- Research article
- Open access
- Published: 07 May 2014

The endogenous and reactive depression subtypes revisited: integrative animal and human studies implicate multiple distinct molecular mechanisms underlying major depressive disorder
- Karim Malki 1 ,
- Robert Keers 1 ,
- Maria Grazia Tosto 1 , 2 ,
- Anbarasu Lourdusamy 3 ,
- Lucia Carboni 4 ,
- Enrico Domenici 5 nAff6 ,
- Rudolf Uher 1 , 7 ,
- Peter McGuffin 1 &
- Leonard C Schalkwyk 1
BMC Medicine volume 12 , Article number: 73 ( 2014 ) Cite this article
12k Accesses
50 Citations
21 Altmetric
Metrics details
Traditional diagnoses of major depressive disorder (MDD) suggested that the presence or absence of stress prior to onset results in either ‘reactive’ or ‘endogenous’ subtypes of the disorder, respectively. Several lines of research suggest that the biological underpinnings of ‘reactive’ or ‘endogenous’ subtypes may also differ, resulting in differential response to treatment. We investigated this hypothesis by comparing the gene-expression profiles of three animal models of ‘reactive’ and ‘endogenous’ depression. We then translated these findings to clinical samples using a human post-mortem mRNA study.
Affymetrix mouse whole-genome oligonucleotide arrays were used to measure gene expression from hippocampal tissues of 144 mice from the Genome-based Therapeutic Drugs for Depression (GENDEP) project. The study used four inbred mouse strains and two depressogenic ‘stress’ protocols (maternal separation and Unpredictable Chronic Mild Stress) to model ‘reactive’ depression. Stress-related mRNA differences in mouse were compared with a parallel mRNA study using Flinders Sensitive and Resistant rat lines as a model of ‘endogenous’ depression. Convergent genes differentially expressed across the animal studies were used to inform candidate gene selection in a human mRNA post-mortem case control study from the Stanley Brain Consortium.
In the mouse ‘reactive’ model, the expression of 350 genes changed in response to early stresses and 370 in response to late stresses. A minimal genetic overlap (less than 8.8%) was detected in response to both stress protocols, but 30% of these genes (21) were also differentially regulated in the ‘endogenous’ rat study. This overlap is significantly greater than expected by chance. The VAMP-2 gene, differentially expressed across the rodent studies, was also significantly altered in the human study after correcting for multiple testing.
Conclusions
Our results suggest that ‘endogenous’ and ‘reactive’ subtypes of depression are associated with largely distinct changes in gene-expression. However, they also suggest that the molecular signature of ‘reactive’ depression caused by early stressors differs considerably from that of ‘reactive’ depression caused by late stressors. A small set of genes was consistently dysregulated across each paradigm and in post-mortem brain tissue of depressed patients suggesting a final common pathway to the disorder. These genes included the VAMP-2 gene, which has previously been associated with Axis-I disorders including MDD, bipolar depression, schizophrenia and with antidepressant treatment response. We also discuss the implications of our findings for disease classification, personalized medicine and case-control studies of MDD.
Peer Review reports
Although antidepressants remain the first line treatment for major depressive disorder (MDD), antidepressant response varies considerably between individuals: fewer than half of all patients achieve remission following their first course of treatment [ 1 ]. The absence of robust predictors of treatment response means that the most effective antidepressant for a given patient is currently identified by trial and error. This is often a long and costly process which both delays recovery and has a negative effect on long-term outcome [ 2 ].
Clinicians have long intuited that heterogeneity in treatment response is the direct result of etiological heterogeneity in MDD [ 3 ]. Indeed, traditional diagnoses of major depression proposed that the presence or absence of stress prior to the onset of MDD results in two etiologically distinct subgroups of the disorder with different treatment recommendations. Early studies, which categorized these subtypes as ‘reactive’ (occurring as the result of a stressor) or ‘endogenous’ (occurring in the absence of stress), suggested that those with ‘endogenous’ depression responded more favorably to tricyclic antidepressants (TCAs) than selective serotonin reuptake inhibitors (SSRIs) [ 4 ]. While the validity of these subtypes remains unclear, reports continue to show that both distal stress (occurring early in life [ 5 ]) and proximal stress (occurring near the onset of a depressive episode [ 6 ]) are predictive of treatment response.
It remains unclear how the presence or absence of stress in the etiology of MDD affects response to treatment. However, it has been suggested that ‘endogenous’ and ‘reactive’ subtypes of depression are associated with largely distinct biological mechanisms, which respond differentially to treatment [ 3 ]. In line with this hypothesis, a recent animal study reported that the hippocampal gene-expression profile of a ‘reactive’ model of depression (induced by chronic restraint stress) differed considerably from that of an ‘endogenous’ model [ 7 ].
While this study suggests that the gene-expression profiles of ‘reactive’ depression caused by proximal stress may indeed differ from ‘endogenous’ depression, the role of distal early-life stress in this distinction remains unknown. Several studies have highlighted the importance of the timing of adversity and show that early and late stressors may have differential tissue-specific effects on gene-expression in the hippocampus [ 8 – 12 ]. The pathophysiological processes underlying MDD may therefore differ not only in the presence or absence of a stressor, but also by the timing of adversity (distal vs . proximal stress).
We investigated this hypothesis by exploring hippocampal gene-expression (mRNA) differences in three animal models of depression chosen to represent ‘reactive’ and ‘endogenous’ depression. In the ‘reactive’ depression model, mice were exposed to either distal stress (maternal separation) or proximal stress (unpredictable chronic mild stress). Flinders sensitive rats, which show congenital depression-like behavior, were used to model ‘endogenous’ depression.
Whole genome transcription profiles from disease relevant brain tissues in animals may provide valuable support and important information on the molecular mechanisms that may be relevant in humans. Nevertheless, the specific features of psychiatric illnesses means that molecular mechanisms uncovered in animal models are only suggestive and need to be validated in human studies [ 13 , 14 ]. We therefore used findings from the animal models to inform probe set prioritization in a comparable human post-mortem case-control study of depression from the Stanley Brain Consortium. Specifically, we hypothesize that a set of genes that shows concordant expression differences in response to ‘reactive’ and ‘endogenous’ depression models in the rodent studies may represent a common final pathway to MDD. These same genes may therefore also be differentially regulated in the post-mortem brain tissue of humans with the disorder.
Genome-wide expression profiling of the hippocampus (HIP) from two studies from the rodent arm of the Genome-based Therapeutic Drugs for Depression (GENDEP) study [ 15 ] was used to inform candidate gene selection in a comparable human post-mortem, case-control study on MDD from the Stanley Brain Consortium. The GENDEP project is a large-scale, multi-center human pharmacogenomics study that also includes a series of large-scale studies using animal models and in vitro experiments. The GENDEP project was designed to allow for integrative analysis of the results of the transcriptomics and proteomics on the samples from the human, the rodent and the in vitro studies, in order to gain further insight into the molecular mechanisms of MDD and identify biomarkers of antidepressant drugs (AD) treatment response. The mouse study used 144 animals from four strains of well-characterized inbred mice to model individual variation in humans. The mice were subjected to one of two stress protocols and a control condition (maternal separation (MS) - ‘early stress’, unpredictable chronic mild stress (UCMS) - ‘late stress’ - or the control condition (ENV)) to model ‘reactive’ depression. Litters of each strain were randomly allocated to the MS, UCMS or control group. Findings from the mouse study were cross validated in a parallel rat study that compared HIP mRNA differences between Flinders Sensitive and Flinders Resistant rat lines as models of ‘endogenous’ depression. Finally, genes differentially expressed in response to both stress protocols in the mouse study and in the rat study were used to inform probe set selection in comparable mRNA expression study in humans.
A total of 144 male and female mice (72 of each sex) from four different strains ((129S1/SvImJ, C57LB/6 J, DBA/2 J and FVB/NJ) were bred in the barrier unit at the Institute of Psychiatry, London, UK. Weaning took place when the animals were 21 to 28 days old. Animals were group-housed under standard conditions with a 12:12 h light:dark cycle, 22°C ± 11°C, food and water ad libitum . A total of 144 animals were sacrificed by cervical dislocation. Animals used for the transcriptomic study were not behaviorally tested. The hippocampus, liver and spleen were dissected following previously published protocols [ 16 , 17 ]. All housing and experimental procedures were carried out in accordance with the UK Home Office Animals (Scientific Procedures) Act, 1986.
A total of 39 animals from two cohorts of Flinders Sensitive Lines and Flinders Resistant Lines (22 FRL and 17 FSL) were bred and maintained at Karolinska Institutet (Stockholm) and housed under standard room temperature (22 ± 1°C), relative humidity (45 to 55%) and a 12 h light:dark schedule (light on at 07:00 a.m.). Food and water were available ad libitum . The study was conducted as part of a parallel GENDEP investigation. The Stockholm's Ethical Committee for Protection of Animals approved the study and all procedures were conducted in conformity with the Karolinska Institutet's guidelines for the care and use of laboratory animals, which follows the European Communities Council Directive of 24 November 1986. Additional information on the rat study is available elsewhere [ 18 ].
UCMS (Unpredictable Chronic Mild Stress)
In mice, ‘reactive’ depression caused by proximal stress was modeled using an Unpredictable Chronic Mild Stress (UCMS) paradigm. A third of the 144 mice (48 male and female mice) were exposed to varying stressors on a daily basis for a period of two weeks. Exposure to UCMS commenced when the animals were 10 weeks of age. The UCSM protocols included exposure to different stressors each day in a pseudorandom order. The stressors in the UCMS regime were based on previously published protocols including two hours of home cage tilting at 45°, damp bedding for four hours, cage switching for two hours, flooded cage for 10 minutes, altered length and time of light-dark cycle and air-puff [ 19 ]. Animals were exposed to either one or two stressors each day for varying lengths of time (Figure 1 ). All UCMS-exposed mice were tested and maintained under standard laboratory conditions but were single-housed. Following the UCMS regimen, a set of animals was tested with a battery of behavioral tests including Porsolt as an index of UCMS-evoked depressive-behavior [ 19 ]. However, all animals used for this mRNA characterization were not behaviorally tested to control for the potential stressor effects of the tests.
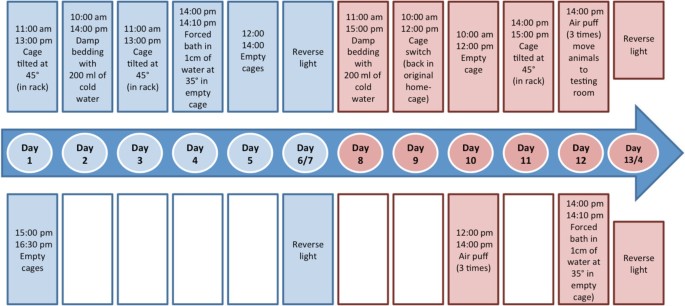
This figure shows the stress administration regime for the unpredictable chronic mild stress paradigm. The duration of the stress regime was for two consecutive weeks and the order of the different stressors was randomized. This figure shows the stressors and time/duration of administration for each of the two weeks.
MS (Maternal Separation)
A maternal separation protocol was used to model ‘reactive’ depression caused by distal stress in a further 48 mice. A single 24-hour separation of the pup from the dam at postnatal day (PND) 9 protocol was chosen to elicit a sufficiently strong biological response. Day of birth was defined as PND 0 for that particular litter. On postnatal Day 9 the dam was removed from the litter for 24 hours. The litter was kept on a heating pad in their home cage at 33°C ± 2°C in a different room than the dam in order to avoid contact through vocalization. Separated pups did not have access to food or water during their separation period. Litters were always separated and reunited with the mother during the first half of the light phase. The first hour after reuniting the litter with the mother was videotaped. Litters were of different sizes and when possible each litter came from a different breeding pair. A more detailed description of the litters is published elsewhere [ 19 ].
‘Endogenous’ model of depression
Flinders Sensitive Lines (FSL) and Flinders Resistant Lines (FRL) rats represent an ‘endogenous’ model of depression [ 20 – 23 ]. Flinders Lines are strains originally obtained by selective breeding of out-bred Sprague-Dawley rats (SD), according to their resistance or sensitivity to anticholinesterase diisopropyl fluorophosphates (DFP) treatment [ 24 ]. FSL are congenitally more sensitive to DFP and cholinergic agonists than FRL, which is a neurobiological feature shared with depressed cases in humans [ 21 ]. They also show many behavioral similarities to human depressed patients, including decreased psychomotor activity and appetite, cholinergic hypersensitivity, immune and sleep abnormalities including delay in rapid eye movement (REM) sleep but preserved cognitive function and hedonic response [ 25 ]. Flinders rats remain a robust model of depression to date [ 26 ].
mRNA extraction and lab protocols
Mouse brains, livers and spleens were dissected from each animal and frozen on dry ice. Total RNA was extracted from frozen hippocampal tissue and 3-ug RNA was processed using the One Cycle Target Labelling kit (Affymetrix, Santa Clara, CA, USA) and hybridized to the mouse MOE430v2 Gene Expression Array (Affymetrix) following standard Affymetrix protocols. Hippocampal mRNA extraction from Flinders rats was performed by another participating group from the GENDEP project [ 18 , 22 ]. Briefly, cRNA probes were obtained and hybridized to Affymetrix Rat Genome 230 2.0 using Affymetrix’s One-Cycle Eukaryotic Target Labelling Assay protocol. Protocols used for the human post-mortem mRNA extraction are described in detail in the paper by Iwamoto and colleagues [ 27 ]. Briefly, total RNA was extracted from 0.1 g of frozen prefrontal cortex tissues using Trizol (Invitrogen, Groningen, The Netherlands). A total of 8 to 10 mg of mRNA was reverse transcribed and synthesized into cDNA, hybridized onto Affymetrix HU95A oligonucleotide arrays and scanned using an HP GeneArray scanner (Hewlett-Packard, Palo Alto, CA, USA). Information on The Stanley Foundation brain collection and Neuropathology Consortium is found elsewhere [ 28 ].
Human samples
The human samples used in this study were donated to the Stanley Foundation Brain Collection at the Department of Psychiatry, University of the Health Sciences, Bethesda, MD, USA and have been made available to researchers world-wide. Human brain tissues were donated under standardized legislation according to the Uniform Anatomical Gift Act (USA). Information on Stanley Medical Research Institute (SMRI) and its research was offered to the next of kin at the time of the donation. Additional information is publically available from the Stanley Brain Consortium website [ 29 ]. The primary transcription-wide analysis was performed and described by Iwamoto and colleagues [ 27 ]. For consistency and quality assurance, the same subset has been used without additions or subtractions of cases. All data have been processed from raw files. The samples used consist of post-mortem prefrontal cortex from the Stanley Foundation Neuropathology Consortium from deceased patients affected with major depressive disorder and carefully matched controls. Exclusion criteria include poor mRNA quality and age (>65). A total of 26 samples, 11 cases and 15 controls, were used congruent with the primary data analysis (Table 1 ). Clinical diagnosis of MDD was made following Diagnostic and Statistical Manual of Mental Disorders – 4 th Edition (DSM-IV) diagnostic guidelines and reviewed independently by a pathologist and psychiatrist. Additional information on the human sample can be found in the Iwamoto and colleagues paper [ 27 ].
Statistical analysis of microarray data
Probe intensity data from 144 Affymetrix mouse whole-genome oligonucleotide arrays (MOE 430 v2) were normalized and summarized using the Robust Multichip Average (RMA method) [ 30 ]. Probe sets that were systematically absent (based on the MAS 5.0 detection present/absent call) across all the arrays were removed leaving 37,231 out of the original 45,101 probe sets. A battery of quality control metrics and exploratory analysis on the 144 arrays identified 10 arrays that differed significantly in quality. These arrays were removed for the purpose of the subsequent analysis; further description on normalization methods is available elsewhere [ 12 , 16 ].
In order to identify genes differentially expressed in response to early and late stress protocols we performed two sets of analyses. First, we compared normalized gene expression measurements between maternally separated animal (MS) and control (CON). Second, we compared normalized gene expression measurements between UCMS and CON. Differences were statistically evaluated using the non-parametric algorithms implemented in the RankProd package in the R environment [ 31 , 32 ]. RankProd enabled us to combine datasets from four different strains using a meta-analysis approach with the RPadvance function. This allowed us to circumvent issues arising from the predominant strain effects by evaluating differences within each strain first. Genes differentially expressed in a single strain were analyzed using rank product (RP) function from the same package, using the ‘data from single origin’ option. The P -values were calculated with 1,000,000 permutations, and multiple testing was taken into account by using the percentage of false prediction at the very conservative threshold of PFP <0.001. A common method to control for the number of rejected hypothesis in ‘omics’ study is to compute and report the false discovery rate (FDR) as proposed by Benjamini and Hochberg. The RankProd package returns proportion of false positive (PFP), which is a method proposed by Fernando and colleagues. Contrary to FDR, PFP does not rely on the correlation between tests and the number of tests performed [ 33 ]. Although PFP and FDR are often equated, the two methods differ in that PFP controls the proportion of accumulated false positives while FDR controls the expected proportion of false positive. FDR is not the best method to use in cases where there is a relationship between variables, which in mRNA studies is generally driven by genetic regulatory pathways and cross hybridization. We therefore corrected using the PFP method across all studies where we use the RankProd algorithim. The genes significantly altered were identified by the PANTHER classification system [ 34 ]. Genes with PFP <0.001 were subsequently uploaded to the Ingenuity database for pathway analysis with the Ingenuity Pathway Analysis (IPA) software (QIAGEN’s Ingenuity® Pathway Analysis (IPA®, Redwood City, USA) [ 35 ].
Expression data from FSL and FRL animals have been made available on the Gene Expression Omnibus (GEO; accession number GS2088, [ 36 ]. Data have been processed from raw. CEL files to ensure consistency of data analysis across all animal studies. To control for potential batch effects we combined the rat datasets from two cohorts using the ComBat function built into the inSilicoMerging package for the R environment [ 37 ]. Probe sets were normalized and summarized using Robust Multichip Average (RMA method). Probe sets that were systematically absent (based on the MAS 5.0 absent/present detection call) were removed. Probe-set summaries from FSL and FRL were then compared using the RankProd non-parametric algorithm implemented in R using the PRadvance function and single origin option. P -values were evaluated using 1,000,000 permutations. A conservative false discovery rate (PFP) threshold of P <0.001 and a change fold >1.5 was used. Probe sets that met the statistical thresholds were subsequently annotated using PANTHER [ 34 ] to obtain a list of gene symbols. We then matched all genes differentially expressed across all rodent studies using scripts written in Python [ 38 ]. Convergent genes differentially expressed across all rodent studies were subsequently analyzed using IPA software. Lastly, all genes differentially expressed in response to both “reactive” and “endogenous” models of depression were used to inform probe set selection in the human study.
Raw scores from 26 Affymetrix human oligonucleotide arrays (HU95A) were normalized and summarized into probe sets using the RMA method, which returned log2 transformed intensities [ 30 ]. Intensity distributions, profile correlations and quality control metrics were applied. MAS 5.0 expression values were calculated based on scaling to a target intensity of 100, then transformed by Log2 and calls were computed using the MAS5.0 present/absent algorithm. Affymetrix HU95A incorporates over 12,000 probe sets, tagging the expression of over 5,000 well-characterized genes. Human genes, ortholog to genes differentially expressed across all three rodent studies, were obtained using the Mouse Genome Informatics orthology query [ 39 ]. The Affymetrix Netaffx tool [ 40 ] was used to identify probe sets on the HU95A chip (Affymetrix) tagging the expression of the human genes. Expression differences between human MDD cases and controls were evaluated using the RankProd non-parametric algorithm implemented in R using the single origin function. Candidate genes in humans informed by the results from the mouse study were considered differentially expressed at a stringent corrected significance threshold PFP <0.05 using permutation testing with 1,000,000 permutations.
Gene expression profiles in ‘reactive’ depression models
The Rankprod method was used to identify the most robustly differentially expressed genes between ‘late’ (UCMS) stressed animals and control and between ‘early’ (MS) stressed animals and control. We considered only those genes that show consistency in the direction of change across all four strains. Inconsistency in the direction of change indicates Stress x Strain interaction effects, which are not specific to our research question. The results of this analysis uncovered 406 probe sets altered in response to UCMS across all four strains. These probes tag the expression of 370 known genes in mice. A summary of genes uncovered from this analysis with a previous association with stress response or MDD is presented in Table 1 . The results reveal a number of genes previously associated with UCMS protocols and believed to play a role in the pathogenesis of MDD. The same analysis was repeated to compare the maternally separated animal (MS) and control. The results from this analysis revealed 396 probe sets differentially regulated in response to the maternal separation protocol. These probe sets could be mapped to 350 known genes in mice. A summary of the top genes differentially expressed in response to maternal separation protocols is presented in Table 2 . We then explored the number of altered genes in response to either ‘early’ or ‘late’ stressors as well as the genetic overlap between the two conditions (Figure 2 ). There were remarkably few. Only 67 genes, less than 8.8% of significantly altered genes were in common between mice exposed to early and late stress paradigms.
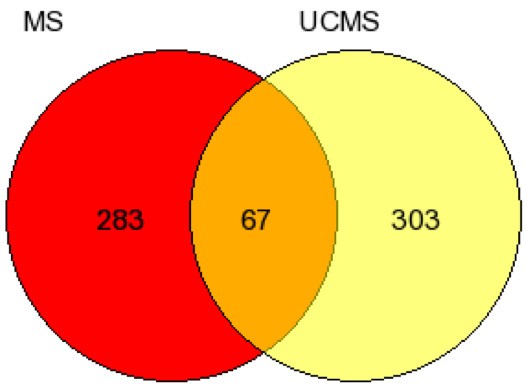
Venn diagram showing the number of genes significantly altered in response each depressogenic protocol. A compelling finding is the limited number of overlapping genes (approximately 8.8%) suggesting that etiologically different molecular mechanisms underpin a congruent set of behaviors.
The minimal gene expression overlap suggests that the biological mechanisms underpinning ‘reactive’ depression caused by early and late stressors differs considerably. In order to gain further understanding into these differences, genes differentially expressed for each of the two models were analyzed using IPA [ 35 ]. This allowed us to uncover gene networks showing the molecular relationship between the genes and evaluate networks according to the fit of significant genes in each dataset [ 12 ]. First, we explored gene networks associated with ‘late’ UCMS protocols. A total of 350 genes from our reference list were found on the IPA database. The top two functional networks identified by IPA have a score >42, with 29 reference molecules included in the first network and 23 in the second. Both networks were significantly associated with stress signaling response. The most significant transcriptional regulators included ELK1/2/4 TFIIA, SMARCB, CREB1 and THRB (see Additional file 1 : Figure S1 and Additional file 2 : Figure S2). We repeated the pathway analysis with genes differentially expressed in response to ‘early’ (MS) stressors. A total of 347 genes from our reference list were found on the IPA knowledge database. IPA returned three networks with a score >40. The associated functions of the top networks include mRNA post-transcriptional modification, protein synthesis and cellular development. The networks are associated with developmental and neurological disorders, which is a good match to the “early” stress protocol used. The top-ranking network (see Additional file 3 : Figure S3) includes 29 focus molecules from our reference gene set. The most prominent interacting genes within this network are with the Yhwaz and Yhwag . These genes are of particular interest as they have been systematically uncovered across several proteomic and transcriptomic studies from the GENDEP project in both mice and rats [ 12 , 13 , 17 , 22 ]. These genes show a direct interaction with the STK25 kinase, which plays a role in stress response. The second network (Additional file 4 : Figure S4) is composed of 27 molecules from our reference dataset. This network is centered on the NF-κB complex. The nuclear factor-κB (NF-κB) is a ubiquitous transcription factor involved in the regulation of gene expression and cell stress response and cell proliferation. Interestingly, NF-κB can be activated by different stimuli, including cytokines (such as TNF-α and IL-1): this finding is congruent with the inflammation hypothesis for MDD [ 41 – 45 ].
Gene expression profiles in ‘endogenous’ depression models
To gain an understanding of the similarities between stress-induced ‘reactive’ depression and a congenital ‘endogenous’ model of depression, we compared genes differentially regulated in response to early (MS) and late stress (UCMS) with mRNA differences between Flinders sensitive and resistant lines. Flinders lines are a genetic animal model of depression that allows us to cross-validate stress-altered genes within a parallel, independent mRNA study where depressive-behaviors occur in the absence of environmental stressors. The RankProd algorithm and conservative cut-offs described previously was used to evaluated mRNA differences between Flinders Sensitive and Flinders Resistant lines. The results revealed 715 down-regulated and 1,145 up-regulated probe sets. To obtain a list of gene names, probe sets obtained were subsequently annotated using PANTHER [ 34 ]. The probe sets tagged the expression of 501 down-regulated and 727 up-regulated genes. A total of 1,228 genes were used for cross-validation with the mouse study. First, we explored the genomic overlap between maternally deprived animals and Flinders line rats. From a total of 350 genes differentially regulated in maternally deprived mice, a total of 65 genes (19%) were also differentially regulated in rats. The same comparison was performed with genes differentially expressed in mice exposed to UCMS. A total of 52 genes (11%) were differentially expressed between ‘late’ stress animals and Flinders rats. A compelling finding is that 21 genes are differentially expressed in rats and in both early and late stressed mice (Figure 3 ). This is an important genetic overlap given that only 67 genes were commonly expressed between early and late stressed animals in the first place. Validations in an independent, methodologically different study using a genetic model of depression point to an important genetic overlap between stress-related and syndrome-related mechanisms. In order to gain further biological insight, genes significantly altered in response to both stresses in mice and between Flinders Sensitive and Resistant lines were carried forward for analysis using Ingenuity’s IPA system. All 21 genes were found in the Ingenuity reference database. A significant network with a score >40 consisting of over 55% of the reference molecules (12/21) was revealed (Figure 4 ). Among the genes in the pathway, four genes ( Ywhaz , Ppm1a , N kfb and Mapk1 ) are of particular interest as they have been previously reported across different “omics” GENDEP investigations [ 12 , 17 , 46 – 48 ].
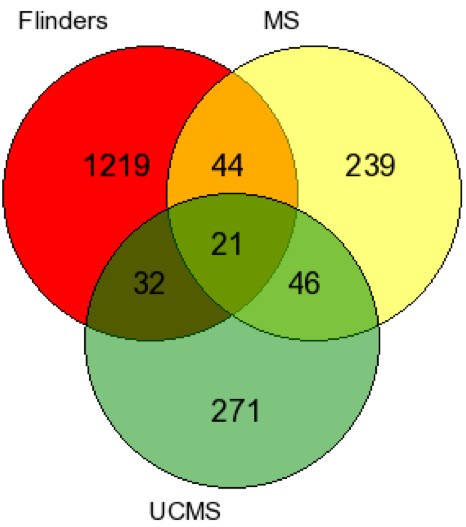
Venn diagram showing the number of genes overlapping across all rodent studies. Only 67 genes were differentially regulated in response to both early (MS) and late stressors (UCMS) pointing at minimal genetic overlap. However, many of these genes (approximately 30%) were also differentially regulated in an endogenous rat model of depression. The replication of these genes in a different organism that shows congenital depression-like symptoms, points at molecular mechanisms that may be involved in the human pathology.
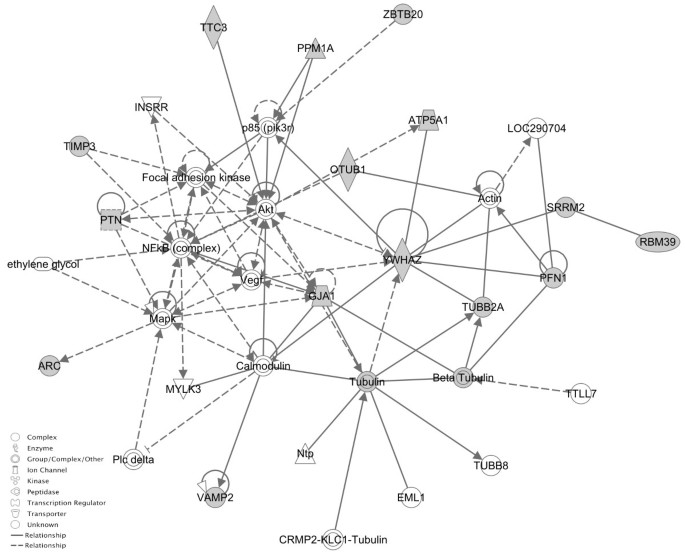
Network analysis performed on converging genes differentially regulated in response to different stresses in mouse and between different selected Flinders lines. The pathway comprises over half the reference molecules uploaded to the Ingenuity database system (12 out of the 21 reference molecules). The pathway implicates a number of genes previously associated with MDD and antidepressant treatment response, including Ppm1a, Ywhaz, NkFb and Mapk.
Translating findings to humans
From a total of 21 genes differentially expressed across all three rodent studies, 15 human orthologs were found. The expression of these 15 genes is tagged by 21 probe sets on the Affymetrix HU95A oligonucleotide array. The RankProd algorithm was used to evaluate expression between post-mortem cases and controls. Out of a total of 15 genes, the VAMP-2 is significantly down-regulated after correcting for the number of multiple non-independent tests using a prediction of false discovery rate of PFP <0.05 (Table 3 ). In our study, the Vesicle-Associated Membrane Protein 2 ( VAMP-2: Synaptobrevin2) gene is significantly altered across all rodent studies and in the human study.
The main objective of this study was to compare the genomic signatures of ‘reactive’ and ‘endogenous’ models of depression in three rodent studies and translate these findings in a human study. We found that all three animal models of depression had largely unique gene-expression profiles indicating divergent molecular mechanisms. Nevertheless, a small set of genes was consistently dysregulated across each paradigm and in the post-mortem brain tissue of depressed patients, which may represent a final common pathway to the disorder.
Gene-expression profiles of ‘endogenous’ and ‘reactive’ models of depression
Consistent with our hypothesis and with previous findings, the gene-expression profiles of both of our ‘reactive’ models of depression were largely distinct from our ‘endogenous’ model. Interestingly, this differed according to which ‘reactive’ depression paradigm was compared. For the early or stress ‘reactive’ model 19% of genes overlapped with the endogenous model, while for the late stress ‘reactive’ model the overlap was considerably lower at just 11%.
Surprisingly, the genomic signatures of our two ‘reactive’ models were more distinct from one another than the ‘endogenous’ model with fewer than 9% of genes shared between the two paradigms. This suggests that the two different models result in depressive-like behavior in mice through distinct biological mechanisms. Interestingly, gene pathway analysis returned plausible functional networks, with the more significant network for ‘early’ stressed animals associated with neurodevelopmental disorders and those of ‘late’ stressed animals associated with cell stress response and cell-signaling. Taken together, our results suggest that early exposure to stress modulates the expression of genes belonging to pathways associated with neurodevelopmental mechanisms. These changes may condition an individual’s exposure to later stresses and response to pharmacological and behavioral interventions later in life in yet unclear ways. Conversely, late onset stresses may act primarily on brain neurochemistry with neurostructural changes occurring via the cascading effects of neurochemically-related mechanisms, including neurogenesis and apoptosis [ 49 ].
Genes differentially regulated across all three paradigms
While each of our three animal models of depression showed largely distinct gene-expression profiles, a set of genes were differentially regulated across all three paradigms. Pathway analysis of these genes revealed a gene network which included Ppm1a , Ywhaz, Nkfb and Mapk .
All four genes have each been implicated in both the etiology of MDD and the response to treatment and may, therefore, represent a final common pathway to the disorder . We previously reported that the expression ppm1a was significantly modulated by the antidepressant nortriptyline. We have also shown that several single nucleotide polymorphisms in the human ortholog of this gene ( PPM1A ) predict a response to the same drug in a parallel human pharmacogenetic study [ 46 ]. Tyrosine 3-monooxygenase/tryptophan 5-monooxygenase activation protein ( Ywhaz ) has systematically been uncovered across several GENDEP studies and plays a role in cell proliferation and neurogenesis, which is a current explanatory model of MDD [ 50 – 54 ]. Moreover, it interacts with IRS1 protein and the MAPK pathway by modulating the activation of JNK1 and p38 MAPK both of which have been systematically associated with depressive mechanisms [ 52 – 56 ]. Lastly, the Nfkb gene has extensively been associated with peripheral inflammation and is consistent with the inflammation hypothesis for MDD [ 57 ].
Convergent animal-human genes
Animal models are an attractive proposition for the study of mood disorders as they allow access to disease relevant brain tissues and to control for environmental conditions. However, given the nature and characteristic of psychiatric disorders, there are aspects of these illnesses that can only be studied in humans. We therefore attempted to translate our set of convergent genes emerging from the rodent studies in a matching human post-mortem mRNA study of depressed cases and controls. One gene, the VAMP-2 gene, remained significantly down-regulated after correcting for multiple testing in the human study. The vesicle-associated membrane protein ( VAMP-2 ; synaptobrevin2) plays a role in the molecular regulation of transmitter release at the presynaptic plasma membrane. The expression of VAMP-2 has been found to be altered in both schizophrenia and bipolar disorder within a combined microarray analysis of the Stanley Foundation's brain collections [ 58 ]. Moreover, several other studies have implicated this gene in Axis-I psychiatric disorders and in antidepressant treatment response [ 59 – 62 ]. Previous studies have also shown that the VAMP2 /synaptobrevin-2 gene is increased in rat frontal cortex after chronic antidepressant treatment and repeated electro-convulsive therapy (ECT), although the finding has not been consistently replicated [ 63 , 64 ].
Implications
If replicated, the results of our study may have far reaching implications for both personalized medicine for MDD and case-control studies of the disorder.
Our findings suggest that etiological factors (such as proximal and distal stressors) could be used to indicate the molecular mechanisms at work in a given patient and, therefore, select the most effective treatment. Indeed, several studies have shown that proximal and distal stress predicts a response to antidepressants. Interestingly, while proximal stressors, such as divorce or job loss, have been linked with a good response [ 3 ], distal stressors, such as childhood maltreatment, are associated with a less favorable outcome [ 5 ]. Our results suggest that these contradictory findings may be explained by the divergent molecular mechanisms underlying ‘reactive’ depression caused by early versus late stressors. Nevertheless, further studies in clinical samples would be required to test this hypothesis.
Heterogeneity in the molecular mechanisms underlying depression could explain why, despite considerable efforts, genome-wide association studies (GWAS) of depression have yet to identify statistically significant associations with MDD [ 65 ]. This same heterogeneity may also explain the paucity of findings from pharmacogenetic studies of MDD, including the very large GWAS, NEWMEDS [ 66 ]. If the molecular mechanisms underlying MDD differ according to stress, it is plausible that different genetic variants would predict response to treatment in stressed and non-stressed individuals. In line with this, several studies have shown that genetic variants and stress have interdependent effects on antidepressant response [ 67 ].
While our findings highlight the heterogeneity of depression, they also suggest that a small set of genes may be involved in a final common pathway to the disorder. Replication of these findings in further transcriptomic studies of clinical samples is necessary before any firm conclusions can be drawn about the role of these genes in MDD. However, if they are successful, the existence of a final common pathway provides an exciting prospect for the development of novel antidepressants. If indeed the heterogeneity of MDD explains inter-individual variation in treatment response, it is plausible that antidepressants, which target this final common pathway, may prove to be effective for all patients, regardless of their etiological factors.
Limitations
Our findings should be considered in the context of several important limitations.
First, we used whole genome gene-expression data from four different samples in our study. While this approach allowed us to conduct integrative analyses across species and translate our findings from rodents to humans, it also meant that our analyses were subject to multiple testings. We used stringent thresholds both within and across analyses in order to protect against the risk of false positive findings. Nevertheless, in taking such an approach it is possible that we inflated the number of false negatives. Further replication of our results in larger independent samples is therefore necessary to confirm our findings.
Second, our rodent models focused exclusively on gene-expression in the hippocampus and did not include further brain structures implicated in the neurobiology of MDD, such as the amygdala. Moreover, limited public human depression hippocampal transcript array data were available at the time of analysis which meant that our human study used gene-expression data collected from a different, but still disease relevant, brain region (the prefrontal cortex). It is plausible, therefore, that the use of a different brain region resulted in false negatives in the human component of our study. Our findings, therefore, require confirmation in further brain regions in both animal and human samples.
Finally, it is important to note that rodents do not capture the complex characteristics of psychiatric illnesses that can only be fully investigated in human studies. Nevertheless, there are many advantages of animal models, which allow access to disease relevant brain tissues and control of environmental conditions. In the current study, we attempted to capture the full potential of both animal and human studies by conducting integrative analyses using several independent animal studies and translating results in a disease-relevant but different brain region in humans.
It is largely accepted that there are multiple causal pathways to MDD consisting of different combinations of genetic and environmental risk factors [ 67 ]. However, it remains unclear whether these factors converge on a unitary molecular mechanism underlying MDD or MDD consists of a heterogeneous group of disorders with multiple causal factors and distinct molecular mechanisms. Our findings provide support for both of these hypotheses. Using an animal model, we have shown that the presence and timing of stress determines distinct molecular processes underlying depressive behavior. However, we also identified a small set of genes which were consistently dysregulated across each stress paradigm and in post-mortem brain tissue of depressed patients suggestive of a final common pathway to the disorder. These genes included VAMP-2, a gene which has previously been associated with Axis-I disorders including MDD, bipolar depression, schizophrenia and with antidepressant treatment response.
Careful consideration of the etiological pathways to MDD may be key to dissecting the heterogeneity of the disorder and understanding and predicting response to treatment. Nevertheless, a final common pathway which unites the disparate etiologies of MDD may yet provide a target for novel treatments which are effective for all, rather than just subsets of patients.
Abbreviations
Diisopropyl fluorophosphates
False discovery rate
Flinders Resistant Line
Flinders Sensitive Line
Genome Wide Association Study
Hippocampus
Ingenuity Pathway Analysis
Major Depressive Disorder
Maternal Separation
Proportion of false positive
Post Natal Day
Robust Multichip Averaging
Selective Serotonin Reuptake Inhibitors
Tricyclic Antidepressants
Unpredictable Chronic Mild Stress.
Thase ME, Entsuah AR, Rudolph RL: Remission rates during treatment with venlafaxine or selective serotonin reuptake inhibitors. Br J Psychiatry. 2001, 178: 234-241.
Article CAS PubMed Google Scholar
Uher R, Huezo-Diaz P, Perroud N, Smith R, Rietschel M, Mors O, Hauser J, Maier W, Kozel D, Henigsberg N, Barreto M, Placentino A, Dernovsek MZ, Schulze TG, Kalember P, Zobel A, Czerski PM, Larsen ER, Souery D, Giovannini C, Gray JM, Lewis CM, Farmer A, Aitchison KJ, McGuffin P, Craig I: Genetic predictors of response to antidepressants in the GENDEP project. Pharmacogenomics J. 2009, 9: 225-233.
Keers R, Uher R: Gene-environment interaction in major depression and antidepressant treatment response. Curr Psychiatry Rep. 2012, 14: 129-137.
Article PubMed Google Scholar
Paroxetine: a selective serotonin reuptake inhibitor showing better tolerance, but weaker antidepressant effect than clomipramine in a controlled multicenter study. Danish University Antidepressant Group. J Affect Disord. 1990, 18: 289-299.
Nanni V, Uher R, Danese A: Childhood maltreatment predicts unfavorable course of illness and treatment outcome in depression: a meta-analysis. Am J Psychiatry. 2012, 169: 141-151.
Chen JD, Liu F, Xun GL, Chen HF, Hu MR, Guo XF, Xiao CQ, Wooderson SC, Guo WB, Zhao JP: Early and late onset, first-episode, treatment-naive depression: same clinical symptoms, different regional neural activities. J Affect Disord. 2012, 143: 56-63.
Andrus BM, Blizinsky K, Vedell PT, Dennis K, Shukla PK, Schaffer DJ, Radulovic J, Churchill GA, Redei EE: Gene expression patterns in the hippocampus and amygdala of endogenous depression and chronic stress models. Mol Psychiatry. 2012, 17: 49-61.
Alfonso J, Frasch AC, Flugge G: Chronic stress, depression and antidepressants: effects on gene transcription in the hippocampus. Rev Neurosci. 2005, 16: 43-56.
Champagne DL, Bagot RC, van Hasselt F, Ramakers G, Meaney MJ, de Kloet ER, Joels M, Krugers H: Maternal care and hippocampal plasticity: evidence for experience-dependent structural plasticity, altered synaptic functioning, and differential responsiveness to glucocorticoids and stress. J Neurosci. 2008, 28: 6037-6045.
Gotlib IH, Joormann J, Minor KL, Hallmayer J: HPA axis reactivity: a mechanism underlying the associations among 5-HTTLPR, stress, and depression. Biol Psychiatry. 2008, 63: 847-851.
Liu D, Diorio J, Tannenbaum B, Caldji C, Francis D, Freedman A, Sharma S, Pearson D, Plotsky PM, Meaney MJ: Maternal care, hippocampal glucocorticoid receptors, and hypothalamic-pituitary-adrenal responses to stress. Science. 1997, 277: 1659-1662.
Malki K, Lourdusamy A, Binder E, Payá-Cano J, Sluyter F, Craig I, Keers R, McGuffin P, Uher R, Schalkwyk LC: Antidepressant-dependent mRNA changes in mouse associated with hippocampal neurogenesis in a mouse model of depression. Pharmacogenet Genomics. 2012, 22: 765-776.
Carboni L, Becchi S, Piubelli C, Mallei A, Giambelli R, Razzoli M, Mathe AA, Popoli M, Domenici E: Early-life stress and antidepressants modulate peripheral biomarkers in a gene-environment rat model of depression. Prog Neuropsychopharmacol Biol Psychiatry. 2010, 34: 1037-1048.
Holmes PV: Rodent models of depression: reexamining validity without anthropomorphic inference. Crit Rev Neurobiol. 2003, 15: 143-174.
GENEDEP Official Site. [ http://gendep.iop.kcl.ac.uk ]
Malki K, Tosto MG, Jumabhoy I, Lourdusamy A, Sluyter F, Craig I, Uher R, McGuffin P, Schalkwyk LC: Integrative mouse and human mRNA studies using WGCNA nominates novel candidate genes involved in the pathogenesis of major depressive disorder. Pharmacogenomics. 2013, 14: 1979-1990.
Malki K, Campbell J, Davies M, Keers R, Uher R, Ward M, Paya-Cano J, Aitchinson KJ, Binder E, Sluyter F, Kuhn K, Selzer S, Craig I, McGuffin P, Schalkwyk LC: Pharmacoproteomic investigation into antidepressant response in two mouse inbred strains. Proteomics. 2012, 12: 2355-2365.
Piubelli C, Carboni L, Becchi S, Mathe AA, Domenici E: Regulation of cytoskeleton machinery, neurogenesis and energy metabolism pathways in a rat gene-environment model of depression revealed by proteomic analysis. Neuroscience. 2011, 176: 349-380.
Binder E, Malki K, Paya-Cano JL, Fernandes C, Aitchison KJ, Mathe AA, Sluyter F, Schalkwyk LC: Antidepressants and the resilience to early-life stress in inbred mouse strains. Pharmacogenet Genom. 2011, 21: 779-789.
Article CAS Google Scholar
Nishi K, Kanemaru K, Diksic M: A genetic rat model of depression, Flinders sensitive line, has a lower density of 5-HT1A receptors, but a higher density of 5-HT1B receptors, compared to control rats. Neurochem Int. 2009, 54: 299-307.
Osterlund MK, Overstreet DH, Hurd YL: The Flinders Sensitive Line rats, a genetic model of depression, show abnormal serotonin receptor mRNA expression in the brain that is reversed by 17 beta-estradiol. Mol Brain Res. 1999, 74: 158-166.
Blaveri E, Kelly F, Mallei A, Harris K, Taylor A, Reid J, Razzoli M, Carboni L, Piubelli C, Musazzi L, Racagni G, Mathé A, Popoli M, Domenici E, Bates S: Expression profiling of a genetic animal model of depression reveals novel molecular pathways underlying depressive-like behaviours. Plos One. 2010, 5: e12596.
Article PubMed PubMed Central Google Scholar
Friedman EM, Becker KA, Overstreet DH, Lawrence DA: Reduced primary antibody responses in a genetic animal model of depression. Psychosom Med. 2002, 64: 267-273.
Overstreet DH: The Flinders sensitive line rats: a genetic animal model of depression. Neurosci Biobehav Rev. 1993, 17: 51-68.
Overstreet DH: Commentary: a behavioral, psychopharmacological, and neurochemical update on the Flinders Sensitive Line rat, a potential genetic animal model of depression. Behav Genet. 1991, 21: 67-74.
Overstreet DH, Wegener G: The Flinders sensitive line rat model of depression–25 years and still producing. Pharmacol Rev. 2013, 65: 143-155.
Iwamoto K, Kakiuchi C, Bundo M, Ikeda K, Kato T: Molecular characterization of bipolar disorder by comparing gene expression profiles of postmortem brains of major mental disorders. Mol Psychiatry. 2004, 9: 406-416.
Torrey EF, Webster M, Knable M, Johnston N, Yolken RH: The Stanley Foundation Brain Collection and Neuropathology Consortium. Schizophr Res. 2000, 44: 151-155.
The Stanley Medical Research Institute. [ http://www.stanleyresearch.org ]
Irizarry RA, Hobbs B, Collin F, Beazer-Barclay YD, Antonellis KJ, Scherf U, Speed TP: Exploration, normalization, and summaries of high density oligonucleotide array probe level data. Biostatistics. 2003, 4: 249-264.
The R Project for Statistical Computing. [ http://www.r-project.org/ ]
Hong F, Breitling R, McEntee CW, Wittner BS, Nemhauser JL, Chory J: RankProd: a bioconductor package for detecting differentially expressed genes in meta-analysis. Bioinformatics. 2006, 22: 2825-2827.
Fernando RL, Nettleton D, Southey BR, Dekkers JC, Rothschild MF, Soller M: Controlling the proportion of false positives in multiple dependent tests. Genetics. 2004, 166: 611-619.
Article CAS PubMed PubMed Central Google Scholar
PANTHER Classification System. [ http://www.pantherdb.org ]
Ingenuity. [ http://www.ingenuity.com/ ]
Gene Expression Omnibus. [ http://www.ncbi.nlm.nih.gov/geo ]
inSilicoMerging. http://www.bioconductor.org/packages/release/bioc/html/inSilicoMerging.html .
Python™. [ http://www.python.org/ ]
Mouse Genome Informatics. [ http://www.informatics.jax.org/ ]
Affymetrix: Biology for a better world. [ http://www.affymetrix.com/analysis/index.affx ]
Zunszain PA, Hepgul N, Pariante CM: Inflammation and depression. Curr Top Behav Neurosci. 2013, 14: 135-151.
Miller AH, Maletic V, Raison CL: Inflammation and its discontents: the role of cytokines in the pathophysiology of major depression. Biol Psychiatry. 2009, 65: 732-741.
Gardner A, Boles RG: Beyond the serotonin hypothesis: mitochondria, inflammation and neurodegeneration in major depression and affective spectrum disorders. Prog Neuropsychopharmacol Biol Psychiatry. 2011, 35: 730-743.
Maes M: The cytokine hypothesis of depression: inflammation, oxidative & nitrosative stress (IO&NS) and leaky gut as new targets for adjunctive treatments in depression. Neuro Endocrinol Lett. 2008, 29: 287-291.
CAS PubMed Google Scholar
Berk M, Williams LJ, Jacka FN, O'Neil A, Pasco JA, Moylan S, Allen NB, Stuart AL, Hayley AC, Byrne ML, Maes M: So depression is an inflammatory disease, but where does the inflammation come from?. BMC Med. 2013, 11: 200.
PubMed PubMed Central Google Scholar
Malki K, Uher R, Paya-Cano J, Binder E, Rietschel M, Zobel A, Mors O, Hauser J, Henigsberg N, Jerman B, Souery D, Placentino A, Ng MY, Cohen-Woods S, Sluyter F, Farmer A, Aitchison KJ, Craig IW, Lewis CM, McGuffin P, Schalkwyk LC: Convergent animal and human evidence suggests a role of PPM1A gene in response to antidepressants. Biol Psychiatry. 2011, 69: 360-365.
Wegener G, Harvey BH, Bonefeld B, Muller HK, Volke V, Overstreet DH, Elfving B: Increased stress-evoked nitric oxide signalling in the Flinders sensitive line (FSL) rat: a genetic animal model of depression. Int J Neuropsychopharmacol. 2010, 13: 461-473.
Carboni L, Piubelli C, Pozzato C, Astner H, Arban R, Righetti PG, Hamdan M, Domenici E: Proteomic analysis of rat hippocampus after repeated psychosocial stress. Neuroscience. 2006, 137: 1237-1246.
Bremner JD, Narayan M, Anderson ER, Staib LH, Miller HL, Charney DS: Hippocampal volume reduction in major depression. Am J Psychiatry. 2000, 157: 115-118.
Choi JE, Hur W, Jung CK, Piao LS, Lyoo K, Hong SW, Kim SW, Yoon HY, Yoon SK: Silencing of 14-3-3zeta over-expression in hepatocellular carcinoma inhibits tumor growth and enhances chemosensitivity to cis-diammined dichloridoplatium. Cancer Lett. 2011, 303: 99-107.
Leivonen SK, Rokka A, Ostling P, Kohonen P, Corthals GL, Kallioniemi O, Perala M: Identification of miR-193b targets in breast cancer cells and systems biological analysis of their functional impact. Mol Cell Proteomics. 2011, 10: M110.005322.
Zhang Z, Luo X, Ding S, Chen J, Chen T, Chen X, Zha H, Yao L, He X, Peng H: MicroRNA-451 regulates p38 MAPK signaling by targeting of Ywhaz and suppresses the mesangial hypertrophy in early diabetic nephropathy. FEBS Lett. 2012, 586: 20-26.
Li XM, Li CC, Yu SS, Chen JT, Sabapathy K, Ruan DY: JNK1 contributes to metabotropic glutamate receptor-dependent long-term depression and short-term synaptic plasticity in the mice area hippocampal CA1. Eur J Neurosci. 2007, 25: 391-396.
Clarke M, Pentz R, Bobyn J, Hayley S: Stressor-like effects of c-Jun N-terminal kinase (JNK) inhibition. Plos One. 2012, 7: e44073.
Brust TB, Cayabyab FS, MacVicar BA: C-Jun N-terminal kinase regulates adenosine A1 receptor-mediated synaptic depression in the rat hippocampus. Neuropharmacology. 2007, 53: 906-917.
Miller AH, Raison CL: Cytokines, p38 MAP kinase and the pathophysiology of depression. Neuropsychopharmacology. 2006, 31: 2089-2090.
Maes M, Yirmyia R, Noraberg J, Brene S, Hibbeln J, Perini G, Kubera M, Bob P, Lerer B, Maj M: The inflammatory & neurodegenerative (I&ND) hypothesis of depression: leads for future research and new drug developments in depression. Metab Brain Dis. 2009, 24: 27-53.
Higgs BW, Elashoff M, Richman S, Barci B: An online database for brain disease research. BMC Genomics. 2006, 7: 70.
Chana G, Lucero G, Salaria S, Lozach J, Du P, Woelk C, Everall I: Upregulation of NRG-1 and VAMP-1 in human brain aggregates exposed to clozapine. Schizophr Res. 2009, 113: 273-276.
Fung SJ, Webster MJ, Weickert CS: Expression of VGluT1 and VGAT mRNAs in human dorsolateral prefrontal cortex during development and in schizophrenia. Brain Res. 2011, 1388: 22-31.
Munakata K, Iwamoto K, Bundo M, Kato T: Mitochondrial DNA 3243A > G mutation and increased expression of LARS2 gene in the brains of patients with bipolar disorder and schizophrenia. Biol Psychiatry. 2005, 57: 525-532.
Webster MJ, Elashoff M, Weickert CS: Molecular evidence that cortical synaptic growth predominates during the first decade of life in humans. Int J Dev Neurosci. 2011, 29: 225-236.
Yamada M, Takahashi K, Tsunoda M, Nishioka G, Kudo K, Ohata H, Kamijima K, Higuchi T, Momose K, Yamada M: Differential expression of VAMP2/synaptobrevin-2 after antidepressant and electroconvulsive treatment in rat frontal cortex. Pharmacogenomics J. 2002, 2: 377-382.
Saito S, Takahashi N, Ishihara R, Ikeda M, Suzuki T, Kitajima T, Yamanouchi Y, Iwata N, Yamada M, Yoshida K, Inada T, Ozaki N: Association study between vesicle-associated membrane protein 2 gene polymorphisms and fluvoxamine response in Japanese major depressive patients. Neuropsychobiology. 2006, 54: 226-230.
Ripke S, Wray NR, Lewis CM, Hamilton SP, Weissman MM, Breen G, Byrne EM, Blackwood DH, Boomsma DI, Cichon S, Heath AC, Holsboer F, Lucae S, Madden PA, Martin NG, McGuffin P, Muglia P, Noethen MM, Penninx BP, Pergadia ML, Potash JB, Rietschel M, Lin D, Müller-Myhsok B, Shi J, Steinberg S, Grabe HJ, Lichtenstein P, Magnusson P, Major Depressive Disorder Working Group of the Psychiatric GWAS Consortium, et al: A mega-analysis of genome-wide association studies for major depressive disorder. Mol Psychiatry. 2013, 18: 497-511.
Tansey KE, Guipponi M, Perroud N, Bondolfi G, Domenici E, Evans D, Hall SK, Hauser J, Henigsberg N, Hu X, Jerman B, Maier W, Mors O, O'Donovan M, Peters TJ, Placentino A, Rietschel M, Souery D, Aitchison KJ, Craig I, Farmer A, Wendland JR, Malafosse A, Holmans P, Lewis G, Lewis CM, Stensbøl TB, Kapur S, McGuffin P, Uher R: Genetic predictors of response to serotonergic and noradrenergic antidepressants in major depressive disorder: a genome-wide analysis of individual-level data and a meta-analysis. PLoS Med. 2012, 9: e1001326.
Keers R, Uher R, Gupta B, Rietschel M, Schulze TG, Hauser J, Skibinska M, Henigsberg N, Kalember P, Maier W, Zobel A, Mors O, Kristensen AS, Kozel D, Giovannini C, Mendlewicz J, Kumar S, McGuffin P, Farmer AE, Aitchison KJ: Stressful life events, cognitive symptoms of depression and response to antidepressants in GENDEP. J Affect Disord. 2010, 127: 337-342.
Piva R, Belardo G, Santoro MG: NF-kappaB: a stress-regulated switch for cell survival. Antioxid Redox Signal. 2006, 8: 478-486.
Pace TW, Mletzko TC, Alagbe O, Musselman DL, Nemeroff CB, Miller AH, Heim CM: Increased stress-induced inflammatory responses in male patients with major depression and increased early life stress. Am J Psychiatry. 2006, 163: 1630-1633.
Pre-publication history
The pre-publication history for this paper can be accessed here: http://www.biomedcentral.com/1741-7015/12/73/prepub
Download references
Acknowledgements
The Genome-Based Therapeutic Drugs for Depression study was funded by a European Commission Framework 6 grant, EC Contract Ref: LSHB-CT-2003–503428. The Biomedical Research Centre for Mental Health at the Institute of Psychiatry, King’s College London and South London, and Maudsley National Health Service Foundation Trust (funded by the National Institute for Health Research, Department of Health, United Kingdom) and GlaxoSmithKline contributed by funding add-on projects at the London Centre. Dr. Uher is supported by the Canada Research Chairs program ( http://www.chairs-chaires.gc.ca/ ). RK is supported by an MRC Population Health Scientist Award (MR/K021281/1).
Author information
Enrico Domenici
Present address: Pharma Research and Early Development, F. Hoffmann–La Roche, Basel, Switzerland
Authors and Affiliations
King’s College London, MRC Social, Genetic and Developmental Psychiatry Centre, at Institute of Psychiatry, SGDP Research Centre (PO80), De Crespigny Park, Denmark Hill, London, SE5 8AF, UK
Karim Malki, Robert Keers, Maria Grazia Tosto, Rudolf Uher, Peter McGuffin & Leonard C Schalkwyk
Department of Psychology, University of York, York, UK
Maria Grazia Tosto
Queen’s Medical Centre, University of Nottingham, Nottingham, UK
Anbarasu Lourdusamy
Department of Pharmacy and Biotechnology, Alma Mater Studiorum, University of Bologna, Bologna, Italy
Lucia Carboni
Center of Excellence for Drug Discovery in Neuroscience, GlaxoSmithKline Medicines Research Centre, Verona, Italy
Department of Psychiatry, Dalhousie University, Halifax, NS, Canada
Rudolf Uher
You can also search for this author in PubMed Google Scholar
Corresponding authors
Correspondence to Karim Malki or Robert Keers .
Additional information
Competing interests.
Prof. Peter McGuffin, Dr. Enrico Domenici and Dr. Lucia Carboni have received consultancy fees and honoraria for participating in expert panels from pharmaceutical companies, including Roche and GlaxoSmithKline. All other authors report no biomedical financial interests or potential conflicts of interest.
Authors’ contributions
KM and RK analyzed the data and conceived the study. MGT and AL contributed to the analyses and methods section. ED and LC conducted the study on the Flinders rats and contributed to the revisions of the manuscript. RU contributed to the design. PM is the GENDEP PI and contributed to the revision and LS conducted the mouse study and revised the manuscript. All authors read and approved the final version of the manuscript.
Karim Malki, Robert Keers contributed equally to this work.
Electronic supplementary material
12916_2013_967_moesm1_esm.png.
Additional file 1: Figure S1: Gene network obtained from genes differentially expressed in response to the UCMS protocol. The most significant network returned from the Ingenuity Pathway Analysis software for genes differentially expressed in response to Unpredictable Chronic Mild Stress. The network consists of 29 reference molecules and is significantly associated with cell stress response. (PNG 2 MB)
12916_2013_967_MOESM2_ESM.png
Additional file 2: Figure S2: Second gene network obtained from genes differentially expressed in response to the UCMS protocol. A second significant network with a score >42 returned by the Ingenuity Pathway Analysis software for genes differentially expressed in the mouse study in response to the Unpredictable Chronic Mild Stress protocol. The pathway includes 23 reference molecules and it is also associated with cell stress response. The pathway is centered on the ELK complex hub and is of particular interest as it shows the VAMP-2 complex and its association with the N-type calcium channel and potassium channel. (PNG 2 MB)
12916_2013_967_MOESM3_ESM.png
Additional file 3: Figure S3: Gene network obtained from genes differentially expressed in response to the Maternal Separation protocol. The most significant network returned from the Ingenuity Pathway Analysis software for genes differentially expressed in response to the maternal separation depressogenic protocol. Of particular interest is the presence of the Yhwaz reference molecule. The Yhwaz gene has been systematically uncovered across several animal studies and been shown to influence neurotransmission of dopamine by regulating exocytosis or phosphorylation of synaptic proteins. The pathway consists of 29 reference molecules and is associated with cell stress response. (PNG 2 MB)
12916_2013_967_MOESM4_ESM.png
Additional file 4: Figure S4: Second Gene network obtained from genes differentially expressed in response to the Maternal Separation protocol. Second pathway returned from the Ingenuity Pathway Analysis software for genes differentially expressed in response to the maternal separation protocol in mouse. This pathway includes 27 reference molecules and is centered on the NF-κB hub. The pathway is associated with cell proliferation and the NF-κB hub has been previously found to be associated with inflammation. Activation of the NF-κB transcription family, by nuclear translocation of cytoplasmic complexes, plays a central role in inflammation [ 68 ]. Human studies have shown that MDD patients with increased early life stress exhibit enhanced inflammatory responsiveness to psychosocial stress [ 69 ]. (PNG 2 MB)
Authors’ original submitted files for images
Below are the links to the authors’ original submitted files for images.
Authors’ original file for figure 1
Authors’ original file for figure 2, authors’ original file for figure 3, authors’ original file for figure 4, rights and permissions.
This article is published under license to BioMed Central Ltd. This is an Open Access article distributed under the terms of the Creative Commons Attribution License ( http://creativecommons.org/licenses/by/4.0 ), which permits unrestricted use, distribution, and reproduction in any medium, provided the original work is properly cited.
Reprints and permissions
About this article
Cite this article.
Malki, K., Keers, R., Tosto, M.G. et al. The endogenous and reactive depression subtypes revisited: integrative animal and human studies implicate multiple distinct molecular mechanisms underlying major depressive disorder. BMC Med 12 , 73 (2014). https://doi.org/10.1186/1741-7015-12-73
Download citation
Received : 13 November 2013
Accepted : 10 April 2014
Published : 07 May 2014
DOI : https://doi.org/10.1186/1741-7015-12-73
Share this article
Anyone you share the following link with will be able to read this content:
Sorry, a shareable link is not currently available for this article.
Provided by the Springer Nature SharedIt content-sharing initiative
- Endogenous Depression
- Reactive Depression
- Stanley Brain Consortium
View archived comments (1)
BMC Medicine
ISSN: 1741-7015
- General enquiries: [email protected]

An official website of the United States government
The .gov means it’s official. Federal government websites often end in .gov or .mil. Before sharing sensitive information, make sure you’re on a federal government site.
The site is secure. The https:// ensures that you are connecting to the official website and that any information you provide is encrypted and transmitted securely.
- Publications
- Account settings
- My Bibliography
- Collections
- Citation manager
Save citation to file
Email citation, add to collections.
- Create a new collection
- Add to an existing collection
Add to My Bibliography
Your saved search, create a file for external citation management software, your rss feed.
- Search in PubMed
- Search in NLM Catalog
- Add to Search
The endogenous and reactive depression subtypes revisited: integrative animal and human studies implicate multiple distinct molecular mechanisms underlying major depressive disorder
Affiliation.
- 1 King's College London, MRC Social, Genetic and Developmental Psychiatry Centre, at Institute of Psychiatry, SGDP Research Centre (PO80), De Crespigny Park, Denmark Hill, London SE5 8AF, UK. [email protected].
- PMID: 24886127
- PMCID: PMC4046519
- DOI: 10.1186/1741-7015-12-73
Background: Traditional diagnoses of major depressive disorder (MDD) suggested that the presence or absence of stress prior to onset results in either 'reactive' or 'endogenous' subtypes of the disorder, respectively. Several lines of research suggest that the biological underpinnings of 'reactive' or 'endogenous' subtypes may also differ, resulting in differential response to treatment. We investigated this hypothesis by comparing the gene-expression profiles of three animal models of 'reactive' and 'endogenous' depression. We then translated these findings to clinical samples using a human post-mortem mRNA study.
Methods: Affymetrix mouse whole-genome oligonucleotide arrays were used to measure gene expression from hippocampal tissues of 144 mice from the Genome-based Therapeutic Drugs for Depression (GENDEP) project. The study used four inbred mouse strains and two depressogenic 'stress' protocols (maternal separation and Unpredictable Chronic Mild Stress) to model 'reactive' depression. Stress-related mRNA differences in mouse were compared with a parallel mRNA study using Flinders Sensitive and Resistant rat lines as a model of 'endogenous' depression. Convergent genes differentially expressed across the animal studies were used to inform candidate gene selection in a human mRNA post-mortem case control study from the Stanley Brain Consortium.
Results: In the mouse 'reactive' model, the expression of 350 genes changed in response to early stresses and 370 in response to late stresses. A minimal genetic overlap (less than 8.8%) was detected in response to both stress protocols, but 30% of these genes (21) were also differentially regulated in the 'endogenous' rat study. This overlap is significantly greater than expected by chance. The VAMP-2 gene, differentially expressed across the rodent studies, was also significantly altered in the human study after correcting for multiple testing.
Conclusions: Our results suggest that 'endogenous' and 'reactive' subtypes of depression are associated with largely distinct changes in gene-expression. However, they also suggest that the molecular signature of 'reactive' depression caused by early stressors differs considerably from that of 'reactive' depression caused by late stressors. A small set of genes was consistently dysregulated across each paradigm and in post-mortem brain tissue of depressed patients suggesting a final common pathway to the disorder. These genes included the VAMP-2 gene, which has previously been associated with Axis-I disorders including MDD, bipolar depression, schizophrenia and with antidepressant treatment response. We also discuss the implications of our findings for disease classification, personalized medicine and case-control studies of MDD.
PubMed Disclaimer
Similar articles
- Gene expression patterns in the hippocampus and amygdala of endogenous depression and chronic stress models. Andrus BM, Blizinsky K, Vedell PT, Dennis K, Shukla PK, Schaffer DJ, Radulovic J, Churchill GA, Redei EE. Andrus BM, et al. Mol Psychiatry. 2012 Jan;17(1):49-61. doi: 10.1038/mp.2010.119. Epub 2010 Nov 16. Mol Psychiatry. 2012. PMID: 21079605 Free PMC article.
- Pervasive and opposing effects of Unpredictable Chronic Mild Stress (UCMS) on hippocampal gene expression in BALB/cJ and C57BL/6J mouse strains. Malki K, Mineur YS, Tosto MG, Campbell J, Karia P, Jumabhoy I, Sluyter F, Crusio WE, Schalkwyk LC. Malki K, et al. BMC Genomics. 2015 Apr 3;16(1):262. doi: 10.1186/s12864-015-1431-6. BMC Genomics. 2015. PMID: 25879669 Free PMC article.
- Identification of genes and gene pathways associated with major depressive disorder by integrative brain analysis of rat and human prefrontal cortex transcriptomes. Malki K, Pain O, Tosto MG, Du Rietz E, Carboni L, Schalkwyk LC. Malki K, et al. Transl Psychiatry. 2015 Mar 3;5(3):e519. doi: 10.1038/tp.2015.15. Transl Psychiatry. 2015. PMID: 25734512 Free PMC article.
- Epidemiology of subtypes of depression. Kessing LV. Kessing LV. Acta Psychiatr Scand Suppl. 2007;(433):85-9. doi: 10.1111/j.1600-0447.2007.00966.x. Acta Psychiatr Scand Suppl. 2007. PMID: 17280574 Review.
- Histone Modifications in Major Depressive Disorder and Related Rodent Models. Deussing JM, Jakovcevski M. Deussing JM, et al. Adv Exp Med Biol. 2017;978:169-183. doi: 10.1007/978-3-319-53889-1_9. Adv Exp Med Biol. 2017. PMID: 28523546 Review.
- Long-Term Effects of Maternal Depression during Postpartum and Early Parenthood Period on Child Socioemotional Development. Suarez A, Shraibman L, Yakupova V. Suarez A, et al. Children (Basel). 2023 Oct 23;10(10):1718. doi: 10.3390/children10101718. Children (Basel). 2023. PMID: 37892381 Free PMC article.
- Microglial Responses to Stress-Induced Depression: Causes and Consequences. Afridi R, Suk K. Afridi R, et al. Cells. 2023 May 31;12(11):1521. doi: 10.3390/cells12111521. Cells. 2023. PMID: 37296642 Free PMC article. Review.
- Connecting Dots between Mitochondrial Dysfunction and Depression. Khan M, Baussan Y, Hebert-Chatelain E. Khan M, et al. Biomolecules. 2023 Apr 20;13(4):695. doi: 10.3390/biom13040695. Biomolecules. 2023. PMID: 37189442 Free PMC article. Review.
- COVID-19 pandemic's impact on networks of depression and anxiety in naturalistic transdiagnostic sample of outpatients with non-psychotic mental illness. Kim ST, Seo JH, Park CI, Kim SJ, Kang JI. Kim ST, et al. Front Psychiatry. 2023 Mar 13;14:1118942. doi: 10.3389/fpsyt.2023.1118942. eCollection 2023. Front Psychiatry. 2023. PMID: 36993919 Free PMC article.
- Identification and Validation of Common Reference Genes for Normalization of Esophageal Squamous Cell Carcinoma Gene Expression Profiles. Xu J, Yang M, Shao AZ, Pan HW, Fan YX, Chen KP. Xu J, et al. Biomed Res Int. 2022 Nov 23;2022:9125242. doi: 10.1155/2022/9125242. eCollection 2022. Biomed Res Int. 2022. PMID: 36467891 Free PMC article.
- Thase ME, Entsuah AR, Rudolph RL. Remission rates during treatment with venlafaxine or selective serotonin reuptake inhibitors. Br J Psychiatry. 2001;178:234–241. - PubMed
- Uher R, Huezo-Diaz P, Perroud N, Smith R, Rietschel M, Mors O, Hauser J, Maier W, Kozel D, Henigsberg N, Barreto M, Placentino A, Dernovsek MZ, Schulze TG, Kalember P, Zobel A, Czerski PM, Larsen ER, Souery D, Giovannini C, Gray JM, Lewis CM, Farmer A, Aitchison KJ, McGuffin P, Craig I. Genetic predictors of response to antidepressants in the GENDEP project. Pharmacogenomics J. 2009;9:225–233. - PubMed
- Keers R, Uher R. Gene-environment interaction in major depression and antidepressant treatment response. Curr Psychiatry Rep. 2012;14:129–137. - PubMed
- Paroxetine: a selective serotonin reuptake inhibitor showing better tolerance, but weaker antidepressant effect than clomipramine in a controlled multicenter study. Danish University Antidepressant Group. J Affect Disord. 1990;18:289–299. - PubMed
- Nanni V, Uher R, Danese A. Childhood maltreatment predicts unfavorable course of illness and treatment outcome in depression: a meta-analysis. Am J Psychiatry. 2012;169:141–151. - PubMed
Publication types
- Search in MeSH
Related information
- GEO DataSets
- Related Project

Grants and funding
- MR/K021281/1/Medical Research Council/United Kingdom
- Department of Health/United Kingdom
LinkOut - more resources
Full text sources.
- BioMed Central
- Europe PubMed Central
- PubMed Central
Other Literature Sources
- scite Smart Citations
- MedlinePlus Health Information
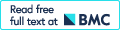
- Citation Manager
NCBI Literature Resources
MeSH PMC Bookshelf Disclaimer
The PubMed wordmark and PubMed logo are registered trademarks of the U.S. Department of Health and Human Services (HHS). Unauthorized use of these marks is strictly prohibited.

An official website of the United States government
The .gov means it’s official. Federal government websites often end in .gov or .mil. Before sharing sensitive information, make sure you’re on a federal government site.
The site is secure. The https:// ensures that you are connecting to the official website and that any information you provide is encrypted and transmitted securely.
- Publications
- Account settings
The PMC website is updating on October 15, 2024. Learn More or Try it out now .
- Advanced Search
- Journal List
- Dialogues Clin Neurosci
- v.10(3); 2008 Sep
Language: English | Spanish | French
Basic concepts of depression
Conceptos básicos sobre la depresión, concepts de base de la dépression, eugene s. paykel.
University of Cambridge, Department of Psychiatry, Cambridge, UK
This paper reviews concepts of depression, including history and classification. The original broad concept of melancholia included all forms of quiet insanity. The term depression began to appear in the nineteenth century as did the modern concept of affective disorders, with the core disturbance now viewed as one of mood. The 1930s saw the introduction of defined criteria into official diagnostic schemes. The modern separation into unipolar and bipolar disorder was introduced following empirical research by Angst and Perris in the 1960s. The partially overlapping distinctions between psychotic and neurotic depression, and between endogenous and reactive depression, started to generate debate in the 1920s, with considerable multivariate research in the 1960s. The symptom element in endogenous depression currently survives in melancholia or somatic syndrome. Life stress is common in various depressive pictures. Dysthymia, a valuable diagnosis, represents a form of what was regarded earlier as neurotic depression. Other subtypes are also discussed.
Este articulo revisa conceptos sobre la depresión, los que incluyen historia y clasificación. El amplio concepto original de melancolía incorporaba todas las formas leves de locura. El término depresión comenzó a aparecer en el siglo XIX, y al igual que el concepto moderno de trastornos afectivos, consideró como esencial la alteración del ánimo. En la década de 1980 se produjo la introducción de criterios definidos en esquemas diagnósticos oficiales. La moderna separación entre el trastorno unipolar y bipolar surgió a partir de la investigación empírica de Angst y Perris en la década de 1960. Las distinciones que se traslapan parcialmente entre depresión psicótica y neurótica, y entre depresión endógena y reactiva comenzaron a generar debate en la década de 1920 con una importante investigación de multiples variables en la década de 1960. El síntoma central de la depresión endógena perdura actualmente en la melancolía o el síndrome somático. El estrés de la vida es común a varios cuadros depresivos. La distimia, un diagnóstico útil, representa una forma de lo que antes se consideró la depresión neurótica. También se discuten otros subtipos de depresión.
Cet article fait le point sur les concepts de la dépression, incluant son histoire et sa classification. À l'origine, la notion large de mélancolie englobait toutes les formes de folie calme. Le terme de dépression est apparu au XIX e siècle, comme le concept moderne de troubles affectifs, les troubles de l'humeur étant aujourd'hui au coeur de la dépression. Des critères précis ont fait leur apparition dans les années 80 avec des arbres diagnostiques officiels. La séparation moderne entre maladie uni- et bipolaire a suivi la recherche empirique d'Angst et Perris dans les années 60. C'est dans les années 20 que les distinctions, bien que comprenant des similarités, entre dépression psychotique et névrotique, et entre dépression endogène et réactive ont commencé à faire débat, générant une recherche considérable dans les années 60. La mélancolie ou le syndrome somatique persistent aujourd'hui comme symptômes dans la dépression endogène. Les événements de vie stressants sont fréquemment retrouvés dans des tableaux dépressifs variés. La dysthymie, entité diagnostique valable, est une forme de ce que l'on appelait autrefois dépression névrotique. L'article analyse également d'autres types de dépression.
Historical background
Prior to the late 19th century, although detailed systems of classification abounded, the main problem for psychiatric nosology was the establishment of the broad major disorders. Melancholia was recognized as early as the time of Hippocrates, and continued through Galenic medicine and medieval times. The earlier connotation of the term was very wide, and included all forms of quiet insanity. It was linked with the humoral theory of causation, specifically, as the term indicates, with black bile.
Most psychiatric terms have changed meaning over their history, and they are always partly dependent on language. Melancholia later became more clearly associated with the more modern idea of melancholy or despair, for instance, in the classic work of the English Renaissance author, Richard Burton, The Anatomy of Melancholy, 1 first published in 1621. The alternation of melancholia and mania in what is now termed bipolar disorder or manic-depressive disorder, although in some respects suggested in the writings of Arateus of Cappadocia, and those of later authors, was not clearly described until 1854, independently by the French psychiatrists, Falret and Baillarger.The term depression also began to appear in the 19th century, to indicate a state of sadness. Detailed accounts of these aspects and later history can be found in Jackson' and Berrios. 3
When Kraepelin, in the late nineteenth century, built on the work of his predecessors and simplified it to delineate the foundations of the modem classification of psychiatric disorders, one of his major categories was that of manicdepressive insanity. Kraepelin's classic textbook went through successive editions, which included some changes in his views. Initially he distinguished a further category, involutional melancholia, but in later editions' he returned it to the manic-depressive category. The latter not only included cases of alternating mania and melancholia, but all cases of mania, and seemed to include all depressions. Kraepelin regarded psychiatric disorders as disease entities based on a medical, neurological model, with specific, organic etiology and pathology. He believed that manicdepressive insanity was largely independent of psychological stress. While such stress might precede the onset of some attacks, it could not be the true cause, but merely something akin to a trigger mechanism. He did, however, regard some pathological depressions as psychogenic in origin. While he did not completely clarify his views on their position in his classification, or how they were to be distinguished from manic-depressive illness with incidental stress, he appeared to regard them as a separate, but relatively small and unimportant, group.
At the same time as Kraepelin and others were establishing a generally accepted classification of the major psychiatric disorders in terms of disease entities based on a medical model and organic etiology, another growing school of European psychiatrists were developing a very different approach. These were the psychoanalysts. Freud and Abraham, in a perceptive group of studies, developed a theory of the origin of depression in relation to actual or symbolic losses of a love object. Here was a theory regarding the origin of most, if not all, depressions as psychogenic.
The case material of Kraepelin, and others like him, consisted of severely ill patients in institutions. The first depressed patients studied psychoanalytically were also severely ill. Subsequently, increasing attention began to be paid to milder forms of disorders, at first particularly by the psychoanalysts. Psychological theories of causation became more widely accepted for these disorders. A challenge now arose as to how to reconcile these theories with older ones of organic causation. Adolf .Meyer, a Swiss psychiatrist who became the highly influential head of the Henry Phipps Psychiatric Clinic at Johns Hopkins University, moved away from the idea of clearcut disease entities, and viewed all psychiatric disorders as reaction types, or psychobiological reactions of the organism to stress. 5 Both psychological and organic factors had to be taken into account. Others preferred to retain a view which kept separate the two types of psychiatric disorders. On one hand were the psychoses, severe illnesses requiring admission to an asylum, and presumed to have organic causes. On the other hand were the neuroses, milder and not requiring admission to an institution, regarded as more related to psychological stress, and amenable to psychological treatment. Hie stage was now set for two competing theories as to the classification of depression, which were to figure strongly in debates about subtypes in later years, and will be reviewed in due course.
The modern concept of depression
The modern concept of depression, as viewed by most psychiatrists and enshrined in the two official classifications, The ICD-10 Classification of Menial and Behavioral Disorders. Clinical descriptions and diagnostic guidelines (ICD 10) 6 and Diagnostic and Statistical Manual of Mental Disorders. 4th ed. (DSM-IV), 7 is essentially one of a clinical syndrome, defined by presence of a number of clinical features, but not requiring a specific etiology, and acknowledging the possibility of both psychological and biological causative factors in a somewhat Meyerian way. DSM-IV does exclude states where the symptoms are “better accounted for by bereavement,” an imprecise criterion, which is expanded by specifications of not persisting for longer than 2 months, or characterized by marked functional impairment, morbid preoccupation with worthless ness, suicidal ideation, psychotic symptoms, or psychomotor retardation. The value of this exclusion has been debated. 8 Evidence from symptom studies indicates considerable similarities to nonbereavement depression. Further studies arc still needed, particularly some which focus on the 2-month period which is crucial in the DSM-IV définition, and include investigations which ask if the picture of bereavement depressions in this period is different from other depressions, and whether they subside or continue outside this time.
This definition of depression is essentially syndromal and medical, resembling that of a syndrome in other fields of medicine. This implies a cluster of symptoms and signs which tend to occur together, which are assumed to reflect a common pathophysiology, that may not yet be understood, but may have diverse etiologies in different cases. Examples from internal medicine include the malabsorption syndrome, and congestive cardiac failure.
This is an aspect of the medical theory of diseases. In the medical concept each disease is regarded as having a specific, well defined etiology, pathology, clinical picture, and often a specific treatment. The advantages of being able to assign individuals to the correct disease have been great. Essentially, as pointed out many years ago by a philosopher, C. G. Hempcl, 9 they involve generalization of information. Once a patient is correctly diagnosed, much additional information is available regarding such aspects as underlying mechanisms, causation, prediction of outcome, and best treatment.
A syndrome at the level indicated above does not correspond fully to a disease, since multiple causes, and therefore separate diseases, may underlie it. In psychiatry, matters are more complex and often not clearcut. Different syndromes may overlap and co-occur. Defining pure diseases by etiology has generally not succeeded, since causes often appear to be multiple, even in the single case, and not all etiological factors arc known. Nevertheless, many of the above advantages do apply to syndromal diagnoses, including assignment of appropriate treatment and prediction of outcome. It is possible that, as genes involved in psychiatric disorders become elucidated, endophenotypes reflected in underlying disturbances, and genetically defined disorders, may come to correspond more closely to true diseases.
The classical method of identifying a disorder, for most of the history of psychiatry, was for the influential psychiatrist to discern and describe disorders based on his or her clinical experience, with little attempt at precise definition or method-based research. The main method of forming diagnoses in modern psychiatric nosology has been by committee agreement, based sometimes on quite limited empirical, research. Diagnostic criteria are then defined by listing certain symptoms, to define the number necessary for the diagnosis, with duration of time, other requirements, and exclusions. In DSM-IV, eight symptoms are listed as qualifying for major depression, with a requirement that at least five be present, including at least one of two core symptoms, together with duration of 2 weeks or more, presence of clinically significant distress or impairment of function, with absence respectively of mixed episode, direct effects of a drug of abuse, a medication or other substance, or of a general medical condition, or of bereavement, and for depressive disorder, of bipolar disorder or certain other psychotic diagnoses. For dysthymia, fewer symptoms are required, but for a longer period of 2 years, and from a shorter list of eligible symptoms.
For ICD-10 depressive episode, the definitions in the clinical criteria are not tightly specified, but they are well specified in the separate Research Criteria, where they tend to be more restrictive than in the clinical criteria. The Research Criteria are less used, and the existence of two different sets of criteria in the classification causes some obvious problems. Eligible symptoms for depressive episode are the same as in DSM-IV, with the addition of one further symptom, loss of confidence or selfesteem, with the number of symptoms required to be present depending on the severity of the episode, and a third symptom, fatigue, placed as eligible with the two other core symptoms, rather than in the additional list. There is an identical minimum length, of 2 weeks, and somewhat similar excluding criteria, but without specifying bereavement. The list of eligible symptoms for dysthymia is longer, with three required.
The core symptoms
The core symptoms of depression, of which at least one is required in DSM-IV, are depressed mood, and loss of interest or pleasure. The further eligible symptom in ICD-10 is decreased energy or fatigability, but, since two core symptoms must be present, in effect depressed mood or loss of interest/pleasure are required in this schema also. The reason for the addition of decreased energy to the core is not clear.
These core symptoms reflect the view that depressive disorder is essentially a disorder of mood or affect. Although textbooks have suggested variously that the word “affect” should refer to short-term states or states which are observable, and “mood” for sustained ones or internal ones, there has been much confusion regarding these two terms. 10 In practice they have long been used as more or less synonymous. The term “depression” came into use in the 19th century, originally as “mental depression,” to describe lowering of spirits, and came to replace melancholia as a diagnosis. The English-language word really uses an analogy, and its earlier, and also still valid, meaning relates to being pressed down, or an area of something which is pressed down. It can now also refer to other quite different phenomena of lowering, one economic and one barometric, as used in meteorology.
The modern word for loss of interest and pleasure, anhe donia, came into official English-speaking psychiatry with DSM-III. 3 The absence of pleasure, and occurrence of feelings of emptiness and flatness rather than overt sadness, have often been described as occurring in some depressions, particularly the more severe ones.
The assignment of mood lowering to ccntrality in the disorder is to some extent a Western concept, and a more recent one. Other aspects of the disorder such as behavioral change were seen as more important earlier. In other cultures, physical and other disturbances may be seen as more important, and may be reflected in the terms used to describe what appears to be the same disorder.
A more neurobiological view would regard all these phenomena as peripheral and subjective, and would regard the key disturbance as a neurobiological one, not yet adequately elucidated, which drives the other phenomena.
Additional symptoms
The additional symptoms which contribute towards a diagnosis of depression in the two official schemes are: appetite or weight loss or gain; insomnia or hypersomnia; agitation or retardation; loss of energy or fatigue (DSM-IV); loss of confidence or self-esteem (ICD-10); worthlessness or guilt; reduced concentration or indecisiveness; thoughts of suicide or suicide attempt. The wording and definitions are not always identical in the two schemes. Some of these symptoms are not specific, as they can be caused by other physical or mental disorders. .For some, such as pathological guilt, this is not the case, and the placing in the subsidiary list does seem to reflect more the view that the mood disturbance is central.
The diagnostic concept as reflected in the use of criteria which in essence count the number of symptoms present has not usually been formulated explicitly. What there appears to be is an assumption that as the disease becomes more severe it also becomes more pervasive, sucking in more of the accompanying symptoms and disturbances. There are not many empirical studies which have looked directly at the validity of this assumption. Studies using latent trait analyses have tended to produce a dimension corresponding to a list of core symptoms. 11 , 12 Clinically, as depression becomes more severe, it does also tend to involve the presence of more symptoms.
There have been some studies which have examined the frequency of symptoms present in diagnosed depressions. A classic study was that of Aaron Beck. 13 In an early phase of the work that led ultimately to the genesis of cognitive therapy, and more immediately to his wellknown Beck Depression Inventory, he and his colleagues tabulated the frequency of symptoms in a large sample of psychiatric patients. Dividing depressive symptoms into emotional, cognitive, motivational, physical and vegetative, and delusions, they showed that all increased with severity of depression present, and all except delusions were common with severe depression.
Classification
Depressive disorders have long been recognized as heterogeneous. Their subclassification has generated as much research, and as much heat, as any controversy in psychiatry.
The two official schemes are parallel, but not identical, and neither is entirely satisfactory. 14 DSM-IV is simpler. Its major categories are depressive disorders and bipolar disorders. Both have subcategories. Within depressive disorders (unipolar depression), the main concern of this paper, the major subcategories are major depressive disorder (itself divided into single episode and recurrent disorder), dysthymic disorder, and the catch-all required to make any official scheme comprehensive for all users, depressive disorder not otherwise specified. The most recent episode can be additionally specified by a set of severity/psychotic/remission specifiers; as chronic; with catatonic features; with melancholic features; with atypical features; with postpartum onset. There is also a further major category for other mood disorders, which include mood disorders due to general medical conditions and substance-induced mood disorder.
In ICD-10 the major categories are manic episode; bipolar affective disorder; depressive episode; recurrent depressive disorder; persistent mood (affective) disorders (dysthymia, cyclothymia); other mood (affective) disorders; unspecified mood (affective) disorder. The two major axes are really bipolar-unipolar, and course (single episode, recurrent, persistent). Within any depressive episode, single or recurrent, there are subcategories by severity (mild, moderate, severe without psychotic symptoms, with psychotic symptoms, in remission for recurrent disorders) and an additional specifier is available for somatic syndrome (melancholia).
DSM-III and ICD-10 represented quite major advances on their predecessors, DSM-II (rooted much more in psychoanalytic and Meyerian concepts of reaction types) and ICD-9, by their use of structured criteria and their use of modern concepts. Structured criteria were used particularly in DSM-III and successors. ICD-10 is ambiguous in this respect, with its two sets of criteria, the Research Criteria which are well defined, the clinical criteria which are not. Both classifications do also have disadvantages. 14 They are complex, in their fine categories. They are not identical, and, national susceptibilities aside, would be much better fused to a single classification, employing the advantages of each, without the disadvantages, sometimes different, that each has. The strong separation into single episode and recurrent is not justified by empirical research, and it is not useful as a major division: all disorders which become recurrent are single episode on the first occasion. The DSM definitions are better. The specification in DSM-III of depressions related to medical disorder and to substance use is not helpful, since there is little to show they differ from the rest of depressions in any major ways.
Bipolar and unipolar disorder
Much of the discussion about the nosology of affective disorder concerns various subtypes. Depression was for many years a fertile ground for classifiers. 15 , 16 Although much of the heat and pressure have subsided, the issues still complicate diagnostic schemes.
The best-accepted and best-substantiated distinction is the bipolar-unipolar one. This was not always so. As described above, Kraepelin viewed all affective disorders as manic-depressive. As late as ICD -9, published in 1978, the ICD did not clearly make the separation, although hidden within the subcategories of manic-depressive disorder (296) for readers of very small print, was a distinction between 296.1, manic-depressive, depressed, which was meant to be unipolar, and 296.3, manic-depressive, circular, depressed, which was meant to be bipolar. Most users of the classification did not realize this, so the distinction was in practice ver}' erratically recorded. The unipolar-bipolar distinction was incorporated into DSMIII when it was issued in 1980, and later into the ICD when ICD-10 was issued.
It was pathfinding work in the 1960s by Angst 17 and Penis 18 that established the value of the distinction. They had been influenced by descriptions by Karl Leonhard, a 20th-century German psychiatrist with a very 19th-century approach to nosology based on his mental hospital clinical experience, of monopolar and bipolar cycloid psychoses. 19
The bipolar-unipolar distinction is clcarcut by definition, depending on the occurrence of a manic episode. Usually it is also so in practice, although late first manic episodes lead to embarrassing changes of diagnosis, and it is hard to be sure of the nature of minor mood elevations, in some cases which are regarded as bipolar II disorder or cyclothymic disorder, or in some subjects with milder mood changes in community epidemiology studies. The status of single-episode mania is debated, but is accepted by most as indicating true bipolar disorder. Some would regard recurrent depression as related to bipolar disorder, but there is not good evidence that this is the case. TTttcrc are good validating features for the distinction. 20 , 21 Bipolar disorder is more familial, and there is much more evidence of bipolar disorder in first-degree relatives of bipolars than in relatives of unipolars, although about half the cases of affective disorder in the relatives of bipolars are nevertheless unipolar. There is also better evidence from twin studies that the familial elevation is genetic. Molecular genetic evidence of different genes could confirm the distinction, but this evidence is not yet clearcut. There is a different sex ratio in bipolar disorder, equal or nearly so, possibly a more equal social class distribution, and some association with milder cyclothymic disorder, although the full status of more recent work on cyclothymia still requires confirmation by validating studies. Treatment response differs, with a better response to maintenance lithium and possibly to anticonvulsants, although in unipolars the evidence is not yet adequate. More manic episodes occur on antidepressants. Bipolar disorder has an earlier onset than severe unipolar disorder, and tends to be more recurrent. Onsets in women are not uncommonly postpartum, particularly in the case of mania.
The present review mainly concerns unipolar depression. There have been a number of recent reports comparing bipolar and unipolar depressions. 22 - 24 In addition to the history features indicated above, bipolar depressions have variously been reported to show more of the following symptom features compared with unipolar: more retardation, hypersomnia, anxiety, mood lability, psychotic features (especially when the age is under 35); less evidence of sad mood, and various somatic complaints. However, often the pictures are indistinguishable.
Psychotic depression and melancholia/somatic syndrome
The greatest controversy of a previous era concerned a dualistic theory of depression, with a dichotomy between what was variously termed psychotic or endogenous depression on the one hand, and neurotic or reactive depression on the other. Starting in the later 1920s, and throughout the 1930s, fierce debates took place, particularly in British psychiatry, between those advancing a dualistic view and those taking a unitary stance, viewing all depressions as part of a single disorder, without any clear separation into subtypes. 15 The debate subsided with the greater preoccupations of World War II, and reappeared in the form of empirical studies using multivariate statistics in the 1960s. 16
Terminology was confused. The term “psychotic” refers to a severe disorder with delusions and hallucinations, “neurotic” to a milder disorder without these, and often with the connotation of a vulnerable personality. “Endogenous” and “reactive” refer in this context to absence or presence of life stress. The reason for the partial fusion is that, in the fully evolved concept, there were viewed as three aspects: (i) absence of life stress; (ii) presence of a clinical picture characterized by greater severity, sometimes delusions or hallucinations, diurnal variation with morning worsening, delayed insomnia with early-morning wakening, greater somatic disturbances such as loss of appetite and weight, psychomotor retardation or agitation; this was the so-called endogenous clinical picture, or what in the 1970s was termed endogenomorphic depression 25 ; (iii) a personality, associated with reactive or neurotic depression, which was stress-vulnerable or maladaptive.
Over time, the concept of psychotic depression has become separated from that of endogenous depression. Psychotic depression has retained a secure place in the official schemes, as a variant of severe depression. It is clearly definable, by presence of delusions (particularly if mood-congruent) or hallucinations and there is validating evidence, for instance in the better response of such depressions to electroconvulsive therapy (ECT) or antipsychotic drugs, than to antidepressants alone.
Endogenous depression and its opposite arc more problematic, both regarding classificatory status and terminology. There is evidence in support. The factor-analytic and cluster- analytic studies of the 1960s and 1970s in most cases found a dimension or group. 26 On detailed examination, this sometimes looks more like the psychotic element and sometimes the melancholic. However, neurotic depression did not emerge as clearly as a single group in these studies, and is heterogeneous. 26 Dexamethasone nonsuppression occurs predominantly in the endogenous group, and to some extent, so do other neuroendocrine abnormalities, such as blunting of growth hormone response to clonidinc and prolactin response to tryptophan. Regarding treatment, the best ECT response is associated with the presence of psychomotor retardation and depressive delusions, characteristic of psychotic depression. 27 The endogenous picture may be useful as a characteristic of depressions that respond better to antidepressants than placebo, but this is not clear. However, boundaries are weak, with mixed cases common, and distributions on factors do not show consistent and convincing bimodality which would indicate separation of disorders. The relationship to severity, the loose and confusing definitions, and the overlap between psychotic depression and melancholia bedevil the area.
Terminology has remained unsatisfactory. The term neurotic has dropped out of use, particularly in American psychiatry, where it was abandoned because of its diversity of meaning, 28 partly because of previous associations with psychoanalysis, and partly to avoid the emphasis placed earlier in the US on personality and charactero logical aspects. Dysthymia, a chronic disorder that would earlier have been regarded as one form of neurotic depression, is now viewed as a mood disorder. In a somewhat parallel way, the term cyclothymic personality has been replaced by cyclothymic disorder, a form of bipolar mood disorder. The term endogenous was abandoned in official schemes because it is really a symptom syndrome that we refer to these days. The term melancholia, used in DSM-III and its successors, is only nonloaded once its original meaning of black bile is forgotten. It seems preferable to the term somatic depression used in ICD-10, because it is easier to use in English, where it easily forms the adjective melancholic. The concept of somatic depression can also refer to something quite different, associated with somatic disease, or with somatization. Whether this classification will survive forthcoming revisions of the official schemes in the next few years remains to be seen, but it still figures extensively in research and the literature. A spirited case has recently been made for its retention. 29
The place of life stress in this distinction has changed considerably. In older views of endogenous and non endogenous depression, life stress had a central role. However, the distinction is now made on the basis of symptom pattern rather than causal factors. Studies 30 have shown that there is little relationship between measures of preceding life events and the presence of melancholic symptoms. Most depressions are preceded by some life stress, often not sufficient to fully account for the episode so that other factors are also involved. In three of our own studies, in two of which the symptom data and the life event data were collected by different interviewers, we found little relationship between symptom type and previous life events. 30 Other studies of patients with and without the symptom pattern have found little difference between the groups regarding the occurrence of stressful life events prior to onset. However, there may be some differences once depressions have become severe and recurrent. In a sample of depressed females, when a melancholic/psychotic score based on the presence and severity of biological and psychotic symptoms was used, then severe life events were significantly less frequent in the melancholic/psychotic group. 31 This significant difference emerged only when episodes other than the first were included. In another study, 32 in which the sample comprised highly recurrent dépressives, fewer life events were found in endogenous than nonendogenous Research Diagnostic Criteria subtypes, which depend on symptom features.
Severity and minor depression
The severity issue deserves further consideration. It is elevated to an important consideration in ICD-10. As an episode qualifier it is useful, since severity does carry implications for treatment, and severe depressions also tend to have worse outcome than do mild. It is not well recognized that, in practice, ICD-10 mild depressive episode is by no means minor, at least in the Research Criteria. The definitions for individual symptoms and the absence of some symptoms from the list means that subjects who fit these criteria usually have sufficient depression also to qualify as DSM-IV major depressives.
This raises another issue, the lower boundary to distinguish pathological depression from normal mood change. Although defined by the number of symptoms present, it is not in fact well-defined, since the thresholds for individual symptoms are not clear or easy to be sure about: when does lowering of mood, even if present every day, cross the threshold in severity to count as being present? The issue is not crucial in the clinic, but it has become important as psychiatric research has extended to the community, and to community epidemiology. Comparatively high rates of depression arc found in community prevalence studies. 33 It is not clear whether all these depressions share fully the qualities of depression presenting for medical or psychiatric treatment. Similar issues arise in the use of “symptomatic volunteers” for research.
There have been a number of studies examining boundaries of DSM major depression. 11 , 12 , 34 - 36 These do support the validity of summing the number of symptoms, although it is doubtful whether there is any true threshold rather than a somewhat arbitrary cutoff on a continuum, and one which as defined may be a little too high. In terms of treatment response, there is good evidence of a threshold, a little below major depression, at which superiority of tricyclic antidepressants to placebo first appears. 37 The threshold for response to serotonin reuptake inhibitors may be a little lower. 38
Dysthymia and subsyndromal depression
Dysthymia was introduced into official classifications in DSM-III, using a term which had been originated by the 19th-century French psychiatrist, Janet. The concept had previously been introduced for research in the predecessor of DSM-III, The Research Diagnostic Criteria, as Intermittent Depression. 39 Partly the use of the term was to avoid use of the term neurotic depression. It reflected the wider modern trend to view such chronic phenomena as primarily disorders of mood rather than of personality.
In the last 20 years dysthymia has proved a useful concept, delineating a form of mood disorder which can produce many problems and have an adverse impact on the life of the sufferer, and it has generated much research. 40 There is a high rate of comorbidity, particularly of anxiety disorders and substance abuse. The majority of dysthymics ultimately also develop an episode of major depression, and such episodes, so-called double depression, have a worse prognosis than pure major depression, both in respect of remission and of recurrence. There appears therefore to be continuity between dysthymia and major depression. The DSM-IV definition rules out an episode of major depression in the first 2 years, but the ICD-10 definition does not. In practice the differentiation of dysthymia from milder chronic major depression or from the residual symptoms with partial remission which frequently occur after major depression, 41 is difficult and may be artificial. There is evidence that dysthymia responds to antidepressants, 42 but controlled trials do not always distinguish uncomplicated dysthymia from superimposed major depression.
A further, milder chronic disorder which has been delineated, recurrent brief depression, 43 has not received general acceptance. Described as comprising frequent episodes of depressive symptoms sufficiently severe for major depression, but only lasting a few days, it does not appear to be very common in patients presenting for treatment, and has not been found to respond to antidepressants in the few studies which have been undertaken. There is also another DSM-IV diagnosis, minor depression, which is included in an appendix of the manual as a provisional category for research. Minor depression was included in the Research Diagnostic Criteria (RDC),but not in DSM-III. Both RDC and the possible criteria in DSM-IV refer to episodes of depression milder than major depression, rather than persistent dysthymia. Minor depressive episodes, excluding dysthymia, have been found to be more prevalent than major depression in an epidemiological study. 44
In recent years, there has been a growing literature regarding so-called subthreshold or subsyndromal depressions, which are common in the community and can cause considerable disability. 45 It is not always clear whether this is episodic or chronic, or residual after major depression and what its overlap is with dysthymia or other milder syndromes.
There may be a case for inclusion of one or more diagnoses equivalent to minor or subsyndromal depression in the official schemes in the future. This would be useful in primary care, and in postpartum depression, where much of the literature refers to mild depressions which are important because of their potential impact on the baby. On the other hand, minor depression as defined in the RDC occurred less commonly than might have been expected, perhaps because by the time the criteria were reached, most depressions also fitted another RDC subcategory which was not included in DSM-IV, probable (but not definite) major depression. More research in this area would be timely
Single depressive episode versus recurrent depression
The strong ICD-10 distinction between single depressive episode and recurrent depression is not useful. Its appearance in ICD-10 was rather unexpected, as it has not been used much in the past in affective disorder. Unfortunate]}', in the light of what we have learned in the last 15 years about the risk of recurrence of depression, the distinction is not helpful. If high proportions of people with their first depressive episodes have further episodes and are redefined later as recurrent, the distinction becomes of little value. There is not much to distinguish first depressive episodes from recurrences in other respects, except where the depression has become quite recurrent, when the role of life stress becomes less, response to treatment poorer, and risk of recurrence higher.
A step change has indeed occurred in conceptualization of depression in the last 30 years. In the 1960s and earlier 1970s, the disorder was seen as an episodic one, with complete remission and often without recurrence. Since then follow-up studies of hospitalized dépressives have shown that at least 60% will be readmitted over 16 years, and rates for recurrence of episodes any severity, not necessarily needing hospital admission, may be up to 90%. 46 We do not yet know if this is true for milder depressions outside hospital, and probably there are many single episodes at community level, linked to stress, which do not recur, but severe depression is undoubtedly a recurrent disorder. Moreover, since remission may be incomplete and partial, and mild and subsyndromal disorder are common in the community and may ultimately be followed by major episodes, it is now common to view depression as often a chronic disorder encompassing, and varying through, a spectrum or continuum.
Other subtypes
There are also some other subtypes. ICD-10 does not have them, nor do previous DSM versions. This is understandable in view of the need not to clutter official classifications with the evanescent. The problem is that clinicians do commonly recognize and use some of them, but have nowhere to record them. DSM-IV does include some which are not coded and seem to be viewed somewhat tentatively.
There are four subtypes among the specifiers in DSM-IV, in addition to those already considered and others related to course. The first is postpartum depression. This is potentially important; although the issue goes wider than mood disorder. 14 At present, researchers and others interested in postpartum disorders have a major problem: there is no official way of recording the disorder. Frequencies of treated disorder are unobtainable. Retrospective identification of subjects for follow-up and other studies is not possible from coded diagnostic records. ICD-10 has a category of mental disorders associated with the pucrperium (F53),but it can only be used if the criteria for disorders coded elsewhere are not met. There is also a qualifier, in the research criteria only, to indicate disorder associated with the puerperium, but as it is not in the clinical guidelines, few people know about it. DSM-IV does have a noncoded specifier for postpartum onset which can be applied to major depression, mania, mixed episode, or brief psychotic disorder, but it is limited to these disorders and the onset requirement, which is within 4 weeks of deliver}', is too short. Case register and other studies indicate a peak of onsets which goes on longer, up to 3 months. 47 What is needed is a specifier which can apply to any disorder, is coded, and applies to the onset in the first 3 postpartum months. Inclusion of this should be a high priority for the future. The second specifier is for seasonal depression. There is now a vast literature on seasonal affective disorder and its treatment. 48 It is time that it was included in official classifications.
A third specifier in DSM-IV is for atypical depression, defined in terms of increased sleep, increased appetite, and other symptoms. Here, the case for inclusion is less clearcut, and there are arguments in either direction. The concept originally came from William Sargent and colleagues at St Thomas' Hospital, London. The meaning of the term has fluctuated. The originators probably had in mind nonendogenous depression and later, depression with anxiety or anxiety disorder alone, rather than the more recent meaning of the term, which focuses on vegetative symptoms reversed from their usual directions in endogenous depression. 49 The concept has always been associated with response to monoamine oxidase inhibitors (MAOIs), but the evidence that atypical depression in its current meaning is associated with good MAOI response is mainly limited to one very influential US research group. Other evidence would point to anxious or phobic patients, but in general, selectivity appears to be weak, and there is evidence that MAOIs, in a high enough dose, are effective in quite a range of depressives. 50 In practice, it appears that clinicians, at least in Europe, do not use the term as much today as they did in the 1960s and 1970s, and its importance may be diminishing. So, too, is research in relation to it.
The fourth DSM-IV specifier is for the presence of catatonic features. This is idiosyncratic, and does not correspond to much in the earlier literature. Its meaning is not very clear, but much of the description seems to be that of psychomotor retardation. Retarded depression does have a considerable lineage, but has not proved a very useful classification and is not very stable between episodes. This subtype could be dropped without loss.
DSM-IV has some other provisional classifications in its appendix. Recurrent brief depression appeared and generated much excitement, but has not proven very useful and now receives less attention, so the case has not been made for its continuing inclusion. Mixed anxiety -depression is common, but can easily be handled by modern ideas of comorbidity and two diagnoses. Premenstrual dysphoric disorder is not purely depressive, and is beyond the scope of this review.
Transcultural aspects
The modern concept of depression, with emphasis on psychological feelings, is particularly Western, and to some extent a 20th-century development. Earlier Western concepts were less psychological. Some other cultures and languages place emphasis on other aspects. 51 It was thought at one time that mood disorders were less common in other cultures, for instance African, than in Western. In general this does not now appear to be the case, but to have been an artefact of previous Western psychiatrists failing to recognize the disorder in other cultural and linguistic groups. Mood disorders do appear to be universal, once they are sought by local psychiatrists who understand the culture, language, and metaphors used to express mood. Rates may differ to a lesser extent, but this is not clear, since it faces formidable problems in establishing equivalence of translated interview instruments and questionnaires.
Presentations may differ. In Zimbabwe, the language lacks a term directly equivalent to depression, and presentations are typically with somatic symptoms. 52 In Chinese subjects, presentations may also be more somatic, but there is evidence that with Western acculturation, this changes. 53 In Hong Kong, 54 lower rates of depression and higher rates of anxiety have been reported than in similar epidemiological studies from the US and other Western countries, suggesting some redirection of symptoms.
It is also possible that other syndromes limited to one or more cultures may be equivalents of depression. Equivalence to depression is difficult to prove in nonde pressed subjects. It would, however, be inappropriate to imply that these are any less valid than disorders seen in Western cultures. There may also be additional nonWestern subtypes which justify inclusion in international diagnostic schemes.
The concepts involved in depression are complex. They have evolved over the years, and often, as is common in psychiatry, the meanings have changed subtly in the process. The core elements, and workable definitions for the disorder and its boundaries, are now well established. Some aspects of classification remain problematic, but the separation of bipolar and unipolar disorder was a major advance. Depressions are the most common disorders in psychiatry, both for psychiatrists and for general practitioners, so that understanding of their elements is important.
Thank you for visiting nature.com. You are using a browser version with limited support for CSS. To obtain the best experience, we recommend you use a more up to date browser (or turn off compatibility mode in Internet Explorer). In the meantime, to ensure continued support, we are displaying the site without styles and JavaScript.
- View all journals
- Explore content
- About the journal
- Publish with us
- Sign up for alerts
- Expert Review
- Open access
- Published: 26 January 2023
The genetic basis of major depressive disorder
- Jonathan Flint ORCID: orcid.org/0000-0002-9427-4429 1
Molecular Psychiatry volume 28 , pages 2254–2265 ( 2023 ) Cite this article
29k Accesses
216 Altmetric
Metrics details
- Neuroscience
The genetic dissection of major depressive disorder (MDD) ranks as one of the success stories of psychiatric genetics, with genome-wide association studies (GWAS) identifying 178 genetic risk loci and proposing more than 200 candidate genes. However, the GWAS results derive from the analysis of cohorts in which most cases are diagnosed by minimal phenotyping, a method that has low specificity. I review data indicating that there is a large genetic component unique to MDD that remains inaccessible to minimal phenotyping strategies and that the majority of genetic risk loci identified with minimal phenotyping approaches are unlikely to be MDD risk loci. I show that inventive uses of biobank data, novel imputation methods, combined with more interviewer diagnosed cases, can identify loci that contribute to the episodic severe shifts of mood, and neurovegetative and cognitive changes that are central to MDD. Furthermore, new theories about the nature and causes of MDD, drawing upon advances in neuroscience and psychology, can provide handles on how best to interpret and exploit genetic mapping results.
Similar content being viewed by others
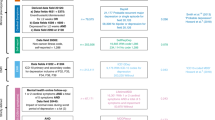
Minimal phenotyping yields genome-wide association signals of low specificity for major depression
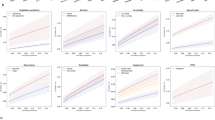
Genetic heterogeneity and subtypes of major depression
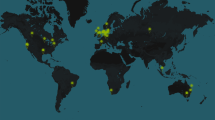
ENIGMA MDD: seven years of global neuroimaging studies of major depression through worldwide data sharing
Introduction.
In this review I consider what is known about the genetic basis of major depressive disorder (MDD), focusing on molecular genetic studies from 2015 onwards (predominantly genome-wide association studies (GWAS)). Previous reviews summarize earlier work [ 1 , 2 ] and cover the unproductive, and sometimes contentious, history of candidate gene studies, including conflicting claims over the presence of gene-by-environment interactions [ 3 ]. The entire field of psychiatric genetics has moved beyond the candidate-gene and candidate-gene-by-environment approach, recognizing that these previous approaches relied on the existence of common genetic variants with large effects, a hypothesis that has now been abandoned. In its place stand the results from a series of GWAS, of which those addressing the genetic basis of MDD are summarized in Table 1 .
Table 1 includes information on the number of cases and controls used by each GWAS, from which it can be see that success, defined in terms of number of loci identified, came with increases in sample size. There is an approximately linear relationship between the number of cases and the number of loci identified (illustrated in Fig. 1 ; for a discussion of the relationship between sample size and loci detected see [ 3 , 4 ]). In short, the larger sample sizes have delivered more genome wide significant risk loci.
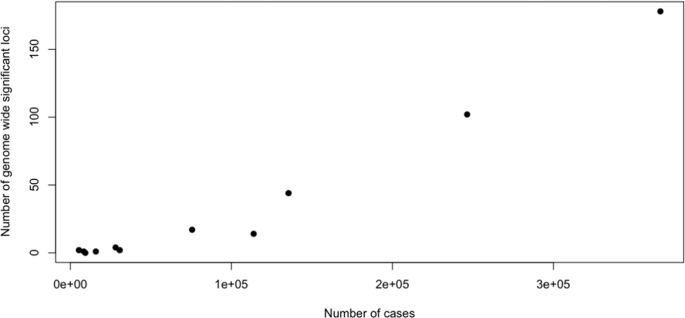
The relationship between the number of cases of MDD (plotted on the horizontal axis) to the number of genome-wide significant loci discovered (plotted on the vertical axis). Each dot represents the findings from a GWAS study.
The sample sizes are large, even by current standards: the most recent GWAS (from 2021) analyzed data from 1.2 million participants to identify 178 genetic risk loci and 223 independently significant single-nucleotide polymorphisms (SNPs) [ 5 ]. Recruiting cohorts on this scale was made possible by using simple and cheap methods to identify cases, methods described in more detail below and which I shall refer to as minimal phenotyping. Realizing that large samples were necessary to obtain robust statistical significance for genetic association, genetic researchers adopted minimal phenotyping strategies on the assumption that even if the phenotype were measured poorly, association would still be detectable for some of the loci contributing to the genetic risk of MDD. As hoped, hundreds of genome-wide significant loci have been found, but the loss of specificity consequent upon the use of minimal phenotyping had a penalty: a large proportion of the signal identified isn’t attributable to MDD, making it hard to use GWAS findings to understand the biology of MDD.
I will discuss below why the current state of MDD genetics is problematic by reviewing the nature of the phenotype that has been mapped, the nature of the loci that have been identified, how minimal phenotyping definitions relate to the gold standard definition of MDD (structured interview to elicit DSM criteria by a clinically experienced interviewer) as well as to other psychiatric conditions, and finally turn to consider ways forward to develop robust genetic analyses of the world’s leading cause of disability [ 6 ].
Most cases in GWAS of MDD have not been shown to meet criteria for MDD
As shown in Table 1 , before the 2016 GWAS report from the consumer genetics company 23&Me [ 7 ] almost all cases were required to meet DSM criteria (though not all were assessed by clinical interview, and different assessment schedules were applied, a complication that I return to later). Studies after 2016 include many cases recruited by methods that do not assess DSM or ICD criteria for MDD. For instance, out of 246,363 cases in one large GWAS from 2019 [ 8 ], 82% were recruited by self-report of depression:127,552 individuals from the UK Biobank who replied yes to the question ‘Have you ever seen a general practitioner for nerves, anxiety, tension or depression?’ or ‘Have you ever seen a psychiatrist for nerves, anxiety, tension or depression?’ and 75,607 cases all diagnosed by answering a single item: “Have you ever been diagnosed with clinical depression?” (answers: “Yes”, “No”, “I’m not sure”). The same study used a replication sample of 414,055 cases, all of which were recruited in this way [ 8 ]. Similarly, the most recent large-scale GWAS [ 5 ] recruited 340,591 cases of which 89% were defined as cases through a minimal phenotyping strategy that did not interrogate whether subjects met either DSM or ICD criteria.
How many of the cases recruited from minimal phenotyping do meet MDD criteria? We can estimate this from the literature on single item screening tests for MDD: from this we learn that more than half of the cases identified from a single item result are false positives [ 9 ]. Short, two-to-three item questionnaires, perform a little better, but only four out of 10 participants who score positive are depressed, and six out of 10 are false positives. It’s reasonable to assume that more than half of the cases in GWAS for MDD, recruited by these simple one or two item assessments, don’t have MDD.
GWAS cases are also recruited by asking about the presence of depressive symptoms, and by examination of electronic health records or deployment of online questionnaires seeking to detect whether a subject meets DMS or ICD criteria. These methods also perform poorly in detecting cases of MDD. For example, case definition in the Million Veteran Program in part used the two-item PHQ scale that asks about the presence of depressive symptoms in the past 2 weeks [ 5 ]. This, and similar assessments, assumes that depressive symptoms and MDD overlap. Do they? Detecting depressive symptoms, diagnosing MDD, and making a diagnosis of lifetime MDD are not the same things. A diagnosis of MDD requires 2 weeks of clinically significant dysphoria or anhedonia, along with a total of five symptoms. Lifetime MDD is diagnosed by asking about the occurrence of MDD at any point in a subject’s life. One way to see the difference between MDD and depressive symptoms is from their respective prevalences. While up to 20% of community-ascertained adults admit to experiencing depressive symptoms in the previous 6 months [ 10 ], the prevalence of MDD that satisfies DSM criteria (diagnosed from structured interviews) is between 2 and 4% [ 11 ]. The 12-month prevalence of MD in the US, similarly diagnosed, is 6.6% [ 12 ], and lifetime prevalence in the US is estimated to be 16.6% for DSM-IV [ 13 ]. The differences between the high prevalence of depressive symptoms from screening scales and the lower prevalence of depressive disorders indicates that there are many people who do not meet diagnostic criteria for MDD, but do have some form of subsyndromal disorder. The relationship of this condition (or conditions) to MDD is poorly understood, though we do know that subsyndromal depression is a strong predictor of the subsequent onset of MDD [ 14 ]. The inclusion of these people in GWAS of MDD contaminates case definition, but by how much we do not currently know. The consequences, though, are known: reduction in the specificity of the genetic signal, as discussed later.
Electronic health records are an alternative source of cases. Rigorous evaluation of their accuracy in detecting MDD cases is lacking. We know that ICD codes (the usual features extracted) have low specificity in the US, largely because clinicians may bill an ICD code for a diagnosis on clinical suspicion rather than for confirmation of disease [ 15 ]. Unsurprisingly, attempts to identify patients with MDD from electronic health records conclude that the data inadequately capture diagnoses [ 16 ]. We don’t have side-by-side comparison of EHR diagnoses and diagnoses obtained from a structured interview carried out by a clinically experienced interviewer (the gold standard), but using a primary care physician’s diagnosis as a comparator, ICD codes were found to have 77% sensitivity and 76% specificity [ 17 ], also supported by analysis of ICD codes from 5487 individuals [ 18 ].
Do more detailed self-assessments perform any better, as some claim [ 19 , 20 ]? The UK Biobank [ 21 ], the Australian Genetics of Depression Study [ 20 , 22 ] and the UK based Genetic Links to Anxiety and Depression Study [ 23 ] have all used a version of the CIDI-SF [ 24 ]. MDD assessed by the online CIDI-SF has higher heritability and captures more of the genetic signal that is specific to depression than briefer assessments [ 25 ], but we lack data comparing MDD diagnosed by gold-standard structured interview with the CIDI-SF (one conference report gives a validation of 81.8% for diagnosing recurrent MDD [ 26 ]). There is one report in the literature comparing MDD diagnosed by interviews and by a detailed self-assessment: the 20 item Centre for Epidemiological Studies Depression Scale (CES-D) [ 27 ]. About a third of cases with MDD were missed, and one third of those exceeding the CES-D threshold were diagnosed at interview with MDD [ 28 ]. In summary, longer self-assessments perform better than shorter ones, but we lack rigorous evaluation of their performance in large scale genetic studies. I turn to consider whether the low specificity matters, and argue that it does.
The majority of the genetic risk loci identified with minimal phenotyping approaches are unlikely to be MDD risk loci
It’s sometimes claimed that MDD cases identified by minimal phenotyping are just less severe forms of MDD, and thus share the same genetic loci [ 1 ]. That would be equivalent to lowering the threshold for disease liability in the population above which “cases” for MDD are defined. Under the liability-threshold model [ 29 ] lowering the threshold would not reduce heritability assessed by single-nucleotide polymorphisms (h 2 SNP ), yet h 2 SNP estimated for the minimal phenotyping definitions of MDD is less than that for the well-defined: three studies have estimated the heritability of severe recurrent depression to be about 25%, compared to <10% for symptom-based depression [ 5 , 25 , 30 ].
It can also be argued that GWAS of a poorly defined phenotype might not matter if it could be shown that the loci identified index a remitting and often relapsing history of episodes of disturbances of sleep and appetite, suicidality, guilty ruminations, anhedonia and low mood, in short, the features that clinicians would want to target for treatment. Minimal phenotyping approaches perform poorly in finding such loci. We know this from two analyses, using different strategies in different samples, that addressed the question of the specificity of genetic action in studies of MDD.
The first analysis applied a minimal phenotyping definition of MDD in 10,148 twin samples from three independent studies, and then estimated the fraction of genetic effects specific to lifetime MDD (as diagnosed by structured interviews by carefully trained mental health professionals [ 31 ]) that is captured by a less well-characterized case definition. The minimal phenotyping definition was more detailed than the single item assessments mentioned above, as it included self-administered questionnaires of current depressive symptoms and the personality trait neuroticism, both of which measure negative affect (central to the concept of MDD). Nevertheless, even this broad phenotype would miss around 65% of the risk loci for MD, including those specific to the syndrome [ 31 ]. Single item assessments, containing less information than the broad definition used here, likely index even less of the MDD-specific genetic risk.
A similar conclusion came from a second study which used SNP-based analysis of heritability (h 2 SNP ), comparing single-item, self-reported treatment seeking for depression with “Lifetime MDD”, defined using answers to a longer questionnaire (both the CIDI-SF and PHQ9) [ 25 ] that contained nearly all of the individual DSM criteria. Again, the majority of the heritability of the more strictly defined MDD is not shared with the lightly phenotyped measure [ 25 ]. The loss of signal unique to MDD is again likely underestimated, because “Lifetime MDD” did not come from a structured interview administered by a clinically trained interviewer, the gold-standard for MDD diagnosis.
The lack of specificity can be seen by comparing the loci mapped by minimal phenotyping with those mapped by other traits. Once we have identified risk loci from a minimal phenotyping definition of MDD, we can ask how many of them also increase the risk for more strictly defined MDD. The answer is shown in Fig. 2 . In the middle are the effects (plotted as odds ratios) for genome-wide significant loci found from a minimal phenotype definition (“GPpsy”) mapped in UK Biobank (data from [ 25 ]). On the left of the figure are the effects of the same loci on a “Lifetime MDD” definition. Consistent with the expectation that the same loci contribute to both traits, the effects at each locus are in the same direction and most are significant.
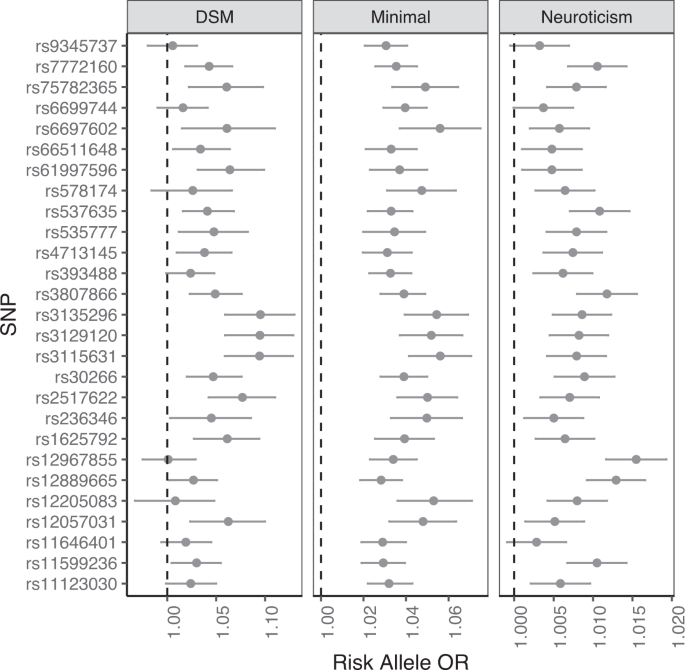
The figure shows 27 loci, listed on the vertical axis, that are significantly associated with a minimal-phenotyping definition of MDD in UKBiobank (GPpsy). The odds ratios (OR) are shown on the horizontal axis for the minimal phenotype, for a DSM-diagnosis of MDD and for the personality trait, neuroticism. The latter is a quantitative phenotype, so to allow comparison with the binary traits, the effect size estimates from the regression (beta values) have been converted into odds ratios. Data are from [ 25 ].
However, Fig. 2 also shows that the loci identified by mapping a minimal phenotyping definition MDD contribute to the personality trait neuroticism, plotted on the right of the diagram. In other words, the strategy has identified non-specific loci. Mapping minimal phenotyping MDD has identified loci shared with neuroticism, and not those that are specific to MDD. We could go ahead and characterize these loci, to identify the biology that they index, but if we did so and use the results to help us design better treatments for MDD, then we can expect those treatments also to affect the personality trait of neuroticism.
The results described above don’t mean that all loci mapped for the minimal phenotyping definition are non-specific. There will be some that also index the features of MDD we are interested in. But how can they be identified? In the absence of a well powered GWAS of MDD (diagnosed by interview) we can’t distinguish specific from non-specific genetic effects.
Genetic correlations between MDD definitions do not demonstrate that the definitions have the same biological basis
An appealing way to validate the use of minimal phenotyping definitions is to use SNP-based methods to demonstrate pleiotropy (i.e., that two traits have the same genetic basis). We could, for example, measure the genetic correlation (rG SNP ) between definitions of MDD and determine how much of the genetic effects are common to the different definitions. This strategy was used to compare data from seven cohorts that made up a GWAS of 135,458 MDD cases and 344,901 controls [ 32 ], and the genetic correlations were interpreted to support “the comparability of the seven cohorts (Supplementary Table 3), as the weighted mean rG SNP was 0.76 (s.e. = 0.03)”. [ 33 ]
There are three problems with this conclusion. First, the estimates are similar in magnitude to those between MDD and other phenotypes, particularly with other internalizing constructs. Table 2 shows the correlations between the seven cohorts, taken from [ 32 ]. Supplemental Table 13 of the same paper reports rG SNP with neuroticism of 0.7 (se 0.03) and 0.67 (se 0.04) with tiredness, both values larger in magnitude than some of the rG SNP estimates between the MDD cohorts. If we use rG SNP of the magnitude reported in Table 2 to justify the use of minimal phenotypes, we would have to admit measures of personality and tiredness to be equally valid measures of MDD. Second, the correlations depend on other features than just pleiotropy, making it hard to interpret a comparison of estimates between cohorts that have not been collected in the same way. Differences in ascertainment, in sex, and in age across cohorts alter the genetic architecture (this is discussed in the section on heterogeneity) [ 33 ]. Third, even if we accept the rG SNP values as correct, then the value of 0.76 [ 32 ] means 43% (calculated as 1 - 0.76 squared) of MD risk variants are not shared among cohorts, clearly a problematic level. Overall, these considerations imply that using rG SNP to make inferences about the biological relationships between MDD cohorts (and with other traits) may be confounded by other features, unrelated to the biology, which undermines the use of the measure to determine the biological similarity of the MDD definitions.
Polygenic risk score (PRS) can also be used to test the relationship between two phenotypes. A PRS sums the genetic effects estimated in one cohort to predict disease status in another. We can ask whether a PRS from the minimal phenotyping definitions does well at predicting MDD in more well-defined cases. The answer to that question is that it depends on the sample size: as sample size increases, PRS accuracy increases (see Fig. 2a in [ 32 ]). However, the issue is not just accuracy. What we want to know is whether the PRS from minimal phenotyping performs as well, or better than that from better defined MDD in predicting MDD meeting DSM criteria in cases. The short answer is that it does not. Once samples of the same size are used, then a PRS from a better defined MDD out-performs the minimal phenotyping PRS [ 25 ]. Putting this observation together with the analysis of non-specific effects above, then we can conclude that increasing the sample size will increase the ability to predict the mostly non-specific genetic components of MDD, although a modest proportion of genetic risk specific to MDD will also be well predicted.
What has been mapped?
Almost all GWAS have mapped a vulnerability to low mood or negative affect, a trait which is best termed dysphoria, to distinguish it from MDD. The genetic basis of dysphoria is in part shared with MDD, but (and this is the critical argument) there is a large genetic component unique to MDD, inaccessible to minimal phenotyping strategies. This includes the cyclic shifts of mood episodes and neurovegetative and cognitive changes central to MDD for which we lack adequate treatment.
If there are genetic effects unique to MDD, distinguishing it from dysphoria, is there any evidence that the genes involved, the biological pathways, are different? It’s too early to draw any definitive conclusions from the available data, not just because it is a hard task to conclusively find genes [ 34 ] but because we have so few results from GWAS for rigorously defined MDD. One study has identified and replicated two genome-wide significant loci in a sample of women with recurrent MDD, meeting diagnostic criteria as determined at structured interview [ 35 ]. While this is not enough to draw any conclusions, additional loci emerged from analysis of gene by environment interaction [ 36 ] and from analysis of rare variants identified from low-coverage sequence data [ 37 ]. Candidate genes identified from these separate analyses are enriched in mitochondrial function, supporting observations of increased amounts of mitochondrial DNA in cases [ 38 , 39 ].
By contrast, genes implicated by GWAS of dysphoria are enriched in neurodevelopmental functions. The two most recent GWAS [ 5 , 20 ] derive candidate gene lists based on the proximity of risk loci to genes (using a computational approach [ 40 ]), and on association with variation in transcript abundance [ 41 ]. The two candidate gene lists share 64 entries. Of the 64 genes present in both lists, twelve (almost 1 in five) contain zinc finger domains (ZNFs, ZSCANs and ZKSCANs). Zinc fingers recognize specific DNA sequences, with consequences that depend on other motifs in the protein, but typically involving the regulation of gene transcription, often in development. Although common, the 3% of genes in the human genome that contain them is far less than the almost 20% of genes in the dysphoria gene lists. Furthermore, the presence of three protocadherins (PCDHA1, PCDHA1 and PCDHA3) together with PAX6, supports the implication from the zinc finger genes of the role of developmental mechanisms, in particular involving neurons (a target of protocadherin function). Perhaps unsurprisingly the most significant functional category among the genes is “Nervous system development” [ 8 ].
More information is needed than just a DSM diagnosis
Given the difficulties of obtaining sufficient cases that meet diagnostic criteria for MDD, it might seem churlish to complain that isn’t enough for genetic studies. If GWAS studies recruited cases based on DSM criteria, a more standardized and reliable phenotype than self-assessments will be mapped, but one which may still have little or no relationship to any underlying biological entity. Indeed, DSM-5 is explicitly atheoretical, making no claim that the depression it describes reflects known neurobiological, or indeed any other, psychological process.
The dangers of concentrating solely on meeting DSM criteria have been recognized for some time: Hyman noted in 2007 “The problematic effects of diagnostic reification were revealed repeatedly in genetic studies, imaging studies, clinical trials, and types of studies where the rigid, operationalized criteria of the DSM-IV defined the goals of the investigation despite the fact that they appeared to be poor mirrors of nature” [ 42 ]. After a detailed review of the diagnostic features of MDD, Kendler points out that “meeting the DSM criteria for major depression is not the same thing as having major depression” [ 43 ], and that we are in danger of becoming “stymied by an excessive respect for our own creation” [ 43 ].
Another way to express this problem is as follows. As explained above, between 60 and 75% of the genetic risk for interview-based lifetime MDD is unique [ 31 ]. If we just map cases with DSM-diagnosed depression, obtained by gold-standard methods, we won’t be able to decide which of the loci we find are unique to MDD (in the sense described above). MDD arises more from environmental than from genetic roots, with a complex and poorly understood set of interactions between the two; the disorder is highly comorbid with other psychiatric disorders and with chronic disease; differences in personality, sex and age all contribute differentially to the risk of developing the illness [ 44 , 45 ]. That complexity has to be incorporated into genetic analysis if we are to adequately interpret GWAS results.
MDD is likely heterogeneous
A complication for the genetic analysis of MDD, and one that strongly indicates the need for us to collect more information than the diagnosis, is that multiple lines of evidence indicate the disorder is heterogeneous. Clinical features [ 46 , 47 , 48 , 49 , 50 ], comorbidities (~75% of patients with depression will meet criteria for at least one additional psychiatric disorder [ 51 ]), co-occurrence of diagnostic biomarkers [ 52 , 53 , 54 ], clustering subjects according to shared signatures of brain function [ 55 , 56 , 57 ] and treatment response [ 58 , 59 , 60 ], all point to this conclusion, although there is no agreement on, or conclusive demonstrations of, what the subtypes are [ 61 , 62 ]. There is a large literature on this question, including a recent comprehensive review of genetic heterogeneity [ 63 ]. I will focus here on issues relevant to interpreting GWAS findings.
First the genetic contributions to MDD subtypes are likely to differ. There is considerable empirical support for such a view. Most [ 64 , 65 , 66 ], but not all studies [ 67 ] find evidence for a higher heritability for major depression in women than in men, and also report that the genetic effects are not completely shared between the sexes. The largest study of the correlation in genetic effects, using 1.7 million pairs of monozygotic and dizygotic twins and full and half siblings [ 65 ], estimated the correlation to be 0.89 (95% CI = 0.87, 0.91), consistent with two earlier, smaller twin studies [ 68 , 69 ]. There is also evidence that cases ascertained through hospitals have a higher heritability than community acquired cases [ 70 ], that there is higher heritability for recurrent MDD compared to single episode illness, and for early onset compared to later onset [ 71 , 72 , 73 , 74 , 75 , 76 , 77 ]. Conversely, stratifying cases by clinical features, patterns of comorbidity, recurrence and age of onset, identifies differences in SNP-heritability, as was found in the UK Biobank, where genetic correlations between clinically defined subtypes ranged from 0.55 to 0.86 [ 78 ].
The presence of genetic heterogeneity has important consequences for interpreting GWAS studies. It means groups ascertained under different protocols will not share the same genetic risk loci, as demonstrated from the genetic correlations between 29 cohorts from the Psychiatric Genomics Consortium (PGC): rG SNP estimates varied from 0.52 to 1 (Supplementary Table 2 [ 32 ]). Genetic analysis carried out in ignorance of the presence of subtypes, as will happen with studies that use minimal phenotyping, enriches non-specific signals. Large sample sizes will eventually overcome sample heterogeneity [ 32 ], but at the cost of losing signal that is specific to the disease.
Ignoring subtypes can also introduce discrepancies between studies. As an example, a meta-analysis of 6561 cases found a significant inverse association between MDD and an obesity risk variant (in an intron of the FTO gene [ 79 ]; odds ratio = 0.92 (0.89, 0.97), P = 3.0E−04) [ 80 ]. An independent sample failed to replicate the association, except by stratifying on clinical features, when the locus was found to increase the risk of atypical MDD (odds ratio = 1.42-fold, P = 1.84E−04) [ 81 ] (the ‘atypical’ subtype was differentiated mainly by the direction of change in appetite, weight and sleep [ 82 ]). The sample sizes are relatively small and the delineation of subtypes incomplete so we cannot draw firm conclusions from this finding, but it is an indication of what will happen if subtypes are not considered.
To what extent can genetic analysis validate subtypes? There are conflicting claims that it can detect subtypes [ 78 ], and also that it cannot [ 83 , 84 ]. We can state with certainty that there is almost no evidence for the presence of experiments of nature, large mutations, that will cast light on the depression’s pathogenesis [ 2 ] (despite continuing hints that there are rare instances of single causes [ 85 ]) but there is much less certainty around what we can expect to be able to detect. The illustrative example here are attempts to stratify MDD by environmental risk. Given the size of the effect (more than half of the risk of developing depression is environmental [ 65 ]), stratifying by environmental risk should be a comparatively easy target. The fact that it is not, is itself instructive.
There’s an old distinction between ‘reactive’ depression, in which cases are caused by exposure to stressful life events, and ‘endogenous’ depression, in which no external cause can be found [ 86 , 87 , 88 ]. A putative precipitating event can be found for about half of MDD cases [ 89 , 90 ], suggesting that additional factors are necessary for the adverse life event to result in a depressive episode. Are there genetic differences between those exposed and those not exposed to life adversity? One report has identified different risk loci in the two groups [ 36 ] but, in general, attempts to detect such heterogeneity have yielded contradictory results.
Almost all studies addressing this question resort to the use of a polygenic risk score (PRS), which sums the effects estimated in one cohort to predict disease status in another. The first such analysis, in a small (1645 MDD cases) well phenotyped sample from the Netherlands, found that PRS have limited impact in predicting MDD risk in individuals with little exposure to childhood trauma, but a large impact in individuals with high exposure to childhood trauma [ 91 ]. A second study (of 1605 MDD cases, again well phenotyped) showed the opposite: cases who experienced more severe childhood trauma had a lower PRS than other cases or controls [ 92 ]; a third study, using 3024 MDD cases from nine cohorts of the PGC, found no evidence of any significant interaction [ 93 ]. A recent analysis of UK Biobank patients used a genomic relationship matrix to capture genetic relationships rather than the PRS, found that genome-by-trauma interaction accounts for greater variance in male than female individuals [ 94 ] (though note that this result applies to the dysphoria phenotype, not MDD). Alternative approaches to investigating the impact of the environment are now being developed [ 95 , 96 ] but robust replicated results are still lacking. The current literature is inconclusive, with no clearly replicable patterns emerging using current methods.
MDD heterogeneity is likely going to be very messy, due to environmental effects operating differently in different cohorts, with an altogether much richer and complex pattern of interactions, a degree of context dependency that we have not so far been able to measure. MDD may consist of many overlapping subtypes, that are only partly distinguishable based on clinical features, disease trajectory, risk factors, response to treatment and genetic risk factors. One instructive example where this possibility has been examined is inflammatory bowel disease in a model which supposes the existence of many environmental variables acting cumulatively over time on a backdrop of many genetic variants [ 97 ]. Testing whether MDD might similarly be best explained as a system-level perturbation of multiple, interacting factors, will require much larger, deeper datasets than are currently available.
Genetic relationships between MDD and other traits
Every GWAS since 2016 has used the genotypes to examine the relationship between what is claimed to be MDD (what I have termed dysphoria) and other disorders. I’ve already illustrated the use of genetic correlations and polygenic scores to examining the relationship between different definitions of MDD; the same methods have been applied to examine the relationship between MDD and other psychiatric disorders, and indeed many other traits and diseases. Table 3 summarizes recent findings, providing data on SNP-based estimates of genetic correlation (rG SNP ) and comparing them where possible to family based and twin-based estimates (rG-family and rG-twin) for four diseases and for the personality trait neuroticism (high neuroticism scores are robustly associated with an increased risk for MDD [ 98 , 99 , 100 ]).
One interpretation of the rG SNP findings in Table 3 is that they indicate the presence of pleiotropy, genetic loci that contribute to the risk of more than one disease, leading for example to the assertion that “genetically informed analyses may provide important ‘scaffolding’ to support restructuring of psychiatric nosology” [ 101 ]. The ease of generating rG SNP results, which require only GWAS summary statistics, has led to an explosion of findings: 669 phenotypes were significantly genetically correlated with dysphoria in the most recent GWAS [ 5 ]. Before accepting this conclusion, we need to assess whether there are alternatives to pleiotropy as explanations for the rG SNP. findings.
A review of the interpretation of rG SNP identified the following features that could bias estimates [ 102 ]: misclassification, assortative mating, population stratification, sample ascertainment (in particular ‘collider bias’ [ 103 ]) and inclusion of ‘super-normal controls’ [ 104 ]. All these probably affect the rG SNP reported in Table 3 , but I will focus here on three which likely make the largest contribution.
The first is mis-diagnosis. Cohorts are inevitably going to contain a proportion of misdiagnoses, as discussed in previous sections. Cross-contamination across two disorders inflates their apparent correlation, and cross-contamination of either with a third will alter the estimate, depending on the true genetic sharing between the third disorder and the two whose rG SNP we are trying to measure. This has already been shown for alcohol consumption [ 105 ] but, for reasons due to the source of MDD cases for GWAS, we lack similar data for MDD.
A second factor is how subjects were recruited into a study (ascertainment). There’s a tendency to assume that just because we deal with genetic data, a classic epidemiological problem of ascertainment can be ignored: after all, genotypes are fixed at conception so their relationship with the phenotypes must be causal. Unfortunately, completely artifactual genetic correlations can arise if two unrelated traits bias recruitment. In the UK Biobank study, enrolment implicitly selected participants having higher educational status and lower prevalence of smoking than the general population, and this introduces a bias in the estimated rG SNP between educational status and smoking [ 103 ]. The same biases will impact other rG SNP estimates, but we don’t know by how much. The choice of diagnostic protocols plays a role here, for example inflating estimates between depression and neuroticism. In the UK Biobank (and presumably in other cohorts) the diagnosis of depression came from a phenotyping strategy that enriches for neuroticism [ 25 ]. When rG SNP with neuroticism was estimated from a cohort with severe major depressive episodes (severe enough to warrant treatment with electroconvulsive therapy, often seen as treatment of last resort), then the rG SNP estimate fell to 0.42, a value consistent with that obtained from twin data (Table 3 ) [ 106 ].
Finally, cross-trait assortative mating over even a few generations will inflate estimates of rG SNP . Assortative mating refers to people choosing their partners because of something they have in common, such as height. Cross-trait assortative mating operates across multiple traits: we choose our partners not only because they are, roughly, similar heights as us, but also because we have other features in common, our likes and dislikes, our educational attainment and so on. Assortative mating induces rG SNP through gametic phase disequilibrium, resulting in positive correlations between independently inherited genetic risk factors [ 107 , 108 ]. Under conditions of random mating the number of risk alleles on one chromosome does not predict the number of risk alleles on a different chromosome, but they can predict this in the presence of assortative mating: the test for assortative mating can be carried out by asking whether risk alleles on odd-numbered chromosomes predicts the number of risk alleles on the even-numbered chromosomes. This test has been applied to explore genetic correlations between MDD and other phenotypes [ 109 ].
Cross-trait assortative mating has a surprisingly large impact on estimates of genetic correlation between MDD and psychiatric disorders, and can on its own be sufficient to account for many of the findings. Adding in the possibility of mis-diagnosis, after five generations of assortative mating and with a 5% bidirectional misdiagnosis (a very conservative estimate) most of the genetic correlation between depression and schizophrenia can be attributed to assortative mating (Fig. S11 of [ 109 ]).
In short, to use rG SNP findings to reveal shared genetic bases between MDD and other phenotypes we must consider misdiagnosis, ascertainment and cross-trait assortative mating, among other things. Currently no method does that. Consequently, at present we can’t use rG SNP estimates to make claims about the extent to which MDD shares biological roots with other traits and diseases.
Turning silver into gold
The poor quality of the phenotyping used in genetic studies of MDD goes largely unremarked. The problem is not just that the aggregate genetic signal in lightly phenotyped samples is substantially weaker, it’s that much of the signal is likely wrong [ 25 ]. Those unfamiliar with the difficulties of psychiatric diagnosis and of the literature on the reliability and interpretation of questionnaire-based assessments could be forgiven for believing claims that GWAS has revealed the position in the genome of hundreds of genetic risk variants to a disease that makes the single largest contribution to disability in the world [ 6 ]. They could also be forgiven for believing claims that the genetic data accumulated from MDD GWAS can be used to make inferences about genetic correlations between MDD and other phenotypes, and to derive genetic risk scores that can be used in out-of-sample prediction. I have argued here these claims are poorly supported by empirical data. In most cases, MDD case definition is so lax we really don’t know what has been mapped. For want of a better term, I’ve called it dysphoria, to distinguish it from MDD. The use of a poorly characterized phenotype, with low specificity for MDD, may mean that advances in MDD genetics turn out to be as poorly substantiated as the earlier claims for the role of candidate genes [ 110 ]. In this section I provide my opinion on how to ensure we take the discoveries we have, even if they are imperfect, and improve them, by turning silver into gold.
How can we recruit better cohorts for MDD genetics? One option is to deploy new technology to improve diagnosis. Computerized adaptive testing [ 111 , 112 ] and digital technologies both provide novel opportunities [ 113 ]. A computerized adaptive diagnostic test fixes the number of items administered and allows measurement uncertainty to vary. It’s faster than questionnaires and one for MDD obtained sensitivity of 95% and specificity of 87%, using an average of 4 items per participant (with a maximum of just 6 items) [ 114 ]. Computerized adaptive diagnostics could improve specificity over many of the existing self-assessments, but their performance compared to structured-interview DSM diagnosis for genetic research is unknown. There has also been progress in using digital phenotyping to infer mood and depression from data collected from phones [ 115 , 116 ] and assess current mood from voice and facial features [ 117 , 118 ], but the relevant literature consists largely of reviews and of methodologies [ 116 , 119 ], rather than transformative advances. There is some success, but nothing that would yet give us the equivalent of a diagnosis of lifetime MDD.
Another, simpler, option is to deploy better self-assessments, such as the CIDI-SF [ 20 , 21 , 22 , 23 ]. This approach will provide better diagnoses, but all self-assessments, however detailed, are to some extent flawed. Fried’s detailed review of MDD assessments argues that the processes involved when people self-score will influence depression measurement [ 120 ]. There is scant literature on this subject, but we know that self-assessments over-estimate the prevalence of depression [ 121 , 122 , 123 , 124 ], sometimes substantially (25% compared to 12% in a meta-analysis of individual participant data [ 124 ]).
In summary, relying on self-assessed MDD, even with multiple item questions, will likely always have relatively low specificity. As such, longer self-assessments cannot replace clinical interviews for MDD diagnosis in recruitment for GWAS studies. Simply increasing sample size using current online screening tools is not enough: we need also to increase the specificity of diagnosis. That raises three further issues: how big a sample of gold-standard cases do we need, who should we recruit, and what additional information should we collect?
We certainly won’t need to obtain hundreds of thousands of interview-based diagnoses. The very large numbers needed for genetic studies can be obtained by phenotypic imputation, a method in which we take a number of the deeply phenotyped subjects and use them to predict high quality MDD diagnoses, and other clinical features, in those for whom we have much less information. Phenotypic imputation has been successfully applied to several phenotypes [ 125 ] but its success depends on the quality of the observed data and the structure of missingness. We need a set of well phenotyped cases to seed imputation, but how to maximize imputation’s effectiveness remains an open question, so it’s not possible to provide robust estimates of the number of interview-based cases required. As an example of what is possible, imputation using data from a questionnaire-based measure of MDD from 67,164 UK biobank into 337,126 individuals with a single-item measure increased both the number of risk loci identified and out-of-sample prediction of MDD accuracy, while preserving better specificity to MDD than the single-item measure [ 126 ].
Who should we recruit? There are strong arguments to be made for the collection of samples of diverse ancestry, as laid out by Peterson and colleagues [ 127 ]. Given that almost 80% of participants in GWAS are of European descent [ 128 ], samples with greater ancestral diversity would help address health disparities in the use of genomic medicine [ 127 ], aid locus discovery and provide more generalizable polygenic risk scores. Sampling diverse populations is beginning, under an initiative from the US National Institute of Mental Health, so we can expect to have data soon that will address the current imbalance in ancestry.
There are also arguments to be made in favor of designing studies to collect specific groups of patients, focusing on one sex, on recurrent depression and hospital rather than community ascertainment. Analysis of between-cohort genetic heterogeneity using data from 29 independent component cohorts of the PGC-MDD demonstrated that cohort ascertainment (e.g., clinical versus community recruitment) in part explains heterogeneity in heritability estimates and genetic correlations [ 32 ]. Targeted recruitment would reduce heterogeneity and potentially increase relevant genetic signal, as shown in the CONVERGE cohort [ 35 ], where recruitment of women with recurrent MDD ascertained in hospitals (predicted to increase heritability and homogeneity), yielded a sample with heritability (h 2 snp ) of ~25% [ 37 ], compared to about 9% reported by the (predominantly European) PGC-MDD group [ 32 ].
What additional information should be collected? I’ve stressed the need for more cases diagnosed by clinical interview, and then asserted that collecting cases that meet diagnostic criteria isn’t enough, since meeting DSM criteria is no guarantee of identifying a biological relevant entity [ 42 , 43 ]. I’ve pointed out that genetic risk loci for DSM-diagnosed MDD consist of a mix of loci specific for the condition and those that are not. We still need to distinguish loci that are specific from those that are non-specific, and to do that we need more data than case status alone. What information should we collect, so as to avoid the problem of reification [ 129 ], and allow us to identify loci that are specific to MDD?
The Australian Genetics of Depression Study provides one example of a set of additional phenotypes that could be acquired [ 20 , 22 ]. These include comorbid disease (other psychiatric conditions, particularly anxiety disorders, [ 12 ] as well as medical disease [ 130 ]), environmental stressors, personality, family history, demographic data including work schedule, as well as the clinical course and treatment history for MDD. Among these features three deserve emphasis.
The major contributor to MDD risk is environmental, and without information about the environment it is hard to see how we can interpret genetic signals. A key unanswered question in MDD genetics is how best to obtain information about the relevant environment. Second, depression is a recurrent illness: up to 85% of cases in specialized mental health care and in primary care will experience recurrence; in the general population the rate is lower, but still high: up to 35% [ 131 ]. Despite its importance, understanding the factors that contribute to recurrence is an area yet to receive the attention of geneticists. Finally, the lack of deep symptomatic profiles is the most egregious omission in genetic studies of MDD. Central to MDD are episodic severe shifts of mood, together with neurovegetative and cognitive changes [ 43 , 132 , 133 ]. We need to document these unique features of MDD and to identify which loci contribute to their risk.
If it is a hard task to obtain thousands of interview-based, diagnoses, then it would appear even harder to collect the additional information. We can however improve the current data sets by taking advantage of the information accumulating in Biobanks. Many phenotypes in biobanks correlate with MDD, and these can be used as proxies for information we are missing. As a demonstration of this we analyzed the UK Biobank, taking the CIDI-SF based Lifetime MDD phenotype to represent a gold-standard assessment [ 126 ]. We then imputed Lifetime MDD in the entire cohort, using 216 other phenotypes in the biobank, chosen regardless of their putative relationship with MDD, using SoftImpute [ 134 ] (a variant of principal component analysis that accommodates missing data, and uses observed phenotype data to identify latent factors). We were able to show that the top phenome-wide factors capture pleiotropic axes for MDD, allowing us to identify genetic effects that are specific to lifetime MDD (which stood in for the gold standard MDD cases) [ 126 ]. Remarkably, we found that the one-item self-assessment measures (which capture general dysphoria), residualized of these latent factors, index core, MDD-specific biology. In short, we can dissect MDD into two components: shared pleiotropic factors and core factors. Both classes of derived phenotype are heritable, with the former defining a highly polygenic background of mental health and social factors, and the latter defining a less polygenic signature of core MDD biology. However, currently our imputation methods do not supply rich phenotypic data about specific symptom patterns, or features of the course of illness.
In discussing how to improve depression measurement, Fried pointed out “we cannot divorce our measures of depression from our theories about what depression is” [ 120 ]. It’s notable how few theories we have about the nature of depression. In part this might be because attempts to replace DSM criteria with neurobiological constructs (the NIH Research Domain Criteria RDoC) [ 135 ] have not progressed well. Despite the collection of relevant behavioral, genetic, and neuroimaging data, achieving transformative progress proved more difficult than expected [ 136 ]. In part it reflects the complexity of depression. In a review of risk factors Kendler identified 37 potential causes [ 45 ] (as he points out, not much less than the 44 identified by Richard Burton in 1621 [ 137 ]).
There are sources for new theories about the nature of depression, but these so far have not been exploited in genetic research. One comes from advances in neuroscience, that enable us to explore cellular and molecular mechanisms by deploying genetically encoded reagents and imaging technologies in animals. For example, investigation of how ketamine has its effect has shown that it reduces bursting in the lateral habenula, an effect isolated to one cell type (astrocytes) and indeed one channel in that cell type: a potassium channel, Kcnj10, that provides a molecular clue to the etiology of at least one form of MDD [ 138 , 139 ]. Human genetic studies have yet to determine whether risk loci act through this mechanism. Such a discovery could be transformative.
A second source of new theories of depression comes from psychology. Moving away from a somewhat stale debate about the values of categorial versus dimensional categorization, Borsboom proposed a network theoretical description of depression [ 140 , 141 ], arguing that the probability of a change from a normal to a depressed state is related to elevated temporal autocorrelation, variance, and correlation between emotions in fluctuations of autorecorded emotions [ 142 ]. Translating these concepts into genetically testable ideas is an important challenge to the field.
The diverse symptomatology, the way MDD is seen to arise from different starting environmental points, from childhood trauma through to adult-onset adversity, its comorbidity with many different chronic diseases, together with hints of multiple, diverse biological causal pathways, all support an etiological heterogeneity that is at odds with claims that its genetic basis is primarily pleiotropic and held in common with many other diseases. There are ways forward, as I have outlined, similar to those that propelled success in cancer research [ 143 ]. Understanding the origins of cancer progressed from careful clinical observation, for example by noticing the effects of folate deficiency on blood cells. For MDD we need new cohorts, more complex, deeper phenotypes, combined with the use of existing data sets, but most crucially we need ideas about the nature of the condition, so that we ask and answer the right clinical questions: what are the different forms of the disorder? What are the characteristics of each? And how can we best treat each form as we discover it and its causes?
McIntosh AM, Sullivan PF, Lewis CM. Uncovering the Genetic Architecture of Major Depression. Neuron. 2019;102:91–103.
Article CAS PubMed PubMed Central Google Scholar
Flint J, Kendler KS. The genetics of major depression. Neuron. 2014;81:484–503.
Park JH, Wacholder S, Gail MH, Peters U, Jacobs KB, Chanock SJ, et al. Estimation of effect size distribution from genome-wide association studies and implications for future discoveries. Nat Genet. 2010;42:570–5.
O’Connor LJ. The distribution of common-variant effect sizes. Nat Genet. 2021;53:1243–9.
Article PubMed Google Scholar
Levey DF, Stein MB, Wendt FR, Pathak GA, Zhou H, Aslan M, et al. Bi-ancestral depression GWAS in the Million Veteran Program and meta-analysis in >1.2 million individuals highlight new therapeutic directions. Nat Neurosci. 2021;24:954–63.
World-Health-Organization. Depression and Other Common Mental Disorders: Global Health Estimates. Geneva: World Health Organization; 2017.
Hyde CL, Nagle MW, Tian C, Chen X, Paciga SA, Wendland JR, et al. Identification of 15 genetic loci associated with risk of major depression in individuals of European descent. Nat Genet. 2016;48:1031–6.
Howard DM, Adams MJ, Clarke TK, Hafferty JD, Gibson J, Shirali M, et al. Genome-wide meta-analysis of depression identifies 102 independent variants and highlights the importance of the prefrontal brain regions. Nat Neurosci. 2019;22:343–52.
Mitchell AJ, Coyne JC. Do ultra-short screening instruments accurately detect depression in primary care? A pooled analysis and meta-analysis of 22 studies. Br J Gen Pract: J R Coll Gen Practitioners. 2007;57:144–51.
Google Scholar
Kessler RC, Avenevoli S, Ries Merikangas K. Mood disorders in children and adolescents: an epidemiologic perspective. Biol Psychiatry. 2001;49:1002–14.
Article CAS PubMed Google Scholar
Andrade L, Caraveo-Anduaga JJ, Berglund P, Bijl RV, De Graaf R, Vollebergh W, et al. The epidemiology of major depressive episodes: results from the International Consortium of Psychiatric Epidemiology (ICPE) Surveys. Int J Methods Psychiatr Res. 2003;12:3–21.
Kessler RC, Berglund P, Demler O, Jin R, Koretz D, Merikangas KR, et al. The epidemiology of major depressive disorder: results from the National Comorbidity Survey Replication (NCS-R). Jama. 2003;289:3095–105.
Kessler RC, Berglund P, Demler O, Jin R, Merikangas KR, Walters EE. Lifetime prevalence and age-of-onset distributions of DSM-IV disorders in the National Comorbidity Survey Replication. Arch Gen Psychiatry. 2005;62:593–602.
Angst J, Sellaro R, Merikangas KR. Depressive spectrum diagnoses. Compr Psychiatry. 2000;41:39–47.
Denny JC. Chapter 13: Mining electronic health records in the genomics era. PLoS Comput Biol. 2012;8:e1002823.
Madden JM, Lakoma MD, Rusinak D, Lu CY, Soumerai SB. Missing clinical and behavioral health data in a large electronic health record (EHR) system. J Am Med Inf Assoc. 2016;23:1143–9.
Article Google Scholar
Trinh NH, Youn SJ, Sousa J, Regan S, Bedoya CA, Chang TE, et al. Using electronic medical records to determine the diagnosis of clinical depression. Int J Med Inf. 2011;80:533–40.
Pena-Gralle APB, Talbot D, Trudel X, Aube K, Lesage A, Lauzier S, et al. Validation of case definitions of depression derived from administrative data against the CIDI-SF as reference standard: results from the PROspective Quebec (PROQ) study. BMC Psychiatry. 2021;21:491.
Article PubMed PubMed Central Google Scholar
Mitchell BL, Thorp JG, Wu Y, Campos AI, Nyholt DR, Gordon SD, et al. Polygenic Risk Scores Derived From Varying Definitions of Depression and Risk of Depression. JAMA Psychiatry. 2021;78:1152–60.
Mitchell BL, Campos AI, Whiteman DC, Olsen CM, Gordon SD, Walker AJ et al. The Australian Genetics of Depression Study: New Risk Loci and Dissecting Heterogeneity Between Subtypes. Biol Psychiatry. 2022;92:227–35.
Davis KAS, Coleman JRI, Adams M, Allen N, Breen G, Cullen B, et al. Mental health in UK Biobank: development, implementation and results from an online questionnaire completed by 157 366 participants. BJPsych Open. 2018;4:83–90.
Byrne EM, Kirk KM, Medland SE, McGrath JJ, Colodro-Conde L, Parker R, et al. Cohort profile: the Australian genetics of depression study. BMJ Open. 2020;10:e032580.
Davies MR, Kalsi G, Armour C, Jones IR, McIntosh AM, Smith DJ, et al. The Genetic Links to Anxiety and Depression (GLAD) Study: Online recruitment into the largest recontactable study of depression and anxiety. Behav Res Ther. 2019;123:103503.
Kessler RC, Ustun TB. The World Mental Health (WMH) Survey Initiative Version of the World Health Organization (WHO) Composite International Diagnostic Interview (CIDI). Int J Methods Psychiatr Res. 2004;13:93–121.
Cai N, Revez JA, Adams MJ, Andlauer TFM, Breen G, Byrne EM, et al. Minimal phenotyping yields genome-wide association signals of low specificity for major depression. Nat Genet. 2020;52:437–47.
Levinson D, Potash J, Mostafavi S, Battle A, Zhu X, Weissman M. T26 - Brief Assessment Of Major Depression For Genetic Studies: Validation Of Cidi-Sf Screening With Scid Interviews. Eur Neuropsychopharmacol. 2017;27:S448.
Radloff LS. The CES-D scale: a self-report depression scale for research in the general population. Appl Psychol Meas. 1977;1:385–401.
Boyd JH, Weissman MM, Thompson WD, Myers JK. Screening for Depression in a Community Sample - Understanding the Discrepancies between Depression Symptom and Diagnostic Scales. Arch Gen Psychiatry. 1982;39:1195–200.
Dempster ER, Lerner IM. Heritability of Threshold Characters. Genetics. 1950;35:212–36.
Giannakopoulou O, Lin K, Meng X, Su MH, Kuo PH, Peterson RE, et al. The Genetic Architecture of Depression in Individuals of East Asian Ancestry: A Genome-Wide Association Study. JAMA Psychiatry. 2021;78:1258–69.
Kendler KS, Gardner CO, Neale MC, Aggen S, Heath A, Colodro-Conde L et al. Shared and specific genetic risk factors for lifetime major depression, depressive symptoms and neuroticism in three population-based twin samples. Psychol Med. 2019;49:2745–53.
Wray NR, Ripke S, Mattheisen M, Trzaskowski M, Byrne EM, Abdellaoui A, et al. Genome-wide association analyses identify 44 risk variants and refine the genetic architecture of major depression. Nat Genet. 2018;50:668–81.
Trzaskowski M, Mehta D, Peyrot WJ, Hawkes D, Davies D, Howard DM, et al. Quantifying between-cohort and between-sex genetic heterogeneity in major depressive disorder. Am J Med Genet B Neuropsychiatr Genet. 2019;180:439–47.
Visscher PM, Brown MA, McCarthy MI, Yang J. Five years of GWAS discovery. Am J Hum Genet. 2012;90:7–24.
Converge Consortium. Sparse whole-genome sequencing identifies two loci for major depressive disorder. Nature. 2015;523:588–91.
Article PubMed Central Google Scholar
Peterson RE, Cai N, Dahl AW, Bigdeli TB, Edwards AC, Webb BT, et al. Molecular Genetic Analysis Subdivided by Adversity Exposure Suggests Etiologic Heterogeneity in Major Depression. Am J Psychiatry. 2018;175:545–54.
Peterson RE, Cai N, Bigdeli TB, Li Y, Reimers M, Nikulova A, et al. The Genetic Architecture of Major Depressive Disorder in Han Chinese Women. JAMA Psychiatry. 2017;74:162–8.
Cai N, Li Y, Chang S, Liang J, Lin C, Zhang X, et al. Genetic Control over mtDNA and Its Relationship to Major Depressive Disorder. Curr Biol. 2015;25:3170–7.
Cai N, Chang S, Li Y, Li Q, Hu J, Liang J, et al. Molecular signatures of major depression. Curr Biol. 2015;25:1146–56.
de Leeuw CA, Mooij JM, Heskes T, Posthuma D. MAGMA: generalized gene-set analysis of GWAS data. PLoS Comput Biol. 2015;11:e1004219.
Gusev A, Ko A, Shi H, Bhatia G, Chung W, Penninx BW, et al. Integrative approaches for large-scale transcriptome-wide association studies. Nat Genet. 2016;48:245–52.
Hyman SE. Can neuroscience be integrated into the DSM-V? Nat Rev Neurosci. 2007;8:725–32.
Kendler KS. The Phenomenology of Major Depression and the Representativeness and Nature of DSM Criteria. Am J Psychiatry. 2016;173:771–80.
Fried EI. Moving forward: how depression heterogeneity hinders progress in treatment and research. Expert Rev Neurother. 2017;17:423–5.
Kendler KS. From Many to One to Many-the Search for Causes of Psychiatric Illness. JAMA Psychiatry. 2019;76:1085–91.
Parker G, Fink M, Shorter E, Taylor MA, Akiskal H, Berrios G, et al. Issues for DSM-5: whither melancholia? The case for its classification as a distinct mood disorder. Am J Psychiatry. 2010;167:745–7.
Klein DN. Classification of depressive disorders in the DSM-V: proposal for a two-dimension system. J Abnorm Psychol. 2008;117:552–60.
Chen L, Eaton WW, Gallo JJ, Nestadt G. Understanding the heterogeneity of depression through the triad of symptoms, course and risk factors: a longitudinal, population-based study. J Affect Disord. 2000;59:1–11.
Ballard ED, Yarrington JS, Farmer CA, Lener MS, Kadriu B, Lally N, et al. Parsing the heterogeneity of depression: An exploratory factor analysis across commonly used depression rating scales. J Affect Disord. 2018;231:51–7.
Lux V, Kendler KS. Deconstructing major depression: a validation study of the DSM-IV symptomatic criteria. Psychol Med. 2010;40:1679–90.
Kessler RC, Nelson CB, McGonagle KA, Liu J, Swartz M, Blazer DG. Comorbidity of DSM-III-R major depressive disorder in the general population: results from the US National Comorbidity Survey. Br J Psychiatry. 1996;168:17–30.
Carroll BJ, Feinberg M, Greden JF, Tarika J, Albala AA, Haskett RF, et al. A specific laboratory test for the diagnosis of melancholia. Standardization, validation, and clinical utility. Arch Gen Psychiatry. 1981;38:15–22.
Gold PW, Chrousos GP. Organization of the stress system and its dysregulation in melancholic and atypical depression: high vs low CRH/NE states. Mol Psychiatry. 2002;7:254–75.
Lewy AJ, Sack RL, Miller LS, Hoban TM. Antidepressant and circadian phase-shifting effects of light. Science. 1987;235:352–4.
Drysdale AT, Grosenick L, Downar J, Dunlop K, Mansouri F, Meng Y, et al. Resting-state connectivity biomarkers define neurophysiological subtypes of depression. Nat Med. 2017;23:28–38.
Liston C, Chen AC, Zebley BD, Drysdale AT, Gordon R, Leuchter B, et al. Default mode network mechanisms of transcranial magnetic stimulation in depression. Biol Psychiatry. 2014;76:517–26.
Downar J, Geraci J, Salomons TV, Dunlop K, Wheeler S, McAndrews MP, et al. Anhedonia and reward-circuit connectivity distinguish nonresponders from responders to dorsomedial prefrontal repetitive transcranial magnetic stimulation in major depression. Biol Psychiatry. 2014;76:176–85.
McGrath CL, Kelley ME, Holtzheimer PE, Dunlop BW, Craighead WE, Franco AR, et al. Toward a neuroimaging treatment selection biomarker for major depressive disorder. JAMA Psychiatry. 2013;70:821–9.
Hieronymus F, Emilsson JF, Nilsson S, Eriksson E. Consistent superiority of selective serotonin reuptake inhibitors over placebo in reducing depressed mood in patients with major depression. Mol Psychiatry. 2016;21:523–30.
Chekroud AM, Gueorguieva R, Krumholz HM, Trivedi MH, Krystal JH, McCarthy G. Reevaluating the Efficacy and Predictability of Antidepressant Treatments A Symptom Clustering Approach. Jama Psychiatry. 2017;74:370–8.
Harald B, Gordon P. Meta-review of depressive subtyping models. J Affect Disord. 2012;139:126–40.
Beijers L, Wardenaar KJ, van Loo HM, Schoevers RA. Data-driven biological subtypes of depression: systematic review of biological approaches to depression subtyping. Mol Psychiatry. 2019;24:888–900.
Cai N, Choi KW, Fried EI. Reviewing the genetics of heterogeneity in depression: Operationalizations, manifestations, and etiologies. Hum Mol Genet. 2020;29:R10–R18.
Kendler KS, Neale MC, Kessler RC, Heath AC, Eaves LJ. A population-based twin study of major depression in women. The impact of varying definitions of illness. Arch Gen Psychiatry. 1992;49:257–66.
Kendler KS, Ohlsson H, Lichtenstein P, Sundquist J, Sundquist K. The Genetic Epidemiology of Treated Major Depression in Sweden. Am J Psychiatry. 2018;175:1137–44.
Fernandez-Pujals AM, Adams MJ, Thomson P, McKechanie AG, Blackwood DH, Smith BH, et al. Epidemiology and Heritability of Major Depressive Disorder, Stratified by Age of Onset, Sex, and Illness Course in Generation Scotland: Scottish Family Health Study (GS:SFHS). PLoS ONE. 2015;10:e0142197.
Sullivan PF, Neale MC, Kendler KS. Genetic epidemiology of major depression: review and meta-analysis. Am J Psychiatry. 2000;157:1552–62.
Kendler KS, Gardner CO, Neale MC, Prescott CA. Genetic risk factors for major depression in men and women: similar or different heritabilities and same or partly distinct genes? Psychol Med. 2001;31:605–16.
Kendler KS, Gatz M, Gardner CO, Pedersen NL. A Swedish national twin study of lifetime major depression. Am J Psychiatry. 2006;163:109–14.
McGuffin P, Katz R, Watkins S, Rutherford J. A hospital-based twin register of the heritability of DSM-IV unipolar depression. Arch Gen Psychiatry. 1996;53:129–36.
Kendler KS, Gardner CO, Prescott CA. Clinical characteristics of major depression that predict risk of depression in relatives. Arch Gen Psychiatry. 1999;56:322–7.
Kendler KS, Gatz M, Gardner CO, Pedersen NL. Age at onset and familial risk for major depression in a Swedish national twin sample. Psychol Med. 2005;35:1573–9.
Kendler KS, Gatz M, Gardner CO, Pedersen NL. Clinical indices of familial depression in the Swedish Twin Registry. Acta Psychiatr Scandinavica. 2007;115:214–20.
Article CAS Google Scholar
Kendler KS, Neale MC, Kessler RC, Heath AC, Eaves LJ. The clinical characteristics of major depression as indices of the familial risk to illness. Br J Psychiatry. 1994;165:66–72.
Ferentinos P, Rivera M, Ising M, Spain SL, Cohen-Woods S, Butler AW, et al. Investigating the genetic variation underlying episodicity in major depressive disorder: suggestive evidence for a bipolar contribution. J Affect Disord. 2014;155:81–9.
Ferentinos P, Koukounari A, Power R, Rivera M, Uher R, Craddock N, et al. Familiality and SNP heritability of age at onset and episodicity in major depressive disorder. Psychol Med. 2015;45:2215–25.
Power RA, Tansey KE, Buttenschon HN, Cohen-Woods S, Bigdeli T, Hall LS, et al. Genome-wide Association for Major Depression Through Age at Onset Stratification: Major Depressive Disorder Working Group of the Psychiatric Genomics Consortium. Biol Psychiatry. 2017;81:325–35.
Nguyen TD, Harder A, Xiong Y, Kowalec K, Hagg S, Cai N, et al. Genetic heterogeneity and subtypes of major depression. Mol Psychiatry. 2022;27:1667–75.
Frayling TM, Timpson NJ, Weedon MN, Zeggini E, Freathy RM, Lindgren CM, et al. A common variant in the FTO gene is associated with body mass index and predisposes to childhood and adult obesity. Science. 2007;316:889–94.
Samaan Z, Anand SS, Zhang X, Desai D, Rivera M, Pare G, et al. The protective effect of the obesity-associated rs9939609 A variant in fat mass- and obesity-associated gene on depression. Mol Psychiatry. 2013;18:1281–6.
Milaneschi Y, Lamers F, Mbarek H, Hottenga JJ, Boomsma DI, Penninx BW. The effect of FTO rs9939609 on major depression differs across MDD subtypes. Mol Psychiatry. 2014;19:960–2.
Lamers F, Vogelzangs N, Merikangas KR, de Jonge P, Beekman AT, Penninx BW. Evidence for a differential role of HPA-axis function, inflammation and metabolic syndrome in melancholic versus atypical depression. Mol Psychiatry. 2013;18:692–9.
Hall LS, Adams MJ, Arnau-Soler A, Clarke TK, Howard DM, Zeng Y, et al. Genome-wide meta-analyses of stratified depression in Generation Scotland and UK Biobank. Transl Psychiatry. 2018;8:9.
Howard DM, Folkersen L, Coleman JRI, Adams MJ, Glanville K, Werge T, et al. Genetic stratification of depression in UK Biobank. Transl Psychiatry. 2020;10:163.
Pan LA, Martin P, Zimmer T, Segreti AM, Kassiff S, McKain BW, et al. Neurometabolic Disorders: Potentially Treatable Abnormalities in Patients With Treatment-Refractory Depression and Suicidal Behavior. Am J Psychiatry. 2017;174:42–50.
Gillespie RD. The clinical differentiation of types of depression. Guy’s Hospital Rep. 1929;9:1109–14.
Kendell RE. The classification of depressions: a review of contemporary confusion. Br J Psychiatry. 1976;129:15–28.
Andreasen NC, Scheftner W, Reich T, Hirschfeld RM, Endicott J, Keller MB. The validation of the concept of endogenous depression. A family study approach. Arch Gen Psychiatry. 1986;43:246–51.
Kessler RC. The effects of stressful life events on depression. Annu Rev Psychol. 1997;48:191–214.
Mazure CM. Life stressors as risk factors in depression. Clin Psychol: Sci Pract. 1998;5:291–313.
Peyrot WJ, Milaneschi Y, Abdellaoui A, Sullivan PF, Hottenga JJ, Boomsma DI, et al. Effect of polygenic risk scores on depression in childhood trauma. Br J Psychiatry. 2014;205:113–9.
Mullins N, Power RA, Fisher HL, Hanscombe KB, Euesden J, Iniesta R, et al. Polygenic interactions with environmental adversity in the aetiology of major depressive disorder. Psychol Med. 2016;46:759–70.
Peyrot WJ, Van der Auwera S, Milaneschi Y, Dolan CV, Madden PAF, Sullivan PF, et al. Does Childhood Trauma Moderate Polygenic Risk for Depression? A Meta-analysis of 5765 Subjects From the Psychiatric Genomics Consortium. Biol Psychiatry. 2018;84:138–47.
Chuong M, Adams MJ, Kwong ASF, Haley CS, Amador C, McIntosh AM. Genome-by-Trauma Exposure Interactions in Adults With Depression in the UK Biobank. JAMA Psychiatry. 2022;79:1110–7.
Dahl A, Nguyen K, Cai N, Gandal MJ, Flint J, Zaitlen N. A Robust Method Uncovers Significant Context-Specific Heritability in Diverse Complex Traits. Am J Hum Genet. 2020;106:71–91.
Gillett AC, Jermy BS, Lee SH, Pain O, Howard DM, Hagenaars SP, et al. Exploring polygenic-environment and residual-environment interactions for depressive symptoms within the UK Biobank. Genet Epidemiol. 2022;46:219–33.
Graham DB, Xavier RJ. Pathway paradigms revealed from the genetics of inflammatory bowel disease. Nature. 2020;578:527–39.
Angst J, Clayton P. Premorbid personality of depressive, bipolar and schizophrenic patients with special reference to suicidal issues. Compr Psychiatry. 1986;27:511–32.
Hirschfeld RMA, Klerman GL, Lavori P, Keller MB, Griffith P, Coryell W. Premorbid personality assessments of first onset of major depression. Arch Gen Psychiatry. 1989;46:345–50.
Kendler KS, Neale MC, Kessler RC, Heath AC, Eaves LJ. A longitudinal twin study of personality and major depression in women. Arch Gen Psychiatry. 1993;50:853–62.
Brainstorm Consortium, Anttila V, Bulik-Sullivan B, Hilary KF, Raymond KW, Jose B, et al. Analysis of shared heritability in common disorders of the brain. Science. 2018;360:eaap8757.
van Rheenen W, Peyrot WJ, Schork AJ, Lee SH, Wray NR. Genetic correlations of polygenic disease traits: from theory to practice. Nat Rev Genet. 2019;20:567–81.
Munafo MR, Tilling K, Taylor AE, Evans DM, Davey, Smith G. Collider scope: when selection bias can substantially influence observed associations. Int J Epidemiol. 2018;47:226–35.
Kendler KS, Chatzinakos C, Bacanu SA. The impact on estimations of genetic correlations by the use of super-normal, unscreened, and family-history screened controls in genome wide case-control studies. Genet Epidemiol. 2020;44:283–9.
Xue A, Jiang L, Zhu Z, Wray NR, Visscher PM, Zeng J, et al. Genome-wide analyses of behavioural traits are subject to bias by misreports and longitudinal changes. Nat Commun. 2021;12:20211.
Clements CC, Karlsson R, Lu Y, Jureus A, Ruck C, Andersson E, et al. Genome-wide association study of patients with a severe major depressive episode treated with electroconvulsive therapy. Mol Psychiatry. 2021;26:2429–39.
Yengo L, Robinson MR, Keller MC, Kemper KE, Yang Y, Trzaskowski M, et al. Imprint of assortative mating on the human genome. Nat Hum Behav. 2018;2:948–54.
Border R, O’Rourke S, de Candia T, Goddard ME, Visscher PM, Yengo L et al. Assortative Mating Biases Marker-based Heritability Estimators. Nat Comm. 2022;13:660.
Border R, Athanasiadis G, Buil A, Schork AJ, Cai N, Young AI, et al. Cross-trait assortative mating is widespread and inflates genetic correlation estimates. Science. 2022;378:754–61.
Border R, Johnson EC, Evans LM, Smolen A, Berley N, Sullivan PF, et al. No Support for Historical Candidate Gene or Candidate Gene-by-Interaction Hypotheses for Major Depression Across Multiple Large Samples. Am J Psychiatry. 2019;176:376–87.
Gibbons RD, Weiss DJ, Kupfer DJ, Frank E, Fagiolini A, Grochocinski VJ, et al. Using computerized adaptive testing to reduce the burden of mental health assessment. Psychiatr Serv. 2008;59:361–8.
Gibbons RD, Weiss DJ, Frank E, Kupfer D. Computerized Adaptive Diagnosis and Testing of Mental Health Disorders. Annu Rev Clin Psychol. 2016;12:83–104.
Insel TR. Digital phenotyping: a global tool for psychiatry. World Psychiatry. 2018;17:276–7.
Gibbons RD, Hooker G, Finkelman MD, Weiss DJ, Pilkonis PA, Frank E, et al. The computerized adaptive diagnostic test for major depressive disorder (CAD-MDD): a screening tool for depression. J Clin Psychiatry. 2013;74:669–74.
Shah RV, Grennan G, Zafar-Khan M, Alim F, Dey S, Ramanathan D, et al. Personalized machine learning of depressed mood using wearables. Transl Psychiatry. 2021;11:338.
De Angel V, Lewis S, White K, Oetzmann C, Leightley D, Oprea E, et al. Digital health tools for the passive monitoring of depression: a systematic review of methods. NPJ Digit Med. 2022;5:3.
Rohani DA, Faurholt-Jepsen M, Kessing LV, Bardram JE. Correlations Between Objective Behavioral Features Collected From Mobile and Wearable Devices and Depressive Mood Symptoms in Patients With Affective Disorders: Systematic Review. Jmir Mhealth Uhealth. 2018;6:e165.
Low DM, Bentley KH, Ghosh SS. Automated assessment of psychiatric disorders using speech: A systematic review. Laryngoscope Investig. 2020;5:96–116.
Kamath J, Leon Barriera R, Jain N, Keisari E, Wang B. Digital phenotyping in depression diagnostics: Integrating psychiatric and engineering perspectives. World J Psychiatry. 2022;12:393–409.
Fried EI, Flake JK, Robinaugh DJ. Revisiting the theoretical and methodological foundations of depression measurement. Nat Rev Psychol. 2022;1:358–68.
von Glischinski M, von Brachel R, Thiele C, Hirschfeld G. Not sad enough for a depression trial? A systematic review of depression measures and cut points in clinical trial registrations. J Affect Disord. 2021;292:36–44.
Levis B, Benedetti A, Ioannidis JPA, Sun Y, Negeri Z, He C, et al. Patient Health Questionnaire-9 scores do not accurately estimate depression prevalence: individual participant data meta-analysis. J Clin Epidemiol. 2020;122:115–28.e111.
Levis B, Yan XW, He C, Sun Y, Benedetti A, Thombs BD. Comparison of depression prevalence estimates in meta-analyses based on screening tools and rating scales versus diagnostic interviews: a meta-research review. BMC Med. 2019;17:65.
Brehaut E, Neupane D, Levis B, Wu Y, Sun Y, Krishnan A, et al. Depression prevalence using the HADS-D compared to SCID major depression classification: An individual participant data meta-analysis. J Psychosom Res. 2020;139:110256.
Dahl A, Iotchkova V, Baud A, Johansson A, Gyllensten U, Soranzo N, et al. A multiple-phenotype imputation method for genetic studies. Nat Genet. 2016;48:466–72.
Dahl A, Thompson M, An U, Krebs M, Appadurai V, Border R et al. Phenotype integration improves power and preserves specificity in biobank-based genetic studies of MDD. bioRxiv 2022; https://doi.org/10.1101/2022.08.15.503980 .
Peterson RE, Kuchenbaecker K, Walters RK, Chen CY, Popejoy AB, Periyasamy S, et al. Genome-wide Association Studies in Ancestrally Diverse Populations: Opportunities, Methods, Pitfalls, and Recommendations. Cell. 2019;179:589–603.
Sirugo G, Williams SM, Tishkoff SA. The Missing Diversity in Human Genetic Studies. Cell. 2019;177:26–31.
Hyman SE. The diagnosis of mental disorders: the problem of reification. Annu Rev Clin Psychol. 2010;6:155–79.
Moussavi S, Chatterji S, Verdes E, Tandon A, Patel V, Ustun B. Depression, chronic diseases, and decrements in health: results from the World Health Surveys. Lancet. 2007;370:851–8.
Hardeveld F, Spijker J, De Graaf R, Nolen WA, Beekman AT. Prevalence and predictors of recurrence of major depressive disorder in the adult population. Acta Psychiatr Scand. 2010;122:184–91.
Kendler KS, Aggen SH, Flint J, Borsboom D, Fried EI. The centrality of DSM and non-DSM depressive symptoms in Han Chinese women with major depression. J Affect Disord. 2018;227:739–44.
Kendler KS. The genealogy of major depression: symptoms and signs of melancholia from 1880 to 1900. Mol Psychiatry. 2017;22:1539–53.
Mazumder R, Hastie T, Tibshirani R. Spectral Regularization Algorithms for Learning Large Incomplete Matrices. J Mach Learn Res. 2010;99:2287–322.
Sanislow CA, Pine DS, Quinn KJ, Kozak MJ, Garvey MA, Heinssen RK, et al. Developing constructs for psychopathology research: research domain criteria. J Abnorm Psychol. 2010;119:631–9.
Kupfer DJ, Regier DA. Neuroscience, clinical evidence, and the future of psychiatric classification in DSM-5. Am J Psychiatry. 2011;168:672–4.
Burton R. The Anatomy of Melancholy. What it is, With All the Kinds, Causes, Symptomes, Prognostickes, and Seuerall Cures of it. Oxford: John Lichfield and James Short for Henry Cripps; 1621.
Cui Y, Yang Y, Ni Z, Dong Y, Cai G, Foncelle A, et al. Astroglial Kir4.1 in the lateral habenula drives neuronal bursts in depression. Nature. 2018;554:323–7.
Yang Y, Cui Y, Sang K, Dong Y, Ni Z, Ma S, et al. Ketamine blocks bursting in the lateral habenula to rapidly relieve depression. Nature. 2018;554:317–22.
Borsboom D. Reflections on an emerging new science of mental disorders. Behav Res Ther. 2022;156:104127.
Robinaugh DJ, Hoekstra RHA, Toner ER, Borsboom D. The network approach to psychopathology: a review of the literature 2008-2018 and an agenda for future research. Psychol Med. 2020;50:353–66.
van de Leemput IA, Wichers M, Cramer AO, Borsboom D, Tuerlinckx F, Kuppens P, et al. Critical slowing down as early warning for the onset and termination of depression. Proc Natl Acad Sci USA. 2014;111:87–92.
Mukherjee S. The Emperor of All Maladies: A Biography of Cancer. New York: Simon & Schuster; 2010.
Sullivan PF, de Geus EJ, Willemsen G, James MR, Smit JH, Zandbelt T, et al. Genome-wide association for major depressive disorder: a possible role for the presynaptic protein piccolo. Mol Psychiatry. 2009;14:359–75.
Rietschel M, Mattheisen M, Frank J, Treutlein J, Degenhardt F, Breuer R, et al. Genome-wide association-, replication-, and neuroimaging study implicates HOMER1 in the etiology of major depression. Biol Psychiatry. 2010;68:578–85.
Muglia P, Tozzi F, Galwey NW, Francks C, Upmanyu R, Kong XQ, et al. Genome-wide association study of recurrent major depressive disorder in two European case-control cohorts. Mol Psychiatry. 2010;15:589–601.
Lewis CM, Ng MY, Butler AW, Cohen-Woods S, Uher R, Pirlo K, et al. Genome-wide association study of major recurrent depression in the U.K. population. Am J Psychiatry. 2010;167:949–57.
Shyn SI, Shi J, Kraft JB, Potash JB, Knowles JA, Weissman MM, et al. Novel loci for major depression identified by genome-wide association study of Sequenced Treatment Alternatives to Relieve Depression and meta-analysis of three studies. Mol Psychiatry. 2011;16:202–15.
Shi J, Potash JB, Knowles JA, Weissman MM, Coryell W, Scheftner WA, et al. Genome-wide association study of recurrent early-onset major depressive disorder. Mol Psychiatry. 2011;16:193–201.
Kohli MA, Lucae S, Saemann PG, Schmidt MV, Demirkan A, Hek K, et al. The neuronal transporter gene SLC6A15 confers risk to major depression. Neuron. 2011;70:252–65.
Wray NR, Pergadia ML, Blackwood DH, Penninx BW, Gordon SD, Nyholt DR, et al. Genome-wide association study of major depressive disorder: new results, meta-analysis, and lessons learned. Mol Psychiatry. 2012;17:36–48.
Ripke S, Wray NR, Lewis CM, Hamilton SP, Weissman MM, Breen G, et al. A mega-analysis of genome-wide association studies for major depressive disorder. Mol Psychiatry. 2013;18:497–511.
Howard DM, Adams MJ, Shirali M, Clarke TK, Marioni RE, Davies G, et al. Genome-wide association study of depression phenotypes in UK Biobank identifies variants in excitatory synaptic pathways. Nat Commun. 2018;9:1470.
McGuffin P, Rijsdijk F, Andrew M, Sham P, Katz R, Cardno A. The heritability of bipolar affective disorder and the genetic relationship to unipolar depression. Arch Gen Psychiatry. 2003;60:497–502.
Mullins N, Forstner AJ, O’Connell KS, Coombes B, Coleman JRI, Qiao Z, et al. Genome-wide association study of more than 40,000 bipolar disorder cases provides new insights into the underlying biology. Nat Genet. 2021;53:817–29.
Consortium C-DGotPG. Genomic Relationships, Novel Loci, and Pleiotropic Mechanisms across Eight Psychiatric Disorders. Cell. 2019;179:1469–82.e1411.
Kendler KS, Gatz M, Gardner CO, Pedersen NL. Personality and major depression: a Swedish longitudinal, population-based twin study. Arch Gen Psychiatry. 2006;63:1113–20.
Kendler KS, Myers J. The genetic and environmental relationship between major depression and the five-factor model of personality. Psychol Med. 2010;40:801–6.
Download references
Acknowledgements
This works was supported by NIH grant R01 MH122569. The review is based on discussions over Zoom with the following (in alphabetical order): Silviu-Alin Bacanu, Richard Border, Na Cai, Andy Dahl, Kenneth S Kendler, Morten Dybdahl Krebs, Joel Mefford, Elior Rahmani, Andrew Schork, Sriram Sankararaman, and Noah Zaitlen.
Author information
Authors and affiliations.
Department of Psychiatry and Biobehavioral Sciences, Billy and Audrey Wilder Endowed Chair in Psychiatry and Neuroscience, Center for Neurobehavioral Genetics, 695 Charles E. Young Drive South, 3357B Gonda, Box 951761, Los Angeles, CA, 90095-1761, USA
Jonathan Flint
You can also search for this author in PubMed Google Scholar
Contributions
All contributions were from a single author: JF devised, wrote, edited, and revised all aspects of the paper, including figures and tables.
Corresponding author
Correspondence to Jonathan Flint .
Ethics declarations
Competing interests.
The author declares no competing interests.
Additional information
Publisher’s note Springer Nature remains neutral with regard to jurisdictional claims in published maps and institutional affiliations.
Rights and permissions
Open Access This article is licensed under a Creative Commons Attribution 4.0 International License, which permits use, sharing, adaptation, distribution and reproduction in any medium or format, as long as you give appropriate credit to the original author(s) and the source, provide a link to the Creative Commons license, and indicate if changes were made. The images or other third party material in this article are included in the article’s Creative Commons license, unless indicated otherwise in a credit line to the material. If material is not included in the article’s Creative Commons license and your intended use is not permitted by statutory regulation or exceeds the permitted use, you will need to obtain permission directly from the copyright holder. To view a copy of this license, visit http://creativecommons.org/licenses/by/4.0/ .
Reprints and permissions
About this article
Cite this article.
Flint, J. The genetic basis of major depressive disorder. Mol Psychiatry 28 , 2254–2265 (2023). https://doi.org/10.1038/s41380-023-01957-9
Download citation
Received : 18 June 2022
Revised : 30 December 2022
Accepted : 11 January 2023
Published : 26 January 2023
Issue Date : June 2023
DOI : https://doi.org/10.1038/s41380-023-01957-9
Share this article
Anyone you share the following link with will be able to read this content:
Sorry, a shareable link is not currently available for this article.
Provided by the Springer Nature SharedIt content-sharing initiative
Quick links
- Explore articles by subject
- Guide to authors
- Editorial policies


IMAGES
VIDEO
COMMENTS
To gain an understanding of the similarities between stress-induced ‘reactive’ depression and a congenital ‘endogenous’ model of depression, we compared genes differentially regulated in response to early (MS) and late stress (UCMS) with mRNA differences between Flinders sensitive and resistant lines.
The findings showed that there are a plethora of risk and protective factors (relating to biological, psychological, and social determinants) that are related to depression; these determinants are interlinked and influence depression outcomes through a web of causation.
The main objective of this study was to compare the genomic signatures of ‘reactive’ and ‘endogenous’ models of depression in three rodent studies and translate these findings in a human study.
Our results suggest that 'endogenous' and 'reactive' subtypes of depression are associated with largely distinct changes in gene-expression. However, they also suggest that the molecular signature of 'reactive' depression caused by early stressors differs considerably from that of 'reactive' depress ….
The symptom element in endogenous depression currently survives in melancholia or somatic syndrome. Life stress is common in various depressive pictures. Dysthymia, a valuable diagnosis, represents a form of what was regarded earlier as neurotic depression. Other subtypes are also discussed.
The genetic dissection of major depressive disorder (MDD) ranks as one of the success stories of psychiatric genetics, with genome-wide association studies (GWAS) identifying 178 genetic risk...