Have a language expert improve your writing
Run a free plagiarism check in 10 minutes, generate accurate citations for free.
- Knowledge Base
Methodology
- Random vs. Systematic Error | Definition & Examples

Random vs. Systematic Error | Definition & Examples
Published on May 7, 2021 by Pritha Bhandari . Revised on June 22, 2023.
In scientific research, measurement error is the difference between an observed value and the true value of something. It’s also called observation error or experimental error.
There are two main types of measurement error:
Random error is a chance difference between the observed and true values of something (e.g., a researcher misreading a weighing scale records an incorrect measurement).
- Systematic error is a consistent or proportional difference between the observed and true values of something (e.g., a miscalibrated scale consistently registers weights as higher than they actually are).
By recognizing the sources of error, you can reduce their impacts and record accurate and precise measurements. Gone unnoticed, these errors can lead to research biases like omitted variable bias or information bias .
Table of contents
Are random or systematic errors worse, random error, reducing random error, systematic error, reducing systematic error, other interesting articles, frequently asked questions about random and systematic error.
In research, systematic errors are generally a bigger problem than random errors.
Random error isn’t necessarily a mistake, but rather a natural part of measurement. There is always some variability in measurements, even when you measure the same thing repeatedly, because of fluctuations in the environment, the instrument, or your own interpretations.
But variability can be a problem when it affects your ability to draw valid conclusions about relationships between variables . This is more likely to occur as a result of systematic error.
Precision vs accuracy
Random error mainly affects precision , which is how reproducible the same measurement is under equivalent circumstances. In contrast, systematic error affects the accuracy of a measurement, or how close the observed value is to the true value.
Taking measurements is similar to hitting a central target on a dartboard. For accurate measurements, you aim to get your dart (your observations) as close to the target (the true values) as you possibly can. For precise measurements, you aim to get repeated observations as close to each other as possible.
Random error introduces variability between different measurements of the same thing, while systematic error skews your measurement away from the true value in a specific direction.
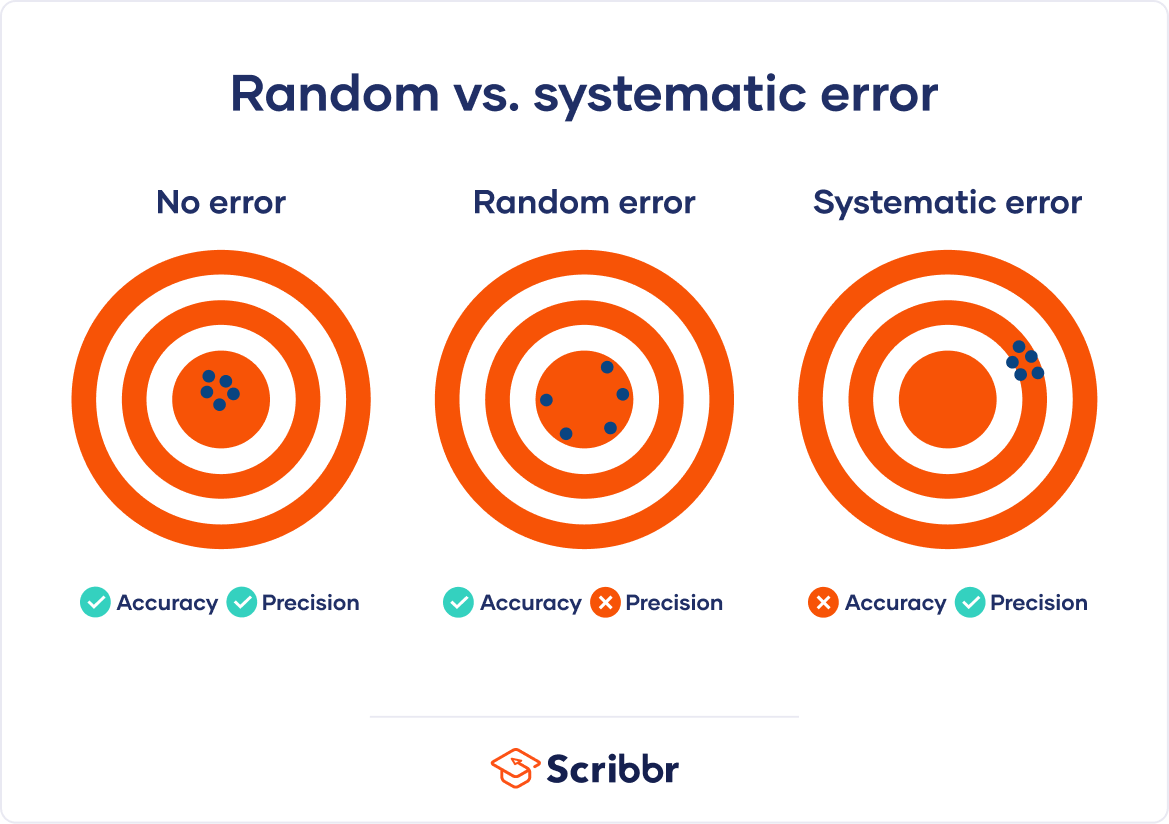
When you only have random error, if you measure the same thing multiple times, your measurements will tend to cluster or vary around the true value. Some values will be higher than the true score, while others will be lower. When you average out these measurements, you’ll get very close to the true score.
For this reason, random error isn’t considered a big problem when you’re collecting data from a large sample—the errors in different directions will cancel each other out when you calculate descriptive statistics . But it could affect the precision of your dataset when you have a small sample.
Systematic errors are much more problematic than random errors because they can skew your data to lead you to false conclusions. If you have systematic error, your measurements will be biased away from the true values. Ultimately, you might make a false positive or a false negative conclusion (a Type I or II error ) about the relationship between the variables you’re studying.
Receive feedback on language, structure, and formatting
Professional editors proofread and edit your paper by focusing on:
- Academic style
- Vague sentences
- Style consistency
See an example
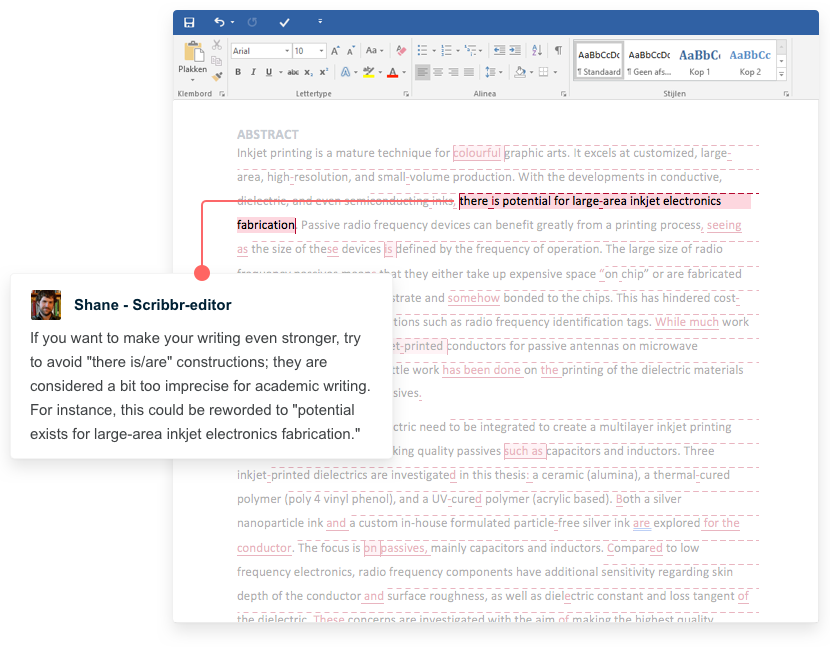
Random error affects your measurements in unpredictable ways: your measurements are equally likely to be higher or lower than the true values.
In the graph below, the black line represents a perfect match between the true scores and observed scores of a scale. In an ideal world, all of your data would fall on exactly that line. The green dots represent the actual observed scores for each measurement with random error added.
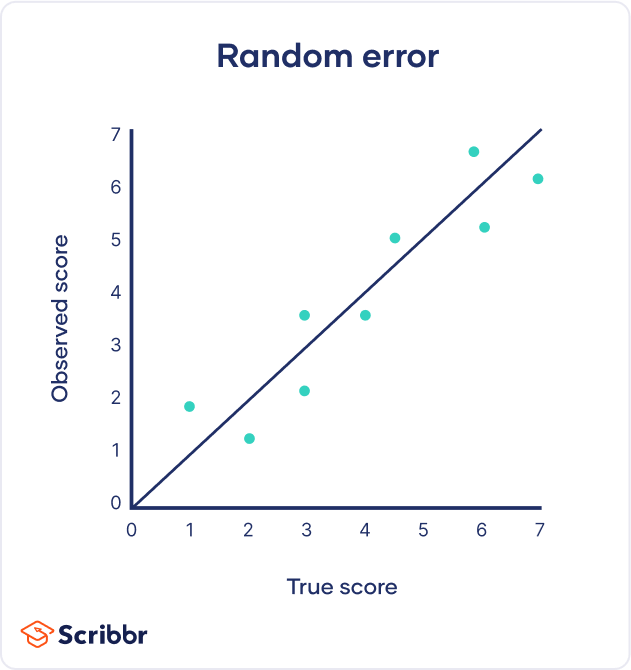
Random error is referred to as “noise”, because it blurs the true value (or the “signal”) of what’s being measured. Keeping random error low helps you collect precise data.
Sources of random errors
Some common sources of random error include:
- natural variations in real world or experimental contexts.
- imprecise or unreliable measurement instruments.
- individual differences between participants or units.
- poorly controlled experimental procedures.
Random error source | Example |
---|---|
Natural variations in context | In an about memory capacity, your participants are scheduled for memory tests at different times of day. However, some participants tend to perform better in the morning while others perform better later in the day, so your measurements do not reflect the true extent of memory capacity for each individual. |
Imprecise instrument | You measure wrist circumference using a tape measure. But your tape measure is only accurate to the nearest half-centimeter, so you round each measurement up or down when you record data. |
Individual differences | You ask participants to administer a safe electric shock to themselves and rate their pain level on a 7-point rating scale. Because pain is subjective, it’s hard to reliably measure. Some participants overstate their levels of pain, while others understate their levels of pain. |
Random error is almost always present in research, even in highly controlled settings. While you can’t eradicate it completely, you can reduce random error using the following methods.
Take repeated measurements
A simple way to increase precision is by taking repeated measurements and using their average. For example, you might measure the wrist circumference of a participant three times and get slightly different lengths each time. Taking the mean of the three measurements, instead of using just one, brings you much closer to the true value.
Increase your sample size
Large samples have less random error than small samples. That’s because the errors in different directions cancel each other out more efficiently when you have more data points. Collecting data from a large sample increases precision and statistical power .
Control variables
In controlled experiments , you should carefully control any extraneous variables that could impact your measurements. These should be controlled for all participants so that you remove key sources of random error across the board.
Systematic error means that your measurements of the same thing will vary in predictable ways: every measurement will differ from the true measurement in the same direction, and even by the same amount in some cases.
Systematic error is also referred to as bias because your data is skewed in standardized ways that hide the true values. This may lead to inaccurate conclusions.
Types of systematic errors
Offset errors and scale factor errors are two quantifiable types of systematic error.
An offset error occurs when a scale isn’t calibrated to a correct zero point. It’s also called an additive error or a zero-setting error.
A scale factor error is when measurements consistently differ from the true value proportionally (e.g., by 10%). It’s also referred to as a correlational systematic error or a multiplier error.
You can plot offset errors and scale factor errors in graphs to identify their differences. In the graphs below, the black line shows when your observed value is the exact true value, and there is no random error.
The blue line is an offset error: it shifts all of your observed values upwards or downwards by a fixed amount (here, it’s one additional unit).
The purple line is a scale factor error: all of your observed values are multiplied by a factor—all values are shifted in the same direction by the same proportion, but by different absolute amounts.
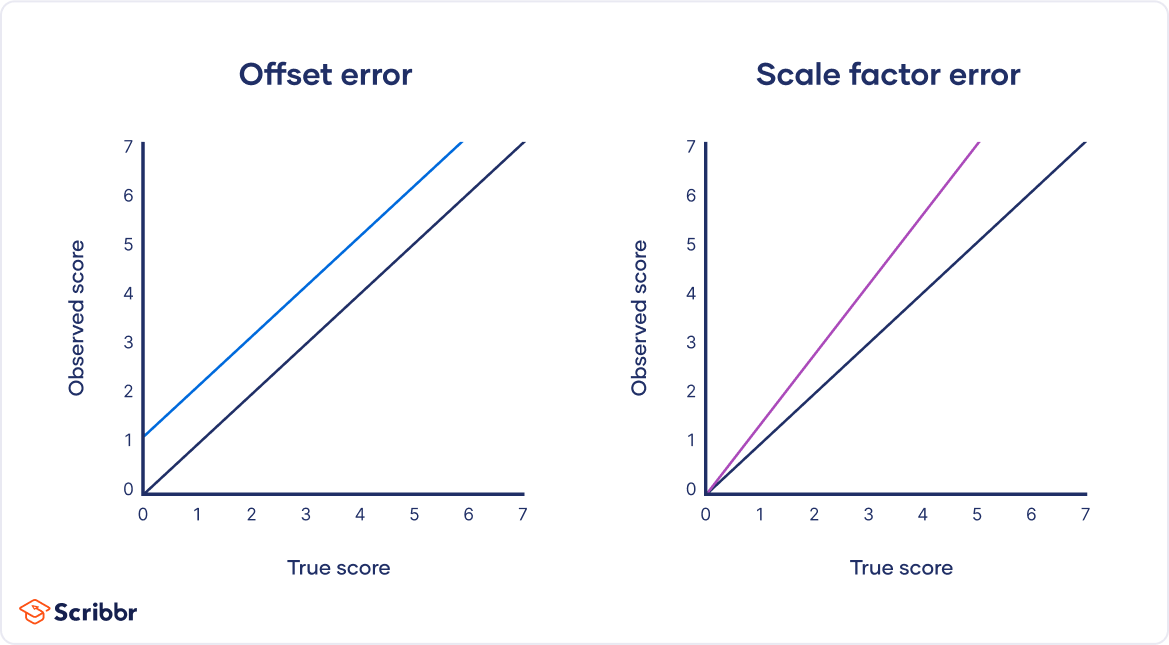
Sources of systematic errors
The sources of systematic error can range from your research materials to your data collection procedures and to your analysis techniques. This isn’t an exhaustive list of systematic error sources, because they can come from all aspects of research.
Response bias occurs when your research materials (e.g., questionnaires ) prompt participants to answer or act in inauthentic ways through leading questions . For example, social desirability bias can lead participants try to conform to societal norms, even if that’s not how they truly feel.
Your question states: “Experts believe that only systematic actions can reduce the effects of climate change. Do you agree that individual actions are pointless?”
Experimenter drift occurs when observers become fatigued, bored, or less motivated after long periods of data collection or coding, and they slowly depart from using standardized procedures in identifiable ways.
Initially, you code all subtle and obvious behaviors that fit your criteria as cooperative. But after spending days on this task, you only code extremely obviously helpful actions as cooperative.
Sampling bias occurs when some members of a population are more likely to be included in your study than others. It reduces the generalizability of your findings, because your sample isn’t representative of the whole population.
Prevent plagiarism. Run a free check.
You can reduce systematic errors by implementing these methods in your study.
Triangulation
Triangulation means using multiple techniques to record observations so that you’re not relying on only one instrument or method.
For example, if you’re measuring stress levels, you can use survey responses, physiological recordings, and reaction times as indicators. You can check whether all three of these measurements converge or overlap to make sure that your results don’t depend on the exact instrument used.
Regular calibration
Calibrating an instrument means comparing what the instrument records with the true value of a known, standard quantity. Regularly calibrating your instrument with an accurate reference helps reduce the likelihood of systematic errors affecting your study.
You can also calibrate observers or researchers in terms of how they code or record data. Use standard protocols and routine checks to avoid experimenter drift.
Randomization
Probability sampling methods help ensure that your sample doesn’t systematically differ from the population.
In addition, if you’re doing an experiment, use random assignment to place participants into different treatment conditions. This helps counter bias by balancing participant characteristics across groups.
Wherever possible, you should hide the condition assignment from participants and researchers through masking (blinding) .
Participants’ behaviors or responses can be influenced by experimenter expectancies and demand characteristics in the environment, so controlling these will help you reduce systematic bias.
If you want to know more about statistics , methodology , or research bias , make sure to check out some of our other articles with explanations and examples.
- Normal distribution
- Degrees of freedom
- Null hypothesis
- Discourse analysis
- Control groups
- Mixed methods research
- Non-probability sampling
- Quantitative research
- Ecological validity
Research bias
- Rosenthal effect
- Implicit bias
- Cognitive bias
- Selection bias
- Negativity bias
- Status quo bias
Random and systematic error are two types of measurement error.
Systematic error is a consistent or proportional difference between the observed and true values of something (e.g., a miscalibrated scale consistently records weights as higher than they actually are).
Systematic error is generally a bigger problem in research.
With random error, multiple measurements will tend to cluster around the true value. When you’re collecting data from a large sample , the errors in different directions will cancel each other out.
Systematic errors are much more problematic because they can skew your data away from the true value. This can lead you to false conclusions ( Type I and II errors ) about the relationship between the variables you’re studying.
Random error is almost always present in scientific studies, even in highly controlled settings. While you can’t eradicate it completely, you can reduce random error by taking repeated measurements, using a large sample, and controlling extraneous variables .
You can avoid systematic error through careful design of your sampling , data collection , and analysis procedures. For example, use triangulation to measure your variables using multiple methods; regularly calibrate instruments or procedures; use random sampling and random assignment ; and apply masking (blinding) where possible.
Cite this Scribbr article
If you want to cite this source, you can copy and paste the citation or click the “Cite this Scribbr article” button to automatically add the citation to our free Citation Generator.
Bhandari, P. (2023, June 22). Random vs. Systematic Error | Definition & Examples. Scribbr. Retrieved June 18, 2024, from https://www.scribbr.com/methodology/random-vs-systematic-error/
Is this article helpful?
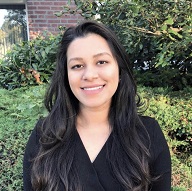
Pritha Bhandari
Other students also liked, reliability vs. validity in research | difference, types and examples, what is a controlled experiment | definitions & examples, extraneous variables | examples, types & controls, "i thought ai proofreading was useless but..".
I've been using Scribbr for years now and I know it's a service that won't disappoint. It does a good job spotting mistakes”
Random Error vs. Systematic Error
Two Types of Experimental Error
- Scientific Method
- Chemical Laws
- Periodic Table
- Projects & Experiments
- Biochemistry
- Physical Chemistry
- Medical Chemistry
- Chemistry In Everyday Life
- Famous Chemists
- Activities for Kids
- Abbreviations & Acronyms
- Weather & Climate
- Ph.D., Biomedical Sciences, University of Tennessee at Knoxville
- B.A., Physics and Mathematics, Hastings College
No matter how careful you are, there is always some error in a measurement. Error is not a "mistake"—it's part of the measuring process. In science, measurement error is called experimental error or observational error.
There are two broad classes of observational errors: random error and systematic error. Random error varies unpredictably from one measurement to another, while systematic error has the same value or proportion for every measurement. Random errors are unavoidable but cluster around the true value. Systematic error can often be avoided by calibrating equipment, but if left uncorrected, it can lead to measurements far from the true value.
Key Takeaways
- The two main types of measurement error are random error and systematic error.
- Random error causes one measurement to differ slightly from the next. It comes from unpredictable changes during an experiment.
- Systematic error always affects measurements by the same amount or proportion, provided that a reading is taken the same way each time. It is predictable.
- Random errors cannot be eliminated from an experiment, but most systematic errors may be reduced.
Systematic Error Examples and Causes
Systematic error is predictable and either constant or proportional to the measurement. Systematic errors primarily influence a measurement's accuracy .
What Causes Systematic Error?
Typical causes of systematic error include observational error, imperfect instrument calibration, and environmental interference. For example:
- Forgetting to tare or zero a balance produces mass measurements that are always "off" by the same amount. An error caused by not setting an instrument to zero prior to its use is called an offset error.
- Not reading the meniscus at eye level for a volume measurement will always result in an inaccurate reading. The value will be consistently low or high, depending on whether the reading is taken from above or below the mark.
- Measuring length with a metal ruler will give a different result at a cold temperature than at a hot temperature, due to thermal expansion of the material.
- An improperly calibrated thermometer may give accurate readings within a certain temperature range, but become inaccurate at higher or lower temperatures.
- Measured distance is different using a new cloth measuring tape versus an older, stretched one. Proportional errors of this type are called scale factor errors.
- Drift occurs when successive readings become consistently lower or higher over time. Electronic equipment tends to be susceptible to drift. Many other instruments are affected by (usually positive) drift, as the device warms up.
How Can You Avoid Systematic Error?
Once its cause is identified, systematic error may be reduced to an extent. Systematic error can be minimized by routinely calibrating equipment, using controls in experiments, warming up instruments before taking readings, and comparing values against standards .
While random errors can be minimized by increasing sample size and averaging data, it's harder to compensate for systematic error. The best way to avoid systematic error is to be familiar with the limitations of instruments and experienced with their correct use.
Random Error Examples and Causes
If you take multiple measurements , the values cluster around the true value. Thus, random error primarily affects precision . Typically, random error affects the last significant digit of a measurement.
What Causes Random Error?
The main reasons for random error are limitations of instruments, environmental factors, and slight variations in procedure. For example:
- When weighing yourself on a scale, you position yourself slightly differently each time.
- When taking a volume reading in a flask, you may read the value from a different angle each time.
- Measuring the mass of a sample on an analytical balance may produce different values as air currents affect the balance or as water enters and leaves the specimen.
- Measuring your height is affected by minor posture changes.
- Measuring wind velocity depends on the height and time at which a measurement is taken. Multiple readings must be taken and averaged because gusts and changes in direction affect the value.
- Readings must be estimated when they fall between marks on a scale or when the thickness of a measurement marking is taken into account.
How Can You Avoid (or Minimize) Random Error?
Because random error always occurs and cannot be predicted , it's important to take multiple data points and average them to get a sense of the amount of variation and estimate the true value. Statistical techniques such as standard deviation can further shed light on the extent of variability within a dataset.
Cochran, W. G. (1968). "Errors of Measurement in Statistics". Technometrics. Taylor & Francis, Ltd. on behalf of American Statistical Association and American Society for Quality. 10: 637–666. doi:10.2307/1267450
Bland, J. Martin, and Douglas G. Altman (1996). "Statistics Notes: Measurement Error." BMJ 313.7059: 744.
Taylor, J. R. (1999). An Introduction to Error Analysis: The Study of Uncertainties in Physical Measurements. University Science Books. p. 94. ISBN 0-935702-75-X.
- How to Calculate Percent Error
- Difference Between Independent and Dependent Variables
- How to Use a Periodic Table of Elements
- Examples of Independent and Dependent Variables
- What Is the Difference Between Accuracy and Precision?
- Absolute Error or Absolute Uncertainty Definition
- Measurement Definition in Science
- The Relative Uncertainty Formula and How to Calculate It
- Sampling Error
- Scientific Method Vocabulary Terms
- Accuracy Definition in Science
- Absolute and Relative Error Calculation
- How to Measure Mass Using a Balance
- Null Hypothesis Definition and Examples
- Relative Error Definition (Science)
- Hydrometer Definition in Science
Systematic Error / Random Error: Definition and Examples
What is systematic error.
Systematic error (also called systematic bias ) is consistent , repeatable error associated with faulty equipment or a flawed experiment design.
What is Random Error?
Random error (also called unsystematic error, system noise or random variation) has no pattern. One minute your readings might be too small. The next they might be too large. You can’t predict random error and these errors are usually unavoidable.
Systematic vs. Random Errors
Systematic errors are usually caused by measuring instruments that are incorrectly calibrated or are used incorrectly. However, they can creep into your experiment from many sources, including:
- A worn out instrument . For example, a plastic tape measure becomes slightly stretched over the years, resulting in measurements that are slightly too high,
- An incorrectly calibrated or tared instrument, like a scale that doesn’t read zero when nothing is on it,
- A person consistently takes an incorrect measurement. For example, they might think the 3/4″ mark on a ruler is the 2/3″ mark.
The main differences between these two error types are:
- Random errors are (like the name suggests) completely random. They are unpredictable and can’t be replicated by repeating the experiment again.
- Systematic Errors produce consistent errors , either a fixed amount (like 1 lb) or a proportion (like 105% of the true value). If you repeat the experiment, you’ll get the same error .
Systematic errors are consistently in the same direction (e.g. they are always 50 g, 1% or 99 mm too large or too small). In contrast, random errors produce different values in random directions. For example, you use a scale to weigh yourself and get 148 lbs, 153 lbs, and 132 lbs.
Types of Systematic Error
1. Offset Error is a type of systematic error where the instrument isn’t set to zero when you start to weigh items. For example, a kitchen scale includes a “tare” button, which sets the scale and a container to zero before contents are placed in the container. This is so the weight of the container isn’t included in the readings. If the tare isn’t set properly, all readings will have offset error.
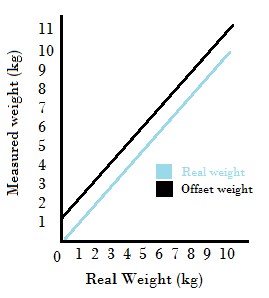
2. Scale Factor Errors. These are errors that are proportional to the true measurement. For example, a measuring tape stretched to 101% of its original size will consistently give results that are 101% of the true value.
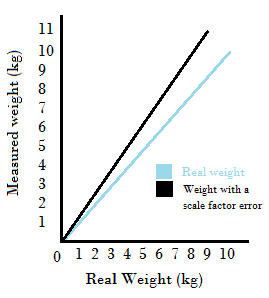
Compare the above two error patterns with random errors , which have no pattern:
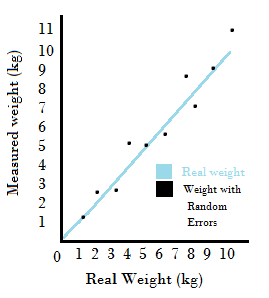
Preventing Errors
Random error can be reduced by:
- Using an average measurement from a set of measurements, or
- Increasing sample size.
It’s difficult to detect — and therefore prevent — systematic error. In order to avoid these types of error, know the limitations of your equipment and understand how the experiment works. This can help you identify areas that may be prone to systematic errors.
Gonick, L. (1993). The Cartoon Guide to Statistics . HarperPerennial. Everitt, B. S.; Skrondal, A. (2010), The Cambridge Dictionary of Statistics , Cambridge University Press. Kotz, S.; et al., eds. (2006), Encyclopedia of Statistical Sciences , Wiley.

- Science Notes Posts
- Contact Science Notes
- Todd Helmenstine Biography
- Anne Helmenstine Biography
- Free Printable Periodic Tables (PDF and PNG)
- Periodic Table Wallpapers
- Interactive Periodic Table
- Periodic Table Posters
- Science Experiments for Kids
- How to Grow Crystals
- Chemistry Projects
- Fire and Flames Projects
- Holiday Science
- Chemistry Problems With Answers
- Physics Problems
- Unit Conversion Example Problems
- Chemistry Worksheets
- Biology Worksheets
- Periodic Table Worksheets
- Physical Science Worksheets
- Science Lab Worksheets
- My Amazon Books
Sources of Error in Science Experiments

Science labs usually ask you to compare your results against theoretical or known values. This helps you evaluate your results and compare them against other people’s values. The difference between your results and the expected or theoretical results is called error. The amount of error that is acceptable depends on the experiment, but a margin of error of 10% is generally considered acceptable. If there is a large margin of error, you’ll be asked to go over your procedure and identify any mistakes you may have made or places where error might have been introduced. So, you need to know the different types and sources of error and how to calculate them.
How to Calculate Absolute Error
One method of measuring error is by calculating absolute error , which is also called absolute uncertainty. This measure of accuracy is reported using the units of measurement. Absolute error is simply the difference between the measured value and either the true value or the average value of the data.
absolute error = measured value – true value
For example, if you measure gravity to be 9.6 m/s 2 and the true value is 9.8 m/s 2 , then the absolute error of the measurement is 0.2 m/s 2 . You could report the error with a sign, so the absolute error in this example could be -0.2 m/s 2 .
If you measure the length of a sample three times and get 1.1 cm, 1.5 cm, and 1.3 cm, then the absolute error is +/- 0.2 cm or you would say the length of the sample is 1.3 cm (the average) +/- 0.2 cm.
Some people consider absolute error to be a measure of how accurate your measuring instrument is. If you are using a ruler that reports length to the nearest millimeter, you might say the absolute error of any measurement taken with that ruler is to the nearest 1 mm or (if you feel confident you can see between one mark and the next) to the nearest 0.5 mm.
How to Calculate Relative Error
Relative error is based on the absolute error value. It compares how large the error is to the magnitude of the measurement. So, an error of 0.1 kg might be insignificant when weighing a person, but pretty terrible when weighing a apple. Relative error is a fraction, decimal value, or percent.
Relative Error = Absolute Error / Total Value
For example, if your speedometer says you are going 55 mph, when you’re really going 58 mph, the absolute error is 3 mph / 58 mph or 0.05, which you could multiple by 100% to give 5%. Relative error may be reported with a sign. In this case, the speedometer is off by -5% because the recorded value is lower than the true value.
Because the absolute error definition is ambiguous, most lab reports ask for percent error or percent difference.
How to Calculate Percent Error
The most common error calculation is percent error , which is used when comparing your results against a known, theoretical, or accepted value. As you probably guess from the name, percent error is expressed as a percentage. It is the absolute (no negative sign) difference between your value and the accepted value, divided by the accepted value, multiplied by 100% to give the percent:
% error = [accepted – experimental ] / accepted x 100%
How to Calculate Percent Difference
Another common error calculation is called percent difference . It is used when you are comparing one experimental result to another. In this case, no result is necessarily better than another, so the percent difference is the absolute value (no negative sign) of the difference between the values, divided by the average of the two numbers, multiplied by 100% to give a percentage:
% difference = [experimental value – other value] / average x 100%
Sources and Types of Error
Every experimental measurement, no matter how carefully you take it, contains some amount of uncertainty or error. You are measuring against a standard, using an instrument that can never perfectly duplicate the standard, plus you’re human, so you might introduce errors based on your technique. The three main categories of errors are systematic errors, random errors , and personal errors. Here’s what these types of errors are and common examples.
Systematic Errors
Systematic error affects all the measurements you take. All of these errors will be in the same direction (greater than or less than the true value) and you can’t compensate for them by taking additional data. Examples of Systematic Errors
- If you forget to calibrate a balance or you’re off a bit in the calibration, all mass measurements will be high/low by the same amount. Some instruments require periodic calibration throughout the course of an experiment , so it’s good to make a note in your lab notebook to see whether the calibrations appears to have affected the data.
- Another example is measuring volume by reading a meniscus (parallax). You likely read a meniscus exactly the same way each time, but it’s never perfectly correct. Another person taking the reading may take the same reading, but view the meniscus from a different angle, thus getting a different result. Parallax can occur in other types of optical measurements, such as those taken with a microscope or telescope.
- Instrument drift is a common source of error when using electronic instruments. As the instruments warm up, the measurements may change. Other common systematic errors include hysteresis or lag time, either relating to instrument response to a change in conditions or relating to fluctuations in an instrument that hasn’t reached equilibrium. Note some of these systematic errors are progressive, so data becomes better (or worse) over time, so it’s hard to compare data points taken at the beginning of an experiment with those taken at the end. This is why it’s a good idea to record data sequentially, so you can spot gradual trends if they occur. This is also why it’s good to take data starting with different specimens each time (if applicable), rather than always following the same sequence.
- Not accounting for a variable that turns out to be important is usually a systematic error, although it could be a random error or a confounding variable. If you find an influencing factor, it’s worth noting in a report and may lead to further experimentation after isolating and controlling this variable.
Random Errors
Random errors are due to fluctuations in the experimental or measurement conditions. Usually these errors are small. Taking more data tends to reduce the effect of random errors. Examples of Random Errors
- If your experiment requires stable conditions, but a large group of people stomp through the room during one data set, random error will be introduced. Drafts, temperature changes, light/dark differences, and electrical or magnetic noise are all examples of environmental factors that can introduce random errors.
- Physical errors may also occur, since a sample is never completely homogeneous. For this reason, it’s best to test using different locations of a sample or take multiple measurements to reduce the amount of error.
- Instrument resolution is also considered a type of random error because the measurement is equally likely higher or lower than the true value. An example of a resolution error is taking volume measurements with a beaker as opposed to a graduated cylinder. The beaker will have a greater amount of error than the cylinder.
- Incomplete definition can be a systematic or random error, depending on the circumstances. What incomplete definition means is that it can be hard for two people to define the point at which the measurement is complete. For example, if you’re measuring length with an elastic string, you’ll need to decide with your peers when the string is tight enough without stretching it. During a titration, if you’re looking for a color change, it can be hard to tell when it actually occurs.
Personal Errors
When writing a lab report, you shouldn’t cite “human error” as a source of error. Rather, you should attempt to identify a specific mistake or problem. One common personal error is going into an experiment with a bias about whether a hypothesis will be supported or rejects. Another common personal error is lack of experience with a piece of equipment, where your measurements may become more accurate and reliable after you know what you’re doing. Another type of personal error is a simple mistake, where you might have used an incorrect quantity of a chemical, timed an experiment inconsistently, or skipped a step in a protocol.
Related Posts
- Skip to secondary menu
- Skip to main content
- Skip to primary sidebar
Statistics By Jim
Making statistics intuitive
Random Error vs Systematic Error
By Jim Frost Leave a Comment
Random error and systematic error are the two main types of measurement error. Measurement error occurs when the measured value differs from the true value of the quantity being measured.
Even when you try your best, you can never measure something perfectly—it’s normal when you measure something. In science, we call this measurement error. There will always be a little uncertainty in our measurements. It’s not that we did something wrong; it’s an inherent part of measuring things. Statisticians also refer to it as experimental error or observational error.
There are two types of measurement error:
- Random error occurs due to chance. Even if we do everything correctly for each measurement, we’ll get slightly different results when measuring the same item multiple times.
- Systematic error is when the measurement system makes the same kind of mistake every time it measures something. Often, that happens because of a problem with the tool we’re using or the way we’re doing the experiment. For example, a caliper might be miscalibrated and always show larger widths than they are.
Researchers must assess measurement error in scientific studies because too much of it reduces the validity and reliability of their experiment.
In this post, I’ll explain the differences between random vs systematic error, provide examples, and explore how they occur and ways to reduce them.
Random Error
Random error is a type of measurement error that is caused by the natural variability in the measurement process. It is unpredictable and occurs equally in both directions (e.g., too high and too low) relative to the correct value. It is usually caused by factors such as limitations in the measuring instrument, fluctuations in environmental conditions, and slight procedural variations.
Statisticians often refer to random error as “noise” because it can interfere with the true value (or “signal”) of what you’re trying to measure. If you can keep the random error low, you can collect more precise data.
For example, imagine you want to measure the height of a tree using a measuring tape. The tree’s height is 10 feet, but due to variations in the measuring tape, the angle you look at the tape, the sun in your eyes, the wind blowing the tape, etc., you get slightly different measurements each time you measure it. The first measurement is 10.2 feet, the second is 9.9 feet, and the third is 10.1 feet. These differences are due to random error.
Unlike systematic error, we can estimate and reduce random error using statistics to analyze repeated measurements. To do this, use the same measurement device and measure the same object at least ten times. Then find the average and the standard deviation. Although there are several ways to report the random error, a standard method is to write the mean plus or minus two times the standard deviation .
To see how random error affects a measurement system’s precision, you can perform a Gage R&R study .
Let’s return to the tree height example to illustrate random error. 10 is the correct height value for this tree.

This graph shows how the measurements randomly cluster around the true value of 10. They have no pattern. The red diamond is the average of the 30 data points, and it is pretty close to the correct value because the positive and negative errors cancel each other out.
Random error primarily affects precision, which is the degree to which repeated measurements of the same thing under similar conditions produce the same result. Additionally, random error mainly affects Reliability in an Experiment . Learn more about Accuracy vs. Precision .
Reducing Random Error
Random error is unavoidable in research, even if you try to control everything perfectly. However, there are simple ways to reduce it, such as:
- Take repeated measurements : If you take multiple measurements of the same thing, you can average them together to get a more precise result.
- Increase your sample size : The more data points you have, the less random error will affect your results. That’s why larger sample sizes are generally better than smaller ones regarding precision and statistical power.
- Increase the precision of measuring instruments : Use more precise instruments or calibrate them regularly.
- Control other variables : In controlled experiments, keep everything as consistent as possible so that extraneous factors don’t introduce random error into your measurements. By controlling all relevant variables, you can minimize sources of error and get more accurate results.
Taking the average of multiple measurements reduces the random error by canceling out the positive and negative errors. This property is a form of the law of large numbers. Learn more about the Law of Large Numbers .
For example, averaging our multiple tree measurements produced a mean close to the correct value. For additional improvements, researchers can measure the tree during calm and stable meteorological conditions to reduce distracting factors. And they can use a more precise measuring tape with finer units marked out. They might even use a specialized rig to hold and measure trees if they need high precision.
Systematic Error
Systematic error is a measurement error that occurs consistently in the same direction. It can be a constant difference or one that varies in a relationship with the actual value of the measurement. Statisticians refer to the former as an offset error and the latter as a scale factor error. In either case, there is a persistent factor that predictably affects all measurements. Systematic errors create bias in your data.
Many factors can cause systematic error, including errors in the measurement instrument calibration, a bias in the measurement process, or external factors that influence the measurement process in a consistent non-random manner.
For example, imagine you want to weigh objects in an experiment. Unfortunately, the scale has a calibration error. It always shows the weight to be 1 kilogram heavier than the true weight. Alternatively, the scale might consistently add a percentage to the correct value. Either way, this difference between the actual and measured values is systematically wrong.
That’s a simple example but imagine more complex scenarios.
A survey might have a systematic error due to a cognitive bias , such as the framing effect , where the wording unduly influences the participants. Perhaps the survey’s language is unintentionally prejudicial in some manner, causing people to react more negatively to survey items than they really feel.
In other cases, the expectations of the measurer and the subject can influence the measurements!
Let’s return to the tree example to illustrate systematic error.

In this graph, the data points are systematically too high relative to the true value of 10. They cluster around the wrong value. For any given measurement, you can predict that the error will be positive, making them non-random. Furthermore, unlike the random error graph, the mean is also wrong for these data. Because the errors are all positive, averaging them doesn’t cancel them out. As an aside, the range of values in this example looks much smaller compared to the previous graph, but that’s only due to the graph scaling .
Systematic error mainly affects accuracy, which is how close the average of a set of measurements is to the correct value. It also affects validity in research because the instrument isn’t measuring what you think it is measuring.
Reducing Systematic Error
To reduce systematic errors, you can use the following methods in your study:
- Triangulation : use multiple techniques to record observations so you’re not relying on only one instrument or method.
- Regular calibration : frequently comparing what the instrument records with the value of a known, standard quantity reduces the likelihood of systematic errors affecting your study.
- Blinding : hiding the condition assignment from participants and researchers helps reduce systematic bias caused by experimenter expectancies and cues in an experimental situation that might influence participants to behave in a certain way or provide specific responses, even if these responses do not reflect their true thoughts or behaviors.
Unfortunately, there are many possible sources of systematic error, each requiring a unique solution. So, a comprehensive list is impossible. Some instances will require a lot of investigation. More on that in the next section!
Random Error vs Systematic Error: Which is Worse?
Both types can be problematic, but systematic error is generally considered to be worse than random error. Systematic error affects all measurements consistently in the same direction, leading to biased results. Random error, on the other hand, affects measurements in different directions, canceling out the errors in the long run.
Systematic error is tricky to figure out and fix. Even if you take many measurements and average them, the error remains. Unlike random error, averaging and larger sample sizes don’t reduce systematic error. You can’t use math to eliminate systematic error or even know it’s there. To minimize systematic error, you can try doing these things:
- Look carefully at the way you’re doing the experiment and try to figure out what might be causing the error. Then, change the procedure or conditions to fix it.
- Compare your results to studies using different equipment or methods. If their results differ from yours, it could signal systematic error in your experiment.
- Try using a known value to check your measurements. This process is called calibration.

Share this:

Reader Interactions
Comments and questions cancel reply.

- school Campus Bookshelves
- menu_book Bookshelves
- perm_media Learning Objects
- login Login
- how_to_reg Request Instructor Account
- hub Instructor Commons
Margin Size
- Download Page (PDF)
- Download Full Book (PDF)
- Periodic Table
- Physics Constants
- Scientific Calculator
- Reference & Cite
- Tools expand_more
- Readability
selected template will load here
This action is not available.
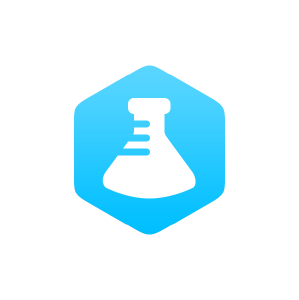
Appendix A: Treatment of Experimental Errors
- Last updated
- Save as PDF
- Page ID 435125
\( \newcommand{\vecs}[1]{\overset { \scriptstyle \rightharpoonup} {\mathbf{#1}} } \)
\( \newcommand{\vecd}[1]{\overset{-\!-\!\rightharpoonup}{\vphantom{a}\smash {#1}}} \)
\( \newcommand{\id}{\mathrm{id}}\) \( \newcommand{\Span}{\mathrm{span}}\)
( \newcommand{\kernel}{\mathrm{null}\,}\) \( \newcommand{\range}{\mathrm{range}\,}\)
\( \newcommand{\RealPart}{\mathrm{Re}}\) \( \newcommand{\ImaginaryPart}{\mathrm{Im}}\)
\( \newcommand{\Argument}{\mathrm{Arg}}\) \( \newcommand{\norm}[1]{\| #1 \|}\)
\( \newcommand{\inner}[2]{\langle #1, #2 \rangle}\)
\( \newcommand{\Span}{\mathrm{span}}\)
\( \newcommand{\id}{\mathrm{id}}\)
\( \newcommand{\kernel}{\mathrm{null}\,}\)
\( \newcommand{\range}{\mathrm{range}\,}\)
\( \newcommand{\RealPart}{\mathrm{Re}}\)
\( \newcommand{\ImaginaryPart}{\mathrm{Im}}\)
\( \newcommand{\Argument}{\mathrm{Arg}}\)
\( \newcommand{\norm}[1]{\| #1 \|}\)
\( \newcommand{\Span}{\mathrm{span}}\) \( \newcommand{\AA}{\unicode[.8,0]{x212B}}\)
\( \newcommand{\vectorA}[1]{\vec{#1}} % arrow\)
\( \newcommand{\vectorAt}[1]{\vec{\text{#1}}} % arrow\)
\( \newcommand{\vectorB}[1]{\overset { \scriptstyle \rightharpoonup} {\mathbf{#1}} } \)
\( \newcommand{\vectorC}[1]{\textbf{#1}} \)
\( \newcommand{\vectorD}[1]{\overrightarrow{#1}} \)
\( \newcommand{\vectorDt}[1]{\overrightarrow{\text{#1}}} \)
\( \newcommand{\vectE}[1]{\overset{-\!-\!\rightharpoonup}{\vphantom{a}\smash{\mathbf {#1}}}} \)
Every measurement that is made in the laboratory is subject to error. An experimenter should try to minimize these errors. However, since they cannot be entirely eliminated, a means to describe and quantify the errors is needed, so that another experimenter can judge and interpret the uncertainties reported with any result. This outline defines certain terms that are important in the treatment of errors. Certain conclusions that are derived from a statistical analysis of random errors are also presented. Finally, some rules are given for discarding questionable data, for propagating errors in calculations, and for finding the best straight line through a set of graphed data.
Types of Error
There are two general classes of errors. Systematic or determinate errors are reproducible in successive measurements and may be detected and corrected. Often systematic error is due to an incorrect calibration, for example of volumetric glassware, an electronic balance or a pH meter, and causes all readings to have the same recurring error. Random or indeterminate errors are due to limitations of the measurement that are beyond the experimenter's control. They cannot be eliminated, and lead to positive and negative fluctuations in successive measurements. Examples of random errors are the fluctuations in the interpolation of the reading of a thermometer scale by different observers and the oscillations in the output of a pH meter due to electrical noise.
Accuracy and Precision
The accuracy of a result refers to how close the result is to its true value, while the precision of a result indicates its reproducibility in successive measurements. If successive measurements are in close agreement, the result has a high precision, which usually implies small random errors. If the result is in good agreement with the true value, it has high accuracy, which suggests small systematic errors.
A well-designed experiment attempts to minimize both systematic and random errors, thereby allowing both high accuracy and high precision from careful measurements. Since systematic errors are not generally manifest in successive measurements, they can be avoided only by careful calibration and consideration of all possible corrections. Random errors are indicated by the fluctuations in successive measurements. They can be quantified and treated by the methods of statistics. In the following we restrict the discussion to random errors, assuming that all systematic errors have been eliminated.
Statistical Treatment of Random Errors
Let's consider as an example the volume of water delivered by a set of 25 mL pipets. A manufacturer produces these to deliver 25.00 mL at 20°C with a stated tolerance of ±0.03 mL. A sample of 100 pipets is tested for accuracy by measuring the delivered volumes. The column graph in Figure 1 shows the fractional number of the sampled pipets that deliver a particular volume in each 0.01 mL interval. The maximum of the column graph indicates that most of the pipets deliver between 24.995 and 25.005 mL. However, other pipets deliver lesser or greater volumes due to random variations in the manufacturing process.
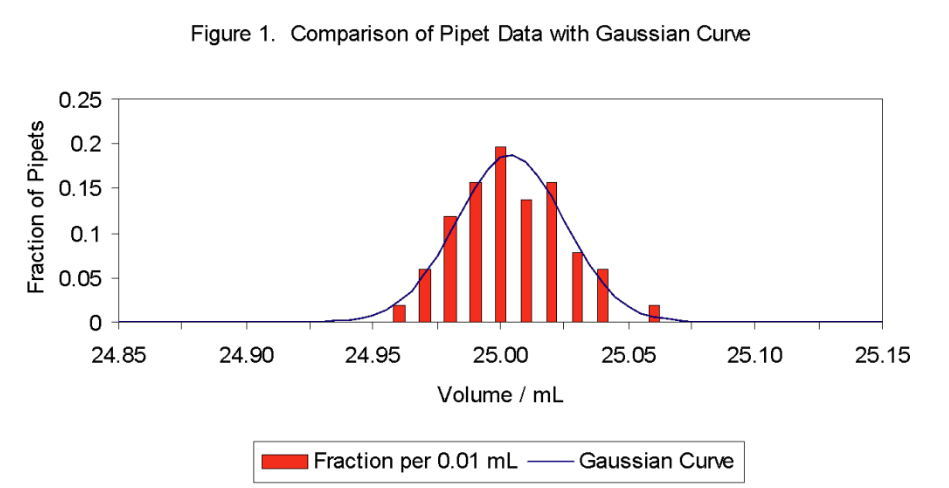
The mean or average, \( \ce{x_{avg}}\), of a set of results is defined by
\( \ce{x_{avg} = \frac{\sum_{i}x_{i}}{N} } \)
where \( \ce{x_{i}}\) is an individual result and N is the total number of results. For the data in Figure 1, the mean volume is 25.0039 mL. The mean alone, however, provides no indication of the uncertainty. The same mean could have resulted from data with either more or less spread. The spread of the individual results about the mean is characterized by the sample standard deviation , s , which is given by
\( \ce{ s = ( \frac{\sum_{i} (x_{i}-x_{avg})^{2}}{N-1} )^{1/2} } \)
The pipet data has a sample standard deviation of 0.0213 mL.
The sample standard deviation gives us a quantitative measurement of the precision. To see how this works, let's imagine that we increase the number of sampled pipets. The bar graph will show less irregularities. A line connecting the tops of the bars will approach a smooth bell-shaped curve as the number of samples approaches infinity and the volume interval approaches zero. This smooth curve is called a Gaussian or normal error curve. Its formula is
\( \ce{ y(x) = \frac {1}{\sigma(2\pi)^{1/2}} exp [-\frac{(x-\mu)^{2}}{2\sigma^{2}} ] } \)
where exp[···] = e [···] with e = 2.178..., the base of natural logarithms. For an infinite or complete data set, the mean is called \( \ce{\mu}\) (the population mean) and the standard deviation \( \ce{\sigma}\) (the population standard deviation). We can never measure \( \ce{\mu}\) and \( \ce{\sigma}\), but \( \ce{x_{avg}}\) and \( \ce{s}\) approach \( \ce{\mu}\) and \( \ce{\sigma}\), respectively, as the number of samples or measurements increases. The smooth curve in Figure 1 shows a graph of equation (3) for \( \ce{\mu}\) = 25.0039 mL and \( \ce{\sigma}\) = 0.0213 mL, as approximated by \( \ce{x_{avg}}\) and \( \ce{s}\).
The Gaussian curve gives the probability of obtaining a particular result \( \ce{x}\) for a given \( \ce{\mu}\) and \( \ce{\sigma}\). This probability is proportional to the \( \ce{y}\) value of the Gaussian curve for the particular \( \ce{x}\) value. The maximum probability occurs at the maximum of the function, which corresponds to \( \ce{x=\mu}\). Other values of \( \ce{x}\) have lower probabilities. Because of the symmetry of the curve, values of \( \ce{x}\) that deviate from \( \ce{\mu}\) by the same magnitude, i.e. , have the same \( \ce{|(x-\mu)|}\), have the same probabilities.
The significance of the standard deviation is that it measures the width of the Gaussian curve. The larger the value of \( \ce{\sigma}\), the broader the curve and the greater the probability of obtaining an \( \ce{x}\) value that deviates from \( \ce{\mu}\). One can calculate the percent of the samples or measurements that occurs in a given range from the corresponding fraction of the area under the Gaussian curve by integral calculus. Representative results are summarized in Table 1. For example, 68.3% of the samples or measurements are expected to lie between \( \ce{\mu-\sigma}\) and \( \ce{\mu+\sigma}\), and 90.0% between \( \ce{\mu-1.645\sigma}\) and \( \ce{\mu+1.645\sigma}\). Table 1. Area under Gaussian Curve
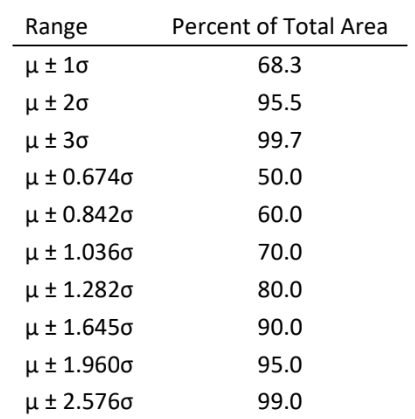
The manufacturer can thus have 90.0% confidence that some other pipet (from the identical manufacturing process) will deliver a volume in the range \( \ce{\mu \pm 1.645 \sigma} \). Estimating \( \ce {\mu = x_{avg} =} \)25.004 mL and \( \ce{\sigma = s =}\) 0.0213 mL, the manufacturer can claim that the pipets deliver 25.00 mL with a tolerance of (1.64)(0.021) = 0.03 mL. The 90% degree of confidence is hidden in this claim, and a higher degree of confidence would have correspondingly poorer (greater) tolerances. The purchaser, however, may not be told these details!
Confidence Limits
Although we cannot determine \(\ce{\mu}\) and \(\ce{\sigma}\) from a limited number of measurements, statistical analysis does allow us to obtain the confidence limits for \(\ce{\mu}\) from a limited data set. Namely, we can state to a certain probability (confidence) that \(\ce{\mu}\) will lie within certain limits of \(\ce{x_{avg}}\). These confidence limits (CL) are given by
\( \ce{CL = \pm \frac{ts}{\sqrt{N}}} \)
where \(\ce{s}\) is the sample standard deviation and N is the number of measurements. The \( \ce{\sqrt{N}} \) term in the denominator accounts for the fact that the sample mean has a greater precision than any individual measurement. 1* The factor t (called Student's t value) is given in Table 2 for several levels of confidence. The t -value for other levels of confidence can be calculated with a microcomputer spreadsheet using the built-in functions. In MS Excel, the function that returns t is TINV(\(\ce{\alpha}\), DF) where 1-\(\ce{\alpha}\) is the confidence level (for 95% confidence, \(\ce{\alpha}\) = 0.05) and DF is the degrees of freedom. The t -value can be viewed as a correction for the limited number of measurements in a data set and the associated errors in approximating \(\ce{\mu}\) and \(\ce{\sigma}\) by \(\ce{x_{avg}}\) and \(\ce{s}\), respectively. If N is infinite, the value of t for the various confidence limits in Table 2 equals the number multiplying \(\ce{\sigma}\) in the range column of Table 1 for the corresponding confidence. For example, for N infinite and 90.0% confidence, Table 2 gives t = 1.645. This agrees with the factor multiplying \(\ce{\sigma}\) for 90.0% confidence in Table 1. However, if N is finite, the value of t for a given confidence must increase as N decreases, since \(\ce{x_{avg}}\) and \(\ce{s}\) become poorer estimates of \(\ce{\mu}\) and \(\ce{\sigma}\).
* Imagine a group of data sets. For each set the sample mean is calculated. The group of means will show a scatter that is also described by a Gaussian curve. However, the width of this curve will be less than the width of the curve associated with a single data set; the standard deviation of the mean is less than the standard deviation of the data. The standard deviation in the mean, \(\ce{s_{x}}\), can be estimated from just one data set and its sample standard deviation, \(\ce{s}\), by \(\ce{s_{x}=\frac{s}{\sqrt{N}}}\). This means that the uncertainty in the mean decreases as the square-root of the number of measurements increases. Hence, to reduce the uncertainty in the mean by a factor of two, the number of measurements must be increased by a factor of four.
Estimates of \(\ce{\mu}\) and its confidence limits become important, say, in the calibration of an individual pipet. Let's assume that 10 determinations of the delivered volume for a particular pipet also yield \(\ce{x_{avg}=}\) 25.004 mL with \(\ce{s}\) = 0.0213 mL. The best estimate of the population mean (the mean from an infinite number of measurements of the delivered volume) is \(\ce{\mu = x_{avg} =}\)25.004 mL. Its 95.0% confidence limits for 10 measurements is then \(\ce{\pm \frac{ts}{\sqrt{N}} = \pm \frac{(2.262)(0.0213)}{\sqrt{10}}= \pm0.0152 } \) mL where the t value was obtained from Table 2. Accordingly, to 95.0% confidence the average volume of the particular pipet is 25.004 ± 0.015 mL. This means that there is a 95% probability that the true or population mean will lie within ±0.015 mL of 25.004 mL.
You will use the foregoing method of estimating the population or true mean and its confidence limits in many of your laboratory experiments. Typically you will make at least three measurements or determinations of a quantity. You will report the sample mean, \( \ce{x_{avg}} \), as an approximation of the true mean; the sample standard deviation, \( \ce{s}\) as an approximation of the population standard deviation; and the 95% confidence limits of the mean. The chosen confidence is that typically used when reporting scientific results. The mean is calculated from equation (1), the standard deviation from equation (2) and the confidence limits of the mean from equation (4) using the t values for 95% confidence in Table 2. Scientific calculators and microcomputer spreadsheets typically have built-in functions to calculate the sample mean and the sample standard deviation, but not confidence limits.
Sometimes a single piece of data appears inconsistent with the remaining data. For example, the questionable point may be much larger or much smaller than the remaining points. In such cases, one requires a valid method to test if the questionable point can be discarded in calculating the sample mean and standard deviation. The Q test is used to help make this decision.
Assume that the outlier (the data point in question) has a value \( \ce{x_{0}} \). Calculate the magnitude of the difference between \( \ce{x_{0}} \) and its nearest value from the remaining data (called the gap), and the magnitude of the spread of the total data including the value \( \ce{x_{0}} \) (called the range). The quantity \( \ce{Q_{Data}} \), given by
\( \ce{Q_{Data} = \frac{gap}{range}} \)
is compared with tabulated critical values of Q for a chosen confidence level. If \( \ce{Q_{Data}>Q_{Critical}} \), the outlier can be discarded to the chosen degree of confidence. The Q test is fairly stringent and not particularly helpful for small data sets if high confidence is required. It is common to use a 90% confidence for the Q test, so that any data point that has less than a 10% chance of being valid can be discarded. Values of \( \ce{Q_{Critical}} \) for 90% confidence are given in Table 3.
Let's consider an example to clarify the use of the Q test. Suppose that you make four determinations of the concentration of a solution, and that these yield 0.1155, 0.1150, 0.1148 and 0.1172 M. The mean concentration is 0.1156 M with a standard deviation of 0.0011 M. The 0.1172 M data point appears questionable since it is nearly two standard deviations away from the mean. The gap is (0.1172 - 0.1155) = 0.0017, and the range is (0.1172 - 0.1148) = 0.0024, so that \( \ce{Q_{Data}} \) = 0.0017/0.0024 = 0.71. Table 3 gives \( \ce{Q_{Critical}} \) = 0.76 for 90% confidence and four determinations. Since \( \ce{Q_{Data}<Q_{Critical}} \), the questionable point cannot be discarded. There is more than a 10% chance that the questionable point is valid.
Several caveats should be noted about the Q test. Firstly, it may be applied to only one outlier of a small data set. Secondly, even at 90% confidence some "bad" data point may be retained. If you are sure that the point is bad because of some action noted during the measurement (for example, you know that you overshot the endpoint of a titration for the particular sample during its analysis), then the point can and should be discarded. Thirdly, an apparent outlier can result in a limited data set simply from the statistical distribution of random errors, as occurred in the above example. Repeating the measurement so as to increase the data set, and thereby decrease the importance of the apparent outlier, is generally much more valuable than any statistical test.
Propagation of Errors
A quantity of interest may be a function of several independent variables, say f(x,y). It would be evaluated by performing arithmetic operations with several numbers (x and y), each of which has an associated random error. These random errors, which we denote as \(\ce{e_{x}}\) and \(\ce{e_{y}}\), may be simply estimates, standard deviations or confidence limits, so long as the same measure is used for both. How do these errors propagate in determining the corresponding error, \(\ce{e_{f}}\), in the final quantity of interest? This error is not simply the sum of the individual errors since some of these are likely to be positive and others negative, so that a certain cancellation will occur. We give below the equations determining \(\ce{e_{f}}\) for simple operations. These equations are obtained using differential calculus.
\( \ce{ f = \alpha x + \beta y } \) with \(\ce{\alpha} \) and \( \ce{\beta} \) constants.
\( \ce {e_{f}^{2} = \alpha^{2}e_{x}^{2} + \beta^{2}e_{y}^{2} } \)
\( \ce{ f = \alpha x^{n}\beta y^{m} } \) with \(\ce{\alpha} \), \(\ce{n} \) and \( \ce{m} \) constants.
\( \ce { \frac{e_{f}^{2}}{f^{2}} = n^{2} (\frac{e_{x}}{x})^{2} + m^{2}(\frac{e_{y}}{y})^{2} } \)
The case \( \ce{ f = \alpha x + \beta y } \) includes addition and subtraction by choosing the signs of \(\ce{\alpha} \) and \( \ce{\beta} \), while the case \( \ce{ f = \alpha x^{n}\beta y^{m} } \) includes multiplication and division by appropriate choice of the exponents. Any of the constants \(\ce{\alpha} \), \(\ce{\beta}\), \(\ce{n} \), or \( \ce{m} \) can be positive, negative or zero. The cases where \(\ce{\beta = 0}\) or \(\ce{m = 0}\), for example, correspond to the function depending on the single variable \(\ce{(x)}\). More complicated cases, involving, for example, both addition and division, can be deduced by treating the various parts or factors separately using the given equations. Two simple examples of error propagation are considered below.
Example 1. You determine the mass (let's call this f) of a substance by first weighing a container \(\ce{(x)}\) and then the container with the substance \(\ce{(y)}\), using a balance accurate to 0.1 mg. The mass of the substance is then \(\ce{f = y ? x}\), and its error from equation (6) is
\(\ce { e_{f} = (e^{2}_{x})^{1/2} = (0.1^{2} + 0.1^{2})^{1/2} = 0.14} \) mg
since \( \ce{\alpha = \beta = 1} \) and \( \ce{ e_{x} = e_{y} = 0.1} \) mg
Example 2. You determine the density, \(\ce{d}\), of a block of metal by separate measurements of its mass, \(\ce{m}\), and volume, \(\ce{V}\). The mass and volume are each measured four times. Their mean values with standard deviations in parentheses are \(\ce{m_{avg}}\) = 54.32 (0.05) g and \(\ce{V_{avg}}\) = 6.78 (0.02) mL. The density is 54.32/6.78 = 8.012 g/mL. The standard deviation in \(\ce{d}\), \(\ce{e_{d}}\), would be calculated from equation (7), which gives
\( \ce{ e_{d} = d[(\frac{e_{m}}{m})^{2} + (\frac{e_{V}}{V})^{2}]^{1/2} } \)
\( \ce { = 8.012 [(\frac{0.05}{54.32})^{2} + (\frac{0.02}{6.78})^{2}]^{1/2} }\)
\( \ce{ 8.012(0.00309) = 0.025 } \) g/mL
We can also obtain the 95% confidence limits for the true density by using the 95% confidence limits for the mass and volume. For four measurements or three degrees of freedom, Table 2 gives t = 3.182. The 95% confidence limits for the mass of the block become \(\ce{ \pm \frac{(3.182)(0.05)}{\sqrt{4}} = \pm{0.08} }\) g, and for its volume \( \ce{ \pm \frac{(3.182)(0.02)}{\sqrt{4}} = 0.03}\) mL. Then,
CLd (95%) \( \ce{ = \pm 8.012[(\frac{0.08}{54.32})^{2} + (\frac{0.03}{6.78})^{2}]^{1/2} } \)
\( \ce{ = \pm 8.012(0.0047) = \pm 0.038 } \) g/mL
Thus, there is a 95% probability that the true density of the metal block falls within \(\ce{\pm}\)0.04 g/mL of 8.01 g/mL, or there is less than a 5% probability that it is outside this range.
Example 3. Suppose that you measure some quantity x, but what you really want is \(\ce{f = x^{1/2}}\). You determine that \(\ce{x = 0.5054}\) with a standard deviation of 0.0004. Hence, \(\ce{f = (0.5054)^{1/2} = 0.7109}\). From equation (7) with \(\ce{n = 1/2}\) and \(\ce{m = 0}\), the propagated standard deviation in f becomes
\( \ce{ e_{f} = f[n^{2}(\frac{e_{x}}{x})^{2}]^{1/2} }\)
\( \ce{ 0.7109[(1/2)^{2}(\frac{0.0004}{0.5054})^{2}]^{1/2} = 0.0003 } \)
Method of Least Squares
You will often be asked to graph some experimental data, and to find the "best" straight line that represents the data. A possible approach is to visually determine the line from the graph with a straight edge, draw the line on the graph, and then measure its slope and intercept from the graph. But, will this line be the "best" line? To answer this question, we have to define what "best" means. However, even without such a definition, it is clear that the slope and intercept obtained by the visual approach are not unique, since different people would make different judgments. The least squares or linear regression method leads to unique answers for the slope and intercept of the best line.
Many scientific calculators and microcomputer spreadsheets have built-in algorithms or functions for a least squares analysis, i.e. , for obtaining the slope and intercept of the best line through a set of data points. The estimated errors (standard deviations) in the slope and intercept are also often available. You can use these functions without knowing the details of the least squares method, which require differential calculus and advanced statistics. However, you should understand its general idea. This is described in the following, sacrificing rigor for (hopefully) clarity.
Let's consider a particular experimental example. The volume of a gas is measured as a function of temperature, while maintaining the amount of gas and the pressure constant. The expected behavior of the gas follows from the ideal gas law, which is
\( \ce{PV = nRT} \)
where \(\ce{P}\) is the pressure, \(\ce{V}\) is the volume, \(\ce{n}\) is the amount in moles, \(\ce{R}\) is the ideal gas constant, and \(\ce{T}\) is the temperature in K (Kelvin). We assume that the temperature is measured in °C (Celsius), which we label t , where \(\ce{T = t + 273.15}\). The ideal gas law can be rewritten to better express the present experiment as
\(\ce{V = (\frac{nR}{P})T}\)
\( \ce{ = (\frac{nR}{P})t + (\frac{nR}{P})(273)}\)
Equation (9) has the form \( \ce {y=mx+b} \), the equation for a straight line, with \( \ce {x=t} \). Thus, a graph of V versus t for the gas should be a straight line with slope \( \ce{ m = (\frac{nR}{P}) }\) and a V-intercept at \( \ce{t=0}\) of \( \ce{ b = (\frac{nR}{P})(273) }\). The equation also shows that the volume of a gas would equal zero at \( \ce{ t = t_{0} = -273 }\), corresponding to absolute zero \( \ce{(T=0)}}\).
Even though absolute zero cannot be realized, we can calculate its temperature in °C, \(\ce{t_{0}}\), from measurements of the volume of a gas at several temperatures with constant pressure and amount of gas. What we require is the slope and intercept of the graph of V versus t , since
\( \ce{ t_{0} = - \frac{(\frac{nR}{P})(273)}{(\frac{nR}{P})} = - \frac{intercept}{slope} }\)
We want the best values of the slope and intercept for this calculation. Figure 2 shows example student data for the volume of a sample of gas as a function of temperature. Both the volume (y) and temperature (x) measurements were subject to random errors, but those in the volume greatly exceeded those in the temperature. Hence, the points deviate from a straight line due mainly to the random errors in the volumes. The best line through the data points should then minimize the magnitude of the vertical (y) deviations between the experimental points and the line. Since these deviations are positive and negative, the squares of the deviations are minimized (hence, the name least squares).
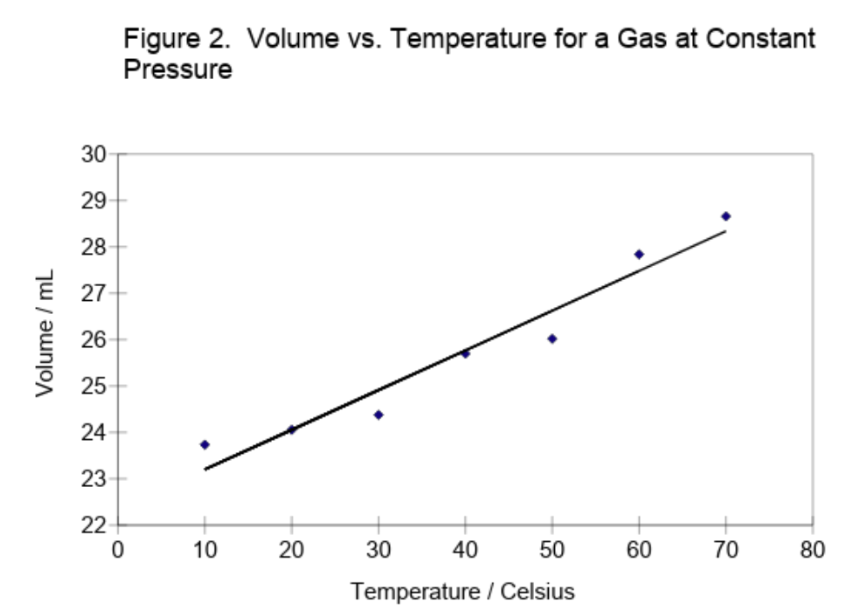
Minimizing the sum of the squares of the deviations corresponds to assuming that the observed set of volumes is the most probable set. The probability of observing a particular volume, \(\ce{V_{i}}\), for some temperature, \(\ce{t_{i}}\), is given by a Gaussian curve with the true volume \(\ce{(\mu_{v})}\) given by equation (9). The probability of obtaining the observed set of volumes is given by the product of these Gaussian curves. Maximizing this probability, so that the observed volumes are the most probable set, is equivalent to minimizing the sum of the squares of the vertical deviations. The minimization is accomplished using differential calculus, and yields equations for the slope and intercept of the best line in terms of all of the data points (all of the pairs \(\ce{V_{i}}\) and \(\ce{t_{i}}\) or, more generally, \(\ce{y_{i}}\) and \(\ce{x_{i}}\)). The resulting equations for the slope and intercept and for the standard deviations in these quantities are given in a variety of texts.* They will not be repeated here, since in this course you will use the built-in functions of a microcomputer spreadsheet to perform least squares analyses.
The line shown in Figure 2 results from a least squares fit to the experimental data. The parameters of this best-fit line and their standard deviations, obtained using a spreadsheet, are given below.
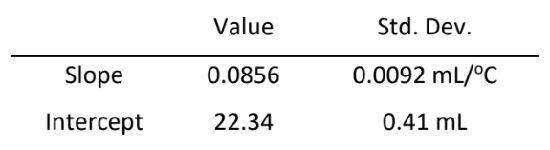
The sum of the vertical deviations, including their signs, of the experimental data points from this line equals zero, which is what one would intuitively attempt to accomplish in visually drawing the line. The sum of the deviations will always be zero for the best line, just as the sum of the deviations from an average or mean will always be zero.
The best-fit parameters yield \( \ce{ t_{0} = - (\frac{22.34}{0.0856}) = -261 }\)°C. We can obtain the uncertainty in \( \ce{t_{0}}\) by propagating the uncertainties in the slope and intercept using the formula given earlier for division involving independent variables. This is only approximately correct in the present case since the slope and intercept are correlated, and not independent. The full treatment for correlated variables, however, is beyond the scope of this introduction. The earlier formula, equation (7), gives
\( \ce{ St_{0} = 261[(\frac{0.0092}{0.0856})^{2} + (\frac{0.41}{22.34})^{2}]^{1/2} }\)
\(\ce{ 261(0.109) = 28 }\)°C
Hence, the extrapolated value of t0 agrees with the expected value within one (approximate) standard deviation.
We will now confess the truth about the "student data" in Figure 2. This was generated by adding a purely random number between -0.5 and +0.5 mL to the volume calculated for each temperature from equation (9) with \(\ce{ n = 1.0 x 10^{-3} }\) moles, \( \ce{ P = 1.0 }\) atm, and \( \ce{ R = 82.06 }\) \(\ce { \frac{mL*atm}{mol*K}} \). Hence, the expected slope is \( \ce{ (\frac{nR}{P}) = 0.08206 \frac{mL}{°C} }\), and the expected intercept is \( \ce{ (\frac{nR}{P})(273.15) = 22.414 }\) mL. The corresponding parameters derived by the least squares analysis differ from the expected values because of the random errors added to the volume. However, the derived parameters do agree with the expected or true values within one standard deviation.
Questions (Only for students who did not take 4A)
1. Multiple determinations of the percent by mass of iron in an unknown ore yield 15.31, 15.30, 15.26, 15.28 and 15.29%. Calculate:
(a) The mean percent by mass of iron;
(b) The sample standard deviation;
(c) The standard deviation of the mean;
(d) and the 95% CL of the mean.
2. For each of the following data sets, determine whether any measurement should be rejected at the 90% confidence level using the Q test.
(a) 2.8, 2.7, 2.5, 2.9, 2.6, 3.0, 2.6
(b) 97.13, 97.10, 97.20, 97.35, 97.10, 97.15
(c) 0.134, 0.120, 0.109, 0.124, 0.131, 0.119
3. A chemist determines the number of moles, n , of some gas from measurements of the pressure, P , volume, V , and temperature, T , of the gas, using the ideal gas equation of state, as \(\ce{n = \frac{PV}{RT}}\). The results of the measurements, with the estimated standard deviations in parentheses, are \(\ce{P = 0.235 (0.005)}\) atm, \(\ce{V = 1.22 (0.03)}\) L, and \(\ce{T = 310 (2)}\) K. The constant R equals \(\ce{0.08206 \frac{L*atm}{mol*K}}\). Calculate n and its estimated standard deviation.
4. Use the method of least squares to find the slope, m , intercept, b , and the respective standard deviations of the best straight line, \(\ce{ y = mx + b}\), for representing the following data
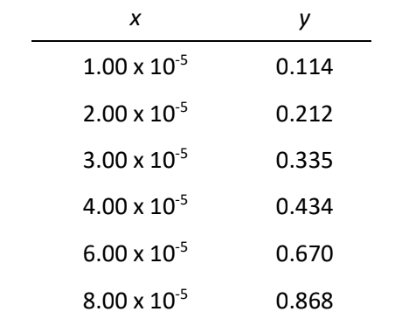
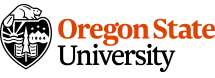
5 Random Error
Learning Objectives
After reading this chapter, you will be able to do the following:
- Define random error and differentiate it from bias
- Illustrate random error with examples
- Interpret a p -value
- Interpret a confidence interval
- Differentiate between type 1 and type 2 statistical errors and explain how they apply to epidemiologic research
- Describe how statistical power affects research
In this chapter, we will cover random error —where it comes from, how we deal with it, and what it means for epidemiology.
What Is Random Error?
First and foremost, random error is not bias . Bias is systematic error and is covered in further detail in chapter 6.
Random error is just what it sounds like: random errors in the data. All data contain random errors, because no measurement system is perfect. The magnitude of random errors depends partly on the scale on which something is measured (errors in molecular-level measurements would be on the order of nanometers, whereas errors in human height measurements are probably on the order of a centimeter or two) and partly on the quality of the tools being used. Physics and chemistry labs have highly accurate, expensive scales that can measure mass to the nearest gram, microgram, or nanogram, whereas the average scale in someone’s bathroom is probably accurate within a half-pound or pound.
To wrap your head around random error, imagine that you are baking a cake that requires 6 tablespoons of butter. To get the 6 tablespoons of butter (three-quarters of a stick, if there are 4 sticks in a pound, as is usually true in the US), you could use the marks that appear on the waxed paper around the stick, assuming they are lined up correctly. Or you could perhaps follow my mother’s method, which is to unwrap the stick, make a slight mark at what looks like one-half of the stick, and then get to three-quarters by eyeballing half of the one-half. Or you could use my method, which is to eyeball the three-quarter mark from the start and slice away. Any of these “measurement” methods will give you roughly 6 tablespoons of butter, which is certainly good enough for the purposes of baking a cake—but probably not exactly 3 ounces’ worth, which is how much 6 tablespoons of butter weighs in the US. [i] The extent to which you’re slightly over 3 ounces this time and perhaps slightly under 3 ounces next time is causing random error in your measurement of butter. If you always underestimated or always overestimated, then that would be a bias—however, your consistently under- or overestimated measurements would within themselves contain random error.
Inherent Variability
For any given variable that we might want to measure in epidemiology (e.g., height, GPA, heart rate, number of years working at a particular factory, serum triglyceride level, etc.), we expect there to be variability in the sample—that is, we do not expect everyone in the population to have exactly the same value. This is not random error. Random error (and bias) occurs when we try to measure these things. Indeed, epidemiology as a field relies on this inherent variability. If everyone were exactly the same, then we would not be able to identify which kinds of people were at higher risk for developing a particular disease.
In epidemiology, sometimes our measurements rely on a human other than the study participant measuring something on or about the participant. Examples would include measured height or weight, blood pressure, or serum cholesterol. For some of these (e.g., weight and serum cholesterol), the random error creeps into the data because of the instrument being used—here, a scale that has probably a half-pound fluctuation, or a laboratory assay with a margin of error of a few milligrams per deciliter. For other measurements (e.g., height and blood pressure), the measurer themselves is responsible for any random error, as in the butter example.
However, many of our measurements rely on participant self-reporting. There are whole textbooks and classes devoted to questionnaire design, and the science behind how to get the most accurate data from people via survey methods is quite good. The Pew Research Center offers a nice introductory tutorial on questionnaire design on its website.
Relevant to our discussion here, random error will appear in questionnaire data as well. For some variables, there will be less random error than others (e.g., self-reported race is probably quite accurate), but there will still be some—for example, people accidentally checking the wrong box. For other variables, there will be more random error (e.g., imprecise answers to questions such as, “In the last year, how many times per month did you eat rice?”). A good question to ask yourself when considering the amount of random error that might be in a variable derived from a questionnaire is, “ Can people tell me this?” Most people could theoretically tell you how much sleep they got last night, but they would be hard-pressed to tell you how much sleep they got on the same night one year ago. Whether or not they will tell you is a different matter and touches on bias (see chapter 6). Regardless, random error in questionnaire data increases as the likelihood that people could tell you the answer decreases.
Quantifying Random Error
While we can—and should—work to minimize random error (using high-quality instruments, training staff on how to take measurements, designing good questionnaires, etc.), it can never be eliminated entirely. Luckily, we can use statistics to quantify the random errors present in a study. Indeed, this is what statistics is for. In this book, I will cover only a small slice of the vast field of statistics: interpretation of p -values and confidence intervals (CI) . Rather than focus on how to calculate them [1] , I will instead focus on what they mean (and what they do not mean). Knowledge of p-values and CIs is sufficient to allow accurate interpretation of the results of epidemiologic studies for beginning epidemiology students.
When conducting scientific research of any kind, including epidemiology, one begins with a hypothesis, which is then tested as the study is conducted. For example, if we are studying average height of undergraduate students, our hypothesis (usually indicated by H 1 ) might be that male students are, on average, taller than female students. However, for statistical testing purposes, we must rephrase our hypothesis as a null hypothesis [2] . In this case, our null hypothesis (usually indicated by H 0 ) would be the following:
We would then undertake our study to test this hypothesis. We first determine the target population (undergraduate students) and draw a sample from this population. We then measure the heights and genders of everyone in the sample, and calculate mean height among men versus that among women. We would then conduct a statistical test to compare the mean heights in the 2 groups. Because we have a continuous variable (height) measured in 2 groups (men and women), we would use a t -test [3] , and the t -statistic calculated via this test would have a corresponding p -value, which is what we really care about.
Let’s say that in our study we find that male students average 5 feet 10 inches, and among female students the mean height is 5 feet 6 inches (for a difference of 4 inches), and we calculate a p -value of 0.04. This means that if there really is no difference in average height between male students and female students (i.e., if the null hypothesis is true) and we repeat the study (all the way back to drawing a new sample from the population), there is a 4% chance that we will again find a difference in mean height of 4 inches or more .
There are several implications that stem from the above paragraph. First, in epidemiology we always calculate 2-tailed p -values. Here this simply means that the 4% chance of a ≥4 inch height difference says nothing about which group is taller—just that one group (either males or females) will be taller on average by at least 4 inches. Second, p-values are meaningless if you happen to be able to enroll the entire population in your study. As an example, say our research question pertains to students in Public Health 425 (H425, Foundations of Epidemiology) during the 2020 winter term at Oregon State University (OSU). Are men or women taller in this population? As the population is quite small and all members are easily identified, we can enroll everyone instead of having to rely on a sample. There will still be random error in the measurement of height, but we no longer use a p -value to quantify it. This is because if we were to repeat the study, we would find exactly the same thing, since we actually measured everyone in the population. P -values only apply if we are working with samples.
Finally, note that the p -value describes the probability of your data, assuming the null hypothesis is true—it does not describe the probability of the null hypothesis being true given your data. This is a common interpretation mistake made by both beginning and senior readers of epidemiologic studies. The p -value says nothing about how likely it is that the null hypothesis is true (and thus on the flip side, about the truth of your actual hypothesis). Rather, it quantifies the likelihood of getting the data that you got if the null hypothesis did happen to be true. This is a subtle distinction but a very important one.
Statistical Significance
What happens next? We have a p -value, which tells us the chance of getting our data given the null hypothesis. But what does that actually mean in terms of what to conclude about a study’s results? In public health and clinical research, the standard practice is to use p ≤ 0.05 to indicate statistical significance . In other words, decades of researchers in this field have collectively decided that if the chance of committing a type I error (more on that below) is 5% or less, we will “reject the null hypothesis.” Continuing height example from above, we would thus conclude that there is a difference in height between genders, at least among undergraduate students. For p -values above 0.05, we “fail to reject the null hypothesis,” and instead conclude that our data provided no evidence that there was a difference in height between male and female undergraduate students.
Failing to Reject the Null vs. Accepting the Null
If p > 0.05, we fail to reject the null hypothesis. We do not ever accept the null hypothesis because it is very difficult to prove the absence of something. “Accepting” the null hypothesis implies that we have proven that there really is no difference in height between male and female students, which is not what happened. If p > 0.05, it merely means that we did not find evidence in opposition to the null hypothesis— not that said evidence doesn’t exist. We might have gotten a weird sample, we might have had too small a sample, etc. There is a whole field of clinical research (comparative effectiveness research vi ) dedicated to showing that one treatment is no better or worse than another; the field’s methods are complex, and the sample sizes required are quite large. For most epidemiologic studies, we simply stick to failing to reject.
Is the p ≤ 0.05 cutoff arbitrary? Absolutely. This is worth keeping in mind, particularly for p -values very near this cutoff. Is 0.49 really that different from 0.51? Likely not, but they are on opposite sides of that arbitrary line. The size of a p -value depends on 3 things: the sample size, the effect size (it is easier to reject the null hypothesis if the true difference in height—were we to measure everyone in the population, rather than only our sample—is 6 inches rather than 2 inches), and the consistency of the data, most commonly measured by the standard deviations around the mean heights in the 2 groups. Thus a p -value of 0.51 could almost certainly be made smaller by simply enrolling more people in the study (this pertains to power , which is the inverse of type II error , discussed below). It is important to keep this fact in mind when you read studies.
Frequentist versus Bayesian Statistics
Statistical significance testing is part of a branch of statistics referred to as frequentist statistics . ii Though extremely common in epidemiology and related fields, this practice is not generally regarded as an ideal science, for a number of reasons. First and foremost, the 0.05 cutoff is entirely arbitrary, iii and strict significance testing would reject the null for p = 0.049 but fail to reject for p = 0.051, even though they are nearly identical. Second, there are many more nuances to interpretation of p -values and confidence intervals than those I have covered in this chapter. iv For instance, the p -value is really testing all analysis assumptions, not just the null hypothesis, and a large p -value often indicates merely that the data cannot discriminate among numerous competing hypotheses. However, since public health and clinical medicine both require yes-or-no decisions (Should we spend resources on that health education campaign? Should this patient get this medication?), there needs to be some system for deciding yay or nay, and statistical significance testing is currently it. There are other ways of quantifying random error, and indeed Bayesian statistics (which instead of a yes-or-no answer yields a probability of something happening) ii is becoming more and more popular. Nonetheless, as frequentist statistics and null hypothesis testing are still by far the most common methods used in epidemiologic literature, they are the focus of this chapter.
Type I and Type II errors
A type I error (usually symbolized by α, the Greek letter alpha , and closely related to p -values) is the probability that you incorrectly reject the null hypothesis – in other words, that you “find” something that’s not really there. By choosing 0.05 as our statistical significance cut-off, we in the public health and clinical research fields have tacitly agreed that we are willing to accept that 5% of our findings will really be type I errors, or false positives .
A type II error (usually symbolized by β, the Greek letter beta ) is the opposite: β is the probability that you incorrectly fail to reject the null hypothesis—in other words, you miss something that really is there.
Power in epidemiologic studies varies widely: ideally it should be at least 90% (meaning the type II error rate is 10%), but often it is much lower. Power is proportional to sample size but in an exponential manner—power goes up as sample size goes up, but to get from 90 to 95% power requires a much larger jump in sample size than to go from 40 to 45% power. If a study fails to reject the null hypothesis, but the data look like there might be a large difference between groups, often the issue is that the study was underpowered, and with a larger sample, the p -value would probably fall below the magic 0.05 cutoff. On the other hand, part of the issue with small samples is that you might just by chance have gotten a non-representative sample, and adding additional participants would not drive the results toward statistical significance. As an example, suppose we are again interested in gender-based height differences, but this time only among collegiate athletes. We begin with a very small study—just one men’s team and one women’s team. If we happen to choose, say, the men’s basketball team and the women’s gymnastics team, we are likely to find a whopping difference in mean heights—perhaps 18 inches or more. Adding other teams to our study would almost certainly result in a much narrower difference in mean heights, and the 18 inch difference “found” in our initial small study would not hold up over time.
Confidence Intervals
Because we have set the acceptable [latex]\alpha[/latex] level at 5%, in epidemiology and related fields, we most commonly use 95% confidence intervals (95% CI). One can use a 95% CI to do significance testing: if the 95% CI does not include the null value (0 for risk difference and 1.0 for odds ratios, risk ratios, and rate ratios), then p < 0.05, and the result is statistically significant .
Though 95% CI can be used for significance testing, they contain much more information than just whether the p-value is <0.05 or not. Most epidemiologic studies report 95% CI around any point estimates that are presented. The correct interpretation of a 95% CI is as follows:
We can also illustrate this visually:
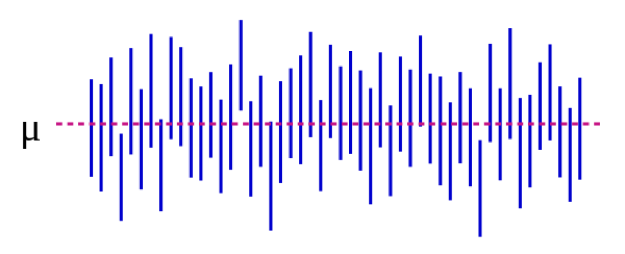
In Figure 5-1, the population parameter μ represents the “real” answer that you would get if you could enroll absolutely everyone in the population in the study. We estimate μ with data from our sample. Continuing with our height example, this might be 5 inches: if we could magically measure the heights of every single undergraduate student in the US (or the world, depending on how you defined your target population), the mean difference between male and female students would be 5 inches. Importantly, this population parameter is almost always unobservable—it only becomes observable if you define your population narrowly enough that you can enroll everyone. Each blue vertical line represents the CI of an individual “study”—50 of them, in this case. The CIs vary because the sample is slightly different each time—however, most of the CIs (all but 3, in fact) do contain μ.
If we conduct our study and find a mean difference of 4 inches (95% CI, 1.5 – 7), the CI tells us 2 things. First, the p -value for our t -test would be <0.05, since the CI excludes 0 (the null value in this case, as we are calculating a difference measure). Second, the interpretation of the CI is: if we repeated our study (including drawing a new sample) 100 times, then 95 of those times our CI would include the real value (which we know here is 5 inches, but which in real life you would not know). Thus looking at the CI here of 1.5 – 7.0 inches gives an idea of what the real difference might be—it almost certainly lies somewhere within that range but could be as small as 1.5 inches or as large as 7 inches. Like p -values, CIs depend on sample size. A large sample will yield a comparatively narrower CI. Narrower CIs are considered to be better because they yield a more precise estimate of what the “true” answer might be.
Random error is present in all measurements, though some variables are more prone to it than others. P -values and CIs are used to quantify random error. A p -value of 0.05 or less is usually taken to be “statistically significant,” and the corresponding CI would exclude the null value. CIs are useful for expressing the potential range of the “real” population-level value being estimated.
i. Butter in the US and the rest of the world. Errens Kitchen . March 2014. https://www.errenskitchen.com/cooking-conversions/butter-measurement-weight-conversions/. Accessed September 26, 2018. ( ↵ Return )
ii. Bayesian vs frequentist approach: same data, opposite results. 365 Data Sci . August 2017. https://365datascience.com/bayesian-vs-frequentist-approach/. Accessed October 17, 2018. ( ↵ Return 1 ) ( ↵ Return 2 )
iii. Smith RJ. The continuing misuse of null hypothesis significance testing in biological anthropology. Am J Phys Anthropol . 2018;166(1):236-245. doi:10.1002/ajpa.23399 ( ↵ Return )
iv. Farland LV, Correia KF, Wise LA, Williams PL, Ginsburg ES, Missmer SA. P-values and reproductive health: what can clinical researchers learn from the American Statistical Association? Hum Reprod Oxf Engl . 2016;31(11):2406-2410. doi:10.1093/humrep/dew192 ( ↵ Return )
v. Greenland S, Senn SJ, Rothman KJ, et al. Statistical tests, p values, confidence intervals, and power: a guide to misinterpretations. Eur J Epidemiol . 2016;31:337-350. doi:10.1007/s10654-016-0149-3
vi. Why is comparative effectiveness research important? Patient-Centered Outcomes Research Institute. https://www.pcori.org/files/why-comparative-effectiveness-research-important. Accessed October 17, 2018. ( ↵ Return )
- There isn’t just one formula for calculating a p -value or a CI. Rather, the formulas change depending on which statistical test is being applied. Any introductory biostatistics text that discusses which statistical methods to use and when would also provide the corresponding information on p -value and CI calculation. ↵
- Don’t spend too long trying to figure out why we need a null hypothesis; we just do. The rationale is buried in centuries of academic philosophy of science arguments. ↵
- How to choose the correct test is beyond the scope of this book—see any book on introductory biostatistics ↵
Inherent in all measurements. “Noise” in the data. Will always be present, but the amount depends on how precise your measurement instruments are. For instance, bathroom scales usually have 0.5 – 1 pound of random error; physics laboratories often contain scales that have only a few micrograms of random error (those are more expensive, and can only weigh small quantities). One can reduce the amount by which random error affects study results by increasing the sample size. This does not eliminate the random error, but rather better allows the researcher to see the data within the noise. Corollary: increasing the sample size will decrease the p-value , and narrow the confidence interval , since these are ways of quantifying random error.
Systematic error. Selection bias stems from poor sampling (your sample is not representative of the target population), poor response rate from those invited to be in a study, treating cases and controls or exposed/unexposed differently, and/or unequal loss to follow up between groups. To assess selection bias, ask yourself "who did they get, and who did they miss?"--and then also ask yourself "does it matter"? Sometimes it does, other times, maybe it doesn't.
Misclassification bias means that something (either the exposure, the outcome, a confounder, or all three) were measured improperly. Examples include people not being able to tell you something, people not being willing to tell you something, and an objective measure that is somehow systematically wrong (eg always off in the same direction, like a blood pressure cuff that is not zeroed correctly). Recall bias , social desirability bias, interviewer bias--these are all examples of misclassification bias. The end result of all of them is that people are put into the wrong box in a 2x2 table . If the misclassification is equally distributed between the groups (eg, both exposed and unexposed have equal chance of being put in the wrong box), it's non-differential misclassification . Otherwise, it's differential misclassification .
A way of quantifying random error . The correct interpretation of a p-value is: the probability that, if you repeated the study (go back to the target population, draw a new sample, measure everything, do the analysis), you would find a result at least as extreme, assuming the null hypothesis is true. If it’s actually true that there’s no difference between the groups, but your study found that there were 15% more smokers in group A with a p-value of 0.06, then that means that there's a 6% chance that, if you repeated the study, you'd again find 15% (or a bigger number) more smokers in one of the groups. In public health and clinical research, we usually use a cut-off of p < 0.05 to mean " statistically significant "--so, we are allowing a type I error rate of 5%. Thus, 5% of the time we'll "find" something, even though really there isn't a difference (ie, even though really the null hypothesis is true). The other 95% of the time, we are correctly rejecting the null hypothesis and concluding that there is a difference between the groups.
A way of quantifying random error . The correct interpretation of a confidence interval is: if you repeated the study 100 times (go back to your target population , get a new sample , measure everything, do the analysis), then 95 times out of 100 the confidence interval you calculate as part of this process will include the true value, assuming the study contains no bias . Here, the true value is the one that you would get if you were able to enroll everyone from the population into your study--this is almost never actually observable, since populations are usually too large to have everyone included in a sample. Corollary: If your population is small enough that you can have everyone in your study, then calculating a confidence interval is moot.
Used in statistical significance testing. The null hypothesis is always that there is not difference between the two groups under study.
A statistical test that determines whether the mean values in two groups are different.
A somewhat-arbitrary method for determining whether or not to believe the results of a study. In clinical and epidemiologic research, statistical significance is typically set at p < 0.05, meaning a type I error rate of <5%. As with all statistical methods, pertains to random error only; a study can be statistically significant but not believable, eg, if there is likelihood of substantial bias. A study can also be statistically significant (eg, p was < 0.05) but not clinically significant (eg, if the different in systolic blood pressure between the two groups was 2 mm Hg—with a large enough sample this would be statistically significant, but it matters not at all clinically).
The probability that a study “finds” something that isn’t there. Typically represented by α, and closely related to p-values . Usually set to 0.05 for clinical and epidemiologic studies.
The probability that your study will find something that is there. Power = 1 – β; beta is the type II error rate. Small studies, or studies of rare events, are typically under-powered.
The probability that a study did not find something that was there. Typically represented by β, and closely related to power . Ideally will be above 90% for clinical and epidemiologic studies, though in practice this often does not happen.
The measure of association that is calculated in a study. Typically presented with a corresponding 95% confidence interval .
Foundations of Epidemiology Copyright © 2020 by Marit Bovbjerg is licensed under a Creative Commons Attribution-NonCommercial 4.0 International License , except where otherwise noted.
Share This Book
Random vs Systematic Error
Random errors.
Examples of causes of random errors are:
- electronic noise in the circuit of an electrical instrument,
- irregular changes in the heat loss rate from a solar collector due to changes in the wind.
The precision of a measurement is how close a number of measurements of the same quantity agree with each other. The precision is limited by the random errors. It may usually be determined by repeating the measurements.
Systematic Errors
- there is something wrong with the instrument or its data handling system, or
- because the instrument is wrongly used by the experimenter.
- Offset or zero setting error in which the instrument does not read zero when the quantity to be measured is zero.
- Multiplier or scale factor error in which the instrument consistently reads changes in the quantity to be measured greater or less than the actual changes.
Examples of systematic errors caused by the wrong use of instruments are:
- errors in measurements of temperature due to poor thermal contact between the thermometer and the substance whose temperature is to be found,
- errors in measurements of solar radiation because trees or buildings shade the radiometer.
Taken from R. H. B. Exell, www.jgsee.kmutt.ac.th/exell/PracMath/ErrorAn.htm
- Testimonials
- Publications
- Class Schedule
- Terms and Conditions
- JC Syllabus
- IP/Secondary
Systematic And Random Errors: What To Look Out For
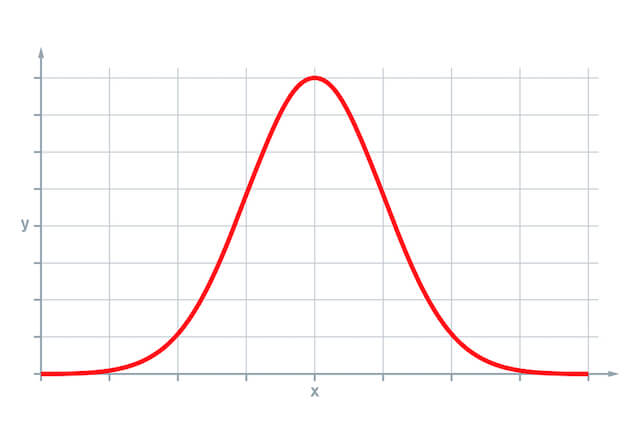
We like to believe that our measuring apparatus are perfect, but the sad fact is that they are not. Several types of errors can occur during your experiments which can affect the way you interpret the results. These include systematic and random errors. Here we will go through how to distinguish between the two types of errors and some important concepts that will help you understand their effects on your results, such as accuracy and precision.
Systematic error
Systematic errors are errors that cause your measurement to shift from the true value by the same amount every time. These errors often arise from faulty or poorly calibrated equipment. They can also be caused by human error if the person conducting the experiment makes the same mistake each time he takes a measurement.
There are two main types of systematic error:
1. Zero error – Measurement instruments in the lab have a zero function. In the case of weighing scales, it allows you to set the weight of the container you place the substance into 0g, such that you only measure the object or substance of interest. If your instrument does not actually set the weight of the container to 0g (e.g. 1g), your measurements will all be off by the weight your container is set to (e.g. +1g).
2. Scale error – This occurs when an instrument is poorly calibrated. If you encounter this error, all your results would be offset by the same fraction.
Systematic errors affect the accuracy of your results. Accuracy of a measurement refers to how close an experimental measurement is to the quantity’s true value.
Random error
Random errors are errors that shift your experimental measurement by a random amount each time. These can occur due to random fluctuations in experimental conditions or poor measurement practices on the researcher’s part. This kind of error often causes replicate results to have a normal distribution, as the measurements are centred around the true value. Here, the mean value is usually the best estimate of the true value, though it may not be the actual true value.
Some examples of random error include:
1. Reaction time – If your experiment involves timing with a stopwatch for example, the speed at which you stop the timing may affect how close to the true value the experimental measurement is. As you may have different reaction times with each round of the experiment, this is a random error.
2. Rounding error – If you were to use an instrument with low precision, rounding off the values may result in random error. Consider if you used a ruler with divisions of 0.1cm to measure the length objects. If the true length of an object is 2.57cm, you may measure it as 2.6cm, +0.03cm of the true value. Whereas if the true length is 2.52cm, you may measure it as 2.5cm, -0.02cm of the true value.
Random error affects the precision or reliability of your results. Precision refers to how close measurements are to one another; i.e. how consistent your measurements are. This has no bearing on the accuracy, i.e. how close your results are to the true value.
Systematic or random?
Sometimes certain errors can be considered systematic and random errors depending on the circumstances – for example, parallax error. If you read all the measurements from the same angle, it would be more likely that you would experience systematic error, as the shift in value would be the same each time. However, if you were to read the measurement from random angles each time, the error would be random.
Differentiating the errors is sometimes straightforward, but can at times be more nuanced. Still, it becomes easier with practice. If you need additional help with these physics concepts or any others, consider engaging us for Physics Tuition to help with your understanding.
TuitionPhysics
LinkedIn Profile
Copyright: Best Physics Tuition™ Centre. All Rights Reserved | User Sitemap
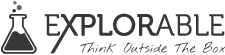
- Foundations
- Write Paper
Search form
- Experiments
- Anthropology
- Self-Esteem
- Social Anxiety
- Statistics >
Random Error
A random error, as the name suggests, is random in nature and very difficult to predict. It occurs because there are a very large number of parameters beyond the control of the experimenter that may interfere with the results of the experiment.
This article is a part of the guide:
- Significance 2
- Sample Size
- Experimental Probability
- Cronbach’s Alpha
- Systematic Error
Browse Full Outline
- 1 Inferential Statistics
- 2.1 Bayesian Probability
- 3.1.1 Significance 2
- 3.2 Significant Results
- 3.3 Sample Size
- 3.4 Margin of Error
- 3.5.1 Random Error
- 3.5.2 Systematic Error
- 3.5.3 Data Dredging
- 3.5.4 Ad Hoc Analysis
- 3.5.5 Regression Toward the Mean
- 4.1 P-Value
- 4.2 Effect Size
- 5.1 Philosophy of Statistics
- 6.1.1 Reliability 2
- 6.2 Cronbach’s Alpha
Random errors are caused by sources that are not immediately obvious and it may take a long time trying to figure out the source.
Random error is also called as statistical error because it can be gotten rid of in a measurement by statistical means because it is random in nature.
Unlike in the case of systematic errors , simple averaging out of various measurements of the same quantity can help offset random errors. Random errors can seldom be understood and are never fixed in nature - like being proportional to the measured quantity or being constant over many measurements.
The reason why random errors can be taken care of by averaging is that they have a zero expected value, which means they are truly random and scattered around the mean value. This also means that the arithmetic mean of the errors is expected to be zero.
There can be a number of possible sources of random errors and their source depends on the type of experiment and the types of measuring instruments being used.
For example, a biologist studying the reproduction of a particular strain of bacterium might encounter random errors due to slight variation of temperature or light in the room. However, when the readings are spread over a period of time, she may get rid of these random variations by averaging out her results.
A random error can also occur due to the measuring instrument and the way it is affected by changes in the surroundings. For example, a spring balance might show some variation in measurement due to fluctuations in temperature, conditions of loading and unloading, etc. A measuring instrument with a higher precision means there will be lesser fluctuations in its measurement.
Random errors are present in all experiments and therefore the researcher should be prepared for them. Unlike systematic errors, random errors are not predictable, which makes them difficult to detect but easier to remove since they are statistical errors and can be removed by statistical methods like averaging.
- Psychology 101
- Flags and Countries
- Capitals and Countries
Siddharth Kalla (Feb 4, 2009). Random Error. Retrieved Jun 20, 2024 from Explorable.com: https://explorable.com/random-error
You Are Allowed To Copy The Text
The text in this article is licensed under the Creative Commons-License Attribution 4.0 International (CC BY 4.0) .
This means you're free to copy, share and adapt any parts (or all) of the text in the article, as long as you give appropriate credit and provide a link/reference to this page.
That is it. You don't need our permission to copy the article; just include a link/reference back to this page. You can use it freely (with some kind of link), and we're also okay with people reprinting in publications like books, blogs, newsletters, course-material, papers, wikipedia and presentations (with clear attribution).
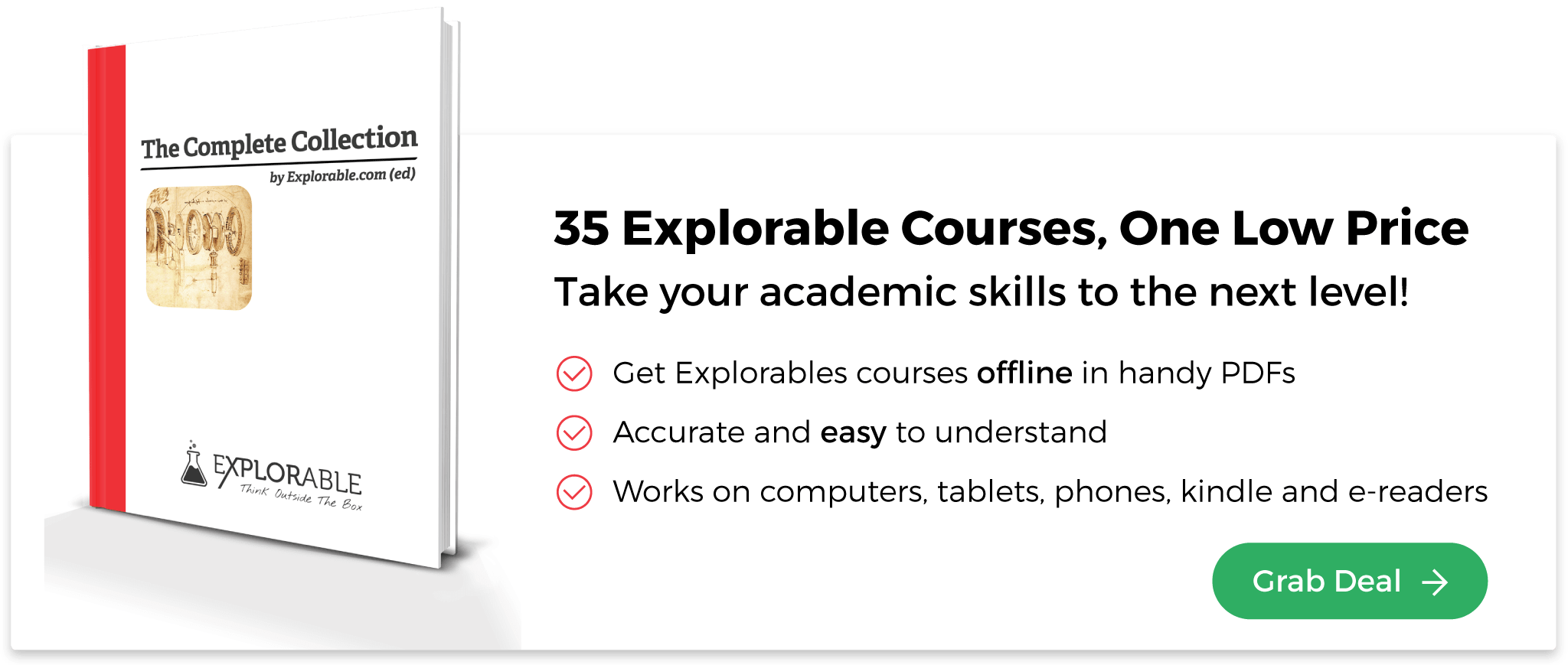
Related articles
Experimental Errors
Type-I Error and Type-II Error
Want to stay up to date? Follow us!
Save this course for later.
Don't have time for it all now? No problem, save it as a course and come back to it later.
Footer bottom
- Privacy Policy
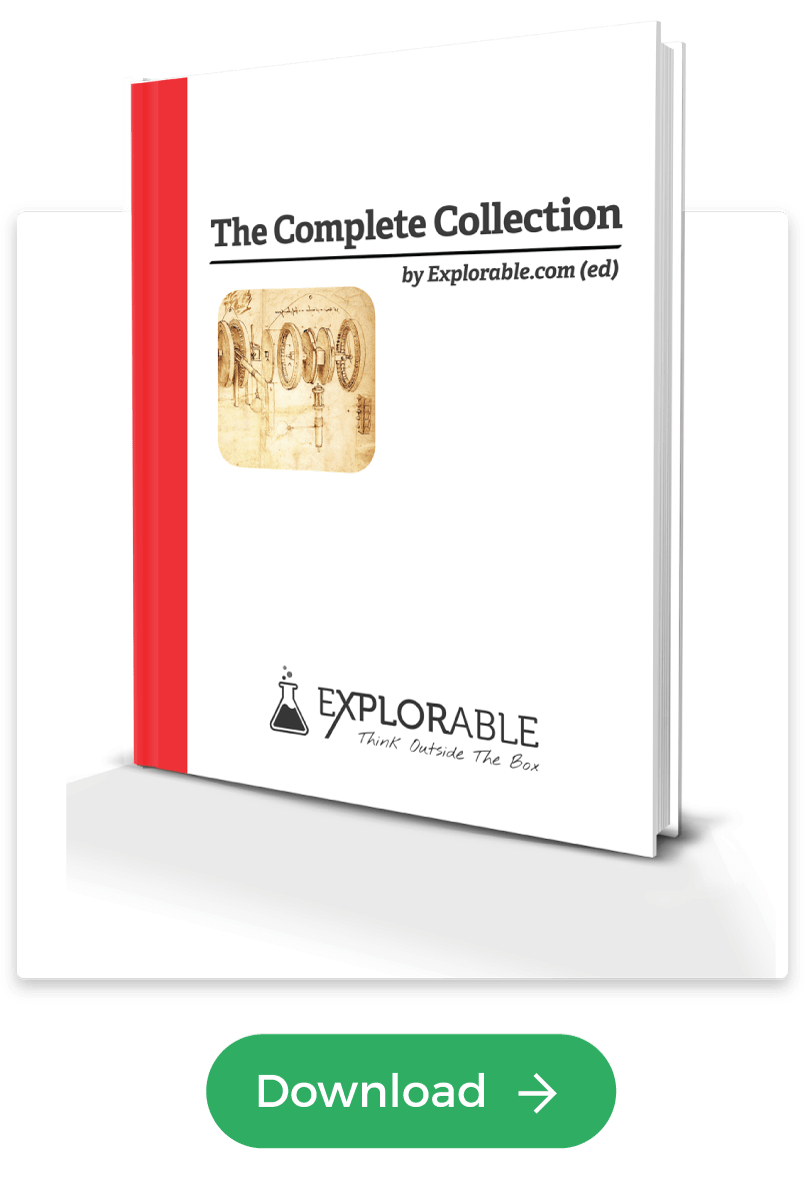
- Subscribe to our RSS Feed
- Like us on Facebook
- Follow us on Twitter
Learn The Types
Learn About Different Types of Things and Unleash Your Curiosity
Understanding Experimental Errors: Types, Causes, and Solutions
Types of experimental errors.
In scientific experiments, errors can occur that affect the accuracy and reliability of the results. These errors are often classified into three main categories: systematic errors, random errors, and human errors. Here are some common types of experimental errors:
1. Systematic Errors
Systematic errors are consistent and predictable errors that occur throughout an experiment. They can arise from flaws in equipment, calibration issues, or flawed experimental design. Some examples of systematic errors include:
– Instrumental Errors: These errors occur due to inaccuracies or limitations of the measuring instruments used in the experiment. For example, a thermometer may consistently read temperatures slightly higher or lower than the actual value.
– Environmental Errors: Changes in environmental conditions, such as temperature or humidity, can introduce systematic errors. For instance, if an experiment requires precise temperature control, fluctuations in the room temperature can impact the results.
– Procedural Errors: Errors in following the experimental procedure can lead to systematic errors. This can include improper mixing of reagents, incorrect timing, or using the wrong formula or equation.
2. Random Errors
Random errors are unpredictable variations that occur during an experiment. They can arise from factors such as inherent limitations of measurement tools, natural fluctuations in data, or human variability. Random errors can occur independently in each measurement and can cause data points to scatter around the true value. Some examples of random errors include:
– Instrument Noise: Instruments may introduce random noise into the measurements, resulting in small variations in the recorded data.
– Biological Variability: In experiments involving living organisms, natural biological variability can contribute to random errors. For example, in studies involving human subjects, individual differences in response to a treatment can introduce variability.
– Reading Errors: When taking measurements, human observers can introduce random errors due to imprecise readings or misinterpretation of data.
3. Human Errors
Human errors are mistakes or inaccuracies that occur due to human factors, such as lack of attention, improper technique, or inadequate training. These errors can significantly impact the experimental results. Some examples of human errors include:
– Data Entry Errors: Mistakes made when recording data or entering data into a computer can introduce errors. These errors can occur due to typographical mistakes, transposition errors, or misinterpretation of results.
– Calculation Errors: Errors in mathematical calculations can occur during data analysis or when performing calculations required for the experiment. These errors can result from mathematical mistakes, incorrect formulas, or rounding errors.
– Experimental Bias: Personal biases or preconceived notions held by the experimenter can introduce bias into the experiment, leading to inaccurate results.
It is crucial for scientists to be aware of these types of errors and take measures to minimize their impact on experimental outcomes. This includes careful experimental design, proper calibration of instruments, multiple repetitions of measurements, and thorough documentation of procedures and observations.
You Might Also Like:
Patio perfection: choosing the best types of pavers for your outdoor space, a guide to types of pupusas: delicious treats from central america, exploring modern period music: from classical to jazz and beyond.
IB Chemistry home > Syllabus 2016 > Data Processing > Random uncertainties and systematic errors
The term 'random' means something that happens by chance. When experiments are carried out there are many unforeseen situations that could affect the recorded data. |
|
Error and uncertainty
Errors in experiments arise from three general sources:
- 1 Instrumental inaccuracy
- 2 Human limitations
- 3 Experimental design
The goal of any good investigator is to minimise and quantify the errors whenever possible. To do this the investigator has to understand where and how the errors arise. This is the purpose of the evaluation.
Errors or uncertainties may be broadly categorised as either random or systematic. In the following sections we will take a look at how errors arise in experiments and what may be done to minimise them.
Instrumental accuracy
The instrumental accuracy must be considered for every piece of apparatus used. Good laboratory apparatus usually has the tolerance marked on it by the manufacturer. For example, a grade 'B' 50ml pipette may have the marking 50ml ± 0.07 @ 20ºC.
The uncertainty in a piece of apparatus is often called the 'tolerance'. The manufacturer includes the tolerance of the measuring instrument, assuming that it is used as per the instructions.
All measurements have an associated random uncertainty. We are limited by both the accuracy of the instruments that are used in measurement and by our own capabilities.
Even electronic instrumentation has to 'decide' on the final decimal place. If an electronic balance measures to two decimal places, it has to choose the second decimal place by considering the (unseen) third decimal. If the third is 5 or greater then the second decimal is 'rounded up' If the third decimal is 4 or less, the second is left unchanged. We don't see this operation in practice, but it means that there is always an uncertainty of +/- 0.005 in a two decimal place measure.
Glassware used to measure volume of solutions may be measuring cylinders, pipettes or burettes. All of these instruments require human judgement to gauge exactly at which level the solution lies. A solution is measured to a given 'mark' on the glassware. This requires our senses to be as accurate as possible, but everybody has human limitations. We just have to accept that there is an uncertainty when we record our results.
Similarly the manufacturer of the glassware is conditioned by the accuracy of the manufacturing process; the machines made to manufacture instrumentation also have their own inaccuracy.
And the conditions affect the measurements. Pipettes, for example are calibrated to measure solutions at 20ºC. This is rarely the exact temperature of a solution, once again introducing an inaccuracy.
So what do we do with all of this inaccuracy? The answer is that we have to accept and record it as part of our experimentation, to understand that it is ever-present and to try and quantify it so that we know the upper and lower limits of each measurement taken.
Human limitations
This is not what students usually understand by the term. Human error does not necessarily indicate that a mistake has been made. To differentiate between mistakes in experimentation and normal human limitation, some authors use the term 'blunders' instead of error to mean mistakes. This gives a sense that the experimenter has done something that he/she should not have, ie spilled some solution to be titrated, or dropped something onto the floor.
Unfortunately the term is often used in everyday life to suggest an incorrect action taken, or mistake made. "The crash was caused by human error"
In scientific terms, human error means the limitations inherent in measurements made by human agency, such as timing the disappearance of a cross drawn onto the side of a beaker due to the formation of a precipitate, judging the end-point of a titration etc.
Systematic errors
These are errors that are consistently produced in the course of an experiment by poor design, or some inherent fault or limitation in the apparatus. They may also be due to poor experimental techniques.
These errors can never be quantified completely, nor can the experiments be made more reliable by repetition. However, they can be improved by changing the experimental design, by improving the measuring techniques etc.
Typical systematic errors include
- Reading the position of the meniscus of a liquid incorrectly
- Losing heat to the environment in a thermochemistry experiment
- Using dirty pipettes, which retain drops of solution, reducing the volume delivered.
Definitions Summary
Systematic errors: - An error that is repeated throughout the course of an experiment is said to be a systematic error. These may be due to inaccuracy in the apparatus, or in the techniques applied.
Precision:- This refers to how close the measured values are to one another. Readings may be very precise, but wildly inaccurate.
Accuracy:- This refers to how close the precise values are to the literature accepted values.
Repeatable:- This is linked to precision in that if one person is conducting the same experiment and produces precise results the experiment is said to be repeatable.
Reproducible:- The is effectively the same as repeatable, but for other groups, or studies that produce the same precise results.
Tolerance:- The accepted accuracy of a piece of apparatus when used in the manner described by the manufacturer.

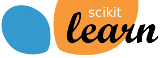
API Reference #
This is the class and function reference of scikit-learn. Please refer to the full user guide for further details, as the raw specifications of classes and functions may not be enough to give full guidelines on their uses. For reference on concepts repeated across the API, see Glossary of Common Terms and API Elements .
Object | Description |
---|---|
| |
| . |
| |
| |
| |
| |
| |
| |
| |
| |
| |
| for simple transformers. |
| |
| |
| |
| |
| |
| |
| |
| |
| |
| |
| |
| |
| |
| |
| |
| |
| |
| |
| |
| |
| |
| |
| |
| |
| |
| |
| |
| |
| |
| |
| |
| |
| |
| |
| |
| |
| |
| |
| |
| |
| |
| |
| |
| |
| |
| |
| |
| |
| |
| |
| |
| |
| |
| |
| |
| |
| |
| |
| |
| |
| |
| |
| |
| |
| |
| |
| |
| |
| |
| |
| |
| |
| |
| |
| |
| |
| |
| |
| |
| |
| |
| |
| |
| |
| |
| |
| |
| |
| |
| |
| |
| |
| |
| |
| |
| |
| |
| |
| |
| |
| |
| |
| |
| |
| |
| |
| |
| |
| |
| |
| |
| |
| |
| |
| |
| |
| |
| |
| |
| |
| |
| |
| |
| |
| |
| |
| |
| |
| |
| |
| |
| |
| |
| |
| |
| |
| |
| |
| |
| |
| |
| |
| |
| |
| |
| |
| |
| |
| |
| |
| |
| |
| |
| |
| |
| |
| |
| |
| |
| |
| |
| |
| |
| |
| |
| |
| |
| |
| |
| |
| |
| |
| |
| |
| |
| |
| |
| |
| |
| |
| |
| |
| |
| |
| |
| |
| |
| |
| |
| |
| |
| |
| kernel takes two kernels \(k_1\) and \(k_2\) |
| |
| |
| kernel takes two kernels \(k_1\) and \(k_2\) |
| |
| |
| |
| |
| |
| . |
| . |
| |
| |
| |
| |
| |
| |
| |
| |
| |
| |
| |
| |
| |
| |
| |
| |
| |
| |
| |
| |
| |
| |
| |
| |
| |
| |
| |
| |
| |
| |
| |
| |
| |
| |
| |
| |
| |
| |
| |
| |
| |
| |
| |
| |
| |
| |
| |
| |
| |
| |
| |
| |
| |
| |
| |
| |
| |
| |
| |
| |
| |
| |
| |
| |
| |
| |
| |
| |
| |
| |
| |
| |
| |
| |
| |
| |
| |
| score function, fraction of log loss explained. |
| |
| |
| |
| |
| |
| |
| |
| |
| |
| |
| |
| |
| |
| |
| |
| |
| |
| |
| |
| regression score function, fraction of absolute error explained. |
| regression score function, fraction of pinball loss explained. |
| regression score function, fraction of Tweedie deviance explained. |
| |
| |
| |
| |
| |
| |
| |
| |
| |
| |
| |
| (coefficient of determination) regression score function. |
| |
| |
| |
| |
| |
| |
| |
| |
| |
| |
| |
| |
| |
| |
| |
| |
| |
| |
| |
| |
| |
| |
| |
| |
| |
| |
| |
| |
| |
| |
| |
| |
| |
| |
| |
| |
| |
| |
| |
| |
| |
| |
| |
| |
| |
| |
| |
| |
| |
| |
| |
| |
| |
| |
| |
| |
| |
| |
| |
| |
| |
| |
| |
| |
| |
| |
| |
| |
| |
| |
| |
| |
| |
| |
| |
| |
| |
| |
| |
| |
| |
| |
| |
| |
| |
| |
| |
| |
| |
| |
| |
| |
| |
| |
| |
| |
| |
| |
| |
| |
| |
| |
| |
| |
| |
| |
| |
| |
| |
| |
| |
| |
| |
| |
| |
| |
| |
| |
| |
| |
| from the given estimators. |
| from the given transformers. |
| |
| |
| |
| . |
| |
| |
| |
| |
| |
| |
| |
| |
| |
| |
| |
| |
| |
| |
| |
| |
| |
| |
| |
| |
| |
| |
| |
| |
| |
| |
| |
| |
| |
| |
| |
| |
| |
| |
| |
| |
| |
| |
| |
| |
| |
| |
| |
| |
| |
| |
| |
| |
| |
| |
| |
| |
| elements from 0 to . |
| evenly spaced slices going up to . |
| |
| |
| |
| |
| |
| |
| |
| |
| |
| |
| |
| |
| is joblib.Memory-like. |
| |
| |
| |
| |
| |
| |
| is in a multilabel format. |
| |
| |
| |
| |
| |
| |
| |
| |
| |
| |
| |
| |
| |
| |
| |
| |
| |
| |
| |
| |
| |
| |
| |
| Request} instance from the given object. |
| |
| . |
| |
| |
| that propagates the scikit-learn configuration. |
| |
| . |
| . |
| |
|

Imputation for Lipidomics and Metabolomics (ImpLiMet): Online optimization and method selection for missing data imputation
- Find this author on Google Scholar
- Find this author on PubMed
- Search for this author on this site
- For correspondence: [email protected]
- Info/History
- Supplementary material
- Preview PDF
Motivation: Missing values are often unavoidable in modern high-throughput measurements due to various experimental or analytical reasons. Imputation, the process of replacing missing values in a dataset with estimated values, plays an important role in multivariate and machine learning analyses. Three missingness patterns have been conceptualized: missing completely at random (MCAR), missing at random (MAR), and missing not at random (MNAR). Each describes unique dependencies between the missing and observed data. As a result, the optimal imputation method for each dataset depends on the type of data, the cause of the missing data, and the nature of relationships between the missing and observed data. The challenge is to identify the optimal imputation solution for a given dataset. Results: Imputation for Lipidomics and Metabolomics (ImpLiMet) is a user-friendly UI-platform that enables users to impute missing data using eight different methods. Using the dataset, ImpLiMet can suggest the optimal imputation solution through a grid search-based investigation of the error rate for imputation across three missingness data simulations. The effect of imputation can be visually assessed by principal component analysis (PCA) comparing the impact of removing all features and samples with missing data with the chosen imputation method. Availability and implementation: ImpLiMet is freely available at https://complimet.ca/shiny/implimet/ with software accessible at https://github.com/complimet/ImpLiMet
Competing Interest Statement
The authors have declared no competing interest.
https://complimet.ca/shiny/implimet/
View the discussion thread.
Supplementary Material
Thank you for your interest in spreading the word about bioRxiv.
NOTE: Your email address is requested solely to identify you as the sender of this article.

Citation Manager Formats
- EndNote (tagged)
- EndNote 8 (xml)
- RefWorks Tagged
- Ref Manager
- Tweet Widget
- Facebook Like
- Google Plus One
Subject Area
- Bioinformatics
- Animal Behavior and Cognition (5387)
- Biochemistry (12153)
- Bioengineering (9100)
- Bioinformatics (30041)
- Biophysics (15421)
- Cancer Biology (12542)
- Cell Biology (17990)
- Clinical Trials (138)
- Developmental Biology (9718)
- Ecology (14560)
- Epidemiology (2067)
- Evolutionary Biology (18722)
- Genetics (12522)
- Genomics (17169)
- Immunology (12265)
- Microbiology (28920)
- Molecular Biology (11990)
- Neuroscience (62960)
- Paleontology (462)
- Pathology (1929)
- Pharmacology and Toxicology (3354)
- Physiology (5166)
- Plant Biology (10763)
- Scientific Communication and Education (1704)
- Synthetic Biology (2989)
- Systems Biology (7516)
- Zoology (1689)
Information
- Author Services
Initiatives
You are accessing a machine-readable page. In order to be human-readable, please install an RSS reader.
All articles published by MDPI are made immediately available worldwide under an open access license. No special permission is required to reuse all or part of the article published by MDPI, including figures and tables. For articles published under an open access Creative Common CC BY license, any part of the article may be reused without permission provided that the original article is clearly cited. For more information, please refer to https://www.mdpi.com/openaccess .
Feature papers represent the most advanced research with significant potential for high impact in the field. A Feature Paper should be a substantial original Article that involves several techniques or approaches, provides an outlook for future research directions and describes possible research applications.
Feature papers are submitted upon individual invitation or recommendation by the scientific editors and must receive positive feedback from the reviewers.
Editor’s Choice articles are based on recommendations by the scientific editors of MDPI journals from around the world. Editors select a small number of articles recently published in the journal that they believe will be particularly interesting to readers, or important in the respective research area. The aim is to provide a snapshot of some of the most exciting work published in the various research areas of the journal.
Original Submission Date Received: .
- Active Journals
- Find a Journal
- Proceedings Series
- For Authors
- For Reviewers
- For Editors
- For Librarians
- For Publishers
- For Societies
- For Conference Organizers
- Open Access Policy
- Institutional Open Access Program
- Special Issues Guidelines
- Editorial Process
- Research and Publication Ethics
- Article Processing Charges
- Testimonials
- Preprints.org
- SciProfiles
- Encyclopedia
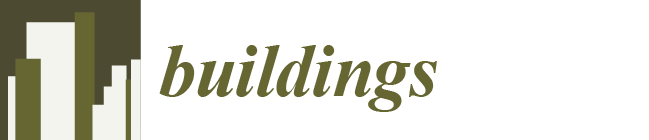
Article Menu
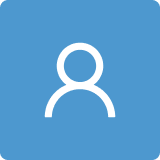
- Subscribe SciFeed
- Recommended Articles
- Google Scholar
- on Google Scholar
- Table of Contents
Find support for a specific problem in the support section of our website.
Please let us know what you think of our products and services.
Visit our dedicated information section to learn more about MDPI.
JSmol Viewer
Parametric modeling and numerical simulation of a three-dimensional random aggregate model of lime–sand piles based on python–abaqus.
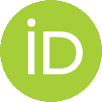
1. Introduction
1.1. research on mesoscopic model modeling, 1.2. research ideas and purposes, 2. meso-model modeling of lime–sand pile, 2.1. aggregate gradation test, 2.2. determination of basic parameters of aggregate, 2.3. the generation of aggregate, 2.4. delivery of aggregate, 3. numerical simulation of lime–sand pile meso-model, 3.1. determination and verification of microscopic parameters, 3.2. mesh generation of mesoscopic model, 3.3. static simulation analysis, 3.3.1. different mixing ratio simulation analysis, 3.3.2. simulation analysis of different heights, 4. conclusions and suggestions, author contributions, data availability statement, conflicts of interest.
- Chen, P.; Cui, Q. Application of Lime Soil Pile in Collapsible Loess Ground Treatment. Adv. Mater. Res. 2014 , 1049–1050 , 256–259. [ Google Scholar ] [ CrossRef ]
- He, Y.Q.; Zhu, Y.P. Theory and experiment of collapsible loess foundation treatment by expansion method. Civ. Build. Environ. Eng. 2009 , 31 , 44–48. [ Google Scholar ]
- Shabir, H.; Muhammad, F.; Ahmed, K.F.; Saeed, Z. Experimental evaluation of lime column as a ground improvement method in soft soils. SN Appl. Sci. 2021 , 3 , 799. [ Google Scholar ]
- Zhang, C.X.; Wang, J.L. Discussion on the effect of lime pile pre-melting treatment of island permafrost foundation. Low Temp. Build. Technol. 2022 , 44 , 167–170+174. [ Google Scholar ] [ CrossRef ]
- Yang, X.M.; Cheng, J.; Cai, H.C. Effect evaluation of application of quicklime pile composite foundation in island frozen soil area. J. Railw. Sci. Eng. 2022 , 19 , 941–948. [ Google Scholar ] [ CrossRef ]
- Wittmann, F.H.; Roelfstra, P.E.; Sadouki, H. Simulation and analysis of composite structures. Mater. Sci. Eng. 1985 , 68 , 239–248. [ Google Scholar ] [ CrossRef ]
- Liu, G.T.; Wang, Z.M. Numerical simulation of fracture of concrete materials by random aggregate model. J. Tsinghua Univ. (Nat. Sci. Ed.) 1996 , 1 , 84–89. [ Google Scholar ]
- Schlangen, E.; Van Mier, J.G.M. Simple lattice modle for numerical simulation of fracture of concrete materials and structures. Mater. Struct. 1992 , 25 , 239–248. [ Google Scholar ] [ CrossRef ]
- Walraven, J.C.; Reinhardt, H.W. Theory and experiments on the mechanical behavior of cracks in plain and reinforced concrete subject to shear loading. Heron 1991 , 26 , 26–35. [ Google Scholar ]
- Qin, W.P.; Yang, X.H.; Chen, C.Y. A fast random placement algorithm for three-dimensional convex concrete aggregate. Hydropower Energy Sci. 2006 , 3 , 39–42+99. [ Google Scholar ]
- Guan, Z.Q.; Gao, Q.H.; Gu, Y.X.; Song, C.; Shan, J. Establishment of three-dimensional finite element mesh model of composite mesostructure. Eng. Mech. 2005 , S1 , 67–72. [ Google Scholar ]
- Qin, W.; Du, C.B. Meso-level mechanical modeling of three-dimensional concrete based on CT slices. Eng. Mech. 2012 , 29 , 186–193. [ Google Scholar ]
- Avdeev, I.; Sobolev, K.; Amirjanov, A.; Hastert, A. Micromechanical Models of Structural Behavior of Concrete. MRS Online Proceeding Libr. Arch. 2010 , 20 , 1276–1290. [ Google Scholar ] [ CrossRef ]
- Zhu, L.; Dang, F.N.; Ding, W.H.; Xue, Y.; Zhang, L. Based on CT technology and gray level co-occurrence matrix theory, the mesoscopic damage evolution process of concrete under different loads is studied. J. Civ. Eng. 2020 , 53 , 97–107. [ Google Scholar ] [ CrossRef ]
- Yang, S.D.; Gao, Y.M.; Tao, Z.; Chen, W. Research on plastic damage coupling mechanical properties of concrete based on random aggregate model. J. Univ. South China (Nat. Sci. Ed.) 2023 , 37 , 47–52+94. [ Google Scholar ] [ CrossRef ]
- Sun, J.Y.; Xie, J.B.; Zhou, Y.; Zhou, Y. A 3D three-phase meso-scale model for simulation of chloride diffusion in concrete based on ANSYS. Int. J. Mech. Sci. 2022 , 219 , 107127. [ Google Scholar ] [ CrossRef ]
- Ma, B. Study on the Diffusion Characteristics of Sulfate Ions in Concrete Based on Random Aggregate Model. Master’s Thesis, Chongqing Jiaotong University, Chongqing, China, 2023. [ Google Scholar ]
- Liu, Y.T. Meso-Simulation Study on Compressive Properties of Recycled Concrete and Analysis of Influencing Factors. Master’s Thesis, Xi’an University of Technology, Xi’an, China, 2023. [ Google Scholar ]
- Wang, S.X. Study on the Size Effect of Compressive Strength of Fly Ash Geopolymer Concrete at High Temperature. Master’s Thesis, Xi’an University of Architecture and Technology, Xi’an, China, 2023. [ Google Scholar ]
- Liu, S.Y.; Miao, Y.C.; Li, M.H.; Selyutina, N.; Smirnov, I.; Liu, Y.; Zhang, Y. Numerical analysis of damage and deterioration of recycled thermal insulation concrete after high temperature. J. Taiyuan Univ. Technol. 2024 , 10 , 1–14. [ Google Scholar ]
- Wu, H.; Lu, S.Y.; Chen, D. Dynamic shear behavior of FRP-concrete bond interface based on concrete 3D mesoscopic model. Eng. Mech. 2024 , 41 , 1–16. [ Google Scholar ]
- Fang, Q.; Zhang, J.H.; Huan, Y.; Zhang, Y. Research on modeling method of three-dimensional mesoscopic model of fully graded concrete. Eng. Mech. 2013 , 30 , 14–21+30. [ Google Scholar ]
- Jiang, B.K.; Sun, W.J. Analysis of geometric model of recycled concrete random aggregate based on MATLAB programming language. Sichuan Cem. 2023 , 6 , 7–9+12. [ Google Scholar ]
- Cheng, S.H.; Ren, Z.G.; Li, P.P.; Shangguan, J. Numerical modeling of concrete three-dimensional random concave-convex aggregate based on LS-DYNA. J. Wuhan Univ. Technol. 2014 , 36 , 89–94+121. [ Google Scholar ]
- Tan, Y.W.; Wang, S.G.; Xu, F.; Liu, W.; Chen, X.; Ran, Y. The application status of COMSOL Multiphysics in the study of concrete durability. J. Silic. 2017 , 45 , 697–707. [ Google Scholar ] [ CrossRef ]
- Caballero, A.; López, C.M.; Carol, I. 3D meso-structural analysis of concrete specimens under uniaxial tension. Comput. Methods Appl. Mech. Eng. 2006 , 195 , 7182–7195. [ Google Scholar ] [ CrossRef ]
- Zhao, C.; Yang, Q.Y.; Zhong, X.G.; Shu, X.; Shen, M. Voronoi-RBSM coupling concrete mesoscopic modeling method. Eng. Mech. 2024 , 40 , 1–11. [ Google Scholar ]
- Guo, R.Q.; Ren, H.Q.; Zhang, L.; Long, Z.; Wu, X.; Li, Z. SHPB simulation study based on concrete meso-aggregate model. Vib. Impact 2019 , 38 , 107–116. [ Google Scholar ] [ CrossRef ]
- Xu, Q.; Zhou, X.S.; Cheng, Z.C. Random aggregate model and meso-mechanical analysis of concrete based on Ansys. J. Wuhan Univ. 2019 , 52 , 1035–1040+1047. [ Google Scholar ] [ CrossRef ]
- Jia, J.Y.; Wang, Z.R.; Xiao, K. Research on concrete crack propagation simulation system based on VC++ and ANSYS. Ind. Build. 2012 , 42 , 539–543+576. [ Google Scholar ] [ CrossRef ]
- Liang, J.; Lou, Z.K.; Han, J.H. Concrete aggregate modeling analysis based on AutoCAD. J. Water Conserv. 2011 , 42 , 1379–1383. [ Google Scholar ] [ CrossRef ]
- Cao, J.F.; Wang, X.C.; Kong, L. Application of Python Language in Abaqus , 2nd ed.; Machine Industry Press: Beijing, China, 2020; pp. 109–182. [ Google Scholar ]
- Qiao, Y.; Si, J.; Yuan, J.; Wang, Y.; Niu, X.; Ju, J.; Zhou, M.; He, L. Multi-Parameter Experimental Research on the Expansion Force Affecting Lime-Sand Piles under Preloading Pressure. Buildings 2024 , 14 , 1208. [ Google Scholar ] [ CrossRef ]
- Xu, Z.J. Monte Carlo Method ; Shanghai Science and Technology Press: Shanghai, China, 1985. [ Google Scholar ]
- Wu, Y.H.; Xiao, Y.X.; Xu, Y.F. Establishment of three-dimensional meso-random model of concrete based on Python-Abaqus. J. Comput. Mech. 2022 , 39 , 566–573. [ Google Scholar ]
- Kerner, E.H. The elastic and thermos-elastic properties of composite media. Proc. Phys. Society. Sect. B 1956 , 69 , 808. [ Google Scholar ] [ CrossRef ]
- Hu, L.P.; Chen, X.D.; Zhu, X.Y.; Chen, C. Mechanical properties analysis and discrete element simulation of steel slag pervious concrete with different aggregate sizes. Compr. Util. Fly Ash 2022 , 36 , 69–75+132. [ Google Scholar ] [ CrossRef ]
- Pei, X.J.; Zhang, F.Y.; Wu, W.J.; Liang, S.Y. Physicochemical and index properties of loess stabilized with lime and fly ash piles. Appl. Clay Sci. 2015 , 114 , 77–84. [ Google Scholar ] [ CrossRef ]
- Zhang, Z.Q.; Li, Y.L.; Zhu, X.Y.; Liu, X. Meso-scale corrosion expansion cracking of ribbed reinforced concrete based on a 3D random aggregate model. J. Zhejiang Univ. -Sci. A (Appl. Phys. Eng.) 2021 , 22 , 924–941. [ Google Scholar ] [ CrossRef ]
- Zhang, X.R.; Cheng, Y.H.; Wu, H. Analysis of dynamic compression behavior of concrete based on 3D mesoscopic model. Explos. Impact 2024 , 44 , 023102. [ Google Scholar ]
- Zhang, X.F.; Tian, Y.; Qin, P.; Xiao, T.; Wu, J. Mesoscopic simulation of uniaxial tension of concrete with deformed aggregate based on cohesion model. Hydropower 2022 , 48 , 73–77. [ Google Scholar ]
- Ke, Y.; Deng, D.; Gao, F.; Zheng, K. Application of meso-mechanical homogeneous model in the evaluation of mechanical and thermal performance parameters of shale ceramsite aggregate. Concrete 2017 , 10 , 32–36. [ Google Scholar ]
- Yu, Q.; Chen, Z.; Yang, J.; Rong, K. Numerical Study of Concrete Dynamic Splitting Based on 3D Realistic Aggregate Mesoscopic Model. Materials 2021 , 14 , 1948. [ Google Scholar ] [ CrossRef ]
Click here to enlarge figure
Lime Type | Name | CaO + MgO | MgO | CO | SO | |
---|---|---|---|---|---|---|
Siliceous lime | Siliceous lime90 | CL90-Q | ≥90 | ≤5 | ≤4 | ≤2 |
Type of Soil | Natural Water Content W (%) | Natural Density (g/cm³) | Plasticity Index | Compression Modulus (MPa) | Liquidity Factor | Shearing Strength | |
---|---|---|---|---|---|---|---|
C/kpa | Φ | ||||||
Loess | 18.8 | 1.75 | 12.8 | 13.46 | <0 | 30.0 | 23.0 |
Aggregate Type | Young’s Modulus (MPa) | Poisson’s Ratio | Natural Density (g/cm³) | Equivalent Coefficient of Linear Expansion (10 /°C) |
---|---|---|---|---|
Lime matrix | 8000 | 0.30 | 2.50 | 9.40 |
Sand aggregate | 10 | 0.20 | 1.50 | - |
Loess aggregate | 23 | 0.40 | 1.75 | - |
Aggregate Type | Grid Type | Grid Size | Element Number |
---|---|---|---|
Lime matrix | C3D4 | 3 mm | 634,800 |
Sand aggregate | C3D4 | 1 mm | 932,225 |
Loess aggregate | C3D4 | 1 mm | 233,056 |
Category | Experimental Value (kN) | Simulation Value (kN) | Relative Error/% |
---|---|---|---|
S-4:5:1 | 8.58 | 8.81 | 2.68 |
M-5:4:1 | 12.37 | 12.61 | 1.94 |
L-6:3:1 | 18.48 | 18.89 | 2.22 |
Groups | Experimental Value (kN) | Analogue Value (kN) | Relative Error/% |
---|---|---|---|
M-50 | 12.37 | 12.61 | 1.94 |
M-100 | 12.42 | 12.57 | 1.21 |
M-150 | 12.33 | 12.52 | 1.54 |
The statements, opinions and data contained in all publications are solely those of the individual author(s) and contributor(s) and not of MDPI and/or the editor(s). MDPI and/or the editor(s) disclaim responsibility for any injury to people or property resulting from any ideas, methods, instructions or products referred to in the content. |
Share and Cite
Yuan, J.; Si, J.; Qiao, Y.; Sun, W.; Qiao, S.; Niu, X.; Zhou, M.; Ju, J. Parametric Modeling and Numerical Simulation of a Three-Dimensional Random Aggregate Model of Lime–Sand Piles Based on Python–Abaqus. Buildings 2024 , 14 , 1842. https://doi.org/10.3390/buildings14061842
Yuan J, Si J, Qiao Y, Sun W, Qiao S, Niu X, Zhou M, Ju J. Parametric Modeling and Numerical Simulation of a Three-Dimensional Random Aggregate Model of Lime–Sand Piles Based on Python–Abaqus. Buildings . 2024; 14(6):1842. https://doi.org/10.3390/buildings14061842
Yuan, Jia, Jianhui Si, Yong Qiao, Wenshuo Sun, Shibo Qiao, Xiaoyu Niu, Ming Zhou, and Junpeng Ju. 2024. "Parametric Modeling and Numerical Simulation of a Three-Dimensional Random Aggregate Model of Lime–Sand Piles Based on Python–Abaqus" Buildings 14, no. 6: 1842. https://doi.org/10.3390/buildings14061842
Article Metrics
Article access statistics, further information, mdpi initiatives, follow mdpi.
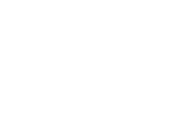
Subscribe to receive issue release notifications and newsletters from MDPI journals

IMAGES
VIDEO
COMMENTS
Systematic errors are much more problematic than random errors because they can skew your data to lead you to false conclusions. If you have systematic error, your measurements will be biased away from the true values.
Learn about the difference between systematic and random error. Get examples of the types of error and the effect on accuracy and precision.
Learn the difference between random error and systematic error in science experiments. Random error varies unpredictably, while systematic error is constant or proportional. See examples and sources.
Random errors are (like the name suggests) completely random. They are unpredictable and can't be replicated by repeating the experiment again. Systematic Errors produce consistent errors , either a fixed amount (like 1 lb) or a proportion (like 105% of the true value).
In performing experiments, try to estimate the e ects of as many systematic errors as you can, and then remove or correct for the most important. By being aware of
Random Errors. Random errors are due to fluctuations in the experimental or measurement conditions. Usually these errors are small. Taking more data tends to reduce the effect of random errors. Examples of Random Errors
This graph shows how the measurements randomly cluster around the true value of 10. They have no pattern. The red diamond is the average of the 30 data points, and it is pretty close to the correct value because the positive and negative errors cancel each other out.
The second type of errors that can occur during data collection are known as systematic errors. These are errors that occur due to two main reasons: 1. The instrument being used to take measurements is faulty. For example, suppose an electrician is measuring the voltage of batteries produced in a particular factory and the device that measures ...
Figure \(\PageIndex{5}\): Figure on left illustrates the deviation of an individual value from the mean (average), and on the right, the percent of the total number of measurements within one to three standard deviations from the mean.
This difference comes from both random sampling (random error) and not reporting black money (systematic error). The tendency not to report black money is a factor that systematically affects the sample mean income. Both random and systematic errors affect study results, but their effects are very different.
Statistical Treatment of Random Errors. Let's consider as an example the volume of water delivered by a set of 25 mL pipets. A manufacturer produces these to deliver 25.00 mL at 20°C with a stated tolerance of ±0.03 mL. A sample of 100 pipets is tested for accuracy by measuring the delivered volumes.
The magnitude of random errors depends partly on the scale on which something is measured (errors in molecular-level measurements would be on the order of nanometers, whereas errors in human height measurements are probably on the order of a centimeter or two) and partly on the quality of the tools being used.
or. dy − dx. - These errors are much smaller. • In general if different errors are not correlated, are independent, the way to combine them is. dz =. dx2 + dy2. • This is true for random and bias errors. THE CASE OF Z = X - Y. • Suppose Z = X - Y is a number much smaller than X or Y.
dealing with statistical errors, and most of the rest of this note will be concerned with them. Systematic errors arise from problems in the design of an experiment. They are not random, and tend to affect all measurements in some well-defined way. For example, suppose you are asked to read
Random Errors Random errors in experimental measurements are caused by unknown and unpredictable changes in the experiment. These changes may occur in the measuring instruments or in the environmental conditions. ... Random errors often have a Gaussian normal distribution (see Fig. 2). In such cases statistical methods may be used to analyze ...
Random errors are errors that shift your experimental measurement by a random amount each time. These can occur due to random fluctuations in experimental conditions or poor measurement practices on the researcher's part.
A random error, as the name suggests, is random in nature and very difficult to predict. It occurs because there are a very large number of parameters beyond the control of the experimenter that may interfere with the results of the experiment. Random errors are caused by sources that are not immediately obvious and it may take a long time ...
Systematic vs Random Errors in Physics | Part 3 of Physics Skills Guide. In Part 3 of the Beginner's Guide to Physics Practical Skills, we discuss systematic and random errors. Read examples of how to reduce the systematic and random errors in science experiments.
These errors are often classified into three main categories: systematic errors, random errors, and human errors. Here are some common types of experimental errors: 1. Systematic Errors. Systematic errors are consistent and predictable errors that occur throughout an experiment. They can arise from flaws in equipment, calibration issues, or ...
3 Experimental design; The goal of any good investigator is to minimise and quantify the errors whenever possible. To do this the investigator has to understand where and how the errors arise. This is the purpose of the evaluation. Errors or uncertainties may be broadly categorised as either random or systematic.
Learn about valuable skills for doing an experiment, like creating hypotheses, identifying risks, and measuring and recording data accurately. ... Random errors are errors made by the person ...
The random errors are those errors, which occur irregularly and hence are random. These can arise due to random and unpredictable fluctuations in experimental conditions ( Example : unpredictable fluctuations in temperature, voltage supply, mechanical vibrations of experimental set-ups, etc, errors by the observer taking readings, etc.
Random errors in experimental measurements are caused by unknown and unpredictable changes in the experiment. Learn its causes, examples, types and how to reduce it. ... Random errors in measurements are referred to as the difference between the observed and actual or true value of the measurement.
Each of these experiments modifies the headline or image associated with an article on Upworthy, as displayed when viewing a different focal article; the outcome is then clicks on these headlines. You may recall Upworthy as a key innovator in "clickbait" and especially clickbait with a particular ideological tilt. ... This is a random ...
API Reference#. This is the class and function reference of scikit-learn. Please refer to the full user guide for further details, as the raw specifications of classes and functions may not be enough to give full guidelines on their uses. For reference on concepts repeated across the API, see Glossary of Common Terms and API Elements.
Motivation: Missing values are often unavoidable in modern high-throughput measurements due to various experimental or analytical reasons. Imputation, the process of replacing missing values in a dataset with estimated values, plays an important role in multivariate and machine learning analyses. Three missingness patterns have been conceptualized: missing completely at random (MCAR), missing ...
Tree-based machine learning algorithms, such as random forest, have emerged as effective tools for estimating fine particulate matter (PM2.5) from satellite observations. However, they typically ...
A lime-sand pile is a three-phase particle composite material composed of a lime matrix, sand, and a loess aggregate at the meso level. Establishing a random aggregate model that can reflect the actual aggregate gradation, content, and morphology is the premise of numerical simulations of the meso-mechanics of lime-sand piles. In this paper, the secondary development of Abaqus 2022 is ...
The dataset was compiled from experimental work and literature sources, focusing on identifying critical parameters in the asphalt mix. Five distinct modeling methods—ANN, random forest (RF), SVM, random tree (RT), and bagging RT-based models—were employed to forecast the MS in these fibrous asphalt concrete mixes.