
- My Favorites

You have successfully logged in but...
... your login credentials do not authorize you to access this content in the selected format. Access to this content in this format requires a current subscription or a prior purchase. Please select the WEB or READ option instead (if available). Or consider purchasing the publication.
OECD Trade Policy Papers
Comparative advantage and trade performance.
NB. No. 1 to No. 139 were released under the previous series title OECD Trade Policy Working Papers .

- ISSN: 18166873 (online)
- https://doi.org/10.1787/18166873
- Subscribe to the RSS feed Subscribe to the RSS feed
Policy Implications
- Click to access:
- Click to download PDF - 780.04KB PDF

Cite this content as:
Author(s) Przemyslaw Kowalski i i OECD
05 Oct 2011
Revealed Comparative Advantage: What Is It Good For?
UNSW Business School Research Paper No. 2014-39B
47 Pages Posted: 19 Nov 2014 Last revised: 11 Feb 2017
Scott French
UNSW Australia Business School, School of Economics
Date Written: January 31, 2017
This paper applies a widely-used class of quantitative trade models to evaluate the usefulness of measures of revealed comparative advantage (RCA) in academic and policy analyses. I find that, while commonly-used indexes are generally not consistent with theoretical notions of comparative advantage, certain indexes can be usefully employed for certain tasks. I explore several common uses of RCA indexes and show that different indexes are appropriate when attempting to (a) uncover countries' fundamental patterns of comparative advantage, (b) evaluate the differential effect of changes in trade barriers across producers of different products, or (c) identify countries who are relatively close competitors in a given market.
Keywords: Relative productivity, index, Ricardian, trade barriers, trade policy, trade elasticity
JEL Classification: F10, F13, F14, F15
Suggested Citation: Suggested Citation
Scott French (Contact Author)
Unsw australia business school, school of economics ( email ).
High Street Sydney, NSW 2052 Australia
Do you have a job opening that you would like to promote on SSRN?
Paper statistics, related ejournals, unsw business school research paper series.
Subscribe to this free journal for more curated articles on this topic
International Trade eJournal
Subscribe to this fee journal for more curated articles on this topic
Econometric Modeling: International Economics eJournal
An Elementary Theory of Comparative Advantage
Comparative advantage, whether driven by technology or factor endowment, is at the core of neoclassical trade theory. Using tools from the mathematics of complementarity, this paper offers a simple, yet unifying perspective on the fundamental forces that shape comparative advantage. The main results characterize sufficient conditions on factor productivity and factor supply to predict patterns of international specialization in a multi-factor generalization of the Ricardian model to which we refer as an "elementary neoclassical economy." These conditions, which hold for an arbitrarily large number of countries, goods, and factors, generalize and extend many results from the previous trade literature. They also offer new insights about the joint effects of technology and factor endowments on international specialization.
I thank Pol Antras, Vince Crawford, Gene Grossman, Gordon Hanson, Navin Kartik, Giovanni Maggi, Marc Melitz, David Miller, Marc Muendler, Jim Rauch, Esteban Rossi-Hansberg, Jon Vogel, and seminar participants at many institutions for helpful comments and discussions. This project was initiated at the department of economics at UCSD and continued at the Princeton International Economics Section, which I thank for their support. A previous version of this paper was circulated under the title: "Heterogeneity and Trade". The views expressed herein are those of the author(s) and do not necessarily reflect the views of the National Bureau of Economic Research.
MARC RIS BibTeΧ
Download Citation Data
Published Versions
More from nber.
In addition to working papers , the NBER disseminates affiliates’ latest findings through a range of free periodicals — the NBER Reporter , the NBER Digest , the Bulletin on Retirement and Disability , the Bulletin on Health , and the Bulletin on Entrepreneurship — as well as online conference reports , video lectures , and interviews .
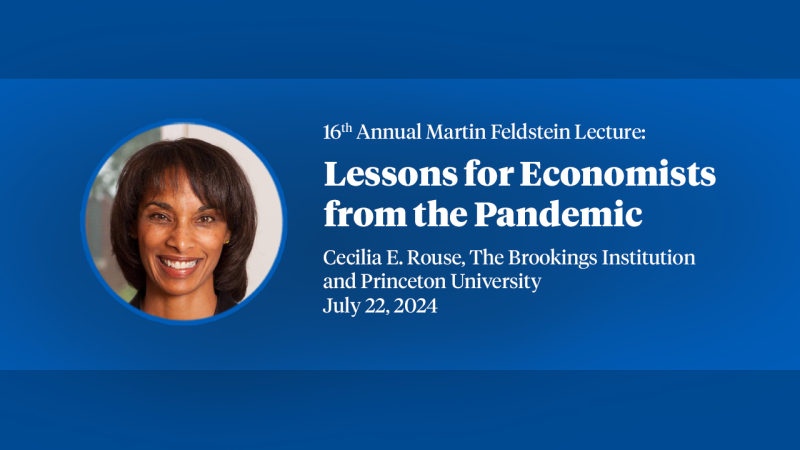
AI-based detection and identification of low-level nuclear waste: a comparative analysis
- Original Article
- Open access
- Published: 22 August 2024
Cite this article
You have full access to this open access article
- Aris Duani Rojas ORCID: orcid.org/0009-0008-8019-5376 1 ,
- Leonel Lagos 3 ,
- Himanshu Upadhyay 2 ,
- Jayesh Soni 4 &
- Nagarajan Prabakar 1
106 Accesses
Explore all metrics
Ensuring environmental safety and regulatory compliance at Department of Energy (DOE) sites demands an efficient and reliable detection system for low-level nuclear waste (LLW). Unlike existing methods that rely on human effort, this paper explores the integration of computer vision algorithms to automate the identification of such waste across DOE facilities. We evaluate the effectiveness of multiple algorithms in classifying nuclear waste materials and their adaptability to newly emerging LLW. Our research introduces and implements five state-of-the-art computer vision models, each representing a different approach to the problem. Through rigorous experimentation and validation, we evaluate these algorithms based on performance, speed, and adaptability. The results reveal a noteworthy trade-off between detection performance and adaptability. YOLOv7 shows the best performance and requires the highest effort to detect new LLW. Conversely, OWL-ViT has lower performance than YOLOv7 and requires minimal effort to detect new LLW. The inference speed does not strongly correlate with performance or adaptability. These findings offer valuable insights into the strengths and limitations of current computer vision algorithms for LLW detection. Each developed model provides a specialized solution with distinct advantages and disadvantages, empowering DOE stakeholders to select the algorithm that aligns best with their specific needs.
Similar content being viewed by others
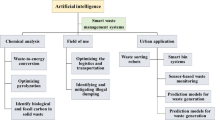
Artificial intelligence for waste management in smart cities: a review
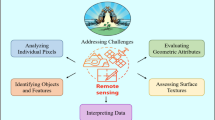
Environmental resilience through artificial intelligence: innovations in monitoring and management
Computer Vision-based Waste Detection and Classification for Garbage Management and Recycling
Explore related subjects.
- Artificial Intelligence
Avoid common mistakes on your manuscript.

1 Introduction
Low-level nuclear waste (LLW) is nuclear waste that is not high-level radioactive waste, spent nuclear fuel, or byproduct materials [ 1 ]. LLW is defined by exclusion, and it is a category for radioactive wastes that do not belong into any other category. As such, at any point in time, there could be a new kind of low-level waste, and that makes the management of low-level nuclear waste a complex task involving several considerations on its detection, identification, transportation, and disposal [ 2 ]. In order to detect and identify LLWs safely and efficiently, there is a need to automate the detection and identification process for both the current and future LLWs.
The usage of deep learning for computer vision tasks like object detection has become increasingly popular with the rise of high-performance computing [ 3 ]. In recent years, there have been many algorithms developed for related tasks like object detection, instance segmentation, semantic segmentation, depth-based instance segmentation, one-shot object detection, among others. Of these tasks, object detection, instance segmentation, and semantic segmentation are closely related. All three tasks require large amounts of labeled data to learn how to detect different objects. However, object detection outputs bounding boxes, class names, and confidence scores while instance segmentation outputs pixel masks, class names, and confidence scores. On the other hand, semantic segmentation outputs pixel masks that do not separate the different instances of the objects. Depth-based instance segmentation is very similar to instance segmentation. However, instead of using color images, it uses disparity images, which provides some advantages discussed in Sect. 4 . Lastly, One-Shot Object Detection does not require large amounts of labeled data to detect different objects, it only requires data to learn how to detect objects in general. The idea behind One-Shot Object Detection is that a query image representing the object to detect is given, and the model outputs bounding boxes and confidence scores for the detection on another image, the target image. However, deep learning computer vision algorithms in general have worse performance when compared to humans, who can do all kinds of computer vision tasks on known and new objects with the least amount of context or reference for the object possible.
The purpose of this paper is to evaluate the performance of different deep learning models on LLW detection and identification and assess their strengths and weaknesses when compared to each other. We aim to evaluate the effectiveness of different computer vision models in detecting and identifying current LLWs, as well as their adaptability to new LLWs. An accurate assessment of deep learning computer vision algorithms for LLWs will allow for better decision making in the automation process of waste stream management.
2 Related work
Currently state-of-the-art LLW detection is focused on object detection and segmentation. YOLO [ 4 ], Faster R-CNN [ 5 ], SSD [ 6 ], and custom CNNs comprised most of the object detection algorithms currently being used. On the other hand, custom CNNs, Mask R-CNN [ 7 ], DeepLab [ 8 ], and U-Net [ 9 ] comprised most of the segmentation algorithms currently being used.
The top three object detection algorithms in use (YOLO, Faster R-CNN, and SSD) take different approaches to object detection. Nevertheless, they share many properties like low inference speed, high mean average precision, and good generalization. [ 10 ] trained SSD and Faster R-CNN on the TrashNet dataset [ 11 ], and then they fine-tuned the resulting models on a custom dataset to obtain state-of-the-art results on LLW detection and identification. However, [ 12 ] showed that YOLOv5 performs better than Faster R-CNN and EfficientDet-D2 on the TrashNet dataset. And [ 13 ] explored the performance between multiple YOLO algorithms and found that YOLOv7 had the best performance on the ZeroWaste dataset [ 14 ], even better than YOLOv5, which is understandable since YOLOv7 builds upon YOLOv5. Furthermore, [ 15 ] used a modified YOLO architecture to improve upon the results of YOLO, SSD, and Faster R-CNN. Advancements in recent years with Transformers, specifically vision transformers, has resulted in transformers being applied to waste object detection where [ 16 ] showed that vision transformers outperform Faster R-CNN and DETR on their custom dataset containing small objects like batteries, bottle caps, nut shells, etc.
The top three segmentation algorithms in use (custom CNNs, Mask R-CNN, and DeepLab) are quite different from each other. Mask R-CNN is an instance segmentation algorithm; while, DeepLab is a semantic segmentation one, and the custom CNNs show a mix of semantic and instance segmentation algorithms. For example, [ 17 ] used Mask R-CNN to detect underwater waste through instance segmentation while [ 18 ] used Mask R-CNN to detect objects in construction sites which are then picked up by a robot. [ 18 ] used DeepLabv3 with a ResNet-101 backbone to improve detection on Trash Annotations in Context (TACO) dataset [ 18 ].
Beyond object detection and instance segmentation, some work has been done on other computer vision tasks for LLW detection and identification that make use of different cameras and positioning. [ 19 ] used a mix of custom CNNs and VGG16 to take as input an RGBD image and output a semantic segmentation mask for the waste in the image. The model developed in this work benefits from the extra depth information contained within the RGBD image that provides the model a stronger signal about the objects in the scene than a RGB image. Also, [ 20 ] used a ResNet50 backbone with Fully Connected Layers to classify LLW into different categories like vinyl, paper, rubber, cotton, no object, etc. However, when the system was deployed, the camera that took the images was capable of zooming in onto any given object allowing the image classification algorithm to behave more like object detection.
3 Research methodology
3.1 deep learning workflow.
Computer vision through deep learning algorithms requires large amounts of data for the models to understand which features from the input images are important. In the identification and detection of LLW, there is no guarantee that the available data will be enough to train the deep learning models or that it will have enough variance to allow for high quality feature extraction. Transfer learning is a popular tool in the field of deep learning that uses large amounts of data available on similar tasks/domains to improve the performance on another task/domain that has less data available. This work uses fine-tuning, a type of transfer learning, to decrease the data requirements for LLW detection and identification. The computer vision algorithms in this work can be split into two sections: feature extraction and feature projection, where the feature extraction converts an input image into a tensor that computers can understand containing a representation of the image. And the feature projection maps that tensor into a desired output like a bounding box, a pixel mask, etc. By leveraging the knowledge acquired through pre-trained feature extraction, we aim to overcome the need for large amounts of LLW data and enhance the models’ ability to learn how to detect new objects with minimal costs in terms of labeling, training, and testing. Figure 1 illustrates the complete deep learning workflow used in this work.
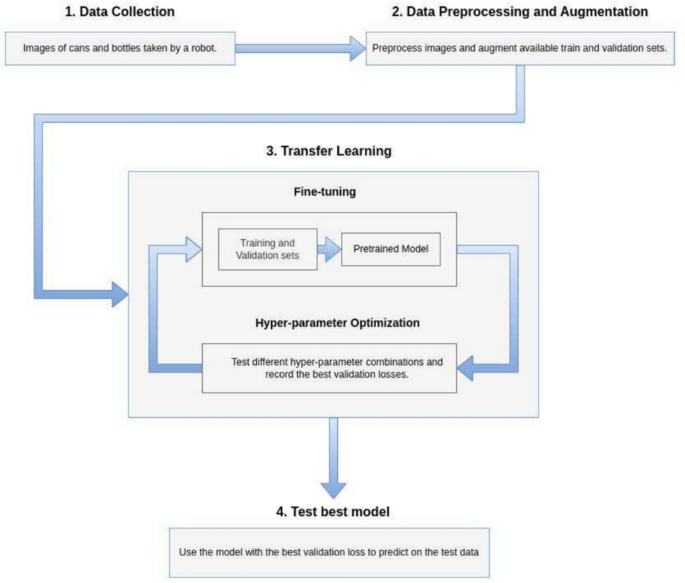
Deep learning workflow for training models
We explore multiple state-of-the-art algorithms for different computer visions tasks like YOLOv7 [ 21 ] for Object Detection and Instance Segmentation, STEGO [ 22 ] for Unsupervised Semantic Segmentation, SD Mask R-CNN [ 23 ] for Depth Based Instance Segmentation, and OWL-Vit [ 24 ] for One-Shot Object Detection. This is done to understand which computer vision architecture and task is best at detecting LLW, where the main point is that the set of objects that are considered LLW is constantly increasing. The results of this study provide insight into the effectiveness these algorithms in learning to detect new objects.
3.2 Data collection
The algorithms studied in this work use two different input formats: 3-channel and 1-channel images. The 3-channel images use red, green, and blue representations of the images and the 1-channel images use the depth representation of the images where objects that are closer to the camera have higher values than objects that are farther away from the camera. For pre-training, the dataset used for the algorithms that take a 3-channel images as input was Common Objects in Context (COCO) and the dataset used for algorithms that take a 1-channel image as input is Warehouse Instance Segmentation Dataset for Object Manipulation (WISDOM-Real).
For fine-tuning, the dataset used is made up of images of cans, bottles, and other miscellaneous items taken by the camera of a robot as shown in Fig. 2 , where the 3-channel and 1-channel input images are taken by different cameras. In this case, the pre-training datasets are a placeholder for all the current LLWs, and the custom datasets are a placeholder for a new LLWs. The evaluation is focused on how easily and effectively the models can adapt to the custom datasets or new LLWs.
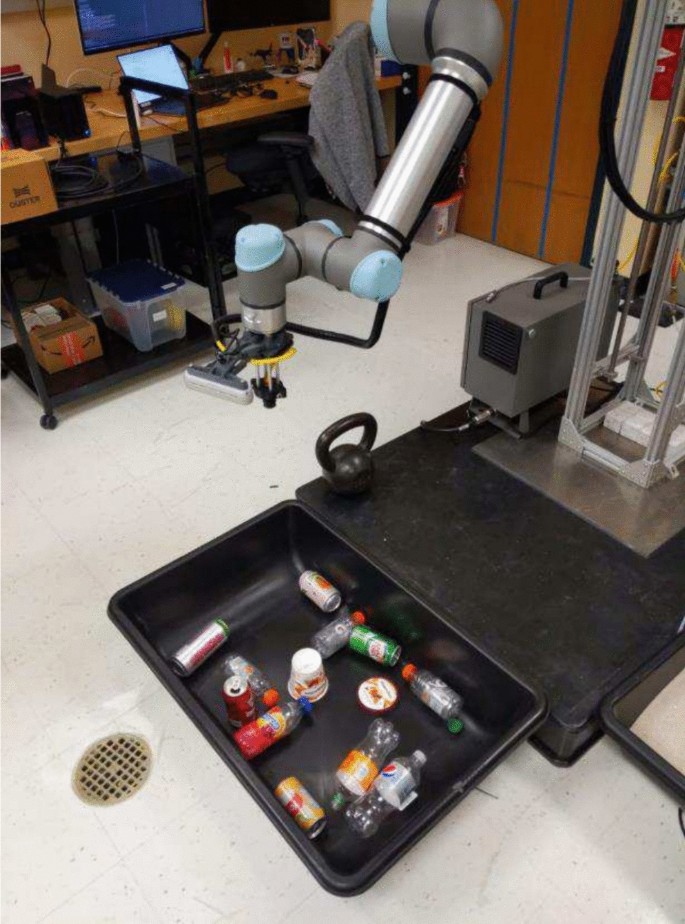
Robot arm which captures the images used in the custom dataset
3.3 Data preprocessing
Prior to training, all datasets are preprocessed to transform the input and improve the models’ performance. The preprocessing consists of resizing the images, normalizing the pixels to a uniform distribution, as well as several data augmentations. The data augmentations include rotations, flips, crops, zoom-in, zoom-out, changes in saturation, among others. All these techniques increase the available data while exposing the models to realistic scenarios they are likely to encounter when deployed in the real world.
3.4 Model architecture
In this section, we discuss the different complexities of the approaches taken by each of the chosen models: YOLOv7, STEGO, SD Mask R-CNN, and OWL-ViT.
YOLOv7 is a real time object detector based on the original YOLO architecture. The YOLO architecture divides an input image into an S × S grid. It passes each image patch through a feature extractor composed of Convolutional and Pooling layers, and then it passes the features through a feature projector composed of Fully Connected Layers. For an input image I , YOLO works in the following manner:
where g (·) is the feature projector and f (·) is the feature extractor. Most computer vision algorithms follow the architecture idea from Eq. 1 .
The feature extraction in the original YOLO algorithm is given by:
And this is for L hidden layers, a matrix of weights W for each layer l ∈ L and a tensor output z for each layer l ∈ L , and then i,j are indices of the convolution or pooling window and n,m are indices of the output tensor.
The feature mapping in the original YOLO algorithm is given by:
where for a given layer l , W ( l ) is the matrix of weights, z ( l −1) is the vector output of the previous layer, b ( l ) is the vector of biases and ϕ (·) is the activation functions of the layer. This is repeated across L mapping of the features until the final output.
For the object detection case, the output of the network is a tensor given by S × S × ( B ·5 + C ), where for each of the S × S grids, the network outputs the x,y,w,h and confidence of the predictions as well as which class it is among C classes. For the instance segmentation case, the output of the network is similar in that it outputs bounding boxes with a given class, and it also outputs prototype masks and mask weights that are used to construct the pixel mask for a given bounding box such that:
where W ( i ) is i th mask weight and proto mask ( i ) is the i th prototype mask for each x, y coordinate in a mask and for each grid from the S × S grid.
From the original YOLO architecture to YOLOv7 there have been many changes including the addition of the instance segmentation described above as well as anchors and non-maximum suppression. Specifically to YOLOv7, there is the addition of E-ELAN based on Efficient Long-Attention Networks (ELAN) whose goal is to enhance the learning ability of the model without increasing the gradient path during backward propagation as well as the addition of model scaling capabilities which allow the model to adjust its width and depth during concatenation to reach different inference speeds. Furthermore, there is the addition of an auxiliary head that makes coarse predictions that support the lead head predictor which makes the fine prediction used as the output of the network. These changes and many others improve the performance of the previously described YOLO architecture. Nevertheless, the major concepts of the architecture have remained the same.
STEGO is an unsupervised semantic segmentation algorithm that can learn how to detect objects without any ground truth labels. This is achieved by computing the feature correspondence between the features of two images. The feature correspondence F is given by:
For C channels and feature tensors f ∈ R CHW and g ∈ R CIJ .
The STEGO algorithm uses a vision transformer to extract the features tensors which are used to calculate the aforementioned feature correspondences as well as given as input to the segmentation head of the architecture, which is composed of Linear layers. The goal of the loss function is that similar feature correspondences should have closer segmentation outputs.
During training, STEGO passes as input an image with its closest KNN neighbor, itself, as well as a random image that does not have any correlation with the input image. Figure 3 shows the STEGO architecture where an image passes through a feature extractor, and those features are passed through linear and cluster probes. The loss function of the feature extractor is given by:
where L(·) describes the loss function that matches similar feature correspondences with closer segmentation predictions and λ and b control how the weight of the learning signals for the different image pairs.
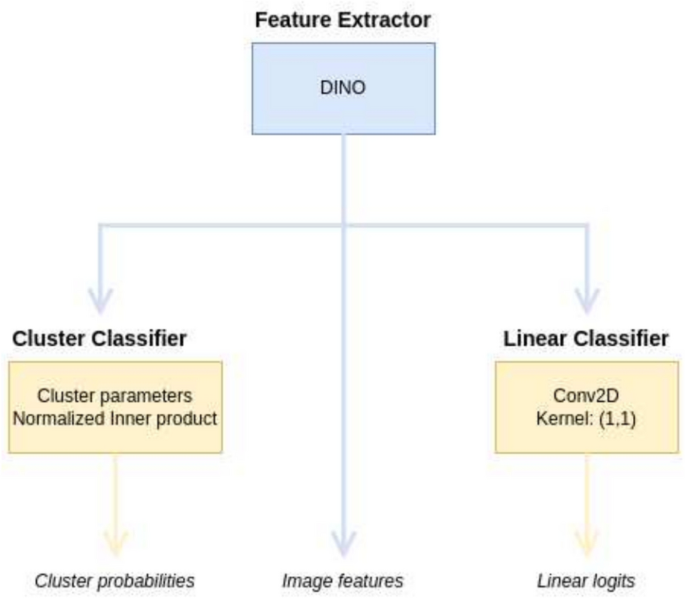
Illustration of the STEGO architecture
The loss function of the cluster probe is given by:
where P c is the probability that the given feature belongs to cluster c and V c is the inner product between the normalized cluster parameters and the normalized features.
The loss function of the linear probe is the cross-entropy loss between the linear probe logits and the labels logits. The linear probe network is used to provide information about the quality of the features created by the feature extraction; It does not influence the loss of the feature extractor or the cluster probe.
During prediction, the STEGO algorithm takes an input image and passes it through the vision transformer and the segmentation head. The resulting segmentation embeddings are clustered and then refined through a Conditional Random Field (CRF) [ 25 ] as shown in Fig. 3 . The goal of the CRF is to refine the pixel class predictions specially around the edges and color-correlated regions of the image.
Synthetic Depth Mask R-CNN is a depth-based instance segmentation algorithm based on the Mask R-CNN architecture. The Mask R-CNN architecture is a two stage process consisting of Region Proposal Generation and Object Detection and Classification. The Region Proposal step works is carried out by a backbone feature extractor followed by a Region Proposal Network that outputs bounding boxes and the likelihood the bounding boxes contain an object. During the Object Detection and Classification stage, for each region of interest, Mask R-CNN outputs a bounding box, an object class, and binary pixel masks in parallel, where the mask predictions are of the shape C × m × m for C classes and mask resolution of m × m . During training, the loss function is given by:
Where \({L}_{mask}\) is the binary cross-entropy between the c-th mask and the ground truth segmentation for an object of class c . Specifically for SD Mask R-CNN, the input is a 1-channel depth image that is triplicated across 3-channels to match the expected input format of Mask R-CNN. The number of classes C = 2 to detect foreground versus background objects, where the foreground objects are the only objects of interest. And lastly, the feature extraction backbone was changed from ResNet-101 to ResNet35 to greatly enhance training and inference speeds while maintaining great performance in instance segmentation.
OWL-ViT is both a One-Shot and Zero-Shot Object Detection algorithm. In this work, OWL-ViT is implemented and used a One-Shot Object Detection algorithm. The first phase of OWL-Vit consists of training Vision Transformer encoder to extract the features of an input image. The algorithm uses a contrastive loss over images in a batch to create image features that are similar when the input images refer to the same object and different when the input images refer to different objects.
Figure 4 illustrates the second phase of training, which is also how the model does predictions. In this phase, OWL-ViT receives a query and a target image as input. Both inputs are passed through the Vision Transformer encoder which extracts the features from the images. The features from the target image are passed through a Linear Layer to obtain the likelihood of a specific object being present and through Multi-Layer Perceptron Layer to obtain the bounding boxes each possible object class. The features from the query image are used to determine which possible objects should be detected, and from that, the bounding boxes that the model should output.
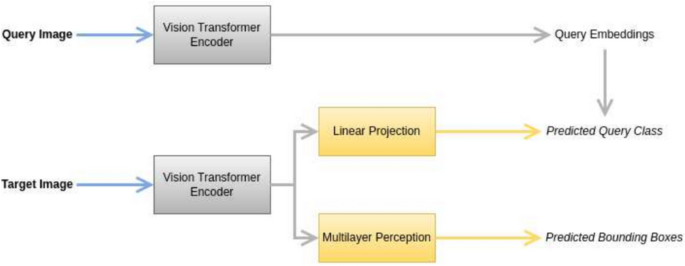
Illustration of the second phase of training of OWL-VitT
3.5 Model training
The training process is the main step in deep learning, where the weights that provide an optimal function approximation to the dataset are found. This section outlines the steps taken during the training of each model, which includes transfer learning, weights optimization, hyperparameter optimization, and evaluations on validation sets.
Each of the object detection models discussed in this paper can be split into a feature extraction backbone and a projection head. For transfer learning, the feature extraction layers were frozen; while, the projection heads were trained using an Adam optimizer to optimize the weights based on the gradients.
The hyper-parameters of the model can and do have a great impact on its final performance. An example of this is the batch size, where a batch size that is too small can cause the model to fail to converge to a stable loss while a batch size that is too high can cause overfitting. There is no extract theoretical framework to determine the best batch size for a given dataset and architecture. As such, all the models were trained using random search to find the best hyperparameters. The hyper-parameters explored were learning rate, input image size, batch size, number of epochs, weight decay, as well as model specific hyper-parameters like number of neighbors for STEGO, and IoU threshold for YOLOv7 and OWL-ViT.
In order to allow for a batch size greater than the free space available in the GPU, gradient accumulation was used for all batch sizes. During the standard training process, the neural network predicts \(\widehat{y}\) for a batch, and the loss is computed with respected to the ground truth y . After that, backward propagation is performed to compute the gradients and update the weights of the neural network based on those gradients. In gradient accumulation, the weights of the neural network are not updated after every batch. Instead, after each batch prediction the gradients are computed and added to the gradients of the neural network, and the weights are updated after several gradient computations. This way, the models are trained as if there was unlimited GPU memory and it allowed for the exploration of their performance across a large range of batch sizes.
Then, after each epoch, the performance of the models was evaluated on the validation set, which consisted of 20% of the training data available. The best models were chosen based on the lowest loss for the validation set among all epochs of training.
3.6 Evaluation metrics
Assessing the performance of different models and algorithms is crucial for building upon current solutions to create new ones. With respect to object detection and similar computer vision tasks, the main metric used in mean Average Precision (mAP). Given a model’s prediction of a bounding box or a pixel mask, it is possible to calculate the Intersection over Union (IoU) given by:
This computes the area of intersection between the prediction and the ground truth and then divides by the total area covered by the prediction and the ground truth. This encourages large overlaps between predictions and the ground truth since it increases and the numerator and encourages small differences in the size of the prediction and the ground truth since it decreases the denominator.
Then, it becomes possible to define more metrics that resemble the ones using in image classification:
True Positive (TP): Predictions with IoU ≥ t.
False Positive (FP): Predictions with IoU < t.
False Negative (FN): No predictions for a ground truth.
True Negative (TN): No predictions for the background (image without ground truths).
Where the value for True Negatives is a large finite value because it would include every possible bounding box or pixel mask that can be created without including the ground truths in the image.
Then, using these new metrics, it is possible to calculate:
where TP + FP is the same as all predictions made by the model and TP + FN is the same as all ground truths in the image.
Then, for a sequence of images I i for i = 1 , 2 , 3 ,…,N , and for each prediction P j i belonging to the image I i and for j = 1 , 2 , 3 ,…,k , it is possible to determine if the prediction P j i is a true positive or a false positive. Afterward, the predictions are sorted by order of confidence, from which the precision and recall are computed. The area under the precision–recall curve is the average precision of the model. Then, for multiple classes of objects, the average precision is computed, and the average of those values are taken to determine mean average precision (mAP) used in the evaluations in this paper.
While quantitative metrics are useful to assess the capabilities of a model, these metrics do not properly capture the goal of object detection and similar computer vision tasks. That is, given two detectors that make predictions as illustrated in Figs. 5 and 6 , the two detectors have the same mAP since the area under the Precision–Recall curve (AP) in both cases is 1 for all classes, which means the mAP is 1 in both detections. However, the detection in Fig. 5 closely matches what is desired from a detector and also matches the kinds of detections a human would make. On the other hand, the detection in Fig. 6 does not make correct predictions and those predictions do not match how humans would detect objects, yet, by the mAP metric, its detections are considered as good as the first detector. As such, it is important to consider both quantitatively and qualitatively measures of performance.
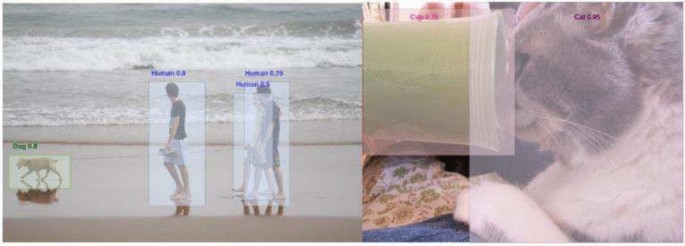
Illustration of the results of a good object detector
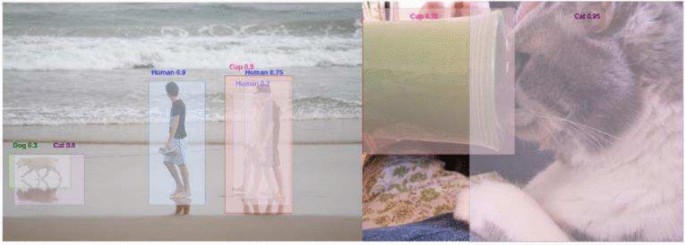
Illustration of the results of a bad object detector
4 Results and discussion
In this section, the performance of each model—YOLOv7, STEGO, SD Mask R-CNN, and OWL-ViT—is compared with the detection of current LLW and future LLW. The total dataset is divided into training, validation, and testing sets in the following manner: The dataset contained 38 images in total, where the images used for training and validation were augmented (using the data augmentations described in Sect. 3.3 ) to increase the samples within those sets by a significant amount. Then, these augmented datasets were used to train and validate the performance of the hyper-parameters of each model. The percent split between training, validation, and testing sets is shown in Table 1 .
While the size of the dataset is small, its purpose is to fine-tune to the models, where a smaller amount of data are needed than what is common for training deep learning models. The idea is to comparatively evaluate the effectiveness of algorithms for learning to detect new objects. As such, if an algorithm does perform better than another with N images, there is an expectation that it would still perform better than another with kN images where k is a positive number. Granted, the metrics will improve as the size of the dataset increases, but the comparative results remain the same.
Table 2 shows the results for the best hyper-parameters found for each algorithm, where these are the hyperparameters shared across all models. However, hyperparameter optimization was carried out for algorithm specific hyper-parameters, too. For example, the number of k-nearest neighbors and the values of λ self , λ knn , λ rand were among some of the hyper-parameters changed in the STEGO architecture. Similarly, SD Mask R-CNN had hyper-parameters about the mean of the pixels in the images and the expected maximum number of predictions that should be made.
Then after each model was trained with these hyperparameters, they were evaluated on the testing set. Figure 7 shows the mAP of YOLOv7-det which is the object detection version of YOLOv7, YOLOv7-seg which is the instance segmentation version of YOLOv7, STEGO, SD Mask R-CNN, and OWL-ViT on the testing set.
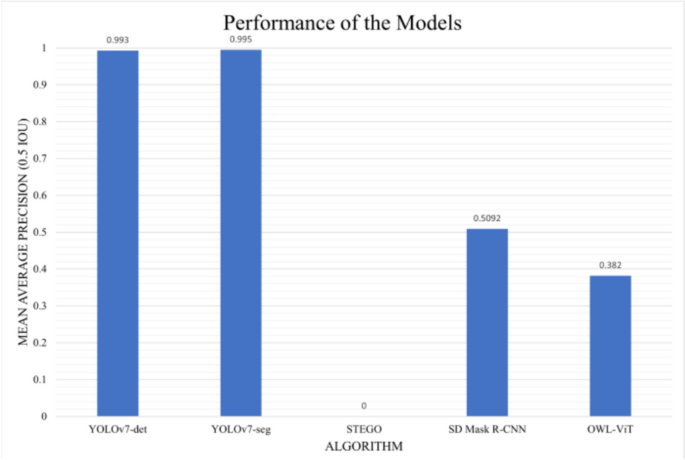
mAP of YOLOv7, STEGO, SD Mask R-CNN, and OWL-ViT on the testing set
Analyzing these results, YOLOv7 for instance segmentation has the best performance at detecting the cans and bottles, reaching a mAP of 0.995. This is closely followed by YOLOv7 for object detection which reached a mAP of 0.993. The third best mAP of 0.5092 was obtained by SD Mask R-CNN on a different dataset since the input images to the model are disparity images and not the RGB images given as input to the other models. The fourth best mAP was reached by OWL-ViT with a value of 0.382 without any training at all. Lastly, STEGO has a mAP of 0. However, this is because the mean average precision was computed at an intersection over union threshold of 0.5, which means that if the predicted masks do not have enough intersection with the ground truths, the predictions are not counted as a true positive. This was an issue for STEGO where because when the cans and the bottles are close to each other, it predicts a single continuous mask to cover all of them, which makes the intersection over union with any given ground truth low despite the predictions being quite accurate in some cases. This is one of the reasons why a qualitative analysis of the performance of the algorithms is needed.
When detecting LLWs in real time, inference speed is also quite important. Scripts that do just inference were implemented using each of these models and were then tested on an Intel CPU with 2.40 GHz for 10 images, and the average inference speed per image was computed. The procedure was repeated 5 times and the number of seconds taken per prediction were averaged. The inference time of the models was tested on a CPU because the computer vision models would be integrated with robot arms that can collect the identified LLW, which means the models must be able to run on hardware that does not have a GPU. Figure 8 displays the results obtained where YOLOv7-det which is YOLOv7 for object detection was the fastest model followed by YOLOv7 for instance segmentation with a 1.24 and 1.96 s inference time, respectively.
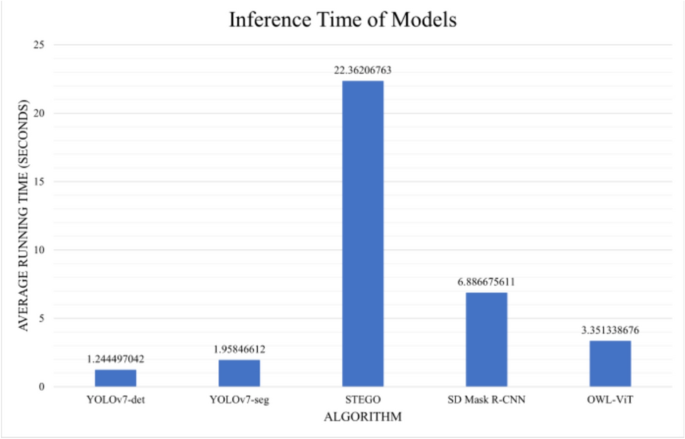
Average inference time of YOLOv7, STEGO, SD Mask R-CNN, and OWL-ViT on CPU
It is worth noting that even though both OWL-ViT and STEGO use vision transformers as their backbone feature extractor, OWL-ViT is significantly faster than STEGO and even faster than SD Mask R-CNN which uses a ResNet backbone. OWL-ViT had an inference speed of 3.35 s; while, STEGO and SD Mask R-CNN had an inference time of 22.36 and 6.89 s, respectively. However, this does not assess the algorithms’ adaptability. That is, the mean average precision and inference speed show the expected performance of each of these algorithms on a known LLW. However, this does not show the expected performance on any new LLW that would emerge. The steps taken to prepare the custom dataset into a format that each algorithm can use will be used as an assessment of the models’ effectiveness when learning to detect new LLWs.
That is, in the case of YOLOv7 for both object detection and instance segmentation, after the images were taken, they had to be labeled with bounding boxes or masks where each label required a human annotator. Then, after labeling, the model had to be trained to detect the new objects. This means that to allow YOLOv7 to detect a new LLW, large amounts of pictures must be taken, annotated, and then the model must be carefully trained to increase the performance on the new objects without losing performance on the previous objects.
Figure 9 shows the results from YOLOv7 for object detection which are quite excellent. It captures the bounding box for each object class and does not have false positive detections with the miscellaneous objects interspersed within the bin.
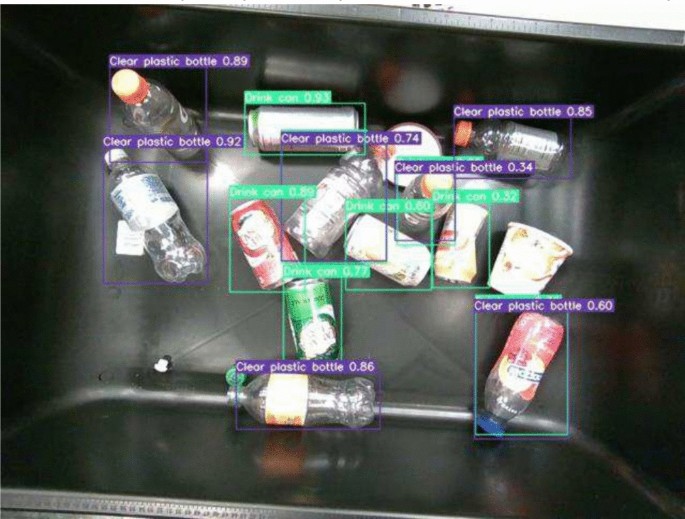
Example prediction from YOLOv7-det
As seen in Fig. 10 , the results from YOLOv7 for instance segmentation are similarly as good as the results for object detection, where the pixel masks closely wrap around the given objects. And the confidence on a prediction for instance segmentation is higher than the confidence for a prediction in object detection. In these two cases, the mean average precision and the quality of the predictions to the human eye are both high, which is a good indicator of a strong detection algorithm.
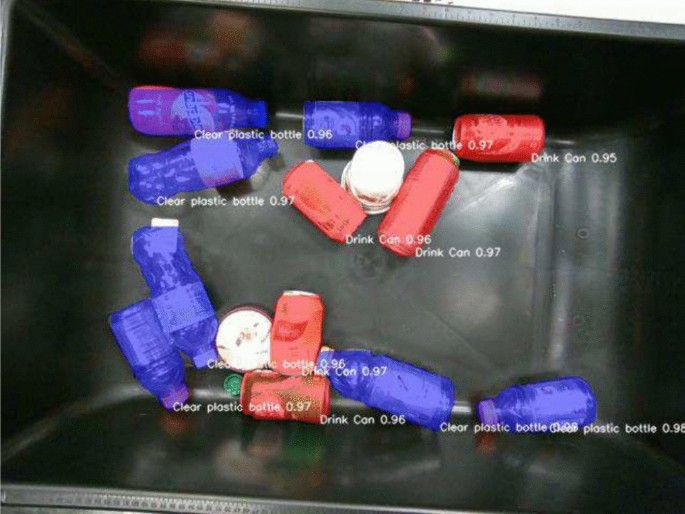
Example prediction from YOLOv7-seg
Then, for STEGO, after the images were taken, no labeling had to be done. The model is simply trained on the images in a self-supervised fashion. However, hyperparameter tuning is quite important and finding the best number of nearest neighbors requires human intuition about what the setting and what the objects are expected to be present in it. As such, to allow STEGO to detect a new LLW, large amounts of images must be taken—the annotation step is not needed—and then the model must be carefully trained to add the objects to a new cluster and not to a previously existing cluster.
As seen in Fig. 11 , the results from STEGO are much better than the mean average precision at 0.5 intersection over union would indicate. This is because, for each image, STEGO predicts large pixel masks. These large pixel masks encompass multiple ground truth objects at the same time. As such, when computing the area of the intersection between a large pixel mask and any given ground truth object it contains, the area is approximately the area of the ground truth object. However, when computing the union between the large pixel mask and any given ground truth object, the area is approximately the area of the pixel mask. Since the pixel mask encompasses multiple objects, the IoU for any given prediction is approximately the area of the object divided by the area of multiple objects (encompassed by the pixel mask), which is certainly less than the 0.5 threshold. The only way to remedy this issue is by applying another deep learning algorithm or computer vision technique to detect the edges and split the predicted large pixel masks into pieces. However, ideally, STEGO would not need that post-processing step and correctly predict a pixel mask per ground truth object and not a pixel mask per many ground truth objects.
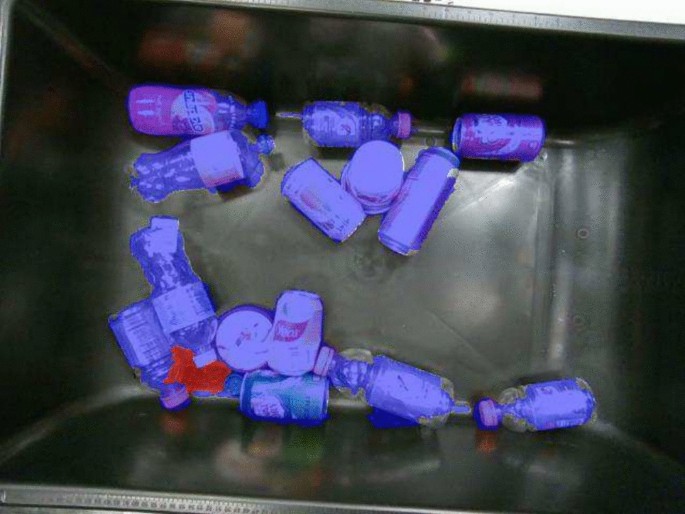
Example prediction from STEGO
In Fig. 11 , the blue color shows the pixel mask output from STEGO, and the red color indicates holes formed by the polygons created using the blue pixel masks. Some of the miscellaneous objects in the bin are thought of as part of the cans and bottles, and some of the pixel masks do not fully cover the target objects.
On the other hand, for SD Mask R-CNN, there is no need to take any images at all. Instead, it is possible to create a 3D mesh of the new LLW and simulate dropping the object into a bin at different orientations and locations. Figure 12 illustrates this principle, where the bin can be at different orientations and the objects can lie with different sides facing upwards and at different locations.
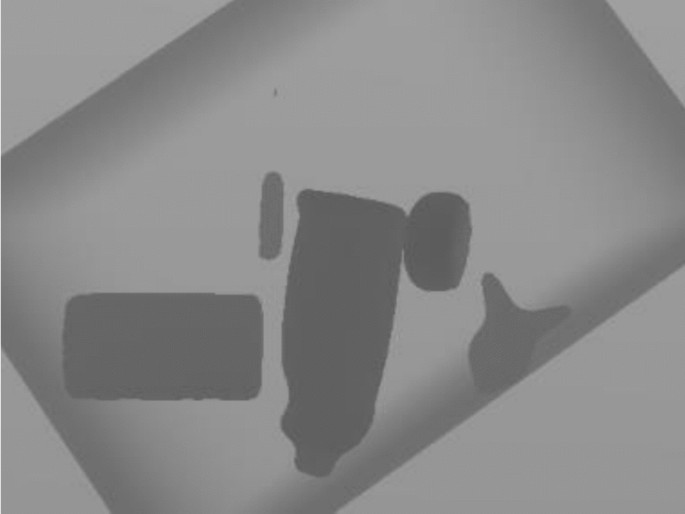
Example simulation result from SD Mask R-CNN
This means that adding a new LLW requires building a 3D mesh of the object, then large amounts of data can be created through simulation and the model can be trained on it. Since SD Mask R-CNN only detects foreground objects as a class, there is no need to take into consideration increasing performance for the new object without decreasing performance for the previous object since all objects fall under the same set.
As seen in Fig. 13 , the results from SD Mask R-CNN are quite accurate despite the false positives. It is able to predict the ground truth quite well on the simulated data and it also predicts other pixel masks for objects that are not there. This seems to be caused by the models’ sensitivity to the foreground pixels it detects. That is, because of the 3D structure of the bin, it is possible predict the parts of the walls of the bin as objects since the walls are closer to the camera and be thought of as foreground pixels. This is a misprediction since the bin is not part of the foreground. This is an indication that the model might struggle when the image contains objects inside objects in a bin, which while not a common scenario, it is a possible one.
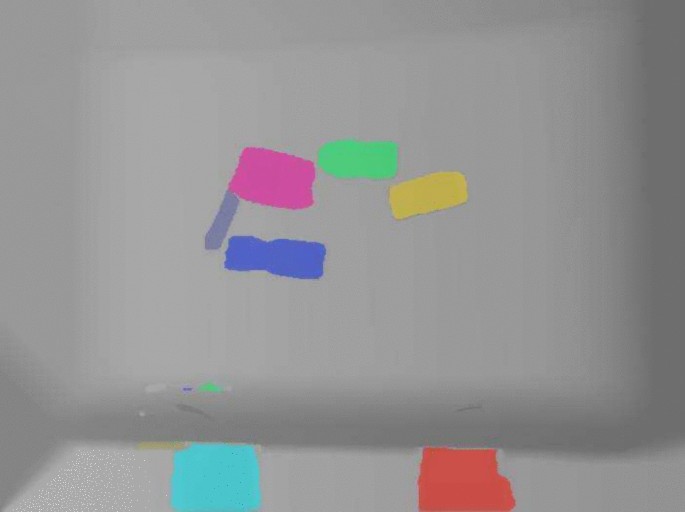
Example prediction from SD Mask R-CNN
Lastly, for OWL-ViT there is no need to take large amounts of pictures or create a 3D mesh of an object, instead a single image of the new LLW is enough. Furthermore, there is no need to train the model since it is supposed to detect new objects in one shot, given a single image.
Figure 14 shows the output bounding boxes from OWL-ViT when queried with a plastic bottle. The results from OWL-ViT are not great despite what the mAP might indicate. The issue.
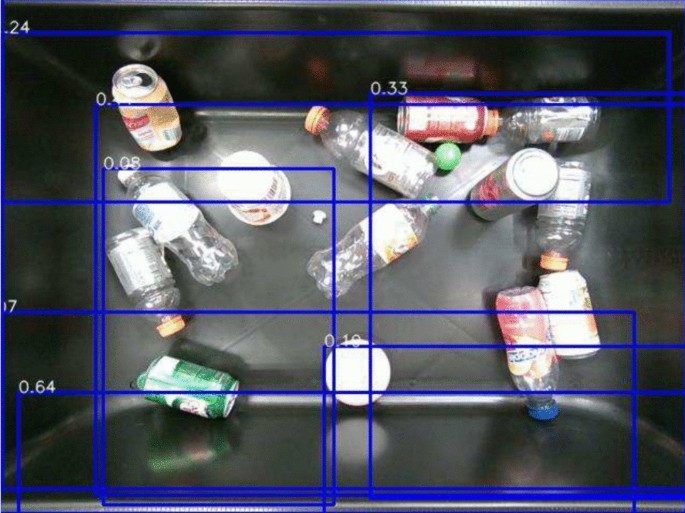
Example prediction from OWL-ViT
with the predictions are that the bounding boxes are much larger than the ground truth. However, the issue is not as large of an issue as with STEGO’s pixel mask predictions.
This issue is caused by two factors: the distance between the objects and the relations between the objects. In the OWL-ViT algorithm, the target images are divided into patches that are used to match with the query image and to predict the bounding boxes. If the objects of the same class are too close together, there will be multiple patches close together that have similar features and match well with the query image. When the algorithm predicts the bounding boxes, it considers all the similar and close together patches as part of the same object prediction, which leads to these bounding boxes that encompass multiple objects.
Analyzing the quality of the prediction results from YOLOv7, STEGO, SD Mask R-CNN, and OWL-ViT, it becomes clear that there is a significant trade-off between performance and adaptability. YOLOv7 for object detection and instance segmentation are the models that had the best performance both according to the mean average precision and the visual.
quality of the results. However, these two algorithms have the worst adaptability and require large amounts of effort to allow them to detect and identify a new object. On the other hand, OWL-ViT had the worst qualitative results, yet it exhibits the best adaptability requiring a single picture to be able to detect a new object. In the middle of the pack, there is STEGO and SD Mask R-CNN, which have good qualitative results better than OWL-ViT but worse than both YOLOv7 algorithms. Additionally, they have decent adaptability where no labeling of pictures is required to detect new objects, though some degree of training is still needed.
However, inference speed does not seem to have a relationship with performance or adaptability, where both YOLOv7 algorithms and OWL-ViT are among the fastest despite having completely different performance and adaptability properties.
As illustrated in Fig. 15 , algorithms that correctly detect the LLW (have high performance) also exhibit low adaptability, that is, the algorithms require a significant amount of human involvement to learn to detect new objects. Conversely, the algorithms that do not correctly detect most of the LLW exhibit high adaptability and require little human involvement to learn to detect new objects. Moreover, it is shown that algorithms that have decent qualitative performance in detecting the LLW tend to require some human effort to detect new objects, but not much.
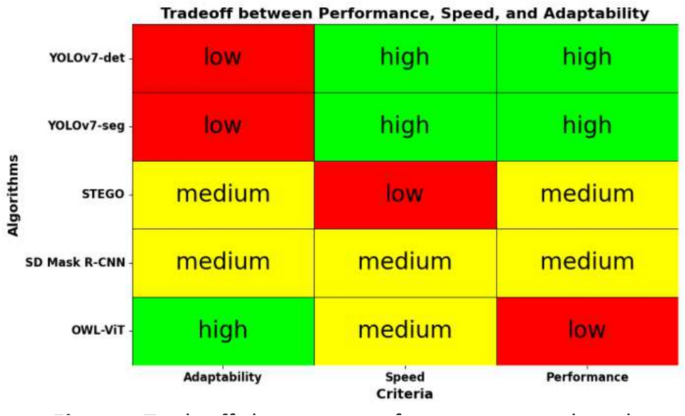
Trade-offs between performance, speed, and adaptability
On that other hand, there does not appear to be any correlation between speed and performance or adaptability. Algorithms with high or low performance like YOLOv7-det, YOLOv7-seg, and OWL-ViT are faster than algorithms with medium performance like STEGO and SD Mask R-CNN. Likewise, the same algorithms with high or low adaptability (YOLOv7-det, YOLOv7-seg, OWL-ViT) are faster than algorithms with medium adaptability (STEGO, SD Mask R-CNN).
As such, when selecting an algorithm to automate the detection and identification of LLW, there is a trade-off to consider between how much human involvement will be available to keep the algorithm detections relevant to the rise of new LLW, and how accurate the algorithms need to be.
5 Conclusion
This work presents a comprehensive overview of different approaches to the problem of detecting and identifying low-level nuclear waste. The detection and identification of low-level nuclear waste are not a static problem, it is dynamic, where a new low-level nuclear waste can be added at any given point in time. Experimental and computational work was carried out on YOLOv7 for object detection and instance segmentation, STEGO for semantic segmentation, SD Mask R-CNN for depth-based instance segmentation, and OWL-ViT for one-shot object detection. From the observed results, there is a trade-off between the performance of the models and their ability to adapt to new low-level nuclear waste. YOLOv7 for instance segmentation had the highest performance on detection and identification; however, it has high requirements to add a new low-level waste. In contrast, OWL-ViT allows for the straightforward addition of new low-level waste for detection, thought its performance is significantly inferior than YOLOv7. And there is no trade-off between performance and speed or adaptability and speed, so it is possible to have highly adaptable and fast models or highly performant and fast models. As future work, it is crucial to improve the performance of one-shot object detection algorithms as well as evaluate approaches using 3D meshes of objects to generate few-shot images that can be used to detect new objects in a more comprehensive manner where all the orthogonal views of an object are made available to the model.
Data availability
The COCO and WISDOM datasets are available at https://cocodataset.org/ and https://sites.google.com/view/ wisdom-dataset/ respectively. The generated and custom datasets are available from the corresponding author on reasonable request.
E. National Academies of Sciences and Medicine, Low-Level Radioactive Waste Management and Disposition: Proceedings of a Workshop. The National Academies Press, Washington, 2017.
O. of Nuclear Energy, Spent fuel and waste disposition, 2023.
Huerta EA, Khan A, Davis E, Bushell C, Gropp WD, Katz DS, Kindratenko V, Koric S, Kramer WTC, McGinty B, McHenry K, Saxton A (2020) Convergence of artificial intelligence and high performance computing on NSF-supported cyberinfrastructure. J Big Data 7:88
Article Google Scholar
J. Redmon SK, Divvala RB, Girshick R, Farhadi A. (2015) You only look once: Unified, real-time object detection, CoRR, vol. abs/1506.02640
Ren S, He K, Girshick RB, Sun J (2015) Faster R-CNN: towards real-time object detection with region proposal networks, CoRR, vol. abs/1506.01497
Liu W, Anguelov D, Erhan D, Szegedy C, Reed SE, Fu C, (2015) Berg AC SSD: single shot multibox detector, CoRR, vol. abs/1512.02325
He K, Gkioxari G, Dollar P, Girshick RB, (2017) Mask R-CNN, CoRR, vol. abs/1703.06870
Chen L, Papandreou G, Kokkinos I, Murphy K, Yuille AL, (2016) Deeplab: Semantic image segmentation with deep convolutional nets, atrous convolution, and fully connected crfs, CoRR, vol. abs/1606.00915
Ronneberger O, Fischer P, Brox T (2015) U-net: Convolutional networks for biomedical image segmentation, CoRR, vol. abs/1505.04597
Melinte DO, Travediu AM, Dumitriu DN (2020) Deep convolutional neural networks object detector for real time waste identification. Appl Sci 10(20):7301
G. Thung and M. Yang, (2016) Classification of trash for recyclability status
Singh N, Sastry P, Niharika BS, Sinha A, Umadevi V (2023) Performance analysis of object detection algorithms for waste segregation, in 2023 Third International Conference on Artificial Intelligence and Smart Energy (ICAIS), pp. 940–945
Vo ND, Tran BN, Van HNN, Duong KB, Le TV, Nguyen N (2022) Empirical study of real-time one-stage object detection methods on recyclable waste dataset, in 2022 RIVF International Conference on Computing and Communication Technologies (RIVF), pp 268–273
Bashkirova D, Abdelfattah M, Saenko K, (2021) Zerowaste dataset: Towards deformable object segmentation in cluttered scenes. Proceedings of the IEEE/CVF Conference on Computer Vision and Pattern Recognition
Zailan NA, Azizan MM, Hasikin K, Mohd Khairuddin AS, Khairuddin U (2022) An automated solid waste detection using the optimized YOLO model for riverine management. Public Health Front 10:907280
Qi J, Nguyen M, Yan WQ (2023) Small visual object detection in smart waste classification using transformers with deep learning. In International Conference on Image and Vision Computing New Zealand (pp. 301-314). Cham: Springer Nature Switzerland
H Deng, D Ergu, F Liu, B Ma, Y Cai (2021) An embeddable algorithm for automatic garbage detection based on complex marine environment, Sensors, 21(19)
Chen X, Huang H, Liu Y, Li J, Liu M (2022) Robot for automatic waste sorting on construction sites. Autom Constr 141:104387
Wang T, Cai Y, Liang L, Ye D (2020) A multi-level approach to waste object segmentation. Sensors 20(14):3816
Proenca PF, Simoes P, (2020) Taco: Trash annotations in context for litter detection, arXiv preprint arXiv:2003.06975
Sun L, Zhao C, Stolkin R, (2017) Weakly-supervised DCNN for RGBD object recognition in real-world applications which lack large-scale annotated training data, CoRR, vol. abs/1703.06370
Kim JG, Jang SC, Kang IS, Lee DJ, Lee JW, Park HS (2020) A study on object recognition using deep learning for optimizing categorization of radioactive waste. Prog Nucl Energy 130:103528
Wang CY, Bochkovskiy A, Liao HYM (2022) Yolov7: Trainable bag-of-freebies sets new state-of-the-art for real-time object detectors
Hamilton M, Zhang Z, Hariharan B, Snavely N, Freeman WT (2022) Unsupervised semantic segmentation by distilling feature correspondences
Danielczuk M, Matl M, Gupta S, Li A, Lee A, Mahler J, Goldberg K, (2019) Segmenting unknown 3d objects from real depth images using mask r-cnn trained on synthetic data
Minderer M, Gritsenko A, Stone A, Neumann M, Weissenborn D, Dosovitskiy A, Mahendran A, Arnab A, Dehghani M, Shen Z, Wang X, Zhai X, Kipf T, Houlsby N (2022) Simple open-vocabulary object detection. In European Conference on Computer Vision (pp. 728-755). Cham: Springer Nature Switzerland
Sutton C, McCallum A (2010) An introduction to conditional random fields
Download references
Office of Environmental Management, DE-EM00005213, Leonel Lagos
Author information
Authors and affiliations.
Knight Foundation School of Computing and Information Sciences, Florida International University, Miami, FL, USA
Aris Duani Rojas & Nagarajan Prabakar
Department of Electrical and Computer Engineering, Florida International University, Miami, FL, USA
Himanshu Upadhyay
Moss Department of Construction Management, Florida International University, Miami, FL, USA
Leonel Lagos
Applied Research Center, Florida International University, Miami, FL, USA
Jayesh Soni
You can also search for this author in PubMed Google Scholar
Corresponding author
Correspondence to Aris Duani Rojas .
Ethics declarations
Conflict of interest.
The authors have no competing interests to declare that are relevant to the content of this article.
Additional information
Publisher's note.
Springer Nature remains neutral with regard to jurisdictional claims in published maps and institutional affiliations.
Rights and permissions
Open Access This article is licensed under a Creative Commons Attribution-NonCommercial-NoDerivatives 4.0 International License, which permits any non-commercial use, sharing, distribution and reproduction in any medium or format, as long as you give appropriate credit to the original author(s) and the source, provide a link to the Creative Commons licence, and indicate if you modified the licensed material. You do not have permission under this licence to share adapted material derived from this article or parts of it. The images or other third party material in this article are included in the article’s Creative Commons licence, unless indicated otherwise in a credit line to the material. If material is not included in the article’s Creative Commons licence and your intended use is not permitted by statutory regulation or exceeds the permitted use, you will need to obtain permission directly from the copyright holder. To view a copy of this licence, visit http://creativecommons.org/licenses/by-nc-nd/4.0/ .
Reprints and permissions
About this article
Duani Rojas, A., Lagos, L., Upadhyay, H. et al. AI-based detection and identification of low-level nuclear waste: a comparative analysis. Neural Comput & Applic (2024). https://doi.org/10.1007/s00521-024-10238-7
Download citation
Received : 16 April 2024
Accepted : 12 July 2024
Published : 22 August 2024
DOI : https://doi.org/10.1007/s00521-024-10238-7
Share this article
Anyone you share the following link with will be able to read this content:
Sorry, a shareable link is not currently available for this article.
Provided by the Springer Nature SharedIt content-sharing initiative
- Low-level nuclear waste
- Environmental safety
- Computer Vision
- Find a journal
- Publish with us
- Track your research

IMAGES
VIDEO
COMMENTS
This paper consists of three main parts i.e. theory, analytical tool and case studies of comparative advantage. Firstly, we review the theory and various empirical measures of comparative ...
2Technically, the term \comparative advantage" refers to the comparison of autarky prices (Deardor 1980), and thus encompasses all determinants of relative production cost di erences. To streamline exposition, this paper uses \comparative advantage" as a short-hand for \relative sectoral productivity di erences," i.e., the Ricardian
1. Introduction. The concept of comparative advantage refers to the ability of a country to produce some good/service not only with higher productivity, as initially proposed by Ricardo, but also higher product differentiation than other countries in a given trade area (Lafay, Citation 1987).Since the pioneer work of Balassa (Citation 1965), the standard method for the measurement of ...
Renewed interest in comparative advantage also stems from the rapid recent growth in North-South and South-South trade, which ostensibly gives resource and technology differences between countries a prominent role in determining global commerce (Hanson 2012). In this paper, we characterize how country export advantages evolve over time.
Levchenko, Andrei A. & Zhang, Jing, 2016. "The evolution of comparative advantage: Measurement and welfare implications," Journal of Monetary Economics, Elsevier, vol. 78 (C), pages 96-111. citation courtesy of. Founded in 1920, the NBER is a private, non-profit, non-partisan organization dedicated to conducting economic research and to ...
provided by Research Papers in Economics. 58 Tri WIDODO such strict assumptions are replaced with the more realistic ones. Heckscher (1919) ... to "reveal" countries' comparative advantage. This paper aims to review the concept and empirical measures of comparative advantage and to derive an analytical tool, namely "products ...
present-day gap between implied comparative advantage and observed comparative ad-vantage is associated with long-term changes in observed comparative advantage. The rest of the paper is structured as follows. Section 2 gives an overview of the related literature. Section 3 provides the basic model behind our findings. Section 4 discusses the
comparative advantage. This paper uses the newly available World Input Output Database to decompose gross exports into domestic value-added and imported intermediate components in order to demonstrate that value-added measures of trade provide a better understanding of comparative advantage from the perspective of trade in tasks and by industry.
The concept of comparative advantage is a cornerstone of economic theory. Since the seminal paper of Balassa (), comparative advantages have usually been measured by Revealed Comparative Advantage (RCA) indexes Footnote 1.RCA indexes are computed on the basis of trade data and provide synthetic measures of comparative advantages (Danna-Buitrago 2017).
In this paper, we propose the normalized revealed comparative advantage (NRCA) index as an alternative measure of comparative advantage. The NRCA index is demonstrated capable of revealing the extent of comparative advantage that a country has in a commodity more precisely and consistently than other alternative RCA indices in the literature. As a result, the NRCA index is comparable across ...
each country has a greater relativ e facility of production in one commodity: Compar ative advantage 3. wine is relatively less expensive to produce than cloth in Portugal, and cloth. relatively ...
The broad policy and institutional areas posited as determinants of comparative advantage in this paper include: physical capital, human capital (distinguishing between secondary, tertiary education and average years of schooling), financial development, energy supply, business climate, labour market institutions as well as import tariff policy
McPhail and Cory Smith for excellent research assistance. This paper has been prepared for the 2012 American Economic Review Papers and Proceedings. The views expressed herein are those of the ... 1In line with Ricardo™s theory of comparative advantage, the focus of our paper is on the supply-side of the economy, not the demand-side ...
On one hand is the economics literature which has, for two centuries, focused on the notion of comparative advantage (technology, factor proportions) while on the other is the business literature which has recently developed the concept of competitive advantage. This paper presents a reconciliation of the two based on global value chain/supply ...
This paper applies a widely-used class of quantitative trade models to evaluate the usefulness of measures of revealed comparative advantage (RCA) in academic and policy analyses. I find that, while commonly-used indexes are generally not consistent with theoretical notions of comparative advantage, certain indexes can be usefully employed for ...
1995, or 29.6% of the sample. By 2010, 7,089 of these present industries became absent (a disappearance rate of 7.5%) while 3,648 initially absent industries became present. (an appearance rate of 7.7%).We can now use our density indices for the implied comparative advantage to ex-plain the appearance and disappeara.
the original theoretical concept of comparative advantage that is based on productivity and factor price difference, and for mixing up comparative advantages with other determinants of trade flows such as trade policy effect s (Leromain and Orefice, 2013). Laursen (2015) cautioned about the misleading . 1
The theory of comparative advantage is a core tool in explaining the patterns of and gains from international trade. In this note, we summarize the theory and apply it to the case of the United ...
In this entry, we discuss the opportunities and challenges of comparative research. We outline the major obstacles in terms of building a comparative theoretical framework, collecting good-quality data and analyzing those data, and we also explicate the advantages and research questions that can be addressed when taking a comparative approach.
Factors of production have well-defined comparative advantage across tasks, which governs the pattern of substitution between skill groups. Technological change can: (1) augment a specific labor type—e.g., increase the productivity of labor in tasks it is already performing; (2) augment capital; (3) automate work by enabling capital to ...
Instead, innovations are increasingly brought to the market by networks of firms, selected according to their comparative advantages, and operating in a coordinated manner. This paper reports on a study of the strategies and practices used by firms that achieve greater success in terms of business value in their collaborative innovation efforts.
This paper argues that Ricardo's discovery of the law of comparative advantage probably occurred in October 1816. The "Ricardo effect" served as a red herring to cause scholars to possibly misread ...
In the United States, comparative policy research is commonly mistaken for international policy research. The confusion may stem from the fact that early comparativists were among the few to be looking at policies abroad, either as a source for inspiration and lesson drawing or, equally widespread, as self-proclaimed proof that there was little to learn from other countries.
An Elementary Theory of Comparative Advantage. Arnaud Costinot. Working Paper 14645. DOI 10.3386/w14645. Issue Date January 2009. Comparative advantage, whether driven by technology or factor endowment, is at the core of neoclassical trade theory. Using tools from the mathematics of complementarity, this paper offers a simple, yet unifying ...
Ensuring environmental safety and regulatory compliance at Department of Energy (DOE) sites demands an efficient and reliable detection system for low-level nuclear waste (LLW). Unlike existing methods that rely on human effort, this paper explores the integration of computer vision algorithms to automate the identification of such waste across DOE facilities. We evaluate the effectiveness of ...