandemic.
Identify the main demographic characteristic(s) that is important to engage with your trial, and then consider how other characteristics may impact how they react to the recruitment strategy you have in mind. For example, if you know you want to include young people, consider using TikTok, whereas Facebook may be preferable for older participants. It is important to think about other characteristics that may impact if/how social media is used, e.g. mental health status, socioeconomic status, health status, gender.
There are a growing number of community-led mental illness specific support groups on social media. Can you access and/or engage these groups to help with recruitment? Care should be taken not to harm the safe spaces afforded by these groups, for example for a researcher joining a group purely for the purpose of trial promotion.
Data management
RE-MIND found that for people living with mental health illness, there remained a significant element of fear and mistrust in using online methods underpinned by the stigma and vulnerability of mental health illness with the potential for confidentiality to be broken [ 10 ]. Understanding safeguards for the range of digital platforms was particularly complex and in line with other research suggests better regulation is needed of digital platforms [ 17 ], which at times were not deemed as stringent as clinical trial requirements.
Consider putting appropriate safeguards in place for the recruitment methods selected, e.g. firewalls, General data Protection Regulation (GDPR), secure server.
Can you use a quick response (QR) code to improve security and safety. A QR code is an image scannable by a digital device that can impart information.
Does your organisation have data management policies for use of digital platforms such as social media that must be adhered to? Consider local policies required for multi-site trials.
Is your recruitment method a credible source, e.g. not mistaken for spam, phishing?
Allocate a moderator for engagement with online public groups to ensure safeguarding and wellbeing of people engaging with the content.
How will you inform potential participants about how their data will be shared and/or managed online?
Consider the resources required to adequately manage large numbers of enquiries generated by online strategies:
Do you have the resources to support the additional work associated with screening and monitoring of data quality?
Ensure that eligibility criteria are clearly communicated to potential participants.
Invest adequate time and resources in ensuring your data management systems are secure and safe for participants. You may want to make use restrictive software features for online methods.
Invest time to ensure security and safety methods are communicated clearly. You should work in partnership with potential participants and members of the public that share characteristics with your potential participant group to do this.
Staff training and support
The process of targeting recruitment using an online strategy has been considered as more time-efficient and cost-effective than traditional offline (in-person) recruitment [ 12 ]. However, knowledge of digital platforms and access to organisational and technical support and funding were the most common challenges researchers cited when selecting a recruitment strategy in the RE-MIND study [ 10 ]. It appears that despite advances in technology offering greater opportunity to reach wider audiences, many of these advances remain underutilised without adequate support and resources.
Consider identifying trials involving similar participant populations to learn from their experience of recruitment:
For example, information on trials can be accessed from ClinicalTrials.Gov, PubMed, etc.
Remember that this relies on adequate reporting of recruitment strategy.
Think about how previous trials could have been improved.
Consider the impact of researchers/recruiters being in/adequately trained and knowledgeable on how to use the online recruitment methods you have chosen:
If you are using social media, does your organisation have policies and/or expertise that can be used to support engagement on specific platforms
Does your organisation have procedures for payment for social media promotion?
Do you have local services available to support at an organisational level when things go wrong, e.g. IT, marketing, or communications teams?
Ensure your recruitment methods are appropriately funded, for example, advertising costs per click; do you need a professional designer to produce visual summaries of the research, such as infographics?
Do you have lived experience patient and public input on the selection of recruitment strategy, including content and presentation?
Make a conscious effort to learn from previous trials aimed at the populations you are intending to recruit. Reflect upon how these trials may differ from yours and how that may impact your selection of recruitment process (e.g., severity or stage of mental health illness, intervention type, locality, country, setting, healthcare system, culture).
Ensure research teams are adequately trained on systems and software, and that they know where to go when systems fail, or if they have unanswered questions.
Conclusions
This list of considerations and recommendations is based on the experiences of key partners and the findings from the RE-MIND project, outlining factors to consider when planning recruitment strategies in mental health research/clinical trials. It should be used as a starting point for discussions among the trial team. We acknowledge the potential limitations of each consideration in context of individual and/or organisational capacity, funding and resources available.
The process of selecting a suitable recruitment method should give due consideration to the study population as well as the resources (including staff time and training) needed to implement that method. The ideal juncture to do this is when writing a trial grant funding proposal to ensure adequate resourcing. However, we encourage trial teams that are struggling to recruit to use our considerations and recommendations to re-evaluate their approach to recruitment.
The considerations are designed to be used flexibly based on the target population to be recruited. Greater consideration should be given to using online or mixed methods recruitment strategies that adopt a tailored approach, offering flexibility and choice, to enable wider participation. For future work, we recommend revisiting and re-evaluating these considerations after they have been implemented in practical settings. This process of reassessment will allow us to gain valuable insights into the real-world impact and effectiveness of our proposed strategies. It will also enable us to make necessary adjustments, fine-tune our recommendations, and ensure their continued relevance and success in evolving contexts.
Availability of data and materials
The data collected, used, and/or analysed during the current study are available from the Nottingham Clinical Trials Unit (NCTU) via the corresponding author on reasonable request.
Abbreviations
General Data Protection Regulation
Patient and public involvement
Nottingham Clinical Trials Unit
National health Service
National Institute for Health and Care Research
Quick response
Randomised controlled trial
Short message service
REcruitment in Mental health trials: broadening the ‘net’, opportunities for INclusivity through online methoDs
United Kingdom
National Institute for Health Research. Improving inclusion of under-served groups in clinical research: Guidance from the NIHR INCLUDE project 2020 [09August2023]. Available from: https://www.nihr.ac.uk/documents/improving-inclusion-of-under-served-groups-in-clinical-research-guidance-from-include-project/25435 .
Clark LT, Watkins L, Piña IL, Elmer M, Akinboboye O, Gorham M, et al. Increasing diversity in clinical trials: overcoming critical barriers. Curr Probl Cardiol. 2019;44(5):148–72.
Article PubMed Google Scholar
Robards F, Kang M, Usherwood T, Sanci L. How marginalized young people access, engage with, and navigate health-care systems in the digital age: systematic review. J Adolesc Health. 2018;62(4):365–81.
Pattyn E, Verhaeghe M, Bracke P. The gender gap in mental health service use. Soc Psychiatry Psychiatr Epidemiol. 2015;50(7):1089–95.
Tiwari SK, Wang J. Ethnic differences in mental health service use among White, Chinese, South Asian and South East Asian populations living in Canada. Soc Psychiatry Psychiatr Epidemiol. 2008;43(11):866–71.
Brenes GA, Danhauer SC, Lyles MF, Hogan PE, Miller ME. Barriers to mental health treatment in rural older adults. Am J Geriatr Psychiatry. 2015;23(11):1172–8.
Article PubMed PubMed Central Google Scholar
Barak A. Psychological applications on the Internet: a discipline on the threshold of a new millennium. Appl Prev Psychol. 1999;8(4):231–45.
Article Google Scholar
Akers L, Gordon JS. Using Facebook for large-scale online randomized clinical trial recruitment: effective advertising strategies. J Med Internet Res. 2018;20(11): e290.
Iflaifel M, Hall CL, Green HR, Willis A, Rennick-Egglestone S, Juszczak E, et al. Recruitment strategies in mental health clinical trials: a scoping review (RE-MIND Study). Trials [In Peer Review]. June 2024.
Iflaifel M, Hall CL, Green HR, Willis A, Rennick-Egglestone S, Juszczak E, et al. Widening participation – recruitment methods in mental health randomised controlled trials: a qualitative study. BMC Med Res Methodol. 2023;23(1):211.
Farmer T, Robinson K, Elliott SJ, Eyles J. Developing and Implementing a Triangulation Protocol for Qualitative Health Research. Qual Health Res. 2006;16(3):377–94.
Brøgger-Mikkelsen M, Ali Z, Zibert JR, Andersen AD, Thomsen SF. Online patient recruitment in clinical trials: systematic review and meta-analysis. J Med Internet Res. 2020;22(11): e22179.
Kaminsky A, Roberts LW, Brody JL. Influences upon willingness to participate in schizophrenia research: an analysis of narrative data from 63 people with schizophrenia. Ethics Behav. 2003;13(3):279–302.
Woodall A, Morgan C, Sloan C, Howard L. Barriers to participation in mental health research: are there specific gender, ethnicity and age related barriers? BMC Psychiatry. 2010;10:103.
Kusnoor SV, Villalta-Gil V, Michaels M, Joosten Y, Israel TL, Epelbaum MI, et al. Design and implementation of a massive open online course on enhancing the recruitment of minorities in clinical trials - Faster Together. BMC Med Res Methodol. 2021;21(1):44.
Niranjan SJ, Durant RW, Wenzel JA, Cook ED, Fouad MN, Vickers SM, et al. Training needs of clinical and research professionals to optimize minority recruitment and retention in cancer clinical trials. J Cancer Educ. 2019;34(1):26–34.
Mühlhoff R, Willem T. Social media advertising for clinical studies: ethical and data protection implications of online targeting. Big Data Soc. 2023;10(1):20539517231156130.
Download references
Acknowledgements
The study authors would like to thank all our focus group and interview participants and the UK Clinical Research Collaboration for their support of the project culminating in these recommendations for future practice.
This project is funded by the National Institute for Health and Care Research (NIHR) CTU Support Funding scheme. The views expressed are those of the author(s) and not necessarily those of the NIHR or the Department of Health and Social Care. Open Access funding provided by The University of Nottingham. Stefan Rennick-Egglestone and Charlotte L Hall were supported by the NIHR Nottingham Biomedical Research Centre (NIHR203310).
Author information
Authors and affiliations.
Nottingham Clinical Trials Unit, University of Nottingham, Nottingham, UK
Mais Iflaifel, Edmund Juszczak & Kirsty Sprange
Institute of Mental Health, School of Medicine, NIHR MindTech MedTech HRC, Mental Health and Clinical Neurosciences, University of Nottingham, Innovation Park, Triumph Road, Nottingham, UK
Charlotte L. Hall & Jennifer Martin
Institute of Mental Health, NIHR Nottingham Biomedical Research Centre, University of Nottingham, Innovation Park, Triumph Road, Nottingham, UK
Charlotte L. Hall, Stefan Rennick-Egglestone & Jennifer Martin
Health Services Research Unit, University of Aberdeen, Aberdeen, UK
Heidi R. Green
COUCH Health, Manchester, UK
Leicester/Diabetes Research Centre, Centre for Ethnic Health Research, University of Leicester, Leicester, UK
Andrew Willis
School of Health Sciences, Institute of Mental Health, University of Nottingham, Nottingham, UK
Stefan Rennick-Egglestone
NIHR Evaluation, Trials and Studies Coordinating Centre (NETSCC), Southampton, UK
Mark Townsend
You can also search for this author in PubMed Google Scholar
Contributions
All authors contributed to designing the RE-MIND study. All authors contributed to the selection and refinement of the recommendations. MI and KS drafted the initial manuscript. All authors reviewed and edited drafts of the manuscript. All authors accepted the final version of the manuscript.
Corresponding author
Correspondence to Kirsty Sprange .
Ethics declarations
Ethics approval and consent to participate.
The RE-MIND study received approval from the University of Nottingham Research Ethics Committee (FMHS 13–0422) on 13 June 2022.
Consent for publication
Not applicable.
Competing interests
The authors declare no competing interests.
Additional information
Publisher’s note.
Springer Nature remains neutral with regard to jurisdictional claims in published maps and institutional affiliations.
Rights and permissions
Open Access This article is licensed under a Creative Commons Attribution 4.0 International License, which permits use, sharing, adaptation, distribution and reproduction in any medium or format, as long as you give appropriate credit to the original author(s) and the source, provide a link to the Creative Commons licence, and indicate if changes were made. The images or other third party material in this article are included in the article's Creative Commons licence, unless indicated otherwise in a credit line to the material. If material is not included in the article's Creative Commons licence and your intended use is not permitted by statutory regulation or exceeds the permitted use, you will need to obtain permission directly from the copyright holder. To view a copy of this licence, visit http://creativecommons.org/licenses/by/4.0/ .
Reprints and permissions
About this article
Cite this article.
Iflaifel, M., Hall, C.L., Green, H.R. et al. Using online methods to recruit participants into mental health clinical trials: considerations and recommendations from the RE-MIND study. Trials 25 , 596 (2024). https://doi.org/10.1186/s13063-024-08435-9
Download citation
Received : 12 January 2024
Accepted : 23 August 2024
Published : 07 September 2024
DOI : https://doi.org/10.1186/s13063-024-08435-9
Share this article
Anyone you share the following link with will be able to read this content:
Sorry, a shareable link is not currently available for this article.
Provided by the Springer Nature SharedIt content-sharing initiative
- Mental health
- Clinical trial
- Recruitment
ISSN: 1745-6215
- Submission enquiries: Access here and click Contact Us
- General enquiries: [email protected]
Information
- Author Services
Initiatives
You are accessing a machine-readable page. In order to be human-readable, please install an RSS reader.
All articles published by MDPI are made immediately available worldwide under an open access license. No special permission is required to reuse all or part of the article published by MDPI, including figures and tables. For articles published under an open access Creative Common CC BY license, any part of the article may be reused without permission provided that the original article is clearly cited. For more information, please refer to https://www.mdpi.com/openaccess .
Feature papers represent the most advanced research with significant potential for high impact in the field. A Feature Paper should be a substantial original Article that involves several techniques or approaches, provides an outlook for future research directions and describes possible research applications.
Feature papers are submitted upon individual invitation or recommendation by the scientific editors and must receive positive feedback from the reviewers.
Editor’s Choice articles are based on recommendations by the scientific editors of MDPI journals from around the world. Editors select a small number of articles recently published in the journal that they believe will be particularly interesting to readers, or important in the respective research area. The aim is to provide a snapshot of some of the most exciting work published in the various research areas of the journal.
Original Submission Date Received: .
- Active Journals
- Find a Journal
- Proceedings Series
- For Authors
- For Reviewers
- For Editors
- For Librarians
- For Publishers
- For Societies
- For Conference Organizers
- Open Access Policy
- Institutional Open Access Program
- Special Issues Guidelines
- Editorial Process
- Research and Publication Ethics
- Article Processing Charges
- Testimonials
- Preprints.org
- SciProfiles
- Encyclopedia
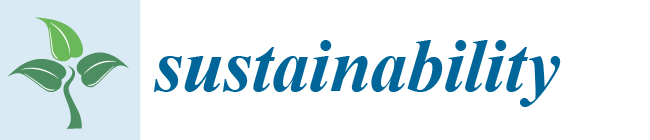
Article Menu
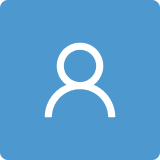
- Subscribe SciFeed
- Recommended Articles
- Google Scholar
- on Google Scholar
- Table of Contents
Find support for a specific problem in the support section of our website.
Please let us know what you think of our products and services.
Visit our dedicated information section to learn more about MDPI.
JSmol Viewer
Agility and resilience in supply chains: investigating their roles in enhancing financial performance.
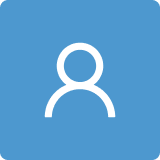
1. Introduction
2. literature review, 2.1. theoretical framework, 2.2. hypotheses development, 2.2.1. supply chain management (scm) and financial performance (fp), 2.2.2. the moderating role of agility in the relationship between scm and fp, 2.2.3. interaction of sca and scres in the relationship between scm and fp.
- To ensure that the damaged system is restored to its desired state within an optimal period and at an optimal cost level;
- To reduce the effects of a possible disturbance by changing the effectiveness level of a potential threat.
3. Methodology
3.1. research design, 3.2. data collection and sampling, 3.3. measurement of variables, 3.4. data analysis techniques, 4.1. descriptive statistics of the sample, 4.2. exploratory factor analysis results, 4.3. confirmatory factor analysis results, 4.4. hayes process macro model 3 results, 5. conclusions and discussion, limitations and recommendations for the future studies, author contributions, institutional review board statement, informed consent statement, data availability statement, conflicts of interest.
Collaboration with suppliers | |
ALL1 | The company confirms communication openness with the basic suppliers. |
ALL2 | The company deals with its suppliers based on the partnership. |
ALL3 | The company works to engage the basic suppliers in process of developing its products and services. |
ALL4 | The company’s strategy depends on building good relationships with the basic suppliers. |
Customer Relationship Management | |
CRM1 | Customer satisfaction is a good which the company seeks for. |
CRM2 | In the company there is a specialized division for the customer’s service. |
CRM3 | The company deals with the customers notes and complaints in an appropriate way. |
CRM4 | The company keeps a complete database about the customers. |
Logistics | |
LOG1 | Does the company respond to the orders from time of receiving the order and during its transportation and till handling the bill and receiving the financial merits? |
LOG2 | Is there a system in the company for accuracy and complete orders—the absence of returned orders? |
LOG3 | Logistics management in the company includes planning, scheduling the productions, and monitoring them. |
LOG4 | Logistic management includes all planning and implementation levels (The Executive and Tactical Strategy). |
Flow Information and Knowledge Sharing | |
INF1 | The company possesses an electronic system to speed up the information exchange internally. |
INF2 | The company uses the electronic networks for exchanging information with the customers. |
INF3 | The company uses the electronic networks to exchange information with the suppliers. |
INF4 | The company shares the knowledge and the information with the suppliers in building its plans. |
SCA1 | Our supply chain is able to respond to changes in demand without overstock or lost sales. |
SCA2 | Our supply chain is capable of forecasting market demand and responding to real market demand. |
SCA3 | Joint planning with suppliers is important in purchasing, production, and logistics. |
SCA4 | Information integration with suppliers, logistic service providers, and customers in the supply chain is important. |
SCA5 | Improving our level of customer service is a high priority. |
SCA6 | Improving delivery reliability is a high priority. |
SCA7 | Improving responsiveness to changing market needs is a high priority. |
SCA8 | Inventory and demand levels are visible throughout the supply chain |
SCRES1 | We are able to cope with changes brought by the supply chain disruption. |
SCRES2 | We are able to adapt to supply chain disruption easily. |
SCRES3 | We are able to provide a quick response to supply chain disruption. |
SCRES4 | We are able to maintain high situational awareness at all times. |
FP1 | Over the past three years, our financial performance has been outstanding. |
FP2 | Over the past three years, our financial performance has exceeded our competitors’. |
FP3 | Over the past three years, our sales growth has been outstanding. |
FP4 | Over the past three years, we have been more profitable than our competitors. |
FP5 | Over the past three years, our sales growth has exceeded our competitors’. |
- Kazancoglu, I.; Ozbiltekin-Pala, M.; Kumar Mangla, S.; Kazancoglu, Y.; Jabeen, F. Role of Flexibility, Agility and Responsiveness for Sustainable Supply Chain Resilience during COVID-19. J. Clean. Prod. 2022 , 362 , 132431. [ Google Scholar ] [ CrossRef ]
- Yu, W.; Jacobs, M.A.; Chavez, R.; Yang, J. Dynamism, Disruption Orientation, and Resilience in the Supply Chain and the Impacts on Financial Performance: A Dynamic Capabilities Perspective. Int. J. Prod. Econ. 2019 , 218 , 352–362. [ Google Scholar ] [ CrossRef ]
- Li, Y.; Zobel, C.W. Exploring Supply Chain Network Resilience in the Presence of the Ripple Effect. Int. J. Prod. Econ. 2020 , 228 , 107693. [ Google Scholar ] [ CrossRef ]
- Yu, M.; Zhang, R. Understanding the Recent Sino-U.S. Trade Conflict. China Econ. J. 2019 , 12 , 160–174. [ Google Scholar ] [ CrossRef ]
- Gereffi, G. What Does the COVID-19 Pandemic Teach Us about Global Value Chains? The Case of Medical Supplies. J. Int. Bus. Policy 2020 , 3 , 287–301. [ Google Scholar ] [ CrossRef ]
- Craighead, C.W.; Ketchen, D.J., Jr.; Darby, J.L. Pandemics and Supply Chain Management Research: Toward a Theoretical Toolbox*. Decis. Sci. 2020 , 51 , 838–866. [ Google Scholar ] [ CrossRef ]
- de Borba, J.C.R.; Trabasso, L.G.; Pessôa, M.V.P. Agile Management in Product Development. Res.-Technol. Manag. 2019 , 62 , 63–67. [ Google Scholar ] [ CrossRef ]
- Alsmairat, M.A.K.; AL-Shboul, M.A. Enabling Supply Chain Efficacy through Supply Chain Absorptive Capacity and Ambidexterity: Empirical Study from Middle East Region—A Moderated-Mediation Model. J. Manuf. Technol. Manag. 2023 , 34 , 917–936. [ Google Scholar ] [ CrossRef ]
- Milewska, B.; Milewski, D. Implications of Increasing Fuel Costs for Supply Chain Strategy. Energies 2022 , 15 , 6934. [ Google Scholar ] [ CrossRef ]
- Sodhi, M.S.; Tang, C.S. Extending AAA Capabilities to Meet PPP Goals in Supply Chains. Prod. Oper. Manag. 2021 , 30 , 625–632. [ Google Scholar ] [ CrossRef ]
- Azadegan, A.; Mellat Parast, M.; Lucianetti, L.; Nishant, R.; Blackhurst, J. Supply Chain Disruptions and Business Continuity: An Empirical Assessment. Decis. Sci. 2020 , 51 , 38–73. [ Google Scholar ] [ CrossRef ]
- Birkie, S.E.; Trucco, P. Do Not Expect Others Do What You Should! Supply Chain Complexity and Mitigation of the Ripple Effect of Disruptions. Int. J. Logist. Manag. 2020 , 31 , 123–144. [ Google Scholar ] [ CrossRef ]
- Katsaliaki, K.; Galetsi, P.; Kumar, S. Supply Chain Disruptions and Resilience: A Major Review and Future Research Agenda. Ann. Oper. Res. 2022 , 319 , 965–1002. [ Google Scholar ] [ CrossRef ] [ PubMed ]
- Swafford, P.M.; Ghosh, S.; Murthy, N. Achieving Supply Chain Agility through IT Integration and Flexibility. Int. J. Prod. Econ. 2008 , 116 , 288–297. [ Google Scholar ] [ CrossRef ]
- Yawson, D.E.; Yamoah, F.A. Review of Strategic Agility: A Holistic Framework for Fresh Produce Supply Chain Disruptions. Sustainability 2022 , 14 , 14977. [ Google Scholar ] [ CrossRef ]
- Allaoui, H.; Guo, Y.; Sarkis, J. Decision Support for Collaboration Planning in Sustainable Supply Chains. J. Clean. Prod. 2019 , 229 , 761–774. [ Google Scholar ] [ CrossRef ]
- Zhou, H.; Benton, W.C. Supply Chain Practice and Information Sharing. J. Oper. Manag. 2007 , 25 , 1348–1365. [ Google Scholar ] [ CrossRef ]
- Chowdhury, A.H.M.Y.; Islam, N. Supply Chain Management and Operational Performance: A Critical Evaluation of Available Literature. Int. J. Appl. Bus. Manag. Sci. 2022 , 2 , 235–251. [ Google Scholar ]
- Jahed, M.A.; Quaddus, M.; Suresh, N.C.; Salam, M.A.; Khan, E.A. Direct and Indirect Influences of Supply Chain Management Practices on Competitive Advantage in Fast Fashion Manufacturing Industry. J. Manuf. Technol. Manag. 2022 , 33 , 598–617. [ Google Scholar ] [ CrossRef ]
- Mukhsin, M.; Suryanto, T. The Effect of Sustainable Supply Chain Management on Company Performance Mediated by Competitive Advantage. Sustainability 2022 , 14 , 818. [ Google Scholar ] [ CrossRef ]
- Leuschner, R.; Rogers, D.S.; Charvet, F.F. A Meta-Analysis of Supply Chain Integration and Firm Performance. J. Supply Chain Manag. 2013 , 49 , 34–57. [ Google Scholar ] [ CrossRef ]
- Pfohl, H.-C.; Köhler, H.; Thomas, D. State of the Art in Supply Chain Risk Management Research: Empirical and Conceptual Findings and a Roadmap for the Implementation in Practice. Logist. Res. 2010 , 2 , 33–44. [ Google Scholar ] [ CrossRef ]
- Ponis, S.T.; Koronis, E. Supply Chain Resilience: Definition Of Concept And Its Formative Elements. J. Appl. Bus. Res. JABR 2012 , 28 , 921–930. [ Google Scholar ] [ CrossRef ]
- Aldrighetti, R.; Battini, D.; Ivanov, D.; Zennaro, I. Costs of Resilience and Disruptions in Supply Chain Network Design Models: A Review and Future Research Directions. Int. J. Prod. Econ. 2021 , 235 , 108103. [ Google Scholar ] [ CrossRef ]
- Blecker, T.; Kersten, W.; Lüthje, C. Innovative Process Optimization Methods in Logistics: Emerging Trends, Concepts and Technologies ; Erich Schmidt Verlag GmbH & Co KG: Berlin, Germany, 2010; ISBN 978-3-503-12683-5. [ Google Scholar ]
- Mason-Jones, R.; Naylor, B.; Towill, D.R. Engineering the Leagile Supply Chain. Int. J. Agile Manag. Syst. 2000 , 2 , 54–61. [ Google Scholar ] [ CrossRef ]
- Aitken, J.; Christopher, M.; Towill, D. Understanding, Implementing and Exploiting Agility and Leanness. Int. J. Logist. Res. Appl. 2002 , 5 , 59–74. [ Google Scholar ] [ CrossRef ]
- Martin, C.; Towill, D.R. Supply Chain Migration from Lean and Functional to Agile and Customised. Supply Chain Manag. Int. J. 2000 , 5 , 206–213. [ Google Scholar ] [ CrossRef ]
- Tufan, C.; Kılıç, Y. Borsa istanbul’da işlem gören lojistik işletmelerinin finansal performanslarinin topsis ve vikor yöntemleriyle değerlendirilmesi. Cumhur. Üniversitesi İktisadi İdari Bilim. Derg. 2019 , 20 , 119–137. [ Google Scholar ]
- Carvalho, H.; Azevedo, S.G.; Cruz-Machado, V. Agile and Resilient Approaches to Supply Chain Management: Influence on Performance and Competitiveness. Logist. Res. 2012 , 4 , 49–62. [ Google Scholar ] [ CrossRef ]
- Oh, S.; Ryu, Y.U.; Yang, H. Interaction Effects between Supply Chain Capabilities and Information Technology on Firm Performance. Inf. Technol. Manag. 2019 , 20 , 91–106. [ Google Scholar ] [ CrossRef ]
- Shi, M.; Yu, W. Supply Chain Management and Financial Performance: Literature Review and Future Directions. Int. J. Oper. Prod. Manag. 2013 , 33 , 1283–1317. [ Google Scholar ] [ CrossRef ]
- Kale, E.; Aknar, A.; Başar, Ö. Absorptive Capacity and Firm Performance: The Mediating Role of Strategic Agility. Int. J. Hosp. Manag. 2019 , 78 , 276–283. [ Google Scholar ] [ CrossRef ]
- Penrose, E.T. The Theory of the Growth of the Firm , 3rd ed.; Oxford University Press: Oxford, UK, 1995; ISBN 978-0-19-828977-7. [ Google Scholar ]
- Peteraf, M.A. The Cornerstones of Competitive Advantage: A Resource-Based View. Strateg. Manag. J. 1993 , 14 , 179–191. [ Google Scholar ] [ CrossRef ]
- Hitt, M.A.; Ireland, R.D.; Hoskisson, R.E. Strategic Management: Competitiveness and Globalization, Concepts , 8th ed.; Cengage Learning: Mason, OH, USA, 2008; ISBN 978-0-324-58112-6. [ Google Scholar ]
- Barney, J.B. Firm Resources and Sustained Competitive Advantage. In Economics Meets Sociology in Strategic Management ; Baum, J.A.C., Dobbin, F., Eds.; Advances in Strategic Management; Emerald Group Publishing Limited: Bradford, UK, 2000; Volume 17, pp. 203–227. ISBN 978-1-84950-051-7. [ Google Scholar ]
- Dyer, J.H.; Singh, H. The Relational View: Cooperative Strategy and Sources of Interorganizational Competitive Advantage. Acad. Manag. Rev. 1998 , 23 , 660–679. [ Google Scholar ] [ CrossRef ]
- Oliver, C. Sustainable Competitive Advantage: Combining Institutional and Resource-Based Views. Strateg. Manag. J. 1997 , 18 , 697–713. [ Google Scholar ] [ CrossRef ]
- Prahalad, C.K.; Hamel, G. The Core Competence of the Corporation. Harv. Bus. Rev. 1990 , 68 , 79–91. [ Google Scholar ]
- Antoni, D.; Jie, F.; Abareshi, A. Critical Factors in Information Technology Capability for Enhancing Firm’s Environmental Performance: Case of Indonesian ICT Sector. Int. J. Agile Syst. Manag. 2020 , 13 , 159. [ Google Scholar ] [ CrossRef ]
- Boxall, P. Achieving Competitive Advantage through Human Resource Strategy: Towards a Theory of Industry Dynamics. Hum. Resour. Manag. Rev. 1998 , 8 , 265–288. [ Google Scholar ] [ CrossRef ]
- Helfat, C.E.; Finkelstein, S.; Mitchell, W.; Peteraf, M.; Singh, H.; Teece, D.; Winter, S.G. Dynamic Capabilities: Understanding Strategic Change in Organizations , 1st ed.; Wiley-Blackwell: Malden, MA, USA, 2007; ISBN 978-1-4051-3575-7. [ Google Scholar ]
- Luo, Y. Dynamic Capabilities in International Expansion. J. World Bus. 2000 , 35 , 355–378. [ Google Scholar ] [ CrossRef ]
- Teece, D.J.; Pisano, G.; Shuen, A. Dynamic Capabilities and Strategic Management. Strateg. Manag. J. 1997 , 18 , 509–533. [ Google Scholar ] [ CrossRef ]
- Eisenhardt, K.M.; Martin, J.A. Dynamic Capabilities: What Are They? Strateg. Manag. J. 2000 , 21 , 1105–1121. [ Google Scholar ] [ CrossRef ]
- Ferreira, J.; Coelho, A.; Moutinho, L. Dynamic Capabilities, Creativity and Innovation Capability and Their Impact on Competitive Advantage and Firm Performance: The Moderating Role of Entrepreneurial Orientation. Technovation 2020 , 92–93 , 102061. [ Google Scholar ] [ CrossRef ]
- Khan, M.S.; Elahi, N.S.; Abid, G. Workplace Incivility and Job Satisfaction: Mediation of Subjective Well-Being and Moderation of Forgiveness Climate in Health Care Sector. Eur. J. Investig. Health Psychol. Educ. 2021 , 11 , 1107–1119. [ Google Scholar ] [ CrossRef ] [ PubMed ]
- Nguyen, P.V.; Huynh, H.T.N.; Lam, L.N.H.; Le, T.B.; Nguyen, N.H.X. The Impact of Entrepreneurial Leadership on SMEs’ Performance: The Mediating Effects of Organizational Factors. Heliyon 2021 , 7 , e07326. [ Google Scholar ] [ CrossRef ]
- Peng, M.Y.-P.; Zhang, Z.; Yen, H.-Y.; Yang, S.-M. Dynamic Capabilities and Firm Performance in the High-Tech Industry: Quadratic and Moderating Effects under Differing Ambidexterity Levels. Sustainability 2019 , 11 , 5004. [ Google Scholar ] [ CrossRef ]
- Pezeshkan, A.; Fainshmidt, S.; Nair, A.; Lance Frazier, M.; Markowski, E. An Empirical Assessment of the Dynamic Capabilities–Performance Relationship. J. Bus. Res. 2016 , 69 , 2950–2956. [ Google Scholar ] [ CrossRef ]
- Matarazzo, M.; Penco, L.; Profumo, G.; Quaglia, R. Digital Transformation and Customer Value Creation in Made in Italy SMEs: A Dynamic Capabilities Perspective. J. Bus. Res. 2021 , 123 , 642–656. [ Google Scholar ] [ CrossRef ]
- Kryscynski, D.; Coff, R.; Campbell, B. Charting a Path between Firm-specific Incentives and Human Capital-based Competitive Advantage. Strateg. Manag. J. 2021 , 42 , 386–412. [ Google Scholar ] [ CrossRef ]
- Fainshmidt, S.; Wenger, L.; Pezeshkan, A.; Mallon, M.R. When Do Dynamic Capabilities Lead to Competitive Advantage? The Importance of Strategic Fit. J. Manag. Stud. 2019 , 56 , 758–787. [ Google Scholar ] [ CrossRef ]
- Mikalef, P.; Boura, M.; Lekakos, G.; Krogstie, J. Big Data Analytics Capabilities and Innovation: The Mediating Role of Dynamic Capabilities and Moderating Effect of the Environment. Br. J. Manag. 2019 , 30 , 272–298. [ Google Scholar ] [ CrossRef ]
- Linde, L.; Sjödin, D.; Parida, V.; Wincent, J. Dynamic Capabilities for Ecosystem Orchestration A Capability-Based Framework for Smart City Innovation Initiatives. Technol. Forecast. Soc. Chang. 2021 , 166 , 120614. [ Google Scholar ] [ CrossRef ]
- Teece, D.J. Explicating Dynamic Capabilities: The Nature and Microfoundations of (Sustainable) Enterprise Performance. Strateg. Manag. J. 2007 , 28 , 1319–1350. [ Google Scholar ] [ CrossRef ]
- Awwad, A.S.; Ababneh, O.M.A.; Karasneh, M. The Mediating Impact of IT Capabilities on the Association between Dynamic Capabilities and Organizational Agility: The Case of the Jordanian IT Sector. Glob. J. Flex. Syst. Manag. 2022 , 23 , 315–330. [ Google Scholar ] [ CrossRef ] [ PubMed ]
- Hamidu, Z.; Boachie-Mensah, F.O.; Issau, K. Supply Chain Resilience and Performance of Manufacturing Firms: Role of Supply Chain Disruption. J. Manuf. Technol. Manag. 2023 , 34 , 361–382. [ Google Scholar ] [ CrossRef ]
- Piprani, A.Z.; Jaafar, N.I.; Ali, S.M.; Mubarik, M.S.; Shahbaz, M. Multi-Dimensional Supply Chain Flexibility and Supply Chain Resilience: The Role of Supply Chain Risks Exposure. Oper. Manag. Res. 2022 , 15 , 307–325. [ Google Scholar ] [ CrossRef ]
- Zhao, N.; Hong, J.; Lau, K.H. Impact of Supply Chain Digitalization on Supply Chain Resilience and Performance: A Multi-Mediation Model. Int. J. Prod. Econ. 2023 , 259 , 108817. [ Google Scholar ] [ CrossRef ]
- Wong, C.W.Y.; Lirn, T.-C.; Yang, C.-C.; Shang, K.-C. Supply Chain and External Conditions under Which Supply Chain Resilience Pays: An Organizational Information Processing Theorization. Int. J. Prod. Econ. 2020 , 226 , 107610. [ Google Scholar ] [ CrossRef ]
- Lee, S.M.; Rha, J.S. Ambidextrous Supply Chain as a Dynamic Capability: Building a Resilient Supply Chain. Manag. Decis. 2016 , 54 , 2–23. [ Google Scholar ] [ CrossRef ]
- Gibilaro, L.; Mattarocci, G. The Impact of Corporate Distress along the Supply Chain: Evidences from United States. Supply Chain Manag. Int. J. 2019 , 24 , 498–508. [ Google Scholar ] [ CrossRef ]
- Ellinger, A.E.; Natarajarathinam, M.; Adams, F.G.; Gray, J.B.; Hofman, D.; O’Marah, K. Supply Chain Management Competency and Firm Financial Success. J. Bus. Logist. 2011 , 32 , 214–226. [ Google Scholar ] [ CrossRef ]
- Tamayo-Torres, I.; Gutierrez-Gutierrez, L.; Ruiz-Moreno, A. Boosting Sustainability and Financial Performance: The Role of Supply Chain Controversies. Int. J. Prod. Res. 2019 , 57 , 3719–3734. [ Google Scholar ] [ CrossRef ]
- Wagner, S.M.; Grosse-Ruyken, P.T.; Erhun, F. The Link between Supply Chain Fit and Financial Performance of the Firm⋆. J. Oper. Manag. 2012 , 30 , 340–353. [ Google Scholar ] [ CrossRef ]
- Hendricks, K.B.; Singhal, V.R. The Effect of Supply Chain Glitches on Shareholder Wealth. J. Oper. Manag. 2003 , 21 , 501–522. [ Google Scholar ] [ CrossRef ]
- Dehning, B.; Richardson, V.J.; Zmud, R.W. The Financial Performance Effects of IT-based Supply Chain Management Systems in Manufacturing Firms. J. Oper. Manag. 2007 , 25 , 806–824. [ Google Scholar ] [ CrossRef ]
- Wang, Z.; Sarkis, J. Investigating the Relationship of Sustainable Supply Chain Management with Corporate Financial Performance. Int. J. Product. Perform. Manag. 2013 , 62 , 871–888. [ Google Scholar ] [ CrossRef ]
- Anantadjaya, S.P.; Nawangwulan, I.M.; Irhamsyah, M.; Carmelita, P.W. Supply Chain Management, Inventory Management & Financial Performance: Evidence from Manufacturing Firms. Linguist. Cult. Rev. 2021 , 5 , 781–794. [ Google Scholar ] [ CrossRef ]
- Zubairu, N.; Dinwoodie, J.; Govindan, K.; Hunter, L.; Roh, S. Supply Chain Strategies as Drivers of Financial Performance in Liquefied Natural Gas Networks. Supply Chain Manag. Int. J. 2021 , 26 , 579–591. [ Google Scholar ] [ CrossRef ]
- Zhao, G.; Feng, T.; Wang, D. Is More Supply Chain Integration Always Beneficial to Financial Performance? Ind. Mark. Manag. 2015 , 45 , 162–172. [ Google Scholar ] [ CrossRef ]
- Sharma, A.; Adhikary, A.; Borah, S.B. COVID-19′s Impact on Supply Chain Decisions: Strategic Insights from NASDAQ 100 Firms Using Twitter Data. J. Bus. Res. 2020 , 117 , 443–449. [ Google Scholar ] [ CrossRef ]
- Helmold, M.; Terry, B. Operations and Supply Management 4.0: Industry Insights, Case Studies and Best Practices ; Springer Nature: Berlin/Heidelberg, Germany, 2021; ISBN 978-3-030-68696-3. [ Google Scholar ]
- Farida, I.; Setiawan, D. Business Strategies and Competitive Advantage: The Role of Performance and Innovation. J. Open Innov. Technol. Mark. Complex. 2022 , 8 , 163. [ Google Scholar ] [ CrossRef ]
- Tatoglu, E.; Bayraktar, E.; Arda, O.A. Adoption of Corporate Environmental Policies in Turkey. J. Clean. Prod. 2015 , 91 , 313–326. [ Google Scholar ] [ CrossRef ]
- Christopher, M.; Ryals, L.J. The Supply Chain Becomes the Demand Chain. J. Bus. Logist. 2014 , 35 , 29–35. [ Google Scholar ] [ CrossRef ]
- Irfan, M.; Wang, M.; Akhtar, N. Impact of IT Capabilities on Supply Chain Capabilities and Organizational Agility: A Dynamic Capability View. Oper. Manag. Res. 2019 , 12 , 113–128. [ Google Scholar ] [ CrossRef ]
- Kumar, V.; Bak, O.; Guo, R.; Shaw, S.L.; Colicchia, C.; Garza-Reyes, J.A.; Kumari, A. An Empirical Analysis of Supply and Manufacturing Risk and Business Performance: A Chinese Manufacturing Supply Chain Perspective. Supply Chain Manag. Int. J. 2018 , 23 , 461–479. [ Google Scholar ] [ CrossRef ]
- Arokodare, M.A.; Asikhia, O.U. Strategic Agility: Achieving Superior Organizational Performance through Strategic Foresight. Glob. J. Manag. Bus. Res. 2020 , 20 , 7–16. [ Google Scholar ]
- Akkaya, B.; Qaısar, I. Linking Dynamic Capabilities and Market Performance of SMEs: The Moderating Role of Organizational Agility. Istanb. Bus. Res. 2021 , 50 , 197–214. [ Google Scholar ] [ CrossRef ]
- Shekarian, M.; Reza Nooraie, S.V.; Parast, M.M. An Examination of the Impact of Flexibility and Agility on Mitigating Supply Chain Disruptions. Int. J. Prod. Econ. 2020 , 220 , 107438. [ Google Scholar ] [ CrossRef ]
- Sadikoglu, E.; Demirkesen, S. Agile Supply Chain Management. In The Palgrave Handbook of Supply Chain Management ; Sarkis, J., Ed.; Springer International Publishing: Cham, Switzerland, 2022; pp. 1–25. ISBN 978-3-030-89822-9. [ Google Scholar ]
- Omoush, M. Investigation the Relationship Between Supply Chain Management Activities and Operational Performance: Testing the Mediating Role of Strategic Agility-A Practical Study on the Pharmaceutical Companies. Int. Bus. Res. 2020 , 13 , 74. [ Google Scholar ] [ CrossRef ]
- Betts, T.; Tadisina, S. Supply Chain Agility, Collaboration and Performance: How Do They Relate. In Proceedings of the POMS 20th Annual Conference, Orlando, FL, USA, 1–4 May 2009. [ Google Scholar ]
- Chakravarty, A.; Grewal, R.; Sambamurthy, V. Information Technology Competencies, Organizational Agility, and Firm Performance: Enabling and Facilitating Roles. Inf. Syst. Res. 2013 , 24 , 976–997. [ Google Scholar ] [ CrossRef ]
- Felipe, C.M.; Leidner, D.E.; Roldán, J.L.; Leal-Rodríguez, A.L. Impact of IS Capabilities on Firm Performance: The Roles of Organizational Agility and Industry Technology Intensity. Decis. Sci. 2020 , 51 , 575–619. [ Google Scholar ] [ CrossRef ]
- Gerald, E.; Obianuju, A.; Chukwunonso, N. Strategic Agility and Performance of Small and Medium Enterprises in the Phase of COVID-19 Pandemic. Int. J. Financ. Account. Manag. 2020 , 2 , 41–50. [ Google Scholar ] [ CrossRef ]
- Ofoegbu, O.E.; Akanbi, P.A. The Influence of Strategic Agility on the Perceived Performance of Manufacturing Firms in Nigeria. Int. Bus. Econ. Res. J. IBER 2012 , 11 , 153–160. [ Google Scholar ] [ CrossRef ]
- Men, F.; Yaqub, R.M.S.; Yan, R.; Irfan, M.; Haider, A. The Impact of Top Management Support, Perceived Justice, Supplier Management, and Sustainable Supply Chain Management on Moderating the Role of Supply Chain Agility. Front. Environ. Sci. 2023 , 10 , 1006029. [ Google Scholar ] [ CrossRef ]
- Tallon, P.P.; Pinsonneault, A. Competing Perspectives on the Link Between Strategic Information Technology Alignment and Organizational Agility: Insights from a Mediation Model. MIS Q. 2011 , 35 , 463. [ Google Scholar ] [ CrossRef ]
- Shin, H.; Lee, J.-N.; Kim, D.; Rhim, H. Strategic Agility of Korean Small and Medium Enterprises and Its Influence on Operational and Firm Performance. Int. J. Prod. Econ. 2015 , 168 , 181–196. [ Google Scholar ] [ CrossRef ]
- Hussain, Z.N.; Abood, Z.A.R.; Talib, A.H. Strategic Agility and Its Impact on Organizational Supply Chain Success: Applied Research in a Sample of the Faculties of the University of Babylon. Int. J. Supply Chain Manag. 2018 , 7 , 578–587. [ Google Scholar ] [ CrossRef ]
- Clauss, T.; Abebe, M.; Tangpong, C.; Hock, M. Strategic Agility, Business Model Innovation, and Firm Performance: An Empirical Investigation. IEEE Trans. Eng. Manag. 2021 , 68 , 767–784. [ Google Scholar ] [ CrossRef ]
- Suradi, S.; Ms, M.; Hasnawati, S. The Mediating Effect of Strategic Agility in the Relationship of Supply Chain Management Activities and Firm Performance of the Textile Industry of Indonesia. Int. J. Supply Chain Manag. 2020 , 9 , 649–656. [ Google Scholar ] [ CrossRef ]
- Inman, R.A.; Green, K.W. Environmental Uncertainty and Supply Chain Performance: The Effect of Agility. J. Manuf. Technol. Manag. 2021 , 33 , 239–258. [ Google Scholar ] [ CrossRef ]
- Salandri, L.; Cascio Rizzo, G.L.; Cozzolino, A.; De Giovanni, P. Green Practices and Operational Performance: The Moderating Role of Agility. J. Clean. Prod. 2022 , 375 , 134091. [ Google Scholar ] [ CrossRef ]
- Hohenstein, N.-O.; Feisel, E.; Hartmann, E.; Giunipero, L. Research on the Phenomenon of Supply Chain Resilience: A Systematic Review and Paths for Further Investigation. Int. J. Phys. Distrib. Logist. Manag. 2015 , 45 , 90–117. [ Google Scholar ] [ CrossRef ]
- Singh, C.S.; Soni, G.; Badhotiya, G.K. Performance Indicators for Supply Chain Resilience: Review and Conceptual Framework. J. Ind. Eng. Int. 2019 , 15 , 105–117. [ Google Scholar ] [ CrossRef ]
- Ishak, A.W.; Williams, E.A. A Dynamic Model of Organizational Resilience: Adaptive and Anchored Approaches. Corp. Commun. Int. J. 2018 , 23 , 180–196. [ Google Scholar ] [ CrossRef ]
- Sheffi, Y.; Rice, J.; James, A. Supply Chain View of the Resilient Enterprise. MIT Sloan Manag. Rev. 2005 , 47 , 41–48. Available online: https://sloanreview.mit.edu/article/a-supply-chain-view-of-the-resilient-enterprise/ (accessed on 4 September 2024).
- Ivanov, D.; Sokolov, B.; Dolgui, A. The Ripple Effect in Supply Chains: Trade-off ‘Efficiency-Flexibility-Resilience’ in Disruption Management. Int. J. Prod. Res. 2014 , 52 , 2154–2172. [ Google Scholar ] [ CrossRef ]
- De Treville, S.; Shapiro, R.D.; Hameri, A. From Supply Chain to Demand Chain: The Role of Lead Time Reduction in Improving Demand Chain Performance. J. Oper. Manag. 2004 , 21 , 613–627. [ Google Scholar ] [ CrossRef ]
- Vencataya, L.; Seebaluck, A.K.; Doorga, D. Assessing the Impact of Supply Chain Management on Competitive Advantage and Operational Performance: A Case of Four Star Hotels of Mauritius. Int. Rev. Manag. Mark. 2016 , 6 , 61–69. [ Google Scholar ]
- Meredith, S.; Francis, D. Journey towards Agility: The Agile Wheel Explored. TQM Mag. 2000 , 12 , 137–143. [ Google Scholar ] [ CrossRef ]
- Lengnick-Hall, C.A.; Beck, T.E. Resilience Capacity and Strategic Agility: Prerequisites for Thriving in a Dynamic Environment. In Resilience Engineering Perspectives, Volume 2 ; CRC Press: Boca Raton, FL, USA, 2009; ISBN 978-1-315-24438-9. [ Google Scholar ]
- Christopher, M.; Peck, H. Building the Resilient Supply Chain. Int. J. Logist. Manag. 2004 , 15 , 1–14. [ Google Scholar ] [ CrossRef ]
- Ayan, B.; Güner, E.; Son-Turan, S. Blockchain Technology and Sustainability in Supply Chains and a Closer Look at Different Industries: A Mixed Method Approach. Logistics 2022 , 6 , 85. [ Google Scholar ] [ CrossRef ]
- Alkhatib, S.F.; Momani, R.A. Supply Chain Resilience and Operational Performance: The Role of Digital Technologies in Jordanian Manufacturing Firms. Adm. Sci. 2023 , 13 , 40. [ Google Scholar ] [ CrossRef ]
- Kim, M.; Chai, S. The Impact of Supplier Innovativeness, Information Sharing and Strategic Sourcing on Improving Supply Chain Agility: Global Supply Chain Perspective. Int. J. Prod. Econ. 2017 , 187 , 42–52. [ Google Scholar ] [ CrossRef ]
- Ambulkar, S.; Blackhurst, J.; Grawe, S. Firm’s Resilience to Supply Chain Disruptions: Scale Development and Empirical Examination. J. Oper. Manag. 2015 , 33–34 , 111–122. [ Google Scholar ] [ CrossRef ]
- Powell, T.C.; Dent-Micallef, A. Information Technology as Competitive Advantage: The Role of Human, Business, and Technology Resources. Strateg. Manag. J. 1997 , 18 , 375–405. [ Google Scholar ] [ CrossRef ]
- Behr, D.; Sha, M. Introduction: Translation of Questionnaires in Cross-National and Cross-Cultural Research. Transl. Interpret. 2018 , 10 , 1–4. [ Google Scholar ] [ CrossRef ]
- Hair, J.; Anderson, R.; Black, B.; Babin, B. Multivariate Data Analysis ; Pearson Education: London, UK, 2016; ISBN 978-0-13-379268-3. [ Google Scholar ]
- Hayes, A.F. Introduction to Mediation, Moderation, and Conditional Process Analysis, Second Edition: A Regression-Based Approach , 2nd ed.; The Guilford Press: New York, NY, USA, 2017; ISBN 978-1-4625-3465-4. [ Google Scholar ]
- Walter, A.-T. Organizational Agility: Ill-Defined and Somewhat Confusing? A Systematic Literature Review and Conceptualization. Manag. Rev. Q. 2021 , 71 , 343–391. [ Google Scholar ] [ CrossRef ]
- Chisnall, P.M. Mail and Internet Surveys: The Tailored Design Method. J. Advert. Res. 2007 , 47 , 207–208. [ Google Scholar ] [ CrossRef ]
Click here to enlarge figure
Groups | N | % | |
---|---|---|---|
Gender | Male | 195 | 64 |
Female | 110 | 36 | |
Age | Less than 25 | 19 | 6.2 |
25–35 | 128 | 42 | |
36–45 | 116 | 38 | |
45+ | 42 | 13.8 | |
Education | High school | 24 | 7.9 |
Bachelor | 243 | 80 | |
Master | 38 | 12.5 | |
Sectoral history of the company | 1–5 years | 14 | 4.6 |
6–10 years | 68 | 22.2 | |
11–20 years | 149 | 49 | |
20 years+ | 74 | 24.2 | |
Time at work | 1–2 years | 68 | 22.2 |
3-5 years | 82 | 26.8 | |
6–9 years | 111 | 36.5 | |
10 years+ | 44 | 14.5 | |
Total work experience | 1–2 years | 28 | 9 |
3–5 years | 62 | 20.2 | |
6–9 years | 94 | 30.8 | |
10 years+ | 121 | 40 | |
TOTAL | 305 | 100 |
Item | Factor Loading | Mean | Std. Dev. | ||
---|---|---|---|---|---|
Supply Chain Management | ALL | ALL1 | 0.893 | 4.666 | 0.585 |
ALL2 | 0.916 | 4.656 | 0.598 | ||
ALL3 | 0.887 | 4.639 | 0.597 | ||
ALL4 | Removed due to low factor loading | ||||
CRM | CRM1 | 0.885 | 4.479 | 0.623 | |
CRM2 | 0.900 | 4.489 | 0.613 | ||
CRM3 | 0.914 | 4.475 | 0.618 | ||
CRM4 | 0.705 | 4.449 | 0.663 | ||
LOG | LOG1 | 0.906 | 4.226 | 0.511 | |
LOG2 | 0.878 | 4.216 | 0.518 | ||
LOG3 | 0.908 | 4.252 | 0.505 | ||
LOG4 | Removed due to low factor loading | ||||
Information Sharing | INF1 | 0.879 | 4.230 | 0.730 | |
INF2 | 0.920 | 4.249 | 0.732 | ||
INF3 | 0.920 | 4.243 | 0.726 | ||
INF4 | Removed due to low factor loading | ||||
Supply Chain Resilience | SCRES1 | 0.819 | 3.836 | 0.963 | |
SCRES2 | 0.869 | 3.534 | 1.016 | ||
SCRES3 | 0.829 | 3.666 | 0.977 | ||
SCRES4 | 0.766 | 3.633 | 0.954 | ||
Supply Chain Agility | SCA1 | Removed due to low factor loading | |||
SCA2 | 0.790 | 4.482 | 0.689 | ||
SCA3 | 0.707 | 4.498 | 0.669 | ||
SCA4 | 0.916 | 4.502 | 0.689 | ||
SCA5 | Removed due to low factor loading | ||||
SCA6 | 0.893 | 4.4918 | 0.703 | ||
SCA7 | Removed due to low factor loading | ||||
SCA8 | 0.877 | 4.4918 | 0.689 | ||
Financial Performance | FP1 | 0.834 | 4.410 | 0.643 | |
FP2 | 0.815 | 4.410 | 0.663 | ||
FP3 | 0.892 | 4.390 | 0.680 | ||
FP4 | Removed due to low factor loading | ||||
FP5 | 0.889 | 4.364 | 0.731 |
Variable | χ | df | χ /df | GFI | CFI | NFI | TLI | RMSEA |
---|---|---|---|---|---|---|---|---|
Criterion | ≤5 | ≥0.85 | ≥0.90 | ≥0.90 | ≥0.90 | ≤0.08 | ||
SCM | 161.318 | 56 | 2.881 | 0.924 | 0.965 | 0.948 | 0.951 | 0.079 |
SCRES | 0.50 | 1 | 0.50 | 1.000 | 1.000 | 1.000 | 1.011 | 0.000 |
SCA | 11.616 | 4 | 2.904 | 0.985 | 0.992 | 0.988 | 0.980 | 0.079 |
FP | 0.581 | 2 | 0.290 | 0.999 | 1.000 | 0.999 | 1.004 | 0.000 |
Variable | AVE | CR | Cronbach’s Alpha |
---|---|---|---|
SCM | 0.78 | 0.97 | 0.810 |
SCRES | 0.67 | 0.89 | 0.847 |
SCA | 0.71 | 0.92 | 0.912 |
FP | 0.78 | 0.93 | 0.918 |
R | R-sq | MSE | F | df1 | df2 | p |
---|---|---|---|---|---|---|
0.4052 | 0.1642 | 0.2545 | 8.3328 | 7 | 297 | 0.000 |
Coefficient | Std. Error | t-Value | p-Value | LLCI | ULCI | |
---|---|---|---|---|---|---|
Constant | −35.5105 | 14.2898 | −2.4850 | 0.0135 | −63.6326 | −7.3884 |
SCM | 37.5993 | 14.4203 | 2.6074 | 0.0096 | 9.2205 | 65.9782 |
SCA | 8.0088 | 3.2869 | 2.4366 | 0.0154 | 1.5403 | 14.4773 |
Int_1 | −7.5983 | 3.2861 | −2.3123 | 0.0214 | −14.0653 | −1.1314 |
SCRES | 9.7585 | 3.8571 | 2.5300 | 0.0119 | 2.1678 | 17.3492 |
Int_2 | −9.4181 | 3.9118 | −2.4076 | 0.0167 | −17.1165 | −1.7197 |
Int_3 | −2.1015 | 0.8910 | −2.3585 | 0.0190 | −3.8550 | −0.3480 |
Int_4 | 2.0026 | 0.8936 | 2.2410 | 0.0258 | 0.2440 | 3.7611 |
SCRES | Effect | F | df1 | df2 | p |
---|---|---|---|---|---|
2.3757 | −2.8409 | 5.5222 | 1 | 297 | 0.0194 |
3.205 | −1.1803 | 4.2911 | 1 | 297 | 0.0392 |
3.7815 | −0.0256 | 0.0036 | 1 | 297 | 0.952 |
SCA | SCRES | Effect | Std. Error | t-Value | p-Value | LLCI | ULCI |
---|---|---|---|---|---|---|---|
3.8714 | 2.3757 | 4.2267 | 0.9132 | 4.6285 | 0.0000 | 2.4296 | 6.0239 |
3.8714 | 3.2050 | 2.8456 | 0.5319 | 5.3496 | 0.0000 | 1.7988 | 3.8924 |
3.8714 | 3.7815 | 1.8854 | 0.5932 | 3.1782 | 0.0016 | 0.7179 | 3.0528 |
4.5522 | 2.3757 | 2.2925 | 0.7635 | 3.0027 | 0.0029 | 0.7900 | 3.7950 |
4.5522 | 3.2050 | 2.0421 | 0.5517 | 3.7011 | 0.0003 | 0.9562 | 3.1279 |
4.5522 | 3.7815 | 1.8679 | 0.6834 | 2.7333 | 0.0066 | 0.5230 | 3.2128 |
4.7945 | 2.3757 | 1.6041 | 0.9058 | 1.7708 | 0.0776 | −0.1786 | 3.3867 |
4.7945 | 3.2050 | 1.7560 | 0.6202 | 2.8313 | 0.0050 | 0.5355 | 2.9766 |
4.7945 | 3.7815 | 1.8617 | 0.7407 | 2.5135 | 0.0125 | 0.4041 | 3.3193 |
3.8714 | 3.2050 | 2.8456 | 0.5319 | 5.3496 | 0.0000 | 1.7988 | 3.8924 |
Interaction | Change in R-Square | F-Value | df1 | df2 | p-Value |
---|---|---|---|---|---|
SCM * SCA * SCRES | 0.0141 | 5.0221 | 1.0 | 297.0 | 0.0258 |
The statements, opinions and data contained in all publications are solely those of the individual author(s) and contributor(s) and not of MDPI and/or the editor(s). MDPI and/or the editor(s) disclaim responsibility for any injury to people or property resulting from any ideas, methods, instructions or products referred to in the content. |
Share and Cite
Tufan, C.; Çiğdem, Ş.; Kılıç, Y.; Sayar, G. Agility and Resilience in Supply Chains: Investigating Their Roles in Enhancing Financial Performance. Sustainability 2024 , 16 , 7842. https://doi.org/10.3390/su16177842
Tufan C, Çiğdem Ş, Kılıç Y, Sayar G. Agility and Resilience in Supply Chains: Investigating Their Roles in Enhancing Financial Performance. Sustainability . 2024; 16(17):7842. https://doi.org/10.3390/su16177842
Tufan, Cenk, Şemsettin Çiğdem, Yunus Kılıç, and Gökçen Sayar. 2024. "Agility and Resilience in Supply Chains: Investigating Their Roles in Enhancing Financial Performance" Sustainability 16, no. 17: 7842. https://doi.org/10.3390/su16177842
Article Metrics
Further information, mdpi initiatives, follow mdpi.
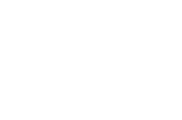
Subscribe to receive issue release notifications and newsletters from MDPI journals

IMAGES
VIDEO
COMMENTS
External validity is the extent to which you can generalize the findings of a study to other situations, people, settings, and measures. Learn about population validity and ecological validity, and how to avoid threats to external validity in your research design.
External validity is the extent to which study findings can be generalized to other populations, settings, or time periods. Learn how to enhance external validity by using representative sampling, diverse participants, multiple settings, and other strategies, and what threats to avoid.
External validity is the extent to which the results of a study can generalize or transport to other situations, people, stimuli, and times. Learn about the threats, methods, and applications of external validity in scientific research.
Learn how internal validity measures the trustworthiness of a study's cause-and-effect relationship, and how external validity measures the applicability of a study's findings to other settings. Find out the factors, threats, and examples of each type of validity in psychology research.
Internal validity refers to the degree of confidence that the causal relationship being tested is not influenced by other factors or variables. Learn about the threats to internal validity, the trade-off with external validity, and how to improve your research design.
External validity is the extent to which you can generalise the findings of a study to other situations, people, settings, and measures. Learn about the two types of external validity (population and ecological), the trade-off with internal validity, and the threats to external validity with examples.
External validity is how well the results of a study can be applied to people, places, or times outside of the study. Learn about the types of external validity, such as population, ecological, and temporal validity, and the research methods to improve them.
Internal, External, and Ecological Validity in Research ...
External validity is the degree to which experimental findings can be generalized to other populations, settings, treatments, measurements, times, and experimenters. Learn about the two types of external validity (population and ecological), the threats to external validity, and how to ensure external validity by replication.
Internal validity is the degree of confidence that a causal relationship is trustworthy and not influenced by other factors. Learn how to ensure internal validity, what threats to avoid, and how it differs from external validity.
The Importance of External Validity - PMC
Learn about the concept of validity in psychology research, which refers to the accuracy and reliability of tests and measurements. Explore different types of validity, such as internal, external, content, criterion, face, and construct validity, with examples and definitions.
Learn about the four main types of validity in research: construct, content, face and criterion. Criterion validity is the most difficult to establish in quantitative research, as it requires comparing the test results with external criteria.
External validity captures the extent to which inferences drawn from a given study's sample apply to a broader population or other target populations. Social scientists frequently invoke external validity as an ideal, but they rarely attempt to make rigorous, credible external validity inferences. In recent years, methodologically oriented scholars have advanced a flurry of work on various ...
Learn the definitions, examples, and threats of internal and external validity in psychological research. Internal validity tests causality, while external validity tests generalizability to other settings and populations.
Internal and external validity: can you apply research study ...
External validity captures the extent to which inferences drawn from a given study's sample apply to a broader population or other target populations. Social scientists frequently invoke external ...
External validity is the extent to which the results of a study can be generalized beyond the sample, population, or setting. Learn about the threats to external validity, such as sample accessibility, personological variables, ecological conditions, and measurement issues.
1 BACKGROUND. External validity is considered an important factor for decision making in health research. 1, 2 Although research on external validity has increased in the last decades, 1 there are still many shortcomings and methodological issues in this regard. 3, 4 Research has focused on examining various aspects of the internal validity (rather than external validity) of randomized ...
This article explains the concepts and methods of external validity, generalisability, applicability and directness in research. It also provides examples, definitions and tips for evaluating and improving the generalisability of research findings.
Learn how internal validity (cause-and-effect) and external validity (generalizability) are assessed in research methods. Find out what factors affect them, how to balance them, and why they matter for different types of studies.
A new population-oriented framework to conceptualize external validity of randomized controlled trials (RCTs) and evaluate their generalizability, reproducibility, and study quality. The framework identifies the population for which a trial's treatment effect will replicate and the criteria necessary for the true replication of experimental evidence.
For external validity, clinical trial participants should reflect the populations that will ultimately receive the interventions being tested, if proven effective. ... focusses on providing recommendations for recruitment strategy selection in future research with the aim to improve trial efficiency. A mixed-methods approach was employed ...
Factor analysis was employed to affirm the scale's validity, and the Hayes model 3 method was utilized to test hypotheses. ... chain management and financial performance if supply chain resilience enhances the resilience of organizations to external challenges. These insights suggest organizations must integrate agility, management, and ...