WHAT ARE YOU LOOKING FOR?
Key searches, artificial intelligence.
Our academic enterprise focuses on addressing the most complex challenges to health, and to make progress, we embrace the opportunities presented by advances in computation.

AI Igniting Discovery in Medicine
The Keck School of Medicine of USC is harnessing artificial intelligence (AI) to turn big data into knowledge, igniting discoveries to solve the world’s toughest health care problems. Dean Carolyn Meltzer and USC SVP Steven Shapiro discuss how to deliver on that promise and minimize risks.
- Read “The future of AI in medicine”
AI in Medicine Collaboratory (AI-MEDx)
Lead by Dr. Paul Thompson, PhD , the AI in Medicine Collaboratory (AI-MEDx) at the Keck School of Medicine is a pioneering convergent research initiative committed to translating our research findings into tangible, patient-centric solutions through collaboration and partnership. Our mission is to leverage our unique strengths in medicine, data science, and the diverse patient population we serve to address clinical unmet needs, drive transformative AI-driven discoveries, and facilitate their translation into equitable and patient-centric healthcare solutions.
- Medical School Focus : As an initiative based at KSOM, we leverage our institutional expertise, resources, and academic environment to drive interdisciplinary collaborations between clinicians, researchers, data scientists, and AI experts. By capitalizing on the unique perspectives and talents within our medical school, we aim to push the boundaries of AI and its applications in medicine.
- University-wide Connectivity : The AI in Medicine AI-MEDx serves as a central hub at the Health Science Campus that connects and integrates with other AI in Medicine efforts across the university. We actively foster collaborations with various departments, research centers, and institutes, promoting knowledge sharing, cross-pollination of ideas, and collaborative initiatives that accelerate progress in AI in Medicine.
- Addressing Clinical Unmet Needs : Our AI-MEDx is driven by a deep commitment to addressing the real-world challenges faced by our clinicians and patients. We prioritize understanding clinical unmet needs, identifying areas where AI has the potential to make a significant impact, and developing innovative solutions that enhance patient care, improve outcomes, and drive healthcare efficiencies.
- Use-Inspired Discovery and Translational Research : At the heart of our mission is the philosophy of use-inspired discovery and translational research. We embrace a pragmatic approach that emphasizes the practical application of AI in solving clinical problems. Through close collaborations with clinicians and basic researchers, we aim to bridge the gap between research and practice, translating cutting-edge AI research into meaningful solutions that directly benefit patients and healthcare providers.
- Embracing Diversity : A key differentiator of our AI-MEDx is our unwavering commitment to embracing the diversity of our patient population and beyond. We recognize that equitable and inclusive healthcare requires tailored solutions that address the unique needs, challenges, and cultural contexts of diverse patient groups. We actively promote diversity in our research, collaborations, and solutions to ensure that AI-driven innovations in medicine benefit all individuals, regardless of their background or circumstances.
Bridging the gap between technology, research, and clinical practice
- Medical Imaging
- Diagnostics
- Biomarker Discovery
- Drug Discovery
- Patient Care
Algorithm Nation: AI Amplifies Core Research Strengths
At the Keck School of Medicine of USC, AI has potential for broad impact — as an enabling tool for investigations to improve human health. Algorithmic brawn fortifies longtime areas of strength at USC, such as research into cancer, neurodegenerative disease and population health, and aids in snooping out the biomedical needle in the haystack.
Keck School AI News

Using AI, USC researchers pioneer a potential new immunotherapy approach for treating glioblastoma
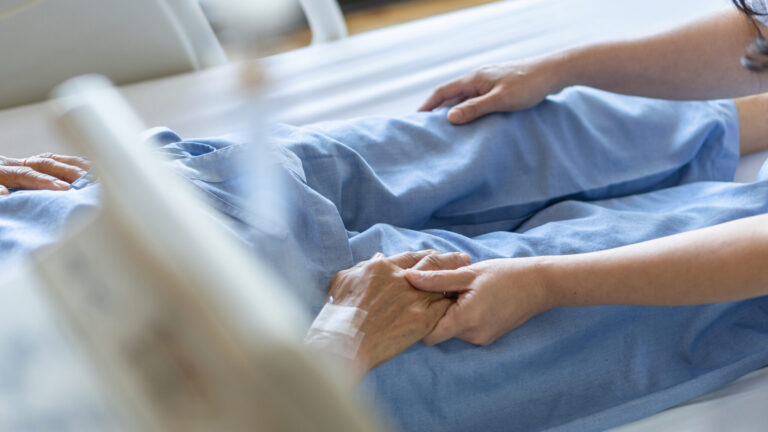
USC-Led Study Leverages Artificial Intelligence to Predict Risk of Bedsores in Hospitalized Patients
A new study in BMJ Open presents a new model for predicting patients most at risk of bedsores in hospitals.
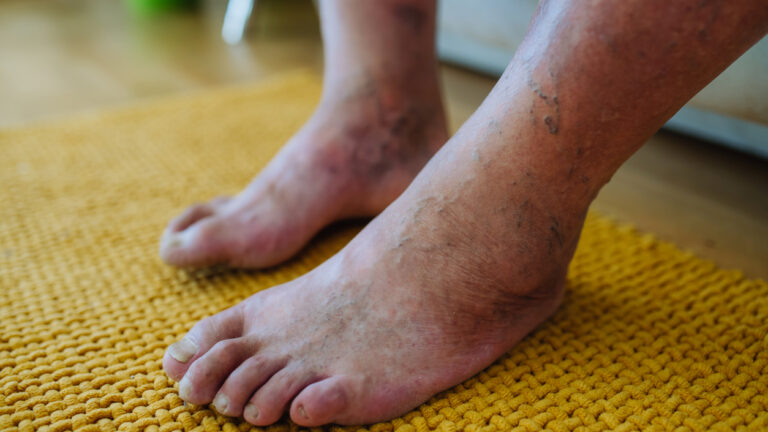
Using AI to Transform Diabetic Foot and Limb Preservation
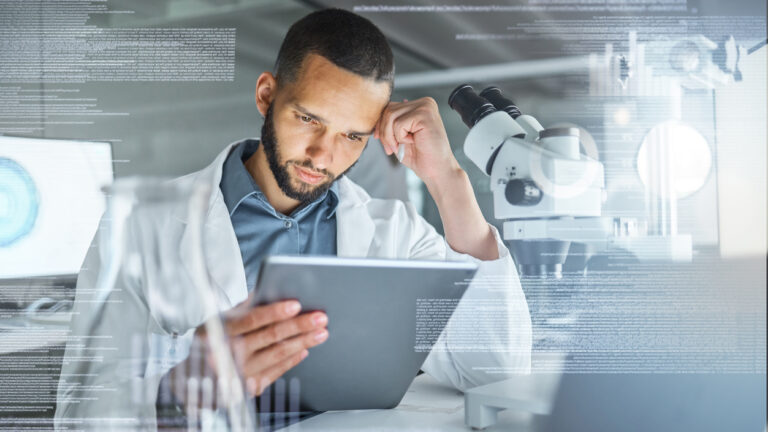
AI in Science Publication: The Good, the Bad and the Questionable

Double Victory: Residency Classmates Turned USC Faculty Achieve NIH Funding Success
In early 2024, ophthalmologists Sun Young Lee, MD, PhD, a retina specialist and Benjamin Xu, MD, PhD, a glaucoma specialist, celebrated a significant achievement – both were awarded their first R01 grants from the National Institutes of Health (NIH). Remarkably, Lee and Xu’s journeys began together over a decade ago when they started residency training at USC in 2013.
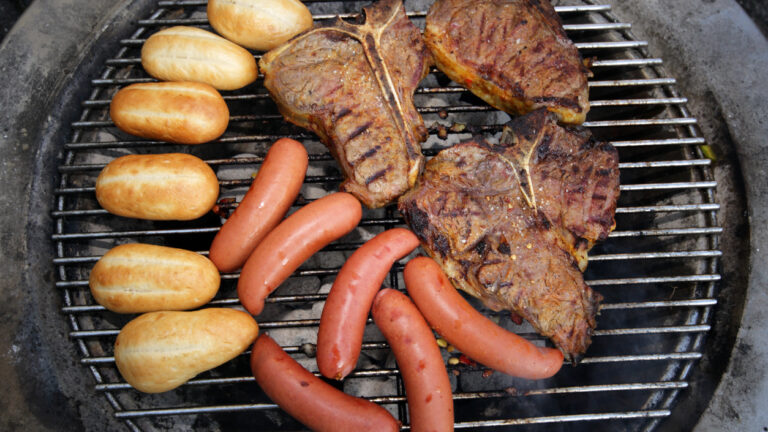
Large-scale study explores genetic link between colorectal cancer and meat intake
Looking to collaborate on AI with the Keck School of Medicine? Contact Vasiliki Anest, PhD, Keck School Chief Innovation Officer and Head of MESH Strategic Partnerships [email protected]
We're sorry but you will need to enable Javascript to access all of the features of this site.
Stanford Online
Artificial intelligence in healthcare.
Stanford School of Medicine , Stanford Center for Health Education
Monthly subscription: $79
Get Started
Artificial intelligence (AI) has transformed industries around the world, and has the potential to radically alter the field of healthcare. Imagine being able to analyze data on patient visits to the clinic, medications prescribed, lab tests, and procedures performed, as well as data outside the health system -- such as social media, purchases made using credit cards, census records, Internet search activity logs that contain valuable health information, and you’ll get a sense of how AI could transform patient care and diagnoses. In this specialization, we'll discuss the current and future applications of AI in healthcare with the goal of learning to bring AI technologies into the clinic safely and ethically. This specialization is designed for both healthcare providers and computer science professionals, offering insights to facilitate collaboration between the disciplines.
- Identify problems healthcare providers face that machine learning can solve
- Analyze how AI affects patient care safety, quality, and research
- Relate AI to the science, practice, and business of medicine
- Apply the building blocks of AI to help you innovate and understand emerging technologies
- Preview Image
- Course/Course #
- Time Commitment
- Availability

Introduction to Healthcare

Introduction to Clinical Data

Fundamentals of Machine Learning for Healthcare

Evaluations of AI Applications in Healthcare

AI in Healthcare Capstone
Flexible enrollment options, monthly subscription: $79.
View and complete course materials, video lectures, assignments, and exams, at your own pace with a monthly subscription to the Artificial Intelligence in Healthcare Specialization.
What Our Learners Are Saying
AI in Healthcare is an incredible program offering content related to the Healthcare System, Clinical Data, Machine Learning, & Artificial Intelligence Applications in Healthcare. After completing this program, one choose more advanced study in the aforementioned topics and/or take a deeper dive in the numerous interrelated subjects such as computational math, stats, programming/coding and algorithms. Simply outstanding!!
John S., Clinical Specialist
Upcoming Events

Health & Medicine Programs Information Session
You may also like.

Introduction to Food and Health
SOM-YCME0004
Stanford School of Medicine

Digital Health Product Development
SOM-XCHE0025
Stanford School of Medicine, Stanford Center for Health Education

Machine Learning Projects in Healthcare
XBIOMEDIN215
Stanford School of Engineering, Stanford School of Medicine

Clinical Trials: Design, Strategy, and Analysis
SOM-XCHE0030
- Engineering
- Artificial Intelligence
- Computer Science & Security
- Business & Management
- Energy & Sustainability
- Data Science
- Medicine & Health
- Explore All
- Technical Support
- Master’s Application FAQs
- Master’s Student FAQs
- Master's Tuition & Fees
- Grades & Policies
- Graduate Application FAQs
- Graduate Student FAQs
- Graduate Tuition & Fees
- Community Standards Review Process
- Academic Calendar
- Exams & Homework FAQs
- HCP History
- Enrollment FAQs
- Tuition, Fees, & Payments
- Custom & Executive Programs
- Free Online Courses
- Free Content Library
- School of Engineering
- Graduate School of Education
- Stanford Doerr School of Sustainability
- School of Humanities & Sciences
- Stanford Human Centered Artificial Intelligence (HAI)
- Graduate School of Business
- Stanford Law School
- School of Medicine
- Learning Collaborations
- Stanford Credentials
- What is a digital credential?
- Grades and Units Information
- Our Community
- Get Course Updates

Main navigation
Please see here for the full program and speaker info., day 1: december 6, 2023.
8:00-9:00am PT | |
Our first keynote presentation will feature Dr. Jessica Mega, who will delve into the development of technology in healthcare and life science, the road to technology adoption, and the use of AI in healthcare and life science through clinical applications. The session will include a moderated fireside chat with Dr. Curt Langlotz, engaging attendees in a dynamic Q&A session, addressing questions, and fostering insightful discussions on the topics explored. |
9:15-10:30am | |
---|---|
Track 1 | This session explores cutting-edge advancements in healthcare through pivotal topics such as: digital innovations for global health communication, deep learning algorithms in clinical care, and the integration of non-invasive imaging and machine learning in pathology. Experts will discuss practical and technical issues, as well as future perspectives, highlighting diverse examples of the transformative potential of digital health innovations in clinical care. |
Track 2 | This session will focus on the recent advances of AI in precision medicine and drug discovery. Various technical and practical issues as well as future perspectives in these rapidly evolving fields will be summarized. |
Track 3 | This session will delve into the challenges and innovations related to foundation models in healthcare. Speakers will discuss the early developments and adoption of foundation models in the healthcare domain, addressing issues such as data accessibility, pre-training approaches, and performance evaluation. Talks and discussions will provide valuable insights into the future of foundation models in healthcare. |
10:45-12:00pm | |
This plenary session will unravel the intricate process of bridging the AI chasm, a crucial step that connects research with real-world implementation. Academic and industry experts will discuss translating AI innovations to clinical settings, offering insights into practical applications and deployment in healthcare, bridging the gap between innovation and implementation. |
12:45-2:00pm | |
---|---|
Track 1 | This session will explore the use of large language models in physician-patient communication and asynchronous care delivery. Stanford’s experience as one of the first health systems to implement an Epic-integrated, LLM-powered auto-reply tool to help manage electronic patient messages will be discussed, focusing on optimizing clinical operations, enhancing physician-patient communication, and reducing physician burnout. |
Track 2 | This session will provide insights into global trends in the application of safe AI within ophthalmology and broader healthcare. It will offer perspectives from international research and tech companies, discussing the applications and challenges of implementing AI in eye care and healthcare at large. |
Track 3 | In an era where technology is evolving at an unprecedented pace, the field of medicine is ripe for transformation. This session will shed light on innovative applications of Generative AI in medical research, diagnostics, treatment planning, and healthcare management. Industry leaders and academic experts will explore the challenges, breakthroughs, and ethical considerations associated with employing Generative AI in healthcare. |
2:15-3:30pm | |
Moderated by Stanford University School of Medicine’s Dean Lloyd Minor, this plenary session will focus on the responsible development and implementation of AI in health. Experts will discuss ethical considerations, best practices, and the future implications of AI in healthcare, ensuring a balanced and ethical integration of AI technologies in the field. |
Day 2: December 7, 2023
8:00-9:00am PT | |
In this keynote plenary session, Dr. Peter Lee will provide insights into the emergence of General AI for Medicine. Future prospects and challenges associated with General AI in the field of healthcare will be explored. The session will include a moderated fireside chat with Dr. Natalie Pageler, engaging attendees in a dynamic Q&A session, addressing questions, and fostering insightful discussions on the topics explored. |
9:15-10:30am | |
---|---|
Track 1 | Digital technologies are transforming healthcare, and health systems can lean in on their care delivery expertise to be active innovators as builders rather than passive customers of vendors. This panel will discuss building and scaling ecosystems for digital innovation. Topics will include operating models, measuring value, leadership buy-in, sustainability, and using clinical informatics for organizational transformation. |
Track 2 | This session will explore the transformative role of AI in dermatology and patient care by delving into the impact of foundation models on education, treatment delivery, and patient outcomes. Gain insights from global perspectives on the promise and challenges of AI in healthcare systems broadly, leaving with a comprehensive understanding of AI's pivotal role in dermatology and beyond. |
Track 3 |
|
10:45-12:00pm | |
---|---|
Track 1 | This session will discuss various applications of AI and machine learning in surgical training and education. Experts will explore how these technologies are improving clinical diagnosis, outcomes, and technical skills of surgeons and proceduralists. Specific research on computer vision, augmented reality, and gaming platforms for skills acquisition will be presented. |
Track 2 | This session will focus on AI applications for understanding RNA and advancing new RNA medicines and diagnostics for precision health. |
Track 3 |
Leading experts in AI applications to cardiovascular health and genomics will present their latest work and discuss the future of understanding the heart and applying that knowledge to medicine. The session will also explore how new long-range language models can be utilized to understand the most fundamental language of all: our DNA. |
12:45-2:00pm | |
---|---|
Track 1 | Delve into the world of big data in healthcare, exploring its types, management strategies, and its transformative potential in personalized health innovations. Experts will discuss various types of big data, management strategies, and data-driven models. The session will highlight how effective data utilization can improve clinical operations and enhance personalized healthcare. |
Track 2 | This session will focus on the clinical-translational applications of AI in medical imaging. Experts will present and discuss new frontiers and emerging applications of AI in the field of medical imaging, showcasing innovative approaches and their potential impact on healthcare. |
Track 3 | This session will discuss Ambient Intelligence (AmI) in healthcare, focusing on the integration of seamlessly interconnected electronic devices and systems supporting patient care in hospitals and homes. Experts will discuss continuous monitoring, smart home adaptations, improved patient experiences, remote health tracking, data-driven decision support, personalized rehabilitation, and predictive health analytics. Challenges related to data security and privacy in AmI will also be discussed. |
2:15-3:30pm | |
In the closing plenary session, Drs. Fei-Fei Li and Lloyd Minor will present “Responsible AI For Safe and Equitable (RAISE) Health”, a new joint initiative between Stanford Medicine and the Stanford Institute for Human-Centered AI to guide the responsible use of AI across biomedical research, education, and patient care. Additional speakers will address ethical considerations and safe implementation of AI in healthcare to promote equity and fairness in its applications. |
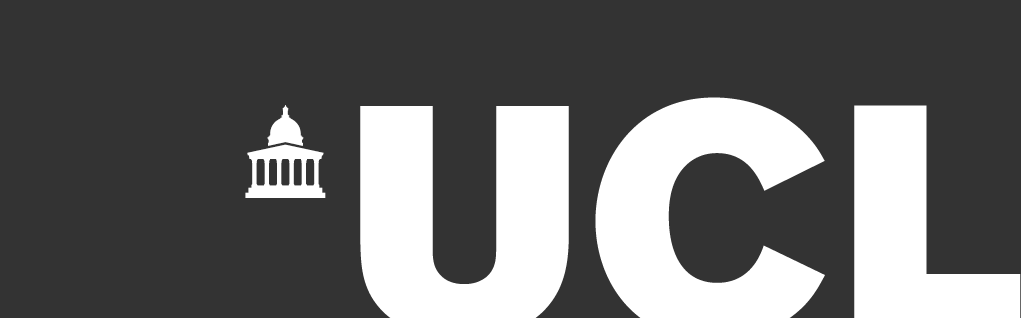
Artificial Intelligence Enabled Healthcare MRes + MPhil/PhD
London, Bloomsbury
Artificial Intelligence (AI) has the potential to transform health and healthcare systems globally, yet few individuals have the required skills and training. To address this challenge, our Centre For Doctoral Training (CDT) in AI-Enabled Healthcare Systems will create a unique interdisciplinary environment to train the brightest and best healthcare artificial intelligence scientists and innovators of the future.
UK tuition fees (2024/25)
Overseas tuition fees (2024/25), programme starts, applications accepted.
Applications closed
The Centre for Doctoral Training recruits in at least two rounds. Applicants are advised to apply early, priority will be given to those who have applied in round one.
- Entry requirements
A minimum of an upper second class honours undergraduate degree, or a Master's degree in a relevant discipline (or equivalent international qualifications or experience). Our preferred subject areas are Physical Sciences (Computer Science, Engineering, Mathematics and Physics) or Clinical / Biomedical Science. Applicants with a clinical background or degree in Biomedical Science must be able to demonstrate strong computational skills. You must be able to demonstrate an interest in creating, developing or evaluating AI-enabled Healthcare systems.
The English language level for this programme is: Level 2
UCL Pre-Master's and Pre-sessional English courses are for international students who are aiming to study for a postgraduate degree at UCL. The courses will develop your academic English and academic skills required to succeed at postgraduate level.
Further information can be found on our English language requirements page.
If you are intending to apply for a time-limited visa to complete your UCL studies (e.g., Student visa, Skilled worker visa, PBS dependant visa etc.) you may be required to obtain ATAS clearance . This will be confirmed to you if you obtain an offer of a place. Please note that ATAS processing times can take up to six months, so we recommend you consider these timelines when submitting your application to UCL.
Equivalent qualifications
Country-specific information, including details of when UCL representatives are visiting your part of the world, can be obtained from the International Students website .
International applicants can find out the equivalent qualification for their country by selecting from the list below. Please note that the equivalency will correspond to the broad UK degree classification stated on this page (e.g. upper second-class). Where a specific overall percentage is required in the UK qualification, the international equivalency will be higher than that stated below. Please contact Graduate Admissions should you require further advice.
About this degree
Every student who is accepted onto the AI-enabled Healthcare Systems Centre for Doctoral Training (CDT) must take the MRes Artificial Intelligence Enabled Healthcare in their first year. This will be followed by a 3 year PhD. Throughout this period the CDT will continue to closely monitor the need for continuing training and support, tailored to each student, and provide ongoing training in research skills. The MRes is not currently available as a stand-alone programme.
The MRes programme covers the core competencies of artificial intelligence and has a central emphasis on how healthcare organisations work. Ethical training for medical artificial intelligence will be explicitly emphasised alongside a broader approach to responsible research, innovation and entrepreneurship.
During the MRes year, students will learn the statistical underpinnings of machine learning theory, get a practical grounding in research software engineering and the principles of healthcare and medical research, as well as a thorough treatment of topics in machine learning, advanced statistics and principles of data science.
As part of the MRes, alongside the core and elective modules, you will complete a substantial Masters-level project of your choice, working with a supervisory team that will normally include a clinician and an academic. The project you work on during your MRes normally leads to the chosen PhD research topic.
The remaining years will be more like a traditional PhD, which leads to the presentation of a PhD thesis at the end of the fourth year. During your PhD you will remain involved in CDT activities and will continue to work closely with relevant health professionals and clinical teams through our NHS partners and leading academics at UCL.
As a cohort based PhD programme, students will also have the opportunity to participate in a range of seminars, training programmes, placements and other activities, including UCL's Doctoral Skills Development Programme.
Training Opportunities The CDT programme consists of a range of activities and events including:
- A Mini-MD programme where trainees undertake an immersive clinical experience within an NHS setting
- Annual CDT Conference
- Seminar series
- PPI Training
- Responsible Research & Innovation
- Communication Skills
- Entrepreneurship
- Ethical Training
- The opportunity to attend training programmes offered by the Alan Turing Institute
- Opportunities for internships and placements with industry partners
More information can be found on the CDT Website .
Who this course is for
The Centre for Doctoral Training programme is for students with an interest in creating and developing AI solutions aimed to transform and solve healthcare challenges. The CDT programme is embedded within a NHS setting, and should appeal to students keen to develop clinical knowledge and algorithmic/ programming expertise.
What this course will give you
- Benefit from UCL's excellence both in computational science and biomedical research innovating in AI;
- Be supervised by world-leading clinicians and AI researchers in areas related to your research;
- Work within a real-world setting, embedded within hospitals, allowing you to gain a practical understanding of the value and limitations of the datasets and the translational skills required to put systems into practice;
- Have the opportunity to not only apply AI to healthcare but to apply healthcare to AI, generating novel large-scale open datasets driving methodological innovation in AI;
- Become a future leader in solving the most pressing healthcare challenges with the most innovative AI solutions;
- Study at UCL, which is rated No.1 for research power and impact in medicine, health and life sciences (REF 2021) and 9th in the world as a university (QS World Rankings 2024).
The foundation of your career
We do not yet have any graduates from the four-year programme, our first cohort of students will be graduating over the next few months. We expect them to stay within the field of AI and healthcare, and much like previous graduates from our experienced CDT supervisors, they will go onto successful careers in academia and industry.
Employability
The distinctive characteristics of our programme allow us to produce graduates who are prepared to:
- engineer adaptive and responsive solutions that use AI to deal with complexity;
- innovate across all levels of care, from community services to specialist hospitals;
- be comfortable working with patients and professionals, and responding to their input;
- appreciate the importance of addressing health needs rather than creating new demand.
The Institute's research departments collaborate with third-sector and governmental organisations, as well as members of the media, both nationally and internationally to ensure the highest possible impact of their work beyond the academic community. Students are encouraged to do internships with relevant organisations where funding permits. Members of staff also collaborate closely with academics from leading institutions globally.
Teaching and learning
Various teaching and learning methods are employed to facilitate effective learning and cater to different learning styles. Below are some common types of teaching methods that may be used across the programme:
Interdisciplinary Teaching: Interdisciplinary teaching involves integrating knowledge and skills from multiple disciplines or subject areas to provide a comprehensive understanding of a topic, particularly AI and healthcare. This approach encourages students to make connections between different subjects and fosters critical thinking and problem-solving abilities.
Lecture-Based Teaching: Lecture-based teaching is a traditional method where the instructor presents information to students through spoken words. It involves the teacher sharing knowledge, concepts, and theories, while students take notes and listen actively. This method is effective for conveying large amounts of information and providing foundational knowledge.
Practical Coding Sessions: Practical coding sessions are hands-on learning experiences where students actively engage in coding exercises, programming tasks, and problem-solving activities. These sessions are essential for AI and programming-related subjects (machine learning, etc) as they allow students to apply theoretical knowledge to real-world scenarios.
Interactive Teaching: Interactive teaching methods encourage active participation and engagement from students. These methods can include discussions, debates, group activities, and case studies, in particular in several modules such as Journal Club. Interactive teaching fosters collaboration, communication skills, and a deeper understanding of the subject matter.
Project-Based Learning: Project-Based Learning involves assigning students long-term projects that require them to investigate and address real-world problems or challenges (such as AI & healthcare group project). It enhances critical thinking, research skills, and creativity while promoting independent learning and teamwork.
Collaborative Learning: Collaborative learning involves students working together in small groups or pairs to solve problems, discuss ideas, and complete tasks. This method promotes teamwork, communication, and the exchange of diverse perspectives.
The use of these teaching/learning methods can vary depending on the subject matter, the goals of the programme, and the preferences of the instructors in the MRes year. Our educational programme incorporates a mix of these methods to cater to the diverse needs of learners and create a well-rounded learning experience.
Compulsory Modules:
CHME0033 Dissertation in Artificial Intelligence Enabled Healthcare
CHME0032 Healthcare Artificial Intelligence Journal Club
Optional Modules
CHME0012 Principles of Health Data Science
CHME0013 Data Methods for Health Research
CHME0015 Advanced Statistics for Records Research
CHME0016 Machine Learning in Healthcare and Biomedicine
CHME0031 Programming with Python for Health Research
CHME0034 Computational Genetics of Healthcare
CHME0035 Advanced Machine Learning for Healthcare
CHME0039 Artificial Intelligence in Healthcare Group Project
COMP0084 Information Retrieval and Data Mining
Please note that the list of modules given here is indicative. This information is published a long time in advance of enrolment and module content and availability is subject to change.
Assessment methods are crucial components of an educational programme, as they evaluate students' understanding, knowledge, skills, and application of concepts. Here are various types of assessment methods that may be used across the programme:
Exams: Traditional exams are a common assessment method that tests students' knowledge and understanding of the course material. These exams typically involve a time-bound written assessment, where students respond to questions related to the subject matter.
Open-Book Exam: In an open-book exam, students are allowed to refer to their textbooks, notes, or other resources during the assessment. The questions in these exams are often designed to test higher-order thinking and problem-solving abilities, as students have access to reference materials.
Coursework: Coursework assessments involve various assignments, essays, reports, or projects that students complete throughout the course. These assessments may cover specific topics or practical applications and help to assess students' comprehension and critical thinking skills.
Coding Exam: A coding exam is specifically designed for courses related to computer science, software development, or programming. Students are given coding challenges or programming tasks that assess their coding proficiency and problem-solving abilities.
Collaborative Project: In a collaborative project assessment, students work in groups to tackle a complex problem or complete a substantial task. This assessment measures teamwork, communication, time management, and the ability to achieve shared goals.
Presentation and Q&A: Presentations require students to deliver a talk on a given topic or project. The presentation assesses their ability to communicate effectively, organize information, and present ideas coherently. Often, a question and answer (Q&A) session follows the presentation to delve deeper into the topic.
Research Proposal: A research proposal is a preliminary plan for a research project that students submit to demonstrate their research capabilities. It outlines the research question, objectives, methodology, and potential outcomes of the study.
Dissertation Writing: Dissertation writing is typically reserved for higher education levels, such as undergraduate and postgraduate studies. It involves an extended research project on a specific subject, allowing students to demonstrate research, analytical, and academic writing skills.
Online Quizzes and Tests: Online quizzes and tests are digital assessments that may be used for formative or summative purposes. They are often employed in blended or online learning environments.
The use of assessment methods will vary based on the nature of the programme, the subject matter throughout the MRes year. A well-balanced combination of assessment types ensures that students' diverse abilities and learning styles are appropriately evaluated while providing a comprehensive understanding of their progress and achievements.
During the MRes 4 hours of a student's time is spent in tutorials per week and/or, 6-8 hours in lectures per week, and a further 20-24 hours in independent study per week.
Research areas and structure
- AI-enabled diagnostics or prognostics
- AI-enabled operations
- AI-enabled therapeutics
- Public Health Data Science
- Machine Learning in Health Care
- Public Health informatics
- Learning health systems
- Electronic health records and clinical knowledge management
- e-health and m-health
- Clinical Decision Support Systems
Research environment
Our research environment offers a unique degree programme that stands out among competitors. We provide students with the exceptional opportunity to explore the cutting-edge intersection of AI technology and healthcare applications. Our curriculum emphasizes research and innovation skills, empowering students to become independent researchers and adept problem solvers. A key difference is our close collaboration with clinicians and front-line practitioners. This interaction fosters a holistic understanding of healthcare challenges and real-world applications, ensuring that our graduates are equipped with practical knowledge and solutions. Our programme is inclusive, welcoming students from both computational and clinical backgrounds, creating a diverse and dynamic learning environment.
Students studying the programme full-time will be expected to complete 180 credits during the academic year.
Students studying the programme part-time will be expected to complete 180 credits across two academic years.
Accessibility
Details of the accessibility of UCL buildings can be obtained from AccessAble accessable.co.uk . Further information can also be obtained from the UCL Student Support and Wellbeing team .
Fees and funding
Fees for this course.
Fee description | Full-time | Part-time |
---|---|---|
Tuition fees (2024/25) | £6,035 | £3,015 |
Tuition fees (2024/25) | £31,100 | £15,550 |
The tuition fees shown are for the year indicated above. Fees for subsequent years may increase or otherwise vary. Where the programme is offered on a flexible/modular basis, fees are charged pro-rata to the appropriate full-time Master's fee taken in an academic session. Further information on fee status, fee increases and the fee schedule can be viewed on the UCL Students website: ucl.ac.uk/students/fees .
Additional costs
All studentships include a research training support grant, which covers additional research costs throughout students' time on the programme.
For more information on additional costs for prospective students please go to our estimated cost of essential expenditure at Accommodation and living costs .
Funding your studies
Please visit the CDT website for funding information.
For a comprehensive list of the funding opportunities available at UCL, including funding relevant to your nationality, please visit the Scholarships and Funding website .
Note for applicants: When applying on UCL Select, please select MRes Artificial Intelligence enabled healthcare to apply for programme.
Please note that you may submit applications for a maximum of two graduate programmes (or one application for the Law LLM) in any application cycle.
Got questions? Get in touch
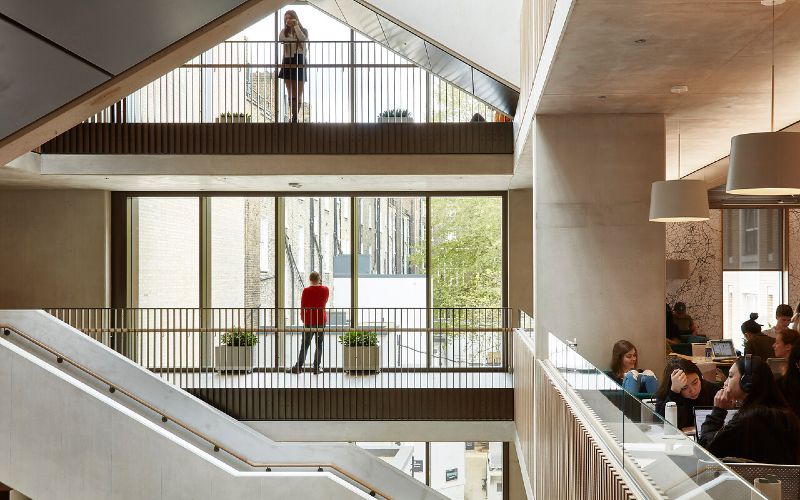
Institute of Health Informatics
UCL is regulated by the Office for Students .
Prospective Students Graduate
- Graduate degrees
- Taught degrees
- Taught Degrees
- Applying for Graduate Taught Study at UCL
- Research degrees
- Research Degrees
- Funded Research Opportunities
- Doctoral School
- Funded Doctoral Training Programmes
- Applying for Graduate Research Study at UCL
- Teacher training
- Teacher Training
- Early Years PGCE programmes
- Primary PGCE programmes
- Secondary PGCE programmes
- Further Education PGCE programme
- How to apply
- The IOE approach
- Teacher training in the heart of London
- Why choose UCL?
- Inspiring facilities and resources
- Careers and employability
- Your global alumni community
- Your wellbeing
- Postgraduate Students' Association
- Your life in London
- Accommodation
- Funding your Master's
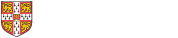
- Study at Cambridge
- About the University
- Research at Cambridge
- Colleges and departments
- Email and phone search
- For business
- For current students
- Libraries and facilities
- Museum and collections
Search form
- Events and open days
- Fees and finance
- Student blogs and videos
- Why Cambridge
- Qualifications directory
- How to apply
- Fees and funding
- Frequently asked questions
- International students
- Continuing education
- Executive and professional education
- Course in education
- Giving to Cambridge
- How the University and Colleges work
- Visiting the University
- Spotlight on...
- About research at Cambridge
Department of Engineering
- Overview of the Department
- 21st Century Engineers
- Staff and Student Directory
- Department Newsletter
- Alumni Relations
- How to Find Us
- Keep in touch
- Undergraduates Overview
- Prospective Undergraduates
- Information for Staff
- Current Undergraduates
- Postgraduates Overview
- Taught courses (MPhil and MRes)
- Centres for Doctoral Training (CDTs)
- PhD in Engineering
- MPhil in Engineering
- Part-time study
- Applying for taught courses and CDTs
- Applying for research courses
- Applying for part-time study
- Requirements for postgraduate students
- English language requirements
- International equivalencies
- Funding opportunities for applicants
- Current Postgraduate Students
- Information for staff
- Research Overview
- Energy, Fluids and Turbomachinery
- Electrical Engineering
- Mechanics, Materials and Design
- Civil Engineering
- Manufacturing and Management
- Information Engineering
- Energy, Transport and Urban Infrastructure
- Manufacturing, Design and Materials
- Bioengineering
- Complex, Resilient and Intelligent Systems
- Research news
- Research Integrity
- Collaboration Overview
- Student Placements
- Short Student Projects
- Longer Projects and Frameworks
- Academic Partnerships
- Consulting and Other Services
- Giving to the Department
- Events and Outreach Overview
- Events and Seminars
- Work Experience at the Department of Engineering
- Services Overview
- Building and Estate Services
- Design & Technical Services
- Health and Safety
- Printing Services
- Centre for Languages and Inter-Communication
Cambridge Centre for AI in Medicine announces its official launch
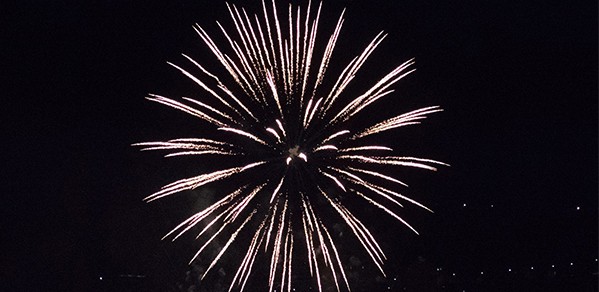
The University of Cambridge has announced a five-year agreement with AstraZeneca and GSK to fund the Cambridge Centre for AI in Medicine (CCAIM). For the five-year duration, AstraZeneca and GSK will support five new PhD studentships per year. This programme will enable the best and brightest young minds in machine learning and bioscience to partner with leaders in industry and academia, wherever they may be in the world.
CCAIM is designed to break down the barriers between machine learning and medical science, to create a unique forum in which we can work together to truly understand the challenges, formalise the problems, and develop practical solutions that can be readily implemented in healthcare. Professor Mihaela van der Schaar
CCAIM has been set up as a cutting-edge research group. Its faculty of 10 University of Cambridge researchers – in addition to world-class PhD students, currently being recruited – have united to develop AI and machine learning (ML) technologies aiming to transform clinical trials, personalised medicine and biomedical discovery.
The centre’s Director is Professor Mihaela van der Schaar , a world leading researcher in ML, and the Co-Director is researcher-clinician Professor Andres Floto. The faculty also includes Dr Sarah Teichmann FMedSci FRS, Head of Cellular Genetics at the Wellcome Sanger Institute and founder and principal leader of the Human Cell Atlas international consortium.
Successfully bridging the gap between the disparate and complex fields of AI and medicine requires building from both sides simultaneously. CCAIM brings together a diverse coalition of leading Cambridge scientists and clinicians, with expertise in machine learning, engineering, mathematics, medicine, computer science, genetics, computational biology, biostatistics, clinical research, healthcare policy and more.
These multi-disciplinary experts from the University of Cambridge will work in close collaboration with scientists and leaders from AstraZeneca and GSK to identify critical challenges facing drug discovery and development that have the potential to be solved through cutting-edge academic research.
The Centre’s research output and the implementation of its ML tools could be transformational not only for the pharmaceutical industry – including in clinical trials and drug discovery – but also for the clinical delivery of healthcare to patients. The CCAIM team already has deep research links with the NHS, and four of the Centre’s members are NHS doctors.
Professor van der Schaar said: “Machine learning has the potential to truly revolutionise the delivery of healthcare, to the great benefit of patients, clinicians and the wider medical ecosystem. But to realise this potential requires true and deep cross-disciplinary understanding – a great challenge because we speak different languages. CCAIM is designed to break down the barriers between machine learning and medical science, to create a unique forum in which we can work together to truly understand the challenges, formalise the problems, and develop practical solutions that can be readily implemented in healthcare.”
Professor Andre Floto said: “We are thrilled that the CCAIM is taking off. From tackling the immediate threats of COVID-19 , to the long-term transformation of healthcare systems, our network of experts and incoming PhD students will bring next-level AI to bear on the most pressing medical issues of our time.”
Professor Andy Neely OBE , Pro-Vice-Chancellor for Enterprise and Business Relations, University of Cambridge, said: “The CCAIM is a terrific and timely venture that builds on the strong relationships between the University of Cambridge and global leaders in the pharmaceutical industry, AstraZeneca and GSK. The depth and diversity of the CCAIM faculty’s expertise means it is uniquely positioned to deliver and accelerate the breakthroughs in medical science and healthcare that AI has long promised. I anticipate the Centre’s impact will be nothing less than transformational.”
Jim Weatherall, Vice President, Data Science & AI, R&D, AstraZeneca, said: “We know the best science doesn’t happen in isolation which is why collaboration is essential to the way we work. This new centre combines world class academia with real-world industrial challenges and will help to develop cutting-edge AI to potentially transform the way we discover and develop medicines.”
Kim Branson, Senior Vice President and Global Head of AI/ML, GSK, said: “The new CCAIM will recruit and train the next generation of practitioners at the intersection of AI, industry and academia. The work of this institute will be critical to translating AI methods from theory to practice, so that we can keep improving our therapeutic discovery efforts and so that together we can make a tangible impact on patients, from diagnosis, to treatment and beyond.”
Biographies
Professor Mihaela van der Schaar, CCAIM Director
Mihaela van der Schaar is John Humphrey Plummer Professor of Machine Learning, Artificial Intelligence and Medicine at the University of Cambridge, where she directs the Cambridge Centre for AI in Medicine and heads up the van der Schaar Lab. In 2019, National Endowment for Science, Technology and the Arts (NESTA), identified Professor van der Shaar as the most-cited female AI researcher in the UK. In 2020, she was among the top 10 authors not only at ICML, but also at NeurIPS, two of the world’s most prestigious machine learning conferences. Professor van der Schaar is also a Turing Faculty Fellow at The Alan Turing Institute in London, a Chancellor’s Professor at UCLA and an IEEE Fellow. A fuller biography, including information on Professor van der Schaar’s many awards and patents, is available here .
Professor Andres Floto, CCAIM Co-Director
Andres Floto is a Professor of Respiratory Biology at the University of Cambridge, a Wellcome Trust Senior Investigator, and Research Director of the Cambridge Centre for Lung Infection at Papworth Hospital, Cambridge. Clinically, he specialises in the treatment of patients with Cystic Fibrosis (CF), non-CF bronchiectasis, and infections with nontuberculous mycobacteria.
Professor Floto research explores how immune cells interact with bacteria, how intracellular killing and inflammation are regulated and sometimes subverted during infection, how population-level whole-genome sequencing can be used to reveal biology of bacterial infection, and how therapeutic enhancement of cell-autonomous immunity may provide novel strategies to treat multi-drug-resistant pathogens.
Dr James Weatherall, Vice President, Data Science & AI, R&D, AstraZeneca
Since joining AstraZeneca in 2007, Dr Weatherall has held diverse roles focused on driving the application of data science, artificial intelligence, advanced analytics and related approaches to unlock the full potential of data – transforming the way medicines are discovered and developed and making a difference to patients’ lives. Dr Weatherall is an Honorary Reader in Computer Science at the University of Manchester and Vice-Chair of the Data Science Section at the Royal Statistical Society. He has contributed to and published in diverse fields such as data visualisation, cryptography, text mining, machine learning and health data science.
Dr Kim Branson, Senior Vice President and Global Head of AI/ML, GSK
Dr Kim Branson leads all GSK’s AI/ML initiatives and projects. Dr Branson has been involved in large scale machine learning and medical informatics initiatives for more than 15 years, over a range of ventures from computational drug design to disease risk prediction.
Dr Branson received degrees from the University of Adelaide, and a PhD from the University of Melbourne.
This article first appeared on the CCAIM website .
The text in this work is licensed under a Creative Commons Attribution-NonCommercial-ShareAlike 4. 0 International License . Images, including our videos, are Copyright ©University of Cambridge and licensors/contributors as identified. All rights reserved. We make our image and video content available in a number of ways that permit your use and sharing of our content under their respective Terms.

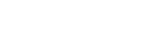
- Spotlight Series
- WhitePapers
- Top Companies to Work For
- Analytics/AI
- Cybersecurity
- Finance/Revenue Cycle
- Interoperability & HIE
- Clinical IT
- Artifical Intelligence/Machine Learning
Harvard Medical School Creating AI in Medicine Ph.D. Track
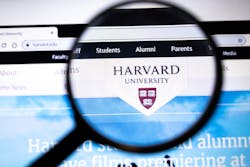
The Department of Biomedical Informatics (DBMI) at Harvard Medical School is creating an AI in Medicine Ph.D. track to prepare the next generation of leaders at the intersection of artificial intelligence and medicine. Applications are opening in September 2023 for a program starting in the fall of 2024.
The Artificial Intelligence in Medicine (AIM) PhD track will be led by co-directors DBMI Chair Isaac “Zak” Kohane and Harvard Medical School Professor of Medicine and Epidemiology Sebastian Schneeweiss.
The program’s mission is to train exceptional computational students, harnessing large-scale biomedical data and cutting-edge AI methods, to create new technologies and clinically impactful research that transform medicine around the world, increasing both the quality and equity of health outcomes.
DBMI stresses that the program transcends traditional boundaries between fields such as statistics, computer science, bioinformatics, artificial intelligence, epidemiology, and clinical medicine, fostering interdisciplinary collaboration and innovation. Students will work to acquire the skills to build tools and infrastructure that improve individual and population health, addressing the needs of patients, providers, and clinical care systems alike.
The trainees will take clinical coursework at Harvard Medical School and perform hospital rotations alongside medical students and other Ph.D. trainees from Harvard and MIT.
The cornerstone of the required core courses in the program is the flagship AI in Medicine I & II sequence, taught by leading AI researchers at DBMI, including Kohane, Arjun Manrai, Chirag Patel, Pranav Rajpurkar, Kun Hsing-Yu, and Marinka Zitnik. This sequence will give students the knowledge to create AI that cuts across the latest modalities in fields such as computer vision, generative language models, and graph neural networks, incorporating diverse data types to improve clinical decision-making and biomedical research.
Another aspect of the AIM track is its co-mentorship model, bringing together both methodological and applied clinical mentorship for each student’s research. With the help of program leadership, students will select one technical mentor in addition to one hospital-based clinical mentor by the end of the second year. This model enables significant value exchange between DBMI and affiliated hospitals while providing our students mentorship that increases the translational impact of their work.
Also launching in the fall of 2024 will be a Master of Medical Sciences in Biomedical Informatics (MMSc-BMI) degree program. Led by DBMI Associate Professor Nils Gehlenborg, it is a two-year thesis master's program for candidates with a baccalaureate or postgraduate degree interested in rigorous didactic and mentored research training in biomedical informatics. MMSc trainees will complete coursework during the first year and engage in a thesis research project under the mentorship of a Harvard Medical School faculty member during their second year. The MMSc-BMI program will be an enhanced version of its predecessor, the Master of Biomedical Informatics (MBI) program, by offering a more rigorous research experience that includes two semesters dedicated to research work and a longer tenure in the Harvard ecosystem.
For the MMSc-BMI program, applications for fall 2024 admission will open in October of 2023.
Continue Reading
New England Journal of Medicine Wades Into Artificial Intelligence
Why UT Health San Antonio, UTSA Created First M.D./M.S. Program in AI
Sponsored recommendations.
Overcoming capacity constraints: Top healthcare leaders share their strategies
MemorialCare Boosts Nurse Engagement and Retention
How to Construct a Sustainable GRC Program in 8 Steps
A Cyber Shield for Healthcare: Exploring HHS's $1.3 Billion Security Initiative
Latest in artifical intelligence/machine learning.
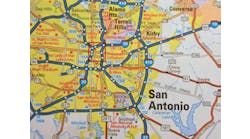
UW Health, Epic Host National Roundtable on Harnessing AI in Healthcare
NAACOS Names New CEO
How Do Patients Feel About Social Risk Screening?
What Is Involved in Creating a Care Operating System?
Spotlight on Artificial Intelligence
Transforming healthcare organizations: Embracing Generative AI in clinical workflows
Trailblazing Technologies: Looking at the Top Technologies for the Emerging U.S. Healthcare System
We have 18 Medicine (artificial intelligence) PhD Projects, Programmes & Scholarships in the UK
United Kingdom
Institution
All Institutions
All PhD Types
All Funding
Medicine (artificial intelligence) PhD Projects, Programmes & Scholarships in the UK
Automated detection and analysis of cancers using artificial intelligence, phd research project.
PhD Research Projects are advertised opportunities to examine a pre-defined topic or answer a stated research question. Some projects may also provide scope for you to propose your own ideas and approaches.
Self-Funded PhD Students Only
This project does not have funding attached. You will need to have your own means of paying fees and living costs and / or seek separate funding from student finance, charities or trusts.
Developing an Artificial Intelligence (AI)-based self-training platform for laparoscopic surgery.
Investigating inequalities in the safety of artificial intelligence triage in general practice, funded phd project (uk students only).
This research project has funding attached. It is only available to UK citizens or those who have been resident in the UK for a period of 3 years or more. Some projects, which are funded by charities or by the universities themselves may have more stringent restrictions.
Exploring the value of using large third-party artificial intelligence models in epidemiology, with examples using Twitter/X data
Automated analysis of qualitative data using ai for patient safety, ai-generated genomes for privacy-preserving collaboration in human genomics, funded phd project (students worldwide).
This project has funding attached, subject to eligibility criteria. Applications for the project are welcome from all suitably qualified candidates, but its funding may be restricted to a limited set of nationalities. You should check the project and department details for more information.
Interdisciplinary Studentship in Human-Centred AI
Competition funded phd project (uk students only).
This research project is one of a number of projects at this institution. It is in competition for funding with one or more of these projects. Usually the project which receives the best applicant will be awarded the funding. The funding is only available to UK citizens or those who have been resident in the UK for a period of 3 years or more. Some projects, which are funded by charities or by the universities themselves may have more stringent restrictions.
Automating Literature Mining and Triangulation with AI and Knowledge Graphs
The integration of routine pharmacogenomics testing into general practice in the norfolk coast healthcare setting (patelm_u25nnhcfmh), phd in cyber-physical systems for medicine development and manufacturing, competition funded phd project (students worldwide).
This project is in competition for funding with other projects. Usually the project which receives the best applicant will be successful. Unsuccessful projects may still go ahead as self-funded opportunities. Applications for the project are welcome from all suitably qualified candidates, but potential funding may be restricted to a limited set of nationalities. You should check the project and department details for more information.
Improving the understanding of risk, outcomes, and treatment of E. coli infections in people with multimorbidity
The genetic map of human molecular phenotypes, early alzheimer's disease diagnosis using deep learning retinal image analysis phd, severity grading and biomarkers for retinal vasculitis, exploring sensorimotor function and developing rehabilitation strategies using experimental and computational approaches.
FindAPhD. Copyright 2005-2024 All rights reserved.
Unknown ( change )
Have you got time to answer some quick questions about PhD study?
Select your nearest city
You haven’t completed your profile yet. To get the most out of FindAPhD, finish your profile and receive these benefits:
- Monthly chance to win one of ten £10 Amazon vouchers ; winners will be notified every month.*
- The latest PhD projects delivered straight to your inbox
- Access to our £6,000 scholarship competition
- Weekly newsletter with funding opportunities, research proposal tips and much more
- Early access to our physical and virtual postgraduate study fairs
Or begin browsing FindAPhD.com
or begin browsing FindAPhD.com
*Offer only available for the duration of your active subscription, and subject to change. You MUST claim your prize within 72 hours, if not we will redraw.
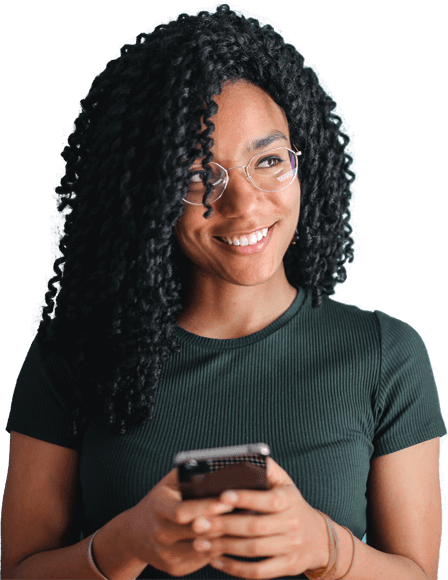
Do you want hassle-free information and advice?
Create your FindAPhD account and sign up to our newsletter:
- Find out about funding opportunities and application tips
- Receive weekly advice, student stories and the latest PhD news
- Hear about our upcoming study fairs
- Save your favourite projects, track enquiries and get personalised subject updates

Create your account
Looking to list your PhD opportunities? Log in here .
Filtering Results

- Weill Cornell Medicine

Artificial Intelligence in Medicine I
Course Director: Fei Wang, Ph.D.
Introduces students to a variety of analytic methods for health data using computational tools. The course covers topics in data mining, machine learning, classification, clustering and prediction. Students engage in hands-on exercises using a popular collection of data mining algorithms.
The Lab for AI in Medicine at TU Munich develops algorithms and models to improve medicine for patients and healthcare professionals.
Our aim is to develop artificial intelligence (AI) and machine learning (ML) techniques for the analysis and interpretation of biomedical data. The group focuses on pursuing blue-sky research, including:
- AI for the early detection, prediction and diagnosis of diseases
- AI for personalised interventions and therapies
- AI for the identification of new biomarkers and targets for therapy
- Safe, robust and interpretable AI approaches
- Privacy-preserving AI approaches
We have particularly strong interest in the application of imaging and computing technology to improve the understanding brain development (in-utero and ex-utero), to improve the diagnosis and stratification of patients with dementia, stroke and traumatic brain injury as well as for the comprehensive diagnosis and management of patients with cardiovascular disease and cancer.
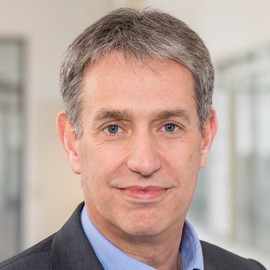
Daniel Rückert
Professor of artificial intelligence in healthcare and medicine.
Medical Image Computing, Data Science in Medicine, Artifical Intelligence in Medicine
Team Support
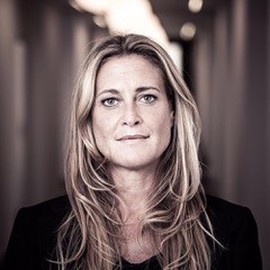
Deborah Carraro
Executive assistant.
Project Management and Administration, Team Management and Support, Communication and Relations
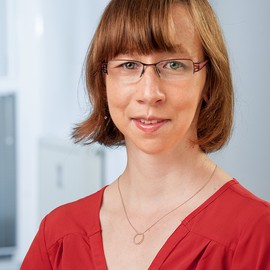
Sabine Franke
Adminstrative assistant, senior researchers.
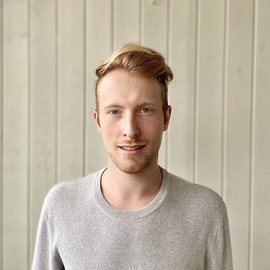
Johannes C. Paetzold
Research scientist.
Graph representation learning, Computer vision, Biomedical image analysis
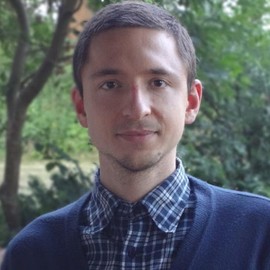
Computational oncology, Physics-based machine learning, Inverse problems
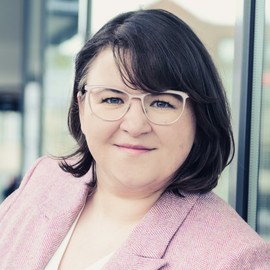
Dr. rer. nat. Simone Gehrer
Scientific manager.
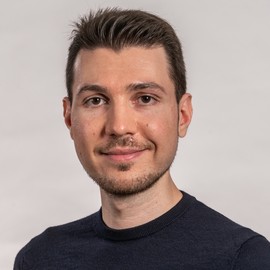
Georgios Kaissis
Senior research scientist.
Privacy-preserving artificial intelligence, Medical image computing, Probabilistic methods
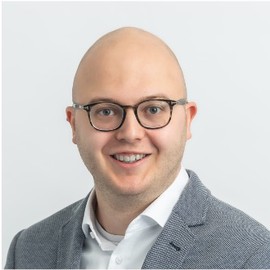
Martin Menten
Weakly and unsupervised deep learning, Generative modeling, Ophthalmologic imaging
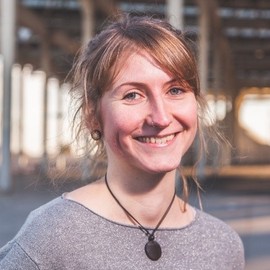
Veronika Zimmer
Medical Image Computing, Ultrasound Image Analysis, Fetal Image Analysis
Researchers
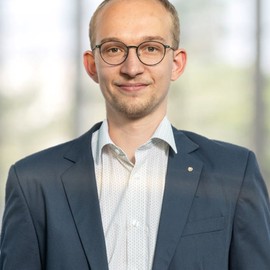
Márton Szép
Phd student.
Natural Language Processing in Medicine, Generative Models, Multimodal AI
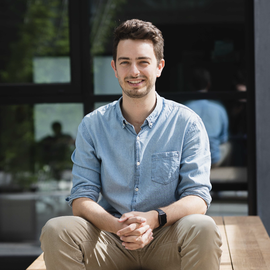
Florian A. Hölzl
Artifical Intelligence in Medicine, Privacy-preserving Deep Learning
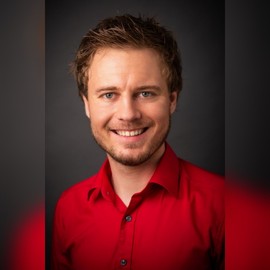
Alexander Ziller
Artifical Intelligence in Medicine, Privacy-preserving Machine Learning
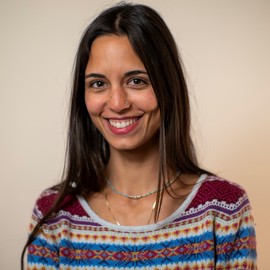
Anna Curto Vilalta
Foundation Models, Multi-Modal Deep Learning, AI in Medical Imaging
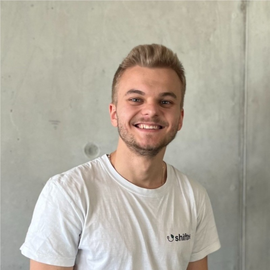
Alexander Berger
3D Medical Imaging, Weakly- and Self-supervised Transfer Learning, Domain Adaptation
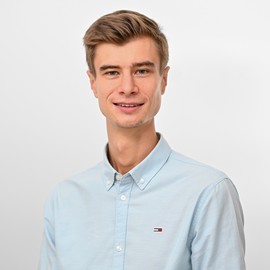
Alexander Selivanov
AI in Medical Imaging, Multimodal Learning, Self-supervised Learning
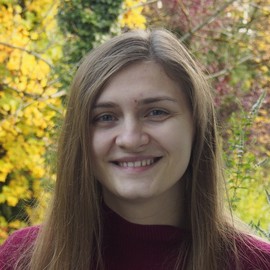
Medical Imaging, Semantic Segmentation, Pancreatic Ductal Adenocarcinoma
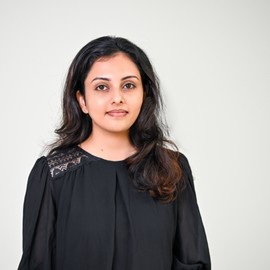
Medical Imaging Computing
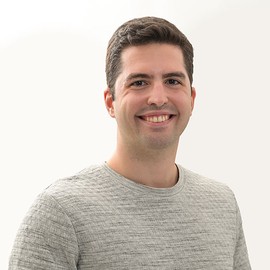
Ayhan Can Erdur
medical imaging, 3D computer vision, disease outcome prediction
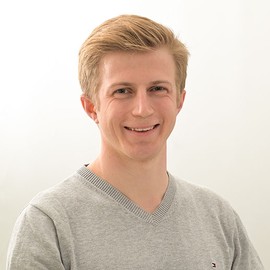
Daniel Scholz
self-supervised learning, representation learning, 3d image segmentation of brain MRIs
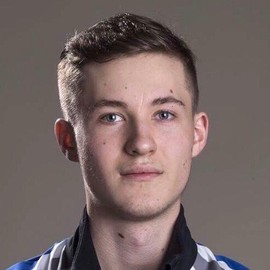
Dmitrii Usynin
Artifical Intelligence in Medicine, Secure and Private Artificial Intelligence
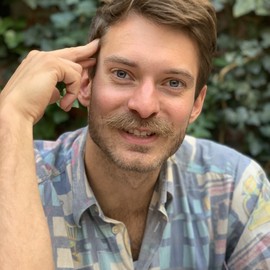

Felix Meissen
Anomaly Detection, Transfer Learning, Generative Models, Bayesian Learning
Florent Dufour
AI in Medical Imaging, Trustworthy AI, Privacy Enhancing Technologies, Confidential Computing, Sovereign Cloud Computing
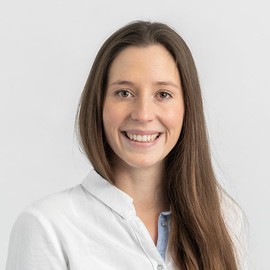
Friederike Jungmann
human-in-the-loop-machine learning, interpretable artificial intelligence, medical image computing
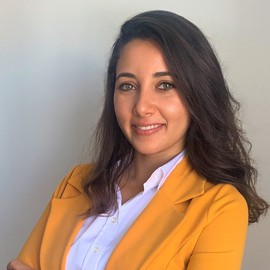
Artificial Intelligence in Medicine, Fairness and Bias in Healthcare
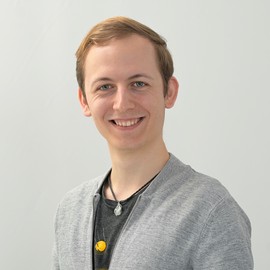
Hendrik Möller
MRI Segmentation, MRI Vertebrae Detection and Labeling, Transitional Vertebrae
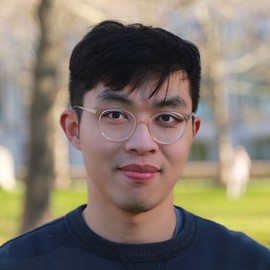
Jiazhen Pan
Medical Imaging Computing, Semantic Segmentation, Medical Image Reconstruction
Johannes Kaiser
AI in Medical Imaging, Privacy-preserving Machine Learning, Trustworthy Machine Learning
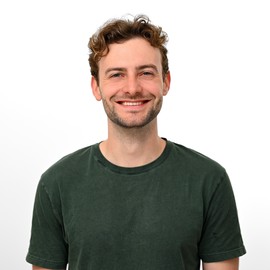
Jonas Kuntzer
Mechanistic interpretability, Differential privacy
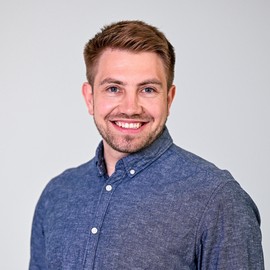
Jonas Weidner
Personalized brain tumor modeling, Physics-informed neural networks, Diffusion tensor imaging, Topological data analysis
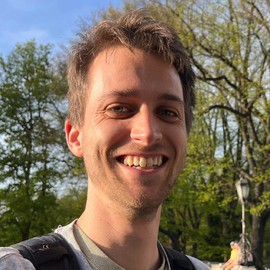
Julian McGinnis
Medical Imaging, Implicit Neural Representations, Multiple Sclerosis Research
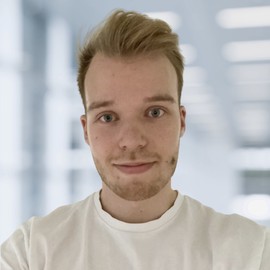
Kristian Schwethelm
AI in Medical Imaging, Privacy-preserving Machine Learning, Differential Geometry
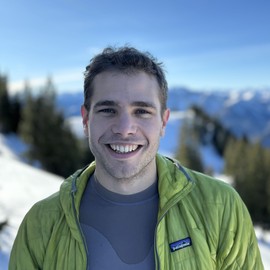
AI in Biomedical Imaging, Graphs in Medical AI, Interpretable AI
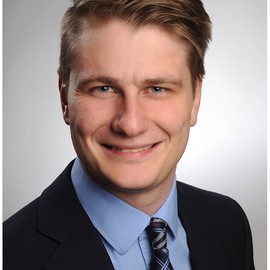
Leonhard Feiner
Machine Learning and Deep Learning, Medical Image Computing, Data Science
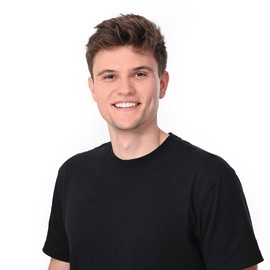
Linus Kreitner
Weakly- and Selfsupervised Machine Learning, Network Dissection and Explainability, Causal Inference
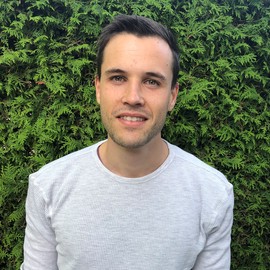
Maik Dannecker
Medical Imaging, Deep Learning, Biomarker Discovery, Demystifying The Human Brain
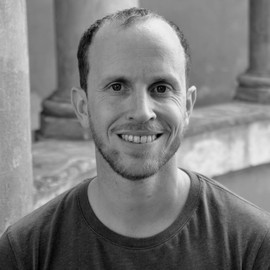
Generative Models and Latent Spaces, Unsupervised Learning, Graph Neural Networks
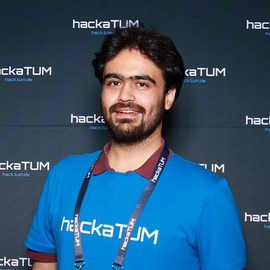
Maulik Cevalī
Privacy-preserving ML, Trustworthy ML, Applied AI in Medicine
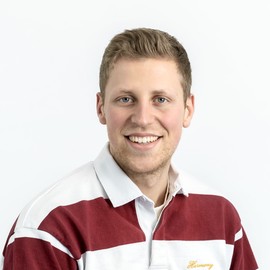
Moritz Knolle
Differential Privacy, Fair & Trustworthy ML, Memorisation in Neural Networks
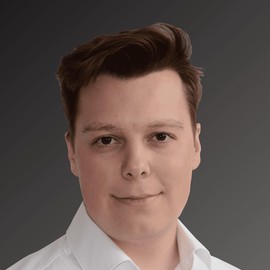
Niklas Bubeck
Generative AI in Medical Imaging, Medical Image Reconstruction, Multi-Modal Foundation Models
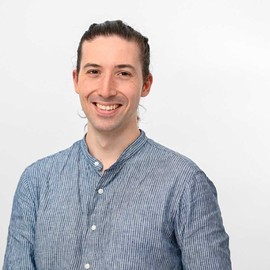
Nil Stolt-Ansó
Medical Image Segmentation, Image Registration, Geometric Deep Learning
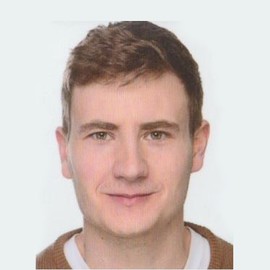
Medical Imaging Computing, Multi-modal Deep Learning, Genetics
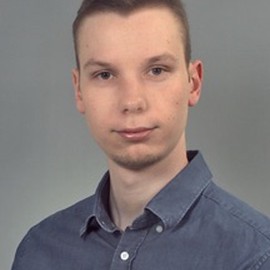
Philip Müller
Multi-Modal Learning, Natural Language Processing, Geometric Deep Learning
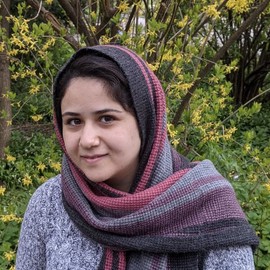
Reihaneh Torkzadehmahani
Phd student.
Privacy-preserving Machine Learning, Meta and Transfer Learning, Generative Models
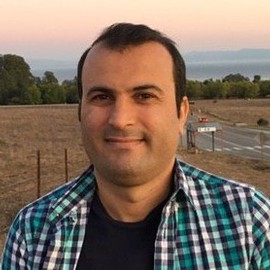
Reza Nasirigerdeh
Privacy-preserving machine learning, Distributed systems, Medical imaging
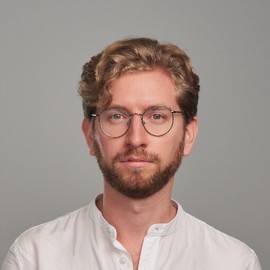
Ricardo Smits Serena
Medical Wearable Technology, Time Series Classification, Gait Analysis
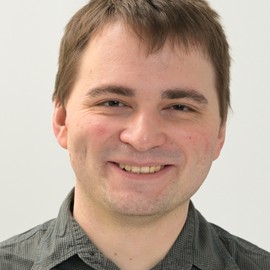
Robert Graf
Computer Vision for Spine Processing, Image2Image, Denoising Diffusion, Large Epidemiological Studies
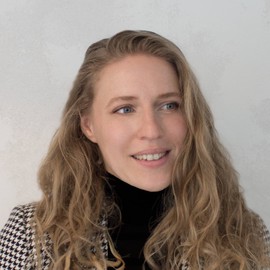
Sarah Lockfisch
AI in Medical Imaging, Interpretability in Deep Learning, Uncertainty Quantification
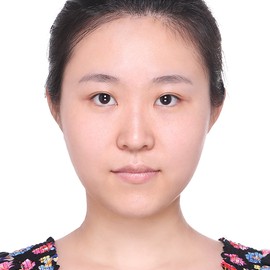
Shuting Liu
Multi-modality Image Analysis, Domain Transfer
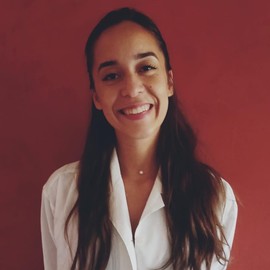
Sophie Starck
Machine Learning, Geometric Deep Learning, Medical Image Computing
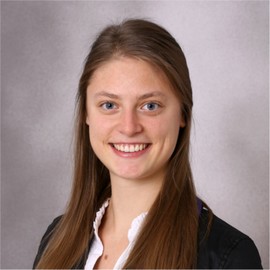
Tamara Müller
Artificial Intelligence in Medicine, Geometric Deep Learning, Computational Neuroscience
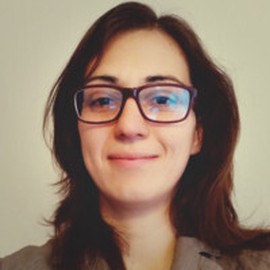
Vasiliki Sideri-Lampretsa
Artifical Intelligence in Medicine, Machine Learning and Deep Learning, Medical Imaging

Wenke Karbole
Generative AI, Temporal Representation Modeling, Ophthalmologic Imaging
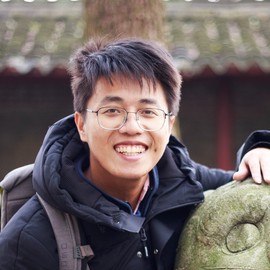
Wenqi Huang
Image Reconstruction, Multi-Task Deep Learning
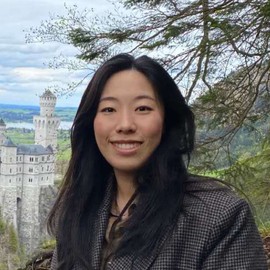
Yundi Zhang
Deep Learning, Medical Image Computing, MRI
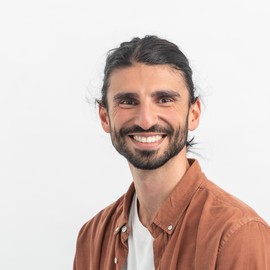
Özgün Turgut
Signal processing, Self-supervised learning, Multimodal AI
Collaborators
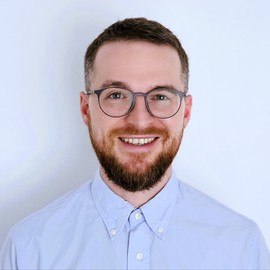
Florian Hinterwimmer
Affiliated researcher.
Multimodal machine learning, Applied AI in musculoskeletal medicine, Data engineering and medical informatics
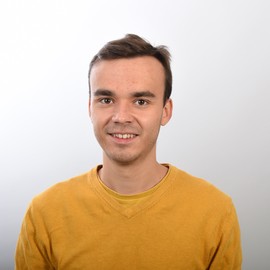
Franz Rieger
Affliated researcher.
ML for connectomics, Self-supervised segmentation, ML for code
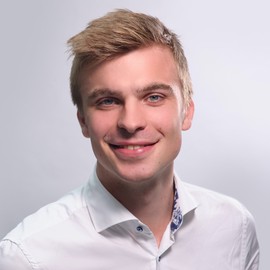
Henrik von Kleist
Interpretable AI, Uncertainty quantification in ML, Causal inference
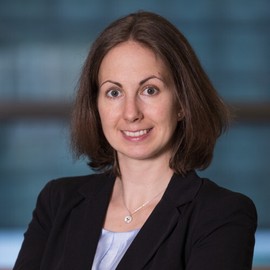
Kerstin Hammernik
Inverse Problems, Machine Learning, MRI, Medical Image Computing
New Module IN2409: Inverse Problems in Medical Imaging

Recent Publications
The developing human connectome project (dhcp) automated resting-state functional processing framework for newborn infants, model-based and data-driven strategies in medical image computing.
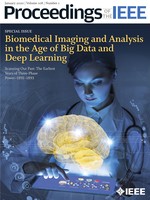
Secure, privacy-preserving and federated machine learning in medical imaging
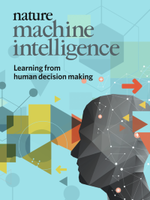
A population-based phenome-wide association study of cardiac and aortic structure and function
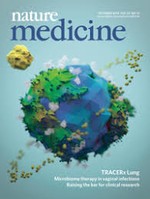
Genetic and functional insights into the fractal structure of the heart
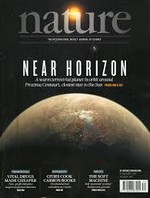
MSc Thesis: Large Language Models in Medicine
Description: Large Language Models (LLMs) have shown exceptional capabilities in understanding and generating human-like text. In the medical field, these models hold the potential to revolutionize patient care, medical research, and healthcare administration.
MSc Thesis: Leveraging Differential Privacy to Learn General and Robust Deep Learning Models
Description Deep learning aims at learning general representations of data allowing for downstream tasks such as classification, regression or generation of new data. In practice, however, there are no formal guarantees to what a model learns, resulting in unwanted memorisation of input data and leaking of private information.
MSc Thesis: Outperforming CNNs and Transformers on Medical Imaging Tasks with Equivariant Networks
Description Equivariant convolutions are a novel approach that incorporate additional geometric properties of the input domain during the convolution process (i.e. symmetry properties such as rotations and reflections) [1]. This additional inductive bias allows the model to learn more robust and general features from less data, rendering them highly promising for application in the medical domain.
MSc Thesis: Privacy-Preserving Synthetic Time Series Data of Electronic Health Records
Description Anonymizing data means removing or replacing any identifying information from a dataset, such as names or addresses. The aim of anonymization is to protect the privacy of individuals whose data is being collected and processed.
Master-Seminar: Multi-modal AI for Medicine (IN2107)
This year’s seminar will look at aspects of multi-modal machine learning in medicine and healthcare, focusing on: Vision language models (VLMs) for medical and healthcare applications Generic multi-modal AI models utilising imaging data, clinical reports, lab test results, electronic health records, and genomics Foundation models for multi-modal medicine Objectives: At the end of the module students should have:
Practical Course: Applied Deep Learning in Medicine
In this course students are given the chance to apply their abilities and knowledge in deep learning to real-world medical data. Students will be assigned a medical dataset and in close consultation with medical doctors create a project plan.
Master Thesis: Deep Learning for Bone Tumor Detection and Segmentation: 2D vs 3D
Abstract: The detection and segmentation of bone tumors using magnetic resonance imaging (MRI) have crucial implications for clinical diagnosis and treatment planning. With the advent of deep learning techniques, there’s a growing interest in leveraging these methods to analyze MRI bone tumor images.
MSc Thesis: Diffusion-based Topology-preserving Medical Image Segmentation
This project can be hosted in Munich and/or Zurich @Biomedical Image Analysis & Machine Learning Group, University of Zurich. Background: Topology is vital in medical image segmentation, emphasizing anatomically correct structures & removing incorrect ones.
IDP/Thesis: Physics-based deep learning for hyperspectral brain surgery imaging
Hyperspectral imaging (HSI) is an optical technique that processes the electromagnetic spectrum at a multitude of monochromatic, adjacent frequency bands. The wide-bandwidth spectral signature of a target object’s reflectance allows fingerprinting its physical, biochemical, and physiological properties.
IDP: iOS app for wearable health data management
Description In an era where technology has seamlessly integrated with our day-to-day lives, health and fitness tracking has seen a revolutionary change. Gone are the days when we passively absorbed health information.
MSc Thesis: Contrastive Learning and Generative Models for Cross-Domain Transfer Learning
In this Master thesis we aim to approach the cross-domain transfer learning problem with two powerful methods that help us to bridge the domain gap between source and target domain: contrastive learning [1] and generative models.
We are recruiting team members who would like to join us for a MSc, BSc or guided research/interdisciplinary project on an ongoing basis! Please look under Teaching to find out which projects we are currently offering. If you’d like to join us for one of these projects, please get in touch by contacting the appropriate staff member via e-mail and attach a motivation letter, transcript of academic records and CV.
Current vacancies
Currently no positions are available.
Internships
Unfortunately we cannot host any external students for internships.
- [email protected]
- +49 89 4140-8851
- TranslaTUM, Einsteinstraße 25 81675 München
- Chagas Disease Alliance at Yale (CDAY)
- Faculty Labs
- Publications
- Clinical Study Guidance
- Core Laboratory Analysis and Committee Management
- Imaging Resources
- Consultation
- Postdoctoral Training Program
- Intracardiac Imaging and Intervention Training
- Faculty & Staff
- Information For Users
- Clinical Programs
- General Cardiovascular Medicine Fellowship
- Adult Congenital Heart Disease Fellowship
- Advanced Cardiac Imaging Fellowship
- Advanced Heart Failure and Transplantation Fellowship
- Advanced Electrophysiology Fellowship
- Advanced Interventional Cardiology Fellowship
- Advanced Endovascular Interventions Fellowship
- Advanced Structural Heart Disease Interventions Fellowship
- Advanced Cardio-Oncology Fellowship
- Cardiovascular Research Training Fellowships
- Current Fellows
- Customizing Your Education
- Clinical Trials
INFORMATION FOR
- Residents & Fellows
- Researchers
2024 AI in Medicine Symposium at Yale School of Medicine
🧬 🤖 Join our groundbreaking symposium for medical professionals and technology enthusiasts! Are you eager to explore the cutting-edge intersection of artificial intelligence and medicine?
Our AI in Medicine Symposium, led by Andrew Taylor, MD, MHS , is curated to offer you deep insights into this rapidly evolving field. Join us for this comprehensive symposium on Friday, February 2.
Discover how AI is transforming biomedical imaging, NLP, clinical applications, bioinformatics, and more. Engage in dynamic lightning talks, panel discussions, and a poster session that will enrich your understanding and network in the realm of medical AI.
The symposium is open to all members of the Yale community. Please register for the symposium here. A virtual option is available as well for remote attendees, but the poster sessions will be in-person only.
8:30 - 9 AM Opening Remarks
Dean Nancy J. Brown, MD, Jean and David W. Wallace Dean of the Yale School of Medicine and C.N.H.Long Professor of Internal Medicine
Lucila Ohno-Machado, MD, MBA, PhD, Waldemar von Zedtwitz Professor of Medicine and Biomedical Informatics and Data Science; Deputy Dean for Biomedical Informatics
9 - 10 AM Lightning Talks I
Biomedical Imaging/Computer Vision
Moderated by Rohan Khera, MD, MS
Enhancing Brain Positron Emission Tomography Image Quality with Artificial Intelligence Head Motion Correction. Eléonore V. Lieffrig, Biomedical Engineering A Multimodality Video-Based Artificial Intelligence Biomarker for Aortic Stenosis Development and Progression. Evangelos K. Oikonomou, MD, DPhil , Internal Medicine (Cardiology) Enhancing Prostate Cancer Diagnosis from Medical Imaging via Image Geometry-Informed Deep Learning. Joanna Chen , Biomedical Engineering, MD-PhD Program Valvular Flow MRI: 2D Phase-Contrast of the Tricuspid Valvular Flow with Automated Valve-Tracking by Deep Learning. Dana Peters, PhD , Radiology
10 - 11 AM Panel I
Generative AI in Medical Education, Basic Science, Clinical Practice
Moderated by Annie Hartley, MD, PhD, MPH
Hua Xu, PhD Robert T. McCluskey Professor of Biomedical Informatics and Data Science; Vice Chair for Research and Development, Section of Biomedical Informatics and Data Science; Assistant Dean for Biomedical Informatics, Yale School of Medicine Rohan Khera, MD, MS Assistant Professor of Medicine (Cardiovascular Medicine) and of Biostatistics (Health Informatics); Clinical Director, Center for Health Informatics and Analytics, YNHH/Yale Center for Outcomes Research & Evaluation (CORE); Director, Cardiovascular Data Science Lab (CarDS) Mark Gerstein, PhD Albert L Williams Professor of Biomedical Informatics, Professor of Molecular Biophysics & Biochemistry, Professor of Computer Science, and Professor of Statistics & Data Science
11 - 11:15 AM Coffee Break
11:15 AM - 12:15 PM Lightning Talks II
Generative AI & Natural Language Processing (NLP)
Moderated by Arman Cohen, PhD
Call It A Night: Leveraging Natural Language Processing to Evaluate User Experiences with a Digital Sleep Intervention to Reduce Drinking. Frances J. Griffith, PhD , Psychiatry Assessing the Usability of GutGPT: A Simulation Study of an AI Clinical Decision Support System for Gastrointestinal Bleeding Risk. Colleen Chan and Sunny Chung, MD , Statistics & Data Science Rethink Biomedical Literature Search and Visualization in the Era of Large Language Models – A Prototype Development. Huan He, PhD , Biomedical Informatics & Data Science Me LLaMA: A Suite of Large Language Models, Datasets, and Tools for Medical Application. Qianqian Xie, PhD , Biomedical Informatics & Data Science
12:15 - 1:15 PM Lunch and Poster Session
1:15 - 2:15 PM Lightning Talks III
Clinical Applications of AI
Moderated by Dennis L Shung, MD, MHS, PhD
Enhancing Clinical Decision Support Accuracy Through a Retrieval Augmented Generation Pipeline for Large Language Models. Mauro Giuffrè, MD , Internal Medicine (Digestive Disease) MEDAGENTS: Large Language Models as Collaborators for Zero-Shot Medical Reasoning. Xiangru Tang, Computer Science ECG-GPT: Automated Complete Diagnosis Generation from ECG Images Using Novel Vision-Text Transformer Model. Akshay Khunte, Computer Science, Internal Medicine (Cardiovascular Medicine) Neural Networks for Kidney Injury Decision Making. William J. Zhang , Chronic Disease Epidemiology
2:15 - 3:15 PM Panel II
Yale New Haven Health System-Yale School of Medicine AI Partnerships
Moderated by Wade Schulz, MD, PhD
Lee Schwamm, MD Associate Dean, Digital Strategy and Transformation, Office of the Dean, Yale School of Medicine; Professor in Biomedical Informatics & Data Sciences at Yale School of Medicine; Senior Vice President and Chief Digital Health Officer, Yale New Haven Health System Allen Hsiao, MD, FAAP, FAMIA Professor of Pediatrics (Emergency Medicine) and of Emergency Medicine; Interim Chief, Pediatric Emergency Medicine; Chief Health Information Officer, Yale School of Medicine & Yale New Haven Health, Yale School of Medicine Daniella Meeker, PhD Associate Professor of Biomedical Informatics and Data Science; Chief Research Information Officer, Yale School of Medicine and Yale New Haven Health System
3:15 - 3:30 PM Coffee Break
3:30 - 4:30 PM Lightning Talks IV
Clinical Applications & Bioinformatics
Moderated by Andrew Taylor, MD, MHS
RCT-Twin-GAN Generates Digital Twins of Randomized Control Trials Adapted to Real-World Patients to Enhance Their Inference and Application. Phyllis Thangaraj, MD, PhD , Cardiology A Roadmap to Artificial Intelligence (AI): Methods for Designing and Building AI Ready Data to Promote Fairness. Farah Kidwai-Khan, DEng , General Internal Medicine, Biomedical Informatics & Data Science Quantum Clique Detection in Biological Graph Networks. Sarah N. Dudgeon , Computational Biology & Bioinformatics Grappling with 10 60 : Geometric Deep Learning for Drug Discovery & Indication Expansion. Dhananjay Bhaskar, PhD , Genetics
4:40 - 4:45 PM Closing Remarks
Andrew Taylor, MD, MHS
4:45 - 6 PM Reception & Poster Session
List of Posters
Artificial Intelligence in Medical Imaging Physics: PET, SPECT, and CT Chi Liu, PhD Artificial Intelligence-Based Morpho-Volumetric Analysis of Pre- and Post-EVAR Infrarenal Abdominal Aortic Aneurysms Characterized on Computed Tomography Angiography David Weiss, MD; Thomas Hager, BS; Mariam Aboian, MD, PhD; MingDe Lin, PhD; Daniel Renninghoff, MSc; Wolfgang Holler, MSc; Uwe Fischer, MD, PhD; Cornelius Deuschl, MD; Sanjay Aneja, MD; Edouard Aboian, MD Evaluating the Utility of Federated Learning Algorithms for Diagnostic Medical Imaging in Oncology Durga V. Sritharan, BS; Ryan Maresca, BS; Saahil Chadha, BA; Nicholas S. Moore, MD; Victor Lee, MD; Thomas Hager, MS; Sanjay Aneja, MD A Novel Vision Transformer-Based Pipeline for Direct Inference on Single-Lead Electrocardiographic Spectrograms Elizabeth Knight MS; Evangelos K. Oikonomou MD, DPhil; Akshay Khunte; Arya Aminorroaya, MD, MPH; Lovedeep Singh Dhingra, MBBS; Andreas Coppi, PhD; Rohan Khera, MD, MS Neuroimage Analysis in Autism: From Model-based Estimation to Data-Driven Learning Jiyao Wang, Nicha Dvorak, Xiaoxiao Li, Juntang Zhuang, Larry Staib, Denis Sukhodolsky, Pam Ventola, James S Duncan Integrating Multimodal Data to Automatically Segment Lesions in Prostate MRI Jiayang Zhong; Lawrence H. Staib, PhD; Rajesh Venkataraman, PhD; John A. Onofrey, PhD Identification of Hypertrophic Cardiomyopathy on Electrocardiographic Images with Deep Learning Lovedeep Singh Dhingra, MBBS; Veer Sangha, BS; Evangelos K. Oikonomou, MD, DPhil; Arya Aminorroaya, MD, MPH; Nikhil V. Sikand, MD, FACC; Sounok Sen, MD; Harlan M. Kumholz, MD, SM; Rohan Khera, MD, MS
Beyond Result Reporting on the Testing Set: Enhancing AI-Assisted Medical Imaging Diagnostic Workflow, External Validations, and Continued Training A Case Study on AI-Assisted Age-Related Macular Degeneration Diagnosis Qingyu Chen, PhD; Tiarnan D. L. Keenan, BM, BCh, PhD; Michael F. Chiang, MD, MA; Michelle R. Hribar, PhD; Emily Y. Chew, MD; Zhiyong Lu, PhD Evaluating the Utility of Self-Configuring Capsule Networks for Brain Image Segmentation Saahil Chadha, BA; Arman Avesta, MD, PhD; Durga Sritharan, BS; Sajid Hossain, MS; Rahul D’Souza; MingDe Lin, PhD; Mariam Aboian, MD, PhD; Harlan Krumholz, MD, SM; Sanjay Aneja, MD Semi-Automatic Approach to Estimate the Severity of Steatosis from Ultrasound Images Simone Kresevic, Mauro Giuffrè, MD; Milos Ajcevic, PhD; Carlo Moretto; Lory Saveria Crocè, MD; Dennis L. Shung, MD, MHS, PhD; Agostino Accardo, MEng Monte-Carlo Frequency Dropout for Predictive Uncertainty Estimation in Deep Learning Tal Zeevi; Lawrence H. Staib, PhD; John A. Onofrey, PhD Lymph Node Metastasis Prediction with Non-Small Cell Lung Cancer Histopathology Imaging Victor Lee, MD; Amber Loren O. King, MD; Durga Sritharan, BS; Nicholas S. Moore, MD; Saahil Chadha, BA; Ryan Maresca, BS; Tommy Hager, MS; Henry S. Park, MD, MPH; Sanjay Aneja, MD Attention Mechanisms Integrated Deep Learning for Differential Diagnosis of Hepatocellular Carcinoma on Multiphase Liver CT Yuenan Wang, PhD, DABR Automated Image Segmentation of Electron Microscopy Images from Hemostatic Thrombi Formed in vivo Ziyi Huang; Meghan Roberts; Catherine House; Sandra J. Young; Maurizio Tomaiuolo, PhD; Timothy J. Stalker, PhD; Lu Lu, PhD; Talid Sinno, PhD
Development and Multinational Validation of a Machine Learning-Based Optimization for Efficient Screening for Elevated Lipoprotein(a) Arya Aminorroaya MD, MPH; Lovedeep Singh Dhingra, MBBS; Evangelos K. Oikonomou, MD, DPhil; Seyedmohammad Saadatagah, MD; Phyllis Thangaraj, MD, PhD; Sumukh Vasisht Shankar, MS; Erica S. Spatz, MD, MHS; Rohan Khera, MD, MS Evaluating the Efficacy of Open-Source Large Language Models in Generating Patient Referral Letters for Specialist Psychiatric Evaluation Brennan Gibson Using Machine Learning to Predict Early Substance Use Using a Nationally Representative Sample of U.S. Adolescents Gaoqianxue Liu; Catherine Jeon; Jenny Meyer; Uzochukwu Imo; Kammarauche Aneni, MBBS, MHS Machine Learning-Based Prediction of Inhibitors and Activators of Alpha-glucosidase as Therapeutics for Type 2 Diabetes Mellitus and Pompe Disease Gertrude Asumpaame Alayine CarDS-Plus ECG Platform: Development and Feasibility Evaluation of a Multiplatform Artificial Intelligence Toolkit for Portable and Wearable Device Electrocardiograms Sumukh Vasisht Shankar, MS; Evangelos K. Oikonomou, MD, DPhil; Rohan Khera, MD, MS A Multicenter Evaluation of the Impact of Procedural and Pharmacological Interventions on Deep Learning-Based Electrocardiographic Markers of Hypertrophic Cardiomyopathy Lovedeep Singh Dhingra, MBBS; Veer Sangha, BS; Arya Aminorroaya, MD, MPH; Robyn Bryde, MD; Andrew Gaballa, MD; Adel H. Ali, MD; Nandini Mehra, MD; Harlan M. Krumholz, MD, SM; Sounok Sen, MD; Christopher M. Kramer, MD; Matthew W. Martinez, MD; Milind Y. Desai, MD, MBA; Evangelos K. Oikonomou, MD, DPhil; Rohan Khera, MD, MS The Art of the Discharge: Proposal for Implementing Generative AI in Healthcare Daniel Fitzgerald
PRESENT-SHD, An Ensemble Deep Learning Model to Automate Screening for Multiple Structural Heart Diseases on 12-Lead Electrocardiograms Lovedeep Singh Dhingra, MBBS; Arya Aminorroaya, MD, MPH; Akshay Khunte; Veer Sangha, BS; Sounok Sen, MD; Norrisa Haynes, MD, MPH; Harlan M. Krumholz, MD, SM; Rohan Khera, MD, MS An Artificial Intelligence Clinical Decision Support System in a Medical Simulation: Qualitative Feedback Niroop Rajashekar; Yeo Eun Shin; Yuan Pu; Sunny Chung; Kisung You; Mauro Giuffrè, MD; Colleen E. Chan; Theo Saarinen; Allen Hsiao; Jasjeet Sekhon; Ambrose H. Wong; Leigh V. Evans; Rene F. Kizilcec; Loren Laine; Terika McCall; Dennis L. Shung, MD, MHS, PhD Personalizing the Empiric Treatment of Gonorrhea Using Machine Learning Models Rachel Murray-Watson, PhD Prognostic Biomarkers of Intracerebral Hemorrhage Identified Using Targeted Proteomics and Machine Learning Algorithms Shubham Misra, PhD; Yuki Kawamura; Praveen Singh; Shantanu Sengupta; Manabesh Nath; Zuhaibur Rahman; Pradeep Kumar; Amit Kumar; Praveen Aggarwal; Achal K. Srivastava; Awadh K. Pandit; Dheeraj Mohania; Kameshwar Prasad; Nishant Kumar Mishra, MD, PhD; Deepti Vibha Lessons from Clinical Communication for Human Centered AI Zahra Abba Omar Pilot Surveillance for Herbal Natural Product Use and Interactions Assessment: Methodology and Insights from an Expanded National U.S. Database Yuelei (Emily) Fu; Termeh Feinberg, PhD, MPH Pedictive Modeling of Racial Disparity in Teenage Hospitalizations Ryan Wu, MS; Azadeh Miran, MS; Yan Cheng, PhD; Yijun Shao, PhD; Joseph L. Goulet, PhD; Qing Zeng-Treitler, PhD DocuMental: Enhancing Mental Health Documentation Through a Secure, Generative AI Workflow Thomas Fernandez, MD
The Endorsement of General and Artificial Intelligence Reporting Guidelines in Medical Informatics Journals: A Meta-Research Study Alyssa Grimshaw, MSLIS, MBA; Dennis L. Shung, MD, MHS, PhD Clinical Characteristics and Outcomes of Patients with Post-Stroke Epilepsy: Protocol for an Individual Patient Data Meta-Analysis Nishant Kumar Mishra, MD, PhD; Patrick Kwan, MD; Tomotaka Tanaka, MD, PhD; Katherina Sunnerhagen, MD; Jesse Dawson, MD; Yize Zhao, PhD; Shubham Misra, PhD; Selena Wang, PhD; Vijay K. Sharma, MD; Rajarshi Mazumder, MD; Melissa C. Funaro, MS; Masafumi Ihara, MD; John-Paul Nicolo, MD; David S. Liebeskind, MD; Clarissa L. Yasuda, MD, PhD; Fernando Cendes, MD; Terence J. Quinn, MD; Zongyuan Ge, PhD; Fabien Scalzo, PhD; Johan Zelano, MD; Scott E. Kasner, MD Enhancing Algorithmic Equity by Capturing Sources of Outcome Variance in Minoritized Populations Christopher Fields, PhD Transfer Learning on Physics-Informed Neural Networks for Tracking the Hemodynamics in the Evolving False Lumen of Dissected Aorta Mitchell Daneker; Shengze Cai; Ying Qian; Myzelev; Arsh Kumbhat; Xiaoning Zheng; He Li; Lu Lu Closing the AI Implementation Gap Jessica Morley, PhD Exploring Convolutional Neural Networks for Facial-Image-Based Diagnosis of Marfan Syndrome John A. Elefteriades, MD; Danny Saksenberg, BS; David Aronowitz, MBA; Mohammad A. Zafar, MBBS; Bulat A. Ziganshin, MD, PhD; Asanish Kalyanasundaram, MD You, Too, Can Responsibly Use Generative AI Michael Ljung Detection of Multiple Structural Heart Diseases Using a Novel Artificial Intelligence-Driven Algorithm for Noisy Real-World Single-Lead ECGs Arya Aminorroaya, MD, MPH; Akshay Khunte; Lovedeep Singh Dhingra, MBBS; Veer Sangham, BS; Evangelos K. Oikonomou, MD, DPhil; Sounok Sen, MD; Norrisa Haynes, MD, MPH; Harlan M. Krumholz, MD, SM; Rohan Khera, MD, MS Novel Application of Flow Matching to Model Dynamic Risk in ICU Patients with Sepsis Yuan Pu
Using Artificial Intelligence to Identify Opportunities to Include Sex and Gender Content in Medical Education Aeka Lakshmi Guru; Haleigh Larson; Kelsey Martin, MD; Margaret Pisani, MD; Carolyn Mazure, PhD Supporting Hematologic Tissue Bank Research Through LLM-Based Information Extraction from Real-World Data Erin Lee; Ahmad Kiwan, MD/PhD; Martin Matthews; Jennifer VanOudenhove, PhD; Sarah N. Dudgeon; Patrick Young, PhD; Thomas Durant, MD; Wade Schulz, MD, PhD; Stephanie Halene, MD, Dr Med Accelerated Extraction of Cardiac MRI Parameters Using Open-Source Clinical Large Language Models James L. Cross; Ruben Mora, MD; David van Dijk, PhD, MSc; Jennifer M. Kwan, MD/PhD Inductive Thematic Analysis of Healthcare Qualitative Interviews Using Open-Source Large Language Models: How Does It Compare To Traditional Methods? Walter S. Mathis, MD Natural Language Processing of Radiology Reports for Retrospective Classification of Oligometastatic Non-Small Cell Lung Cancer Nicholas S. Moore, MD; James H. Laird, MD; Nipun Verma, MD, PhD; Thomas Hager, MS; Durga Sritharan, BS; Ryan Maresca, BS; Victor Lee, MD; Saahil Chadha, BA; Henry S. Park, MD, MPH; Sanjay Aneja, MD Clinical and Biomedical Named Entity Recognition Using Generative Pre-Trained Transformer Models Vipina Keloth, PhD A Deep Learning Approach for Automated Extraction and Categorization of Functional Status and New York Heart Association Class for Heart Failure Patients During Outpatient Encounters Philip Adejumo, BS; Lovedeep Singh Dhingra, MBBS; Arya Aminorroaya, MD, MPH; Xinyu Zhao, BS; Rohan Khera, MD, MS A Systematic Evaluation of Large Language Models for Biomedical Natural Language Processing: Benchmarks, Baselines, and Recommendations Qingyu Chen, PhD; Jingcheng Du, PhD; Yan Hu; Vipina Kuttichi Keloth, PhD; Xueqing Peng, PhD; Kalpana Raja, PhD, MRSB, CSci; Qianqian Xie, PhD; Aidan Gilson; Maxwell Singer, MD; Ron A. Adelman, MD, MPH, MBA, FACS; Rui Zhang, PhD; Zhiyong Lu, PhD, FACMI; Hua Xu, PhD, FACMI
- Nancy J. Brown, MD Jean and David W. Wallace Dean of the Yale School of Medicine and C.N.H. Long Professor of Internal Medicine
- Lucila Ohno-Machado, MD, MBA, PhD Waldemar von Zedtwitz Professor of Medicine and Biomedical Informatics and Data Science; Deputy Dean for Biomedical Informatics; Chair, Department of Biomedical Informatics and Data Science
- Hua Xu, PhD Robert T. McCluskey Professor of Biomedical Informatics and Data Science; Vice Chair for Research and Development, Department of Biomedical Informatics and Data Science; Assistant Dean for Biomedical Informatics, Yale School of Medicine
- Rohan Khera, MD, MS Assistant Professor of Medicine (Cardiovascular Medicine) and of Biostatistics (Health Informatics); Clinical Director, Center for Health Informatics and Analytics, YNHH/Yale CORE; Director, Cardiovascular Data Science (CarDS) Lab
- Mark Gerstein, PhD Albert L Williams Professor of Biomedical Informatics and Professor of Molecular Biophysics & Biochemistry, of Computer Science, and of Statistics & Data Science
- Lee Schwamm, MD Associate Dean, Digital Strategy & Transformation, Office of the Dean, YSM; Professor in Biomedical Informatics & Data Sciences, YSM; Professor of Neurology, YSM; Senior Vice President & Chief Digital Health Officer, YNHHS
- Allen Hsiao, MD, FAAP, FAMIA Professor of Pediatrics (Emergency Medicine) and of Emergency Medicine; Chief Health Information Officer, Yale School of Medicine & Yale New Haven Health, Yale School of Medicine; Vice Chair of Clinical Systems, Biomedical Informatics & Data Science
- Daniella Meeker, PhD Associate Professor of Biomedical Informatics & Data Science; Chief Research Information Officer, Yale School of Medicine and Yale New Haven Health System
- Evangelos K. Oikonomou, MD, DPhil Clinical Fellow; Cardiovascular Medicine, Yale School of Medicine
- Fuyao Chen MD-PhD Student, Biomedical Engineering
- Dana Peters, PhD Professor of Radiology and Biomedical Imaging; Director of Cardiac MRI, Magnetic Resonance Imaging
- Frances J. Griffith Postdoctoral Fellow
- Colleen Chan, PhD
- Sunny Chung Clinical Fellow
- Huan He, PhD Research Scientist in Biomedical Informatics and Data Science
- Qianqian Xie, PhD Postdoctoral Associate in Biomedical Informatics and Data Science
- Mauro Giuffrè, MD Postdoctoral Associate
- William J. Zhang
- Phyllis Thangaraj, MD, PhD Clinical Fellow
- Farah Kidwai-Khan Data Scientist/Associate Research Scientist, Internal Medicine (General Medicine)
- Sarah N. Dudgeon, MPH
- Dhananjay Bhaskar, PhD Postdoctoral Associate
Host Organization
- Biomedical Informatics & Data Science
Related Links
- Attendee Registration
- Virtual attendance
Thank you for visiting nature.com. You are using a browser version with limited support for CSS. To obtain the best experience, we recommend you use a more up to date browser (or turn off compatibility mode in Internet Explorer). In the meantime, to ensure continued support, we are displaying the site without styles and JavaScript.
- View all journals
- Explore content
- About the journal
- Publish with us
- Sign up for alerts
- Brief Communication
- Open access
- Published: 10 August 2024
Disparities in clinical studies of AI enabled applications from a global perspective
- Rui Yang ORCID: orcid.org/0009-0006-0597-7197 1 ,
- Sabarinath Vinod Nair ORCID: orcid.org/0000-0002-7546-2897 1 ,
- Yuhe Ke ORCID: orcid.org/0000-0001-7193-4749 1 , 2 ,
- Danny D’Agostino 1 ,
- Mingxuan Liu ORCID: orcid.org/0000-0002-4274-9613 1 ,
- Yilin Ning ORCID: orcid.org/0000-0002-6758-4472 1 &
- Nan Liu ORCID: orcid.org/0000-0003-3610-4883 1 , 3 , 4
npj Digital Medicine volume 7 , Article number: 209 ( 2024 ) Cite this article
1133 Accesses
14 Altmetric
Metrics details
- Health care
- Medical research
Artificial intelligence (AI) has been extensively researched in medicine, but its practical application remains limited. Meanwhile, there are various disparities in existing AI-enabled clinical studies, which pose a challenge to global health equity. In this study, we conducted an in-depth analysis of the geo-economic distribution of 159 AI-enabled clinical studies, as well as the gender disparities among these studies. We aim to reveal these disparities from a global literature perspective, thus highlighting the need for equitable access to medical AI technologies.
Similar content being viewed by others
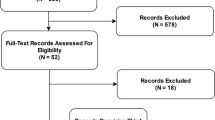
Reporting guidelines in medical artificial intelligence: a systematic review and meta-analysis
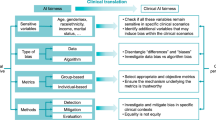
A translational perspective towards clinical AI fairness
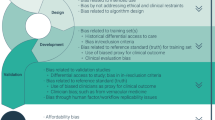
Considerations for addressing bias in artificial intelligence for health equity
In the rapidly developing field of healthcare, artificial intelligence (AI) has emerged as a pivotal force driving innovation in clinical research and improving the efficiency of clinical studies 1 , 2 , 3 , 4 , 5 , 6 . However, despite rapid technological advancements, its practical application in clinical settings remains limited. Concurrently, there are complex disparities in current AI-enabled clinical studies. These disparities, which include data and algorithms, participants and subjects, and access to cutting-edge technologies 7 , 8 , 9 , 10 , challenge the equitable implementation of AI solutions 11 , 12 .
We identified 159 clinical studies of AI-enabled applications from Embase, MEDLINE, and CINAHL through a systematic review. Among these studies, 109 were conducted in hospital settings, while 50 took place in non-hospital environments. Notably, 51.6% (82/159) of the studies utilized AI for treatment and management, and 40.9% (65/159) focused on AI-assisted diagnosis, with a significant portion related to gastroenterology (see Supplementary Table 1 ). Moreover, 5.0% (8/159) of the studies applied AI for prognosis, and 2.5% (4/159) studies explored its use in patient education. In this study, we primarily analyzed the geo-economic distributions as well as the gender disparities among the study subjects.
As depicted in Fig. 1a , the majority of studies were conducted in North America, Europe, and East Asia, with the United States (44 studies) and China (43 studies) leading. A significant portion (74.0%) of the clinical studies were implemented in high-income countries, 23.7% in upper-middle-income countries, 1.7% in lower-middle-income countries, and only one clinical study was conducted in low-income countries (i.e., Mozambique), as shown in Fig. 1b . Meanwhile, we analyzed the geo-economic distribution of 318 first and last authors, which revealed a similar distribution pattern. Additionally, we observed that funding status is correlated with country income level; the funding rate in high-income countries was as high as 83.8%, while it was only 68.3% in upper-middle-income countries, as shown in Fig. 1c .
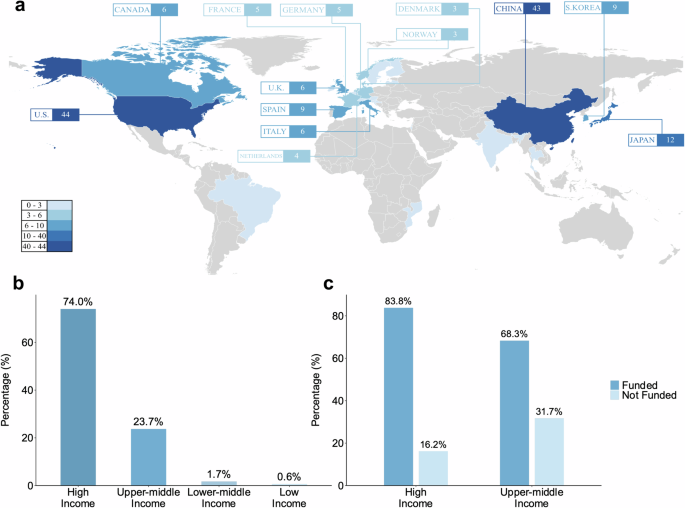
a Geographical distribution of studies. In the figure, we only marked countries that conducted more than two studies. b Income level classification of countries conducting studies. c Funding status of studies by country income level classification (Only studies conducted in a single country were counted. The fund status of studies in lower-middle-income and lower-income countries is not shown in the figure because the number is only 3, and the bars generated are hardly visible). The map was generated using ArcGIS software.
We further explored the gender disparities among the subjects in these AI-enabled clinical studies. After excluding studies of gender-specific diseases, 146 studies reported gender information, of which only 3 (2.1%) reported an equal number of male and female subjects. We then classified the gender ratio of the remaining studies into three categories: “low disparity (0.7–1)”, “moderate disparity (0.3–0.7]”, and “high disparity (0–0.3]”. As illustrated in Fig. 2 , 10.3% (15/146) of the clinical studies exhibited high gender disparity, while another 36.3% (53/146) demonstrated moderate gender disparity. Among the 15 clinical studies with high gender disparity, males predominated in 8 studies, 25% (2/8) of which were related to obstructive sleep apnea (OSA) due to the higher prevalence of OSA in males 13 . Females were the majority in 7 studies, of which 28.6% (2/7) were linked to obesity 14 . Additionally, one study had a higher proportion of female subjects due to the inclusion of a subset of patients undergoing gynecological surgeries. More information is detailed in Supplementary Table 2 .
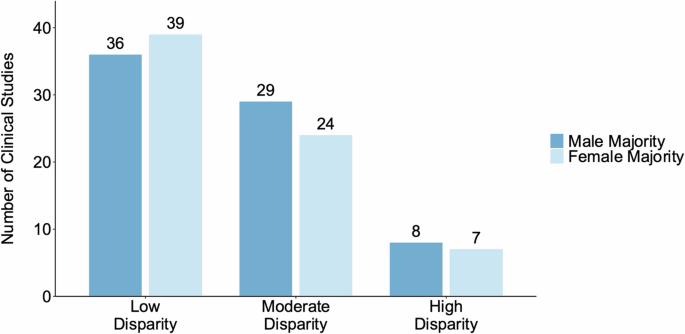
We categorized the studies into “male majority” and “female majority” groups, depending on whether there were more male or female subjects. Within each group, we calculated the “gender ratio” by dividing the number of subjects from the minority gender by the number from the majority gender. The gender ratio was then classified into three levels based on the gender ratio: “low disparity (0.7–1)”, “moderate disparity (0.3–0.7]”, and “high disparity (0–0.3]”.
In summary, our study examined 159 AI-enabled clinical studies (of which only 6.3% (10/159) were international multicenter studies), and the results indicate significant disparities in both the geo-economic distribution of these clinical studies and the gender of the study subjects.
The leading countries and authors are primarily from high-income and upper-middle-income countries. The limited representation of lower-middle-income and low-income countries is concerning since these countries may have different disease profiles and are, therefore, unable to benefit from existing research 15 . Moreover, the presence of gender disparities in clinical studies could lead to potential health inequalities.
Considering these disparities, it is crucial to involve a diverse group of researchers and gender-balanced subjects in the design of AI-enabled clinical studies. Additionally, international multi-center clinical studies should be encouraged, particularly including sites from lower-middle-income and low-income countries. This will enable AI tools to be effectively applied and evaluated in diverse healthcare systems around the world. The pursuit of health equity will lay a solid foundation for the sustainable development and application of AI in healthcare.
Search strategy
We conducted a systematic literature search using Embase, MEDLINE, and CINAHL to identify AI-enabled clinical studies published prior to January 3, 2024. The search strategy we used included keywords and Medical Subject Headings (MeSH) terms related to “Artificial Intelligence” and “Clinical Study”. Additionally, we manually reviewed the references of the included studies to identify more relevant studies (Detailed search strategy can be found in Supplementary Material Search Strategy and PRISMA flow diagram can be found in Supplementary Fig. 1 ).
Inclusion and exclusion criteria
Each article was independently screened by two researchers (R.Y. and S.V.N.) to determine eligibility. Disagreements were resolved through consultation with a third researcher (Y.K.). Studies were included if they: (1) incorporated a significant AI component, defined as a nonlinear computational model (including, but not limited to, support vector machine, decision trees, neural networks, etc.) 6 ; (2) were applied in clinical settings, influencing the patient’s health management; and (3) were published as a full-text article in a peer-reviewed, English-language journal. Additionally, we excluded studies that used linear models (such as linear regression and logistic regression), conducted secondary analyses or did not integrate AI algorithms into clinical practice. For each included study, we extracted information including gender information of the subjects, the country of clinical study implementation, the income level of the country (based on World Bank’s classifications 16 ), the funding, and so on. All extracted information can be found in Supplementary Table 1 .
Data availability
The data used in the manuscript can be found in Supplementary Material .
Rajpurkar, P., Chen, E., Banerjee, O. & Topol, E. J. AI in health and medicine. Nat. Med. 28 , 31–38 (2022).
Article PubMed CAS Google Scholar
Hinton, G. Deep learning—a technology with the potential to transform health care. JAMA 320 , 1101–1102 (2018).
Article PubMed Google Scholar
Woo, M. An AI boost for clinical trials. Nature 573 , S100–S102 (2019).
Yang, R. et al. Large language models in health care: development, applications, and challenges. Health Care Sci. 2 , 255–263 (2023).
Article PubMed PubMed Central Google Scholar
Ke, Y. H. et al. Enhancing diagnostic accuracy through multi-agent conversations: using large language models to mitigate cognitive bias. Preprint at arXiv:1504.14589 (2024).
Han, R. et al. Randomised controlled trials evaluating artificial intelligence in clinical practice: a scoping review. Lancet Digit. Health 6 , e367–e373 (2024).
Article PubMed PubMed Central CAS Google Scholar
Chen, I. Y., Joshi, S. & Ghassemi, M. Treating health disparities with artificial intelligence. Nat. Med. 26 , 16–17 (2020).
Nordling, L. A fairer way forward for AI in health care. Nature 573 , S103–S105 (2019).
Celi, L. A. et al. Sources of bias in artificial intelligence that perpetuate healthcare disparities—a global review. PLoS Digit. Health 1 , e0000022 (2022).
Liu, M. et al. A translational perspective towards clinical AI fairness. npj Digit. Med. 6 , 1–6 (2023).
Article CAS Google Scholar
Serra-Burriel, M., Locher, L. & Vokinger, K. N. Development pipeline and geographic representation of trials for artificial intelligence/machine learning-enabled medical devices (2010 to 2023). NEJM AI https://doi.org/10.1056/aipc2300038 (2023).
Alberto, I. R. I. et al. A scientometric analysis of fairness in health AI literature. PLoS Glob. Public Health 4 , e0002513 (2024).
O’Connor, C., Thornley, K. S. & Hanly, P. J. Gender differences in the polysomnographic features of obstructive sleep apnea. Am. J. Respir. Crit. Care Med. 161 , 1465–1472 (2000).
Cooper, A. J., Gupta, S. R., Moustafa, A. F. & Chao, A. M. Sex/gender differences in obesity prevalence, comorbidities, and treatment. Curr. Obes. Rep. 10 , 458–466 (2021).
Black, E. & Richmond, R. Improving early detection of breast cancer in sub-Saharan Africa: why mammography may not be the way forward. Glob. Health 15 , 1–11 (2019).
Article Google Scholar
Hamadeh, N. et al. New World Bank Country Classifications by Income Level: 2022–2023 (World Bank Blogs, 2022).
Download references
Acknowledgements
This work was supported by the Duke-NUS Signature Research Program funded by the Ministry of Health, Singapore. Any opinions, findings and conclusions or recommendations expressed in this material are those of the author(s) and do not reflect the views of the Ministry of Health. Additionally, we appreciate Jonathan Liew’s contribution to information extraction during the revision stage.
Author information
Authors and affiliations.
Centre for Quantitative Medicine, Duke-NUS Medical School, Singapore, Singapore
Rui Yang, Sabarinath Vinod Nair, Yuhe Ke, Danny D’Agostino, Mingxuan Liu, Yilin Ning & Nan Liu
Department of Anesthesiology, Singapore General Hospital, Singapore, Singapore
Programme in Health Services and Systems Research, Duke-NUS Medical School, Singapore, Singapore
NUS Artificial Intelligence Institute, National University of Singapore, Singapore, Singapore
You can also search for this author in PubMed Google Scholar
Contributions
N.L. conceived the study. R.Y., S.V.N., M.L., Y.N., and N.L. designed the study. R.Y., S.V.N., Y.K., and D.D. collected data. R.Y. conducted data analyses. R.Y. and S.V.N. drafted the manuscript, with further development by N.L. N.L. supervised the study. All authors contributed to the revision of the manuscript and approval of the final version.
Corresponding author
Correspondence to Nan Liu .
Ethics declarations
Competing interests.
N.L. is an Editorial Board Member for npj Digital Medicine. He played no role in the peer review of this manuscript. The remaining authors declare that there are no other financial or non-financial competing interests.
Additional information
Publisher’s note Springer Nature remains neutral with regard to jurisdictional claims in published maps and institutional affiliations.
Supplementary information
Supplementary materials, rights and permissions.
Open Access This article is licensed under a Creative Commons Attribution 4.0 International License, which permits use, sharing, adaptation, distribution and reproduction in any medium or format, as long as you give appropriate credit to the original author(s) and the source, provide a link to the Creative Commons licence, and indicate if changes were made. The images or other third party material in this article are included in the article’s Creative Commons licence, unless indicated otherwise in a credit line to the material. If material is not included in the article’s Creative Commons licence and your intended use is not permitted by statutory regulation or exceeds the permitted use, you will need to obtain permission directly from the copyright holder. To view a copy of this licence, visit http://creativecommons.org/licenses/by/4.0/ .
Reprints and permissions
About this article
Cite this article.
Yang, R., Nair, S.V., Ke, Y. et al. Disparities in clinical studies of AI enabled applications from a global perspective. npj Digit. Med. 7 , 209 (2024). https://doi.org/10.1038/s41746-024-01212-7
Download citation
Received : 03 March 2024
Accepted : 01 August 2024
Published : 10 August 2024
DOI : https://doi.org/10.1038/s41746-024-01212-7
Share this article
Anyone you share the following link with will be able to read this content:
Sorry, a shareable link is not currently available for this article.
Provided by the Springer Nature SharedIt content-sharing initiative
Quick links
- Explore articles by subject
- Guide to authors
- Editorial policies
Sign up for the Nature Briefing newsletter — what matters in science, free to your inbox daily.

Rochester News
- Science & Technology
- Society & Culture
- Campus Life
- University News
From the Magazine
Doctors, patients, algorithms, and avatars
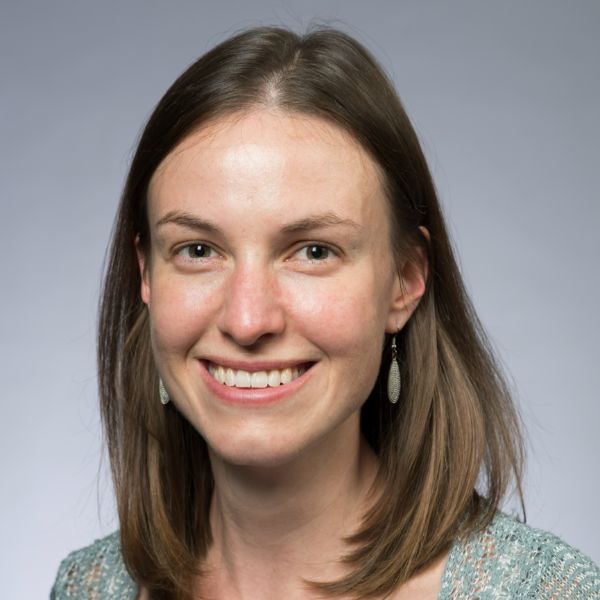
- Facebook Share on Facebook
- X/Twitter Share on Twitter
- LinkedIn Share on LinkedIn
Clinicians, computer scientists, and ethicists are working across the University of Rochester to incorporate reliable and ethical AI into medical diagnosis and treatment.
In 2012, professor of psychiatry Caroline Easton had a eureka moment watching her son play video games: what if she could blend video-game technology with her research on the intertwined issues of addiction and domestic violence?
“Younger clients have grown up with games and having technology at their fingertips,” she says. “We need to have venues of therapy that are relatable to them and that standardize behavioral therapy in a way that is like a medication.”
With that insight in mind, Easton set out to develop a platform that would use an avatar coach to guide patients through cognitive behavioral therapy and help them practice coping skills.
Initially, Easton and her team manually created and animated rudimentary avatars. But increasingly sophisticated artificial intelligence (AI) technology has allowed her to fine-tune the tool, enabling users to customize their avatar coaches to respond to their particular needs.
Funded by the National Institutes of Health, the app is in the pilot phase, with randomized control trials set to begin by the end of the summer. Easton says AI tools like this one promise to transform addiction treatment by not only supporting patients between therapy sessions but also enabling clinicians to spend time and energy with their patients in ways that are even more meaningful.
“We can now use AI to collect data and deploy the most relevant coping skills based on what the client is feeling in the moment,” Easton explains. “And if we can also decrease things like compassion fatigue, vicarious trauma, and work burnout—and allow the clinician more time to focus on the therapeutic alliance—I feel like we are heading in the right direction.”
Today, Easton is the Medical Center’s academic chief of addictions psychiatry and director of digital therapeutics for the Department of Psychiatry . Her use of AI in therapies illustrates one facet of AI’s transformative power in medicine and health care.
At the same time, AI also raises questions among clinicians and researchers, including Easton: How can we ensure everyone benefits from AI advancements fairly and responsibly? As AI takes on a larger role in health care, how do we guarantee it serves everyone’s best interests? How can advanced technology be integrated into medicine without losing the human connection?
The long road to generative AI
Artificial intelligence is not new. It was described in theory as early as the 1950s by philosophers and mathematicians such as Alan Turing, who posited that machines might be able to learn in ways similar to humans. But it took decades before computing power was sufficient to make AI more than just a concept.
In the late 1990s, IBM demonstrated a major breakthrough when its Deep Blue computer defeated world chess champion Garry Kasparov. Then in 2011 a still more powerful IBM computer system called Watson competed on the TV game show Jeopardy! and won over two of the game’s greatest champions, Brad Rutter and Ken Jennings.
When IBM tried to leverage Watson in the health care sector, however, the effort was unsuccessful. Watson was initially trained on highly structured datasets, such as dictionaries and encyclopedias. That training was poorly suited to working with health care data. Inherently unstructured, health care data encompasses a wide range of complexities, including shorthand and misspellings in doctors’ notes; variability in the image quality of medical scans; the presence of anomalies such as rare diseases and complex genomic information; and fragmented datasets spread across different systems. Computers required sophisticated techniques to effectively process and integrate diverse types of data.
In 2023, a paradigm shift occurred with the emergence of generative AI, led by OpenAI’s ChatGPT.
“When the world got introduced to generative AI, in health care—and pretty much every aspect of our daily lives—the potential of AI became very powerful,” says Michael Hasselberg ’13N (PhD) an associate professor of psychiatry, clinical nursing, and data science, and the University of Rochester ’s inaugural chief digital health officer.
While classical machine learning enables computers to analyze data and use it to make predictions, generative AI enables machines to create new content based on learned patterns from large datasets. It does this using complex neural networks designed to mimic the human brain’s ability to recognize patterns and learn from them.
“Generative AI comes trained on more than a trillion parameters—essentially the entire internet and all of its structured and unstructured data,” Hasselberg explains.
AI’s advantages
That powerful foundation allows generative AI to analyze patterns in medical images with remarkable accuracy and speed.
“AI helps us identify urgent issues quickly, which is crucial for conditions like a pulmonary embolism or a brain bleed,” says Jennifer Harvey , the Dr. Stanley M. Rogoff and Dr. Raymond Gramiak Professor in Radiology and chair of the Department of Imaging Sciences .
That means AI tools can act like a second set of eyes for radiologists. Say a patient undergoes a CT scan to check for possible pneumonia. As the scanner captures detailed images of the patient’s chest, an AI algorithm analyzes each of the images, and, within moments, flags a potential pulmonary embolism even if the scan was initially done for a different reason. Potentially urgent problems get prioritized, leading to more efficient and accurate diagnostics.
But radiologists must still carefully examine every patient scan. According to Harvey, AI does not threaten to replace radiologists because most algorithms are built to identify a single finding. For instance, the tool might only be looking for a pulmonary embolism in a set of images. Rare findings continue to be difficult for AI tools to detect.
“Radiologists are still much better at synthesizing the findings in a way that AI tools cannot,” Harvey says. “For example, a chest CT may have one or two findings flagged by AI, but the radiologist must put all of the findings together to generate likely diagnoses.”
Still, she adds, predictive AI tools can offer “critical insights”—and not only in analyzing scans. They can also summarize report results and automate other clinical tasks.
Hasselberg highlights the “army of nurses” at the Medical Center whose jobs previously involved manually extracting data points from patient charts for submission to national registries.
“Tasks like these are well below the scope and practice of a nurse,” he says. “You’re still going to have a human in the loop that will look at the generative AI’s output and see if everything looks right. But the machines take the administrative burden off the clinicians, giving them more time to spend with patients actually doing clinical care.”
Can doctors and patients trust AI?
To rely on algorithms for disease detection and treatment, however, clinicians need to have high confidence in their accuracy. Says Hasselberg: “There is some risk with generative AI because it does generate new content. It can get it wrong.”
Hasselberg’s role includes serving as codirector of the UR Health Lab, using technology such as machine learning, virtual reality, and 3D imaging to enhance patient care, while also tackling ethical challenges. To this end, he has partnered with stakeholders across the University, including not only clinicians like Harvey and Easton but also Chris Kanan , an associate professor of computer science who helped develop the first FDA–cleared pathology tools, and Jonathan Herington , an assistant professor of philosophy and of bioethics who is a nationally recognized expert on the ethical issues surrounding AI.
An ethicist whose research once focused on political philosophy, Herington began writing about AI systems in 2017, prompted by articles in the popular press on the use of machine learning algorithms to predict criminal recidivism. He began to look deeply into how algorithms can perpetuate social and cultural biases.
From 2021 to 2023 he served on the AI Task Force for the Society for Nuclear Medicine and Molecular Imaging. During that time, the task force published two influential papers in the Journal of Nuclear Medicine addressing ethical issues surrounding AI.
Herington highlights one case demonstrating a particularly pressing ethical concern: An insurance algorithm was designed to identify patients who have a high probability of requiring frequent care, with the goal of enrolling them in prevention programs. As a metric for identifying high-need patients, the insurer used cost of care—that is, “How much care did a person cost us last year?” Due to disparities in access, Black patients who were as sick as white patients were rated with lower risk because they had historically accessed care less frequently.
“All of this historic bias in the health care system got baked into this dataset,” Herington says.
One way to remediate bias is to be more deliberate about the data used to train the system. But a larger issue is at play as well. Precisely because the tools can get it wrong, “it is more important than ever—especially as we start moving toward more generative AI tools—to always have a human in the loop,” Herington says.
Perfecting AI tools
Another way to keep humans in the loop is to regulate AI tools as medical devices, which requires FDA compliance certification. Herington favors that approach. “Right now, it’s like the Wild West,” he says. “Models can be implemented without establishing that they work.”
Many companies balk at the rigorous testing—and the delay that ensues—in moving a product to market. However, FDA certification offers benefits even beyond ensuring a product is safe. The distinction helps increase the trust hospitals and clinics have in a product, Kanan says. If a product does not go through the FDA certification process, it must be marketed in the US as “research use only.” FDA distinction also ensures regulatory compliance to avoid legal issues and is more likely to be covered by insurance.
The FDA process proved valuable when Kanan was working with the company Paige.AI to develop Paige Prostate—the first FDA–cleared, AI-assisted pathology tool.
In his lab, Kanan focuses on fundamental research in deep learning as it applies to AI.
His work with Paige began in 2018, with the goal of developing an AI tool that could assist pathologists in diagnosing cancer with unprecedented speed and accuracy. To create such a tool, however, required more than developing sophisticated algorithms. It also meant integrating AI into workflows and ensuring its reliability across various settings.
Kanan and his team had to demonstrate the AI could perform consistently well, regardless of differences in medical data and environments of individual hospitals. For instance, an algorithm trained on data from a specific set of hospitals might be more sensitive to the unique conditions and nuances of those environments. When the same algorithm is then applied to a different patient population or hospital system, it can struggle to perform effectively because of variability in data and clinical practices. Kanan’s team had to ensure Paige Prostate wasn’t overly sensitive to factors such as the type of microscope used or the presence of watermarks on medical scans. They also needed to address potential biases related to patient demographics, such as gender or race, by ensuring Paige Prostate performed equally well across hospitals serving different populations.
Then there was the challenge of making sure the AI could detect a variety of anomalies.
“The majority of the cases are the ones that are obvious,” Kanan says. “It’s the rarer stuff that people don’t have as much familiarity with that are challenging for an AI system to spot.”
The key, Kanan says, is to train AI systems on extensive and diverse datasets. In Paige’s case, the dataset included millions of images and datapoints from numerous sources, ensuring the tool could generalize well across different conditions.
It’s also important that system training be continuous. This is where academic medical centers can play a large role. By implementing AI programs and contributing valuable data, hospitals can work with companies like Paige to refine and enhance AI algorithms. This collaboration ensures that AI tools continuously “learn” and improve.
Locations such as Rochester—which cover broad, diverse patient groups, and, in Rochester’s case, also span dense urban areas as well as small towns and rural communities—are well positioned for the task of testing and refining AI tools.
“Having that heterogeneity of patients is a game changer,” Hasselberg says. “It’s incredibly important to make sure that AI tools are developed and deployed in an ethical way that accounts for a very diverse group of patient populations.”
What no machine can do
Despite the technological advancements in AI, the role of human expertise and empathy remains irreplaceable. Generative AI, even at its most impressive, “doesn’t correlate with the ability to plan or have beliefs, attitudes, or genuine emotional reactions,” Herington says.
Says Hasselberg, “There’s an art to clinical care and the way we make decisions. It’s not all based on data points or an algorithm; it’s based on intuition and experience. There are things we do as clinicians that a machine can’t do.”
That’s precisely why Easton designed her app to be a complement to—not a replacement for—clinician-centered therapy.
The app’s program spans 12 weeks—a length of time consistent with a typical course of cognitive behavioral therapy. Patients start each session by rating their cravings, substance use, and mood in the current moment. The avatar then guides them through real-time coping exercises and positive reinforcements, such as meditations or distraction techniques like taking a walk. Patients provide feedback by rating the effectiveness of the exercises. The tool tracks progress and behaviors with charts and graphs, identifying patterns in each patient’s stressors over time.
Easton envisions that the program could be integrated into a wearable device to take in biomarker data and proactively deploy on-demand coping skills to help prevent psychiatric crises.
But this would be a tool that patients would use in between visits with a human clinician, who could synthesize the information and integrate it into a detailed care plan.
It is never the goal, Easton says, for technology to replace the human therapist.
“We should never be taking the human out of the equation. We can coexist and integrate with technology, but I don’t envision a world where technology would ever take over for people.”
This story appears in the summer 2024 issue of Rochester Review , the magazine of the University of Rochester.
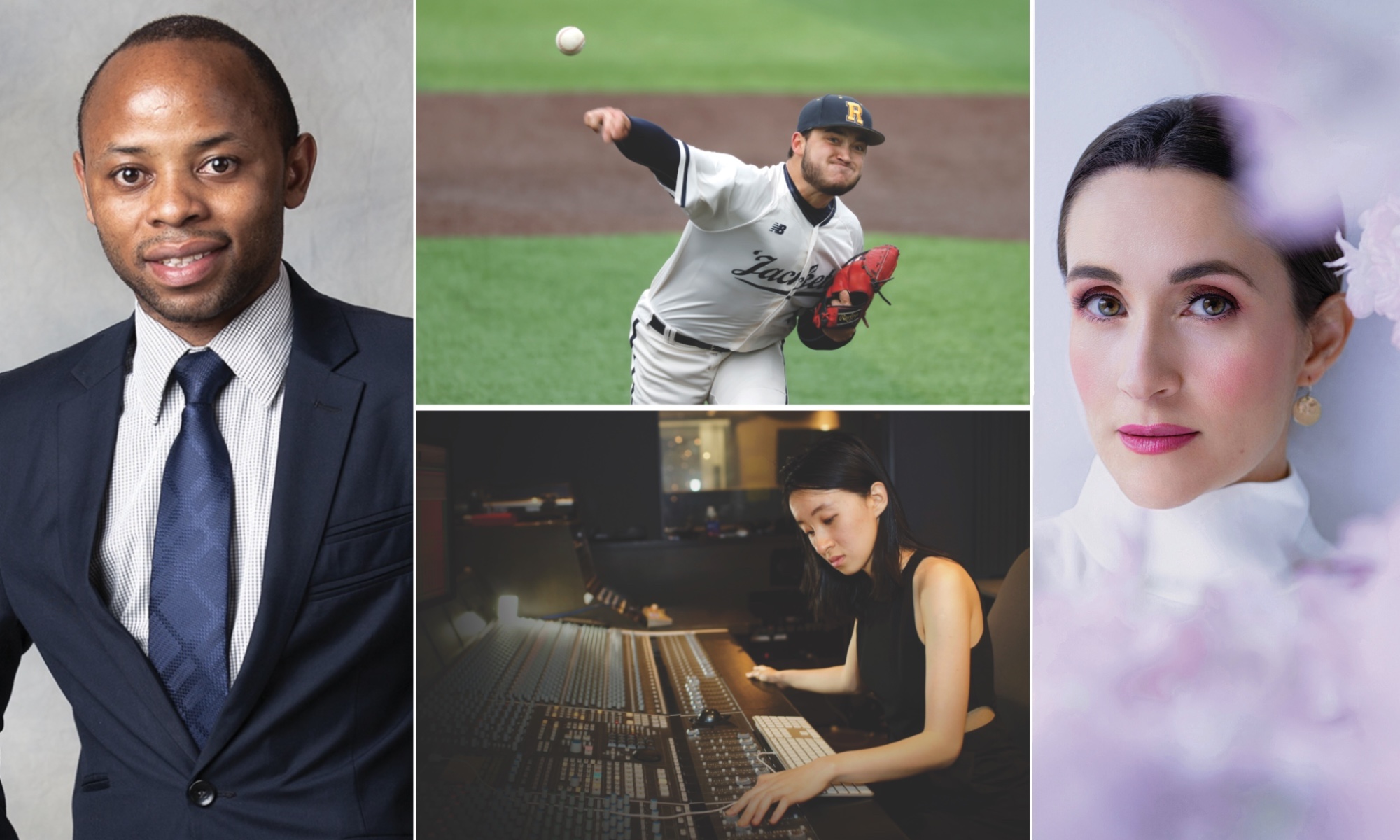
More From Forbes
The promise and challenges of ai in healthcare.
- Share to Facebook
- Share to Twitter
- Share to Linkedin
Dr. Corey Scurlock MD, MBA is the CEO & founder of Equum Medical.
Seemingly overnight, artificial intelligence has caught fire in healthcare, with feverish interest from boards, the C-suite, medical staff, information technology and revenue cycle management — you name it, and there’s an algorithm in the works. Fueled by the flashy arrival of OpenAI’s ChatGPT and its newest large language model, GPT-4, the race is on among IT vendors and care providers to use AI to improve everything from triage to patient communications to treatment to discharge. The dream is that AI can reduce costs, make work so productive that fewer staff are needed, triage patients faster and ensure they are cared for in the right setting.
At least for now, much of the promise of AI in healthcare is a dream deferred. AI systems require stupendous amounts of data and must be trained to recognize patterns in medical data, understand the relationships between different diagnoses and treatments, and provide accurate recommendations that are tailored to each patient. This learning, either guided by a clinician (machine learning) or working on its own (deep learning), takes time.
Integration challenges loom large , including "maximizing AI’s capabilities in EHR systems and providing opportunities to grow their potential and improve overall global healthcare."
As a physician entrepreneur, I am actively exploring ways to incorporate AI into my company’s telehealth-enabled clinical services. AI-powered algorithms can analyze vast amounts of patient data, enabling physicians to make more informed decisions in real time. Our goal is to empower physicians with the tools they need to deliver efficient, personalized care while optimizing patient flow and outcomes. By embracing AI, organizations can drive positive change in healthcare and shape a future where technology and human expertise work hand in hand to revolutionize the patient experience.
Early intervention is crucial in healthcare, as it can significantly impact patient outcomes. AI algorithms can continuously monitor patient data, including vital signs, lab results and patient-reported symptoms, to identify potential warning signs. By leveraging predictive analytics and machine learning, I believe AI could help physicians identify high-risk patients who may require immediate attention, enabling timely intervention and potentially preventing complications or hospitalizations.
I have written previously about the promise of telehealth for easing staffing shortages and overcoming “hot spots” in the patient’s journey through the health system—care transitions that cost every stakeholder through delayed care and worse outcomes. Already, some experts say AI can "analyze patient flow, wait times and time spent doing certain tasks" to ensure staffing levels are appropriate.
Telehealth physicians can access electronic records and clinical decision support systems and have the technological chops to work with AI systems as they mature. As healthcare professionals, we face mounting administrative burdens that often impede our ability to dedicate sufficient time to direct patient care. AI tools such as natural language processing and intelligent automation could assist with tasks such as documentation, coding and scheduling, freeing up valuable time for physicians to focus on what they do best: providing personalized care to patients. This could not only enhance physician productivity but also contributes to reducing burnout and improving satisfaction for patients and caregivers.
Even more promise may be found in AI’s analytical prowess. An article in British Dental Journal explains that AI can "analyze large amounts of patient data, such as medical records, imaging studies and laboratory results, to support clinical decision making and improve patient outcomes." Clinical decision support in current electronic records systems has not always been warmly embraced by care providers.
Recently Microsoft, which invested billions in OpenAI, said it plans to bring GPT-4 to Epic's electronic medical record . The initial use cases will involve patient communication and data visualization.
In medical imaging, one paper explained that "in many applications, the performance of machine learning-based automatic detection and diagnosis systems has shown to be comparable to that of a well-trained and experienced radiologist." Medical diagnostic facilities could see fewer errors and misdiagnoses , reduced operational costs and faster lab results.
There remain significant concerns about AI’s projected impact on organizations and people. Just as driver-assistance tools don’t mean people should take their hands off the steering wheel and take a nap, algorithms should be used to augment clinicians’ decision making rather than replace it entirely. Doctors and their patients should always have the final say in care.
At the same time, data being fed into AI programs can retain or further inherent biases from past treatment decisions. Many systems may not have enough “Ns” on particular minority groups to take into account these populations’ unique healthcare needs.
There are also governance issues. Most health systems have formal IT structures to reduce duplicative systems, standardize care and maintain patient privacy. Anyone who has followed AI knows it makes mistakes and can even make stuff up. We need to be able to answer questions such as: Will AI make IT more or less governable? Who decides where and how it is used and for what purpose? Will we choose the best technology and just roll it out, or should we wait for evidence-based practices in the literature?
Properly regulated, I believe AI has nearly limitless potential for helping solve some of the biggest challenges facing healthcare. New efficiencies should lead to greater productivity. For example, if an AI algorithm could analyze a patient's vital signs and medical history to predict the likelihood of a cardiac event, healthcare professionals, whether onsite or remote, could instantly get that patient into critical care.
I believe AI in healthcare will be transformational, improving patient care from triage to treatment to discharge to final billing. We must also recognize that these advanced tools come with new challenges. When OpenAI’s chief executive asks Congress to regulate AI, it’s probably time to install and maintain the guardrails needed to keep us, humans, in charge.
Forbes Business Council is the foremost growth and networking organization for business owners and leaders. Do I qualify?
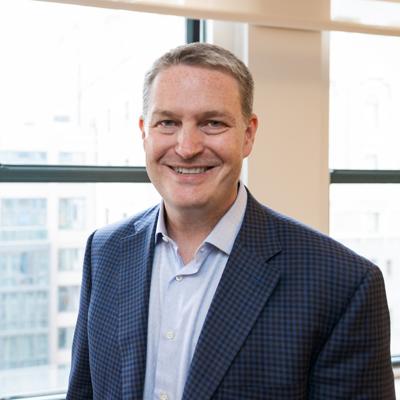
- Editorial Standards
- Reprints & Permissions
- Health Tech
- Health Insurance
- Medical Devices
- Gene Therapy
- Neuroscience
- H5N1 Bird Flu
- Health Disparities
- Infectious Disease
- Mental Health
- Cardiovascular Disease
- Chronic Disease
- Alzheimer's
- Coercive Care
- The Obesity Revolution
- The War on Recovery
- Adam Feuerstein
- Matthew Herper
- Jennifer Adaeze Okwerekwu
- Ed Silverman
- CRISPR Tracker
- Breakthrough Device Tracker
- Generative AI Tracker
- Obesity Drug Tracker
- 2024 STAT Summit
- All Summits
- STATUS List
- STAT Madness
- STAT Brand Studio
Don't miss out
Subscribe to STAT+ today, for the best life sciences journalism in the industry
Can AI help ease medicine’s empathy problem?
Doctors often fail to express empathy. artificial intelligence — done right — might be able to help them.
- Manage alerts for this article
- Email this article
- Share this article

By Evan Selinger and Thomas Carroll
Aug. 15, 2024
Rochester Institute of Technology and University of Rochester Medical Center
Modern medicine has an empathy problem. Artificial intelligence — done right — might be able to help ease it.
Despite the proliferation of communication training programs over the past decade or two, doctors often fail to express empathy, especially in stressful moments when patients and their families are struggling to hear bad news and make difficult decisions. Since empathy has been shown to enhance what patients understand and how much they trust their medical team, falling short compromises the quality of patient care.
advertisement
Can AI help? That might sound like an ironic question, because doctors who struggle to express empathy can come across as robotic. Yet researchers and health care professionals are increasingly asking it, and not just because we’re living through an AI hype cycle .
One reason for the growing interest in AI to help solve medicine’s empathy problem is that this aspect of medical care has proven particularly hard to improve. This isn’t surprising, given that physicians face ever-increasing pressures to quickly see large numbers of patients while finding themselves drowning in paperwork and a myriad of administrative duties. These taxing conditions lead to both a lack of time and, perhaps more importantly, a lack of emotional energy. An American Medical Association report indicated that 48% of doctors experienced burnout last year .
Given the magnitude of the empathy problem and its significant clinical and ethical stakes, various possible uses of AI are being explored. None of them are likely to be silver bullets and, while each is well-intentioned, the entire endeavor is fraught with risks.
One rather extreme option has been suggested by Dr. Arthur Garson Jr., a member of the National Academy of Medicine and a clinical professor of health systems and population health sciences the University of Houston. He urges us to prepare for a time when some human doctors are replaced with AI avatars . Garson thinks it’s possible, even likely, that AI-powered avatars displayed on computer screens could be programmed to look “exactly like a physician” and have “in-depth conversations” with “the patient and family” that are customized to provide “highly appropriate reactions” to a patient’s moods and words.
Whether AI will ever get this advanced raises tricky questions about the ethics of empathy, including the risk of creating negative dehumanizing effects for patients because, for the foreseeable future, computer programs can’t experience empathy . To be sure, not all human doctors who sound empathetic truly feel that way in the moment. Nevertheless, while doctors can’t always control their own feelings, they can recognize and respond appropriately to patients’ emotions, even in the midst of trying circumstances.
Simulated AI “doctors,” no matter how apparently smart, cannot truly care about patients unless they somehow become capable of having the human experience of empathy. Until that day comes — and it may never arise — bot-generated phrases like “I’m sorry to inform you” seem to cheapen the very idea of empathy.
A more moderate vision revolves around various applications of generative AI to support doctors’ communication with patients in real time. Anecdotal evidence suggests this use of this technology is promising, like Dr. Joshua Tamayo-Sarver’s moving account of how ChatGPT saved the day in a California emergency department when he struggled to find the right words to connect with a patient’s distraught family. Preliminary academic research, like a much-discussed article in JAMA Internal Medicine, also suggests generative AI programs based on large language models can effectively simulate empathetic discourse.
Another recent study , however, suggests that while the content of an empathic message matters, so does the messenger’s identity. People rate AI-generated empathic statements as better on average than human-generated ones if they don’t know who or what wrote them. But the machine’s advantage disappears once the recipient learns that the words had been generated by a bot.
In a forthcoming book, “Move Slow and Upgrade,” one of us (E.S.) proposes the following possibility: integrating a version of generative AI into patient portals to help doctors sound more empathetic. Patients see portals as a lifeline, but doctors spend so much time fielding inbox messages that the correspondence contributes to their burnout . Perhaps a win-win is possible. Doctors might improve patient satisfaction and reduce the number of follow-up questions patients ask by pushing an empathy button that edits their draft messages.
While this application of AI-generated empathy is promising in a number of ways, it also runs many risks even if the obvious challenges are resolved, like the technology consistently performs well, is routinely audited, is configured to be HIPAA compliant, neither doctors nor patients are forced to use it, and doctors use it transparently and responsibly. Many tricky issues would still remain. For example, how can doctors use AI quickly and oversee its outputs without placing too much trust in the technology’s performance? What happens if the technology creates a multiple persona problem, where a doctor sounds like a saint online but is a robot in person? And how can a new form of AI dependence be created to avoid further deterioration of human communication?
Some visions capitalize on AI’s potential to enhance doctors’ communication skills. For example, one of us (T.C.) is involved with the SOPHIE Project , an initiative at the University of Rochester to create an AI avatar trained to portray a patient and provide personalized feedback. It could help doctors improve their ability to appropriately express empathy. Preliminary data are promising, although it is too soon to draw firm conclusions, and further clinical trials are ongoing.
This approach has the advantages of being reproducible, scalable, and relatively inexpensive. It will, however, likely have many of the same limitations as traditional, human-actor-based communication training courses. For example, on the individual level, communication skills tend to degrade over time, requiring repeated training. Another issue is that the doctors who most need communication training may be least likely to participate in it. It is also unrealistic to expect SOPHIE-like training programs to overcome system-level stresses and dysfunction, which are a major contributor to the empathy problem in the first place.
Because technology changes so quickly, now is the time to have thoughtful and inclusive conversations about the possibilities we’ve highlighted here. While the two of us don’t have all the answers, we hope discussions about AI and empathic communication are guided by an appreciation that both the messages and the messengers matter. Focusing too much on what AI can do can lead to overestimating the value of its outputs and undervaluing essential relationships of care — relationships that, at least for the foreseeable future, and perhaps fundamentally, can occur only between human beings. At the same time, prematurely concluding that AI can’t help may unnecessarily contribute to preserving a dysfunctional system that leaves far too many patients seeing doctors as robotic.
Evan Selinger, Ph.D., is a professor of philosophy at Rochester Institute of Technology and the co-author, with Albert Fox Cahn, of the forthcoming book “ Move Slow and Upgrade: The Power of Incremental Innovation” (Cambridge University Press). Thomas Carroll, M.D., Ph.D., is an associate professor of medicine at the University of Rochester Medical Center.
Letter to the editor
Have an opinion on this essay? Submit a letter to the editor .
About the reporting
STAT’s investigation is based on interviews with nearly 100 people around the country, including incarcerated patients and grieving families, prison officials, and legal and medical experts. Reporter Nicholas Florko also filed more than 225 public records requests and combed through thousands of pages of legal filings to tell these stories. His analysis of deaths in custody is based on a special data use agreement between STAT and the Department of Justice.
You can read more about the reporting for this project and the methodology behind our calculations.
The series is the culmination of a reporting fellowship sponsored by the Association of Health Care Journalists and supported by The Commonwealth Fund.
Evan Selinger
Thomas Carroll
More on Long Covid

STAT Plus: As Humira biosimilars take over the market, CVS has created a new ploy: the drug ‘rebate credit’

The smartest thinkers in life sciences on what's happening — and what's to come
Your data will be processed in accordance with our Privacy Policy and Terms of Service . You may opt out of receiving STAT communications at any time.

A new era for drug development and research
Recommended

First Opinion readers on Noah Lyles and Covid-19, the dearth of geriatricians, PBMs, and more

STAT Plus: What the newly released Medicare prices for 10 drugs do and don’t tell us

Gilead must be held accountable for the harm caused by ‘patent hopping’ an HIV treatment

HHS says it is working to stop the climate crisis. But it’s really just climate washing

Ultra-processed foods: the tobacco of the 21st century?
Subscriber picks, stat plus: trump keeps losing his train of thought. cognitive experts have theories about why, stat plus: how unitedhealth turned a questionable artery-screening program into a gold mine, stat plus: how unitedhealth harnesses its physician empire to squeeze profits out of patients, stat plus: three mdma therapy papers retracted over ethics violations, stat plus: meet the billionaire media mogul who's taking on the food industry.

IMAGES
COMMENTS
The Artificial Intelligence in Medicine (AIM) PhD track, newly developed by the Department of Biomedical Informatics (DBMI) at Harvard Medical School, will enable future academic, clinical, industry, and government leaders to rapidly transform patient care, improve health equity and outcomes, and accelerate precision medicine by creating new AI technologies that reason across massive-scale ...
The Department of Biomedical Informatics offers a PhD in Biomedical Informatics in the areas of Artificial Intelligence in Medicine (AIM) and Bioinformatics and Integrative Genomics (BIG).. The AIM PhD track prepares the next generation of leaders at the intersection of artificial intelligence and medicine. The program's mission is to train exceptional computational students, harnessing ...
If you have questions or wish to learn more about the PhD program in Health AI, call us or send a message. 424-315-0804. SEND A MESSAGE. The PhD program in Health Artificial Intelligence at Cedars-Sinai prepares students with rigorous training in AI algorithms and methods to improve patient care.
An academic program designed to accelerate AI solutions into clinic practice. AIM study highlighted by MGB News and several outlets - ScienceMag, Science Daily and ecancer. Researchers at AIM investigated the use of LLMs for patient portal messaging. AIM researchers developed AI that can diagnose sarcopenia in head and neck cancer.
The AI in Medicine PhD Track administered by the Department of Biomedical Informatics (DBMI) at Harvard Medical School is designed to confront these challenges by preparing the next generation of leaders at the intersection of artificial intelligence and medicine. The program's mission is to train exceptional computational students ...
The Stanford Center for Artificial Intelligence in Medicine and Imaging (AIMI) was established in 2018 to responsibly innovate and implement advanced AI methods and applications to enhance health for all. ... Ipek Oguz, PhD. Wednesday, October 16, 2024 | 12:00pm - 1:00pm PDT. Hybrid: In-Person | Virtual. Nov 20. Seminar IBIIS-AIMI Seminar: Hugo ...
Interdisciplinary Studentship in Human-Centred AI. University of Southampton Faculty of Engineering and Physical Sciences. The Web Science Institute (WSI) at the University of Southampton is offering PhD studentships for multidisciplinary doctoral research with a particular focus on Human-Centred Artificial Intelligence (AI).
Lead by Dr. Paul Thompson, PhD, the AI in Medicine Collaboratory (AI-MEDx) at the Keck School of Medicine is a pioneering convergent research initiative committed to translating our research findings into tangible, patient-centric solutions through collaboration and partnership. Our mission is to leverage our unique strengths in medicine, data science, and the diverse patient population we ...
The Artificial Intelligence and Emerging Technologies in Medicine multidisciplinary training area of the PhD in Biomedical Sciences program offers students with solid quantitative and technical backgrounds educational and research opportunities in AI/machine learning, next generation medical technologies (medical devices, sensors, robotics, etc ...
The AIM PhD track prepares the next generation of leaders at the intersection of artificial intelligence and medicine. The program's mission is to train exceptional computational students, harnessing large-scale biomedical data and cutting-edge AI methods, to create new technologies and clinically impactful research that transform medicine ...
Artificial intelligence (AI) has transformed industries around the world, and has the potential to radically alter the field of healthcare. Imagine being able to analyze data on patient visits to the clinic, medications prescribed, lab tests, and procedures performed, as well as data outside the health system -- such as social media, purchases made using credit cards, census records, Internet ...
Plenary 1: Exceptional Medicine: AI+Health. Keynote Speaker: Jessica Mega, MD (Stanford); Fireside Chat Moderator: Curtis Langlotz, MD, PhD (Stanford) Our first keynote presentation will feature Dr. Jessica Mega, who will delve into the development of technology in healthcare and life science, the road to technology adoption, and the use of AI ...
Hugo Aerts PhD is Director of the Artificial Intelligence in Medicine (AIM) Program at Harvard-MGB. AIM's mission is to accelerate the application of AI algorithms in medical sciences and clinical practice. This academic program centralizes AI expertise stimulating cross-pollination among clinical and technical expertise areas, and provides a ...
Artificial Intelligence (AI) has the potential to transform health and healthcare systems globally, yet few individuals have the required skills and training. To address this challenge, our Centre For Doctoral Training (CDT) in AI-Enabled Healthcare Systems will create a unique interdisciplinary environment to train the brightest and best healthcare artificial intelligence
The Artificial Intelligence in Medicine (AIM) PhD track, led by co-Directors DBMI Chair Isaac "Zak" Kohane and HMS Professor of Medicine and Epidemiology Sebastian Schneeweiss, is an interdisciplinary program designed to train the next generation of academic and industry leaders in harnessing real-world health data and AI methods to ...
CCAIM has been set up as a cutting-edge research group. Its faculty of 10 University of Cambridge researchers - in addition to world-class PhD students, currently being recruited - have united to develop AI and machine learning (ML) technologies aiming to transform clinical trials, personalised medicine and biomedical discovery. The centre's Director is Professor Mihaela van der Schaar ...
The Windreich Department of Artificial Intelligence and Human Health at the Icahn School of Medicine at Mount Sinai and its centers and institutes embrace the promise of artificial intelligence (AI) to fundamentally revolutionize health care. By enabling us to develop more accurate diagnoses, expedite drug discovery, and deliver greater ...
The Artificial Intelligence in Medicine (AIM) PhD track will be led by co-directors DBMI Chair Isaac "Zak" Kohane and Harvard Medical School Professor of Medicine and Epidemiology Sebastian Schneeweiss. The program's mission is to train exceptional computational students, harnessing large-scale biomedical data and cutting-edge AI methods ...
Students: We welcome applications from undergraduate, graduate, and post-doctoral students, as well as visiting researchers with a background in artificial intelligence, software engineering, or medicine. If you are a student at Harvard or Stanford or a medical doctor, we encourage you to apply through the Medical AI Bootcamp.
Developing an Artificial Intelligence (AI)-based self-training platform for laparoscopic surgery. University of Dundee School of Medicine. Various research throughout the years have been devoted to exploring different and more effective ways of training for laparoscopy, within the conventional frameworks of using box trainers and virtual ...
PhD Program Toggle PhD Program menu options. Program Faculty; Admissions Information Toggle Admissions Information menu options. ... Artificial Intelligence in Medicine I; Artificial Intelligence in Medicine I. Course Code: HINF 5012 . Course Director: Fei Wang, Ph.D. Credits: 3
AI in Medicine. develops algorithms and models to improve medicine for patients and healthcare professionals. Our aim is to develop artificial intelligence (AI) and machine learning (ML) techniques for the analysis and interpretation of biomedical data. The group focuses on pursuing blue-sky research, including:
10 - 11 AM Panel I. Generative AI in Medical Education, Basic Science, Clinical Practice. Moderated by Annie Hartley, MD, PhD, MPH. Panelists: Hua Xu, PhD Robert T. McCluskey Professor of Biomedical Informatics and Data Science; Vice Chair for Research and Development, Section of Biomedical Informatics and Data Science; Assistant Dean for Biomedical Informatics, Yale School of Medicine
Artificial intelligence (AI) has been extensively researched in medicine, but its practical application remains limited. Meanwhile, there are various disparities in existing AI-enabled clinical ...
"When the world got introduced to generative AI, in health care—and pretty much every aspect of our daily lives—the potential of AI became very powerful," says Michael Hasselberg '13N (PhD) an associate professor of psychiatry, clinical nursing, and data science, and the University of Rochester's inaugural chief digital health officer.
Getting to patients more quickly. Other headline speakers were Shauna Overgaard, Ph.D., senior director of AI Strategy and Frameworks, and co-director of the AI Validation and Stewardship Research Program at Mayo Clinic; Thomas Fuchs, D.Sc., dean of Artificial Intelligence and Human Health at the Icahn School of Medicine at Mount Sinai; Anant Madabhushi, Ph.D., executive director for the Emory ...
Seemingly overnight, artificial intelligence has caught fire in healthcare, with feverish interest from boards, the C-suite, medical staff, information technology and revenue cycle management ...
Modern medicine has an empathy problem. Artificial intelligence — done right — might be able to help ease it. Despite the proliferation of communication training programs over the past decade ...