Have a language expert improve your writing
Run a free plagiarism check in 10 minutes, generate accurate citations for free.
- Knowledge Base
Methodology
- What Is Qualitative Research? | Methods & Examples

What Is Qualitative Research? | Methods & Examples
Published on June 19, 2020 by Pritha Bhandari . Revised on June 22, 2023.
Qualitative research involves collecting and analyzing non-numerical data (e.g., text, video, or audio) to understand concepts, opinions, or experiences. It can be used to gather in-depth insights into a problem or generate new ideas for research.
Qualitative research is the opposite of quantitative research , which involves collecting and analyzing numerical data for statistical analysis.
Qualitative research is commonly used in the humanities and social sciences, in subjects such as anthropology, sociology, education, health sciences, history, etc.
- How does social media shape body image in teenagers?
- How do children and adults interpret healthy eating in the UK?
- What factors influence employee retention in a large organization?
- How is anxiety experienced around the world?
- How can teachers integrate social issues into science curriculums?
Table of contents
Approaches to qualitative research, qualitative research methods, qualitative data analysis, advantages of qualitative research, disadvantages of qualitative research, other interesting articles, frequently asked questions about qualitative research.
Qualitative research is used to understand how people experience the world. While there are many approaches to qualitative research, they tend to be flexible and focus on retaining rich meaning when interpreting data.
Common approaches include grounded theory, ethnography , action research , phenomenological research, and narrative research. They share some similarities, but emphasize different aims and perspectives.
Approach | What does it involve? |
---|---|
Grounded theory | Researchers collect rich data on a topic of interest and develop theories . |
Researchers immerse themselves in groups or organizations to understand their cultures. | |
Action research | Researchers and participants collaboratively link theory to practice to drive social change. |
Phenomenological research | Researchers investigate a phenomenon or event by describing and interpreting participants’ lived experiences. |
Narrative research | Researchers examine how stories are told to understand how participants perceive and make sense of their experiences. |
Note that qualitative research is at risk for certain research biases including the Hawthorne effect , observer bias , recall bias , and social desirability bias . While not always totally avoidable, awareness of potential biases as you collect and analyze your data can prevent them from impacting your work too much.
Here's why students love Scribbr's proofreading services
Discover proofreading & editing
Each of the research approaches involve using one or more data collection methods . These are some of the most common qualitative methods:
- Observations: recording what you have seen, heard, or encountered in detailed field notes.
- Interviews: personally asking people questions in one-on-one conversations.
- Focus groups: asking questions and generating discussion among a group of people.
- Surveys : distributing questionnaires with open-ended questions.
- Secondary research: collecting existing data in the form of texts, images, audio or video recordings, etc.
- You take field notes with observations and reflect on your own experiences of the company culture.
- You distribute open-ended surveys to employees across all the company’s offices by email to find out if the culture varies across locations.
- You conduct in-depth interviews with employees in your office to learn about their experiences and perspectives in greater detail.
Qualitative researchers often consider themselves “instruments” in research because all observations, interpretations and analyses are filtered through their own personal lens.
For this reason, when writing up your methodology for qualitative research, it’s important to reflect on your approach and to thoroughly explain the choices you made in collecting and analyzing the data.
Qualitative data can take the form of texts, photos, videos and audio. For example, you might be working with interview transcripts, survey responses, fieldnotes, or recordings from natural settings.
Most types of qualitative data analysis share the same five steps:
- Prepare and organize your data. This may mean transcribing interviews or typing up fieldnotes.
- Review and explore your data. Examine the data for patterns or repeated ideas that emerge.
- Develop a data coding system. Based on your initial ideas, establish a set of codes that you can apply to categorize your data.
- Assign codes to the data. For example, in qualitative survey analysis, this may mean going through each participant’s responses and tagging them with codes in a spreadsheet. As you go through your data, you can create new codes to add to your system if necessary.
- Identify recurring themes. Link codes together into cohesive, overarching themes.
There are several specific approaches to analyzing qualitative data. Although these methods share similar processes, they emphasize different concepts.
Approach | When to use | Example |
---|---|---|
To describe and categorize common words, phrases, and ideas in qualitative data. | A market researcher could perform content analysis to find out what kind of language is used in descriptions of therapeutic apps. | |
To identify and interpret patterns and themes in qualitative data. | A psychologist could apply thematic analysis to travel blogs to explore how tourism shapes self-identity. | |
To examine the content, structure, and design of texts. | A media researcher could use textual analysis to understand how news coverage of celebrities has changed in the past decade. | |
To study communication and how language is used to achieve effects in specific contexts. | A political scientist could use discourse analysis to study how politicians generate trust in election campaigns. |
Qualitative research often tries to preserve the voice and perspective of participants and can be adjusted as new research questions arise. Qualitative research is good for:
- Flexibility
The data collection and analysis process can be adapted as new ideas or patterns emerge. They are not rigidly decided beforehand.
- Natural settings
Data collection occurs in real-world contexts or in naturalistic ways.
- Meaningful insights
Detailed descriptions of people’s experiences, feelings and perceptions can be used in designing, testing or improving systems or products.
- Generation of new ideas
Open-ended responses mean that researchers can uncover novel problems or opportunities that they wouldn’t have thought of otherwise.
Researchers must consider practical and theoretical limitations in analyzing and interpreting their data. Qualitative research suffers from:
- Unreliability
The real-world setting often makes qualitative research unreliable because of uncontrolled factors that affect the data.
- Subjectivity
Due to the researcher’s primary role in analyzing and interpreting data, qualitative research cannot be replicated . The researcher decides what is important and what is irrelevant in data analysis, so interpretations of the same data can vary greatly.
- Limited generalizability
Small samples are often used to gather detailed data about specific contexts. Despite rigorous analysis procedures, it is difficult to draw generalizable conclusions because the data may be biased and unrepresentative of the wider population .
- Labor-intensive
Although software can be used to manage and record large amounts of text, data analysis often has to be checked or performed manually.
If you want to know more about statistics , methodology , or research bias , make sure to check out some of our other articles with explanations and examples.
- Chi square goodness of fit test
- Degrees of freedom
- Null hypothesis
- Discourse analysis
- Control groups
- Mixed methods research
- Non-probability sampling
- Quantitative research
- Inclusion and exclusion criteria
Research bias
- Rosenthal effect
- Implicit bias
- Cognitive bias
- Selection bias
- Negativity bias
- Status quo bias
Quantitative research deals with numbers and statistics, while qualitative research deals with words and meanings.
Quantitative methods allow you to systematically measure variables and test hypotheses . Qualitative methods allow you to explore concepts and experiences in more detail.
There are five common approaches to qualitative research :
- Grounded theory involves collecting data in order to develop new theories.
- Ethnography involves immersing yourself in a group or organization to understand its culture.
- Narrative research involves interpreting stories to understand how people make sense of their experiences and perceptions.
- Phenomenological research involves investigating phenomena through people’s lived experiences.
- Action research links theory and practice in several cycles to drive innovative changes.
Data collection is the systematic process by which observations or measurements are gathered in research. It is used in many different contexts by academics, governments, businesses, and other organizations.
There are various approaches to qualitative data analysis , but they all share five steps in common:
- Prepare and organize your data.
- Review and explore your data.
- Develop a data coding system.
- Assign codes to the data.
- Identify recurring themes.
The specifics of each step depend on the focus of the analysis. Some common approaches include textual analysis , thematic analysis , and discourse analysis .
Cite this Scribbr article
If you want to cite this source, you can copy and paste the citation or click the “Cite this Scribbr article” button to automatically add the citation to our free Citation Generator.
Bhandari, P. (2023, June 22). What Is Qualitative Research? | Methods & Examples. Scribbr. Retrieved September 6, 2024, from https://www.scribbr.com/methodology/qualitative-research/
Is this article helpful?
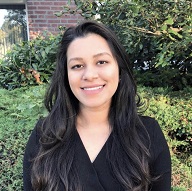
Pritha Bhandari
Other students also liked, qualitative vs. quantitative research | differences, examples & methods, how to do thematic analysis | step-by-step guide & examples, what is your plagiarism score.

An official website of the United States government
The .gov means it’s official. Federal government websites often end in .gov or .mil. Before sharing sensitive information, make sure you’re on a federal government site.
The site is secure. The https:// ensures that you are connecting to the official website and that any information you provide is encrypted and transmitted securely.
- Publications
- Account settings
Preview improvements coming to the PMC website in October 2024. Learn More or Try it out now .
- Advanced Search
- Journal List

What is Qualitative in Qualitative Research
Patrik aspers.
1 Department of Sociology, Uppsala University, Uppsala, Sweden
2 Seminar for Sociology, Universität St. Gallen, St. Gallen, Switzerland
3 Department of Media and Social Sciences, University of Stavanger, Stavanger, Norway
What is qualitative research? If we look for a precise definition of qualitative research, and specifically for one that addresses its distinctive feature of being “qualitative,” the literature is meager. In this article we systematically search, identify and analyze a sample of 89 sources using or attempting to define the term “qualitative.” Then, drawing on ideas we find scattered across existing work, and based on Becker’s classic study of marijuana consumption, we formulate and illustrate a definition that tries to capture its core elements. We define qualitative research as an iterative process in which improved understanding to the scientific community is achieved by making new significant distinctions resulting from getting closer to the phenomenon studied. This formulation is developed as a tool to help improve research designs while stressing that a qualitative dimension is present in quantitative work as well. Additionally, it can facilitate teaching, communication between researchers, diminish the gap between qualitative and quantitative researchers, help to address critiques of qualitative methods, and be used as a standard of evaluation of qualitative research.
If we assume that there is something called qualitative research, what exactly is this qualitative feature? And how could we evaluate qualitative research as good or not? Is it fundamentally different from quantitative research? In practice, most active qualitative researchers working with empirical material intuitively know what is involved in doing qualitative research, yet perhaps surprisingly, a clear definition addressing its key feature is still missing.
To address the question of what is qualitative we turn to the accounts of “qualitative research” in textbooks and also in empirical work. In his classic, explorative, interview study of deviance Howard Becker ( 1963 ) asks ‘How does one become a marijuana user?’ In contrast to pre-dispositional and psychological-individualistic theories of deviant behavior, Becker’s inherently social explanation contends that becoming a user of this substance is the result of a three-phase sequential learning process. First, potential users need to learn how to smoke it properly to produce the “correct” effects. If not, they are likely to stop experimenting with it. Second, they need to discover the effects associated with it; in other words, to get “high,” individuals not only have to experience what the drug does, but also to become aware that those sensations are related to using it. Third, they require learning to savor the feelings related to its consumption – to develop an acquired taste. Becker, who played music himself, gets close to the phenomenon by observing, taking part, and by talking to people consuming the drug: “half of the fifty interviews were conducted with musicians, the other half covered a wide range of people, including laborers, machinists, and people in the professions” (Becker 1963 :56).
Another central aspect derived through the common-to-all-research interplay between induction and deduction (Becker 2017 ), is that during the course of his research Becker adds scientifically meaningful new distinctions in the form of three phases—distinctions, or findings if you will, that strongly affect the course of his research: its focus, the material that he collects, and which eventually impact his findings. Each phase typically unfolds through social interaction, and often with input from experienced users in “a sequence of social experiences during which the person acquires a conception of the meaning of the behavior, and perceptions and judgments of objects and situations, all of which make the activity possible and desirable” (Becker 1963 :235). In this study the increased understanding of smoking dope is a result of a combination of the meaning of the actors, and the conceptual distinctions that Becker introduces based on the views expressed by his respondents. Understanding is the result of research and is due to an iterative process in which data, concepts and evidence are connected with one another (Becker 2017 ).
Indeed, there are many definitions of qualitative research, but if we look for a definition that addresses its distinctive feature of being “qualitative,” the literature across the broad field of social science is meager. The main reason behind this article lies in the paradox, which, to put it bluntly, is that researchers act as if they know what it is, but they cannot formulate a coherent definition. Sociologists and others will of course continue to conduct good studies that show the relevance and value of qualitative research addressing scientific and practical problems in society. However, our paper is grounded in the idea that providing a clear definition will help us improve the work that we do. Among researchers who practice qualitative research there is clearly much knowledge. We suggest that a definition makes this knowledge more explicit. If the first rationale for writing this paper refers to the “internal” aim of improving qualitative research, the second refers to the increased “external” pressure that especially many qualitative researchers feel; pressure that comes both from society as well as from other scientific approaches. There is a strong core in qualitative research, and leading researchers tend to agree on what it is and how it is done. Our critique is not directed at the practice of qualitative research, but we do claim that the type of systematic work we do has not yet been done, and that it is useful to improve the field and its status in relation to quantitative research.
The literature on the “internal” aim of improving, or at least clarifying qualitative research is large, and we do not claim to be the first to notice the vagueness of the term “qualitative” (Strauss and Corbin 1998 ). Also, others have noted that there is no single definition of it (Long and Godfrey 2004 :182), that there are many different views on qualitative research (Denzin and Lincoln 2003 :11; Jovanović 2011 :3), and that more generally, we need to define its meaning (Best 2004 :54). Strauss and Corbin ( 1998 ), for example, as well as Nelson et al. (1992:2 cited in Denzin and Lincoln 2003 :11), and Flick ( 2007 :ix–x), have recognized that the term is problematic: “Actually, the term ‘qualitative research’ is confusing because it can mean different things to different people” (Strauss and Corbin 1998 :10–11). Hammersley has discussed the possibility of addressing the problem, but states that “the task of providing an account of the distinctive features of qualitative research is far from straightforward” ( 2013 :2). This confusion, as he has recently further argued (Hammersley 2018 ), is also salient in relation to ethnography where different philosophical and methodological approaches lead to a lack of agreement about what it means.
Others (e.g. Hammersley 2018 ; Fine and Hancock 2017 ) have also identified the treat to qualitative research that comes from external forces, seen from the point of view of “qualitative research.” This threat can be further divided into that which comes from inside academia, such as the critique voiced by “quantitative research” and outside of academia, including, for example, New Public Management. Hammersley ( 2018 ), zooming in on one type of qualitative research, ethnography, has argued that it is under treat. Similarly to Fine ( 2003 ), and before him Gans ( 1999 ), he writes that ethnography’ has acquired a range of meanings, and comes in many different versions, these often reflecting sharply divergent epistemological orientations. And already more than twenty years ago while reviewing Denzin and Lincoln’ s Handbook of Qualitative Methods Fine argued:
While this increasing centrality [of qualitative research] might lead one to believe that consensual standards have developed, this belief would be misleading. As the methodology becomes more widely accepted, querulous challengers have raised fundamental questions that collectively have undercut the traditional models of how qualitative research is to be fashioned and presented (1995:417).
According to Hammersley, there are today “serious treats to the practice of ethnographic work, on almost any definition” ( 2018 :1). He lists five external treats: (1) that social research must be accountable and able to show its impact on society; (2) the current emphasis on “big data” and the emphasis on quantitative data and evidence; (3) the labor market pressure in academia that leaves less time for fieldwork (see also Fine and Hancock 2017 ); (4) problems of access to fields; and (5) the increased ethical scrutiny of projects, to which ethnography is particularly exposed. Hammersley discusses some more or less insufficient existing definitions of ethnography.
The current situation, as Hammersley and others note—and in relation not only to ethnography but also qualitative research in general, and as our empirical study shows—is not just unsatisfactory, it may even be harmful for the entire field of qualitative research, and does not help social science at large. We suggest that the lack of clarity of qualitative research is a real problem that must be addressed.
Towards a Definition of Qualitative Research
Seen in an historical light, what is today called qualitative, or sometimes ethnographic, interpretative research – or a number of other terms – has more or less always existed. At the time the founders of sociology – Simmel, Weber, Durkheim and, before them, Marx – were writing, and during the era of the Methodenstreit (“dispute about methods”) in which the German historical school emphasized scientific methods (cf. Swedberg 1990 ), we can at least speak of qualitative forerunners.
Perhaps the most extended discussion of what later became known as qualitative methods in a classic work is Bronisław Malinowski’s ( 1922 ) Argonauts in the Western Pacific , although even this study does not explicitly address the meaning of “qualitative.” In Weber’s ([1921–-22] 1978) work we find a tension between scientific explanations that are based on observation and quantification and interpretative research (see also Lazarsfeld and Barton 1982 ).
If we look through major sociology journals like the American Sociological Review , American Journal of Sociology , or Social Forces we will not find the term qualitative sociology before the 1970s. And certainly before then much of what we consider qualitative classics in sociology, like Becker’ study ( 1963 ), had already been produced. Indeed, the Chicago School often combined qualitative and quantitative data within the same study (Fine 1995 ). Our point being that before a disciplinary self-awareness the term quantitative preceded qualitative, and the articulation of the former was a political move to claim scientific status (Denzin and Lincoln 2005 ). In the US the World War II seem to have sparked a critique of sociological work, including “qualitative work,” that did not follow the scientific canon (Rawls 2018 ), which was underpinned by a scientifically oriented and value free philosophy of science. As a result the attempts and practice of integrating qualitative and quantitative sociology at Chicago lost ground to sociology that was more oriented to surveys and quantitative work at Columbia under Merton-Lazarsfeld. The quantitative tradition was also able to present textbooks (Lundberg 1951 ) that facilitated the use this approach and its “methods.” The practices of the qualitative tradition, by and large, remained tacit or was part of the mentoring transferred from the renowned masters to their students.
This glimpse into history leads us back to the lack of a coherent account condensed in a definition of qualitative research. Many of the attempts to define the term do not meet the requirements of a proper definition: A definition should be clear, avoid tautology, demarcate its domain in relation to the environment, and ideally only use words in its definiens that themselves are not in need of definition (Hempel 1966 ). A definition can enhance precision and thus clarity by identifying the core of the phenomenon. Preferably, a definition should be short. The typical definition we have found, however, is an ostensive definition, which indicates what qualitative research is about without informing us about what it actually is :
Qualitative research is multimethod in focus, involving an interpretative, naturalistic approach to its subject matter. This means that qualitative researchers study things in their natural settings, attempting to make sense of, or interpret, phenomena in terms of the meanings people bring to them. Qualitative research involves the studied use and collection of a variety of empirical materials – case study, personal experience, introspective, life story, interview, observational, historical, interactional, and visual texts – that describe routine and problematic moments and meanings in individuals’ lives. (Denzin and Lincoln 2005 :2)
Flick claims that the label “qualitative research” is indeed used as an umbrella for a number of approaches ( 2007 :2–4; 2002 :6), and it is not difficult to identify research fitting this designation. Moreover, whatever it is, it has grown dramatically over the past five decades. In addition, courses have been developed, methods have flourished, arguments about its future have been advanced (for example, Denzin and Lincoln 1994) and criticized (for example, Snow and Morrill 1995 ), and dedicated journals and books have mushroomed. Most social scientists have a clear idea of research and how it differs from journalism, politics and other activities. But the question of what is qualitative in qualitative research is either eluded or eschewed.
We maintain that this lacuna hinders systematic knowledge production based on qualitative research. Paul Lazarsfeld noted the lack of “codification” as early as 1955 when he reviewed 100 qualitative studies in order to offer a codification of the practices (Lazarsfeld and Barton 1982 :239). Since then many texts on “qualitative research” and its methods have been published, including recent attempts (Goertz and Mahoney 2012 ) similar to Lazarsfeld’s. These studies have tried to extract what is qualitative by looking at the large number of empirical “qualitative” studies. Our novel strategy complements these endeavors by taking another approach and looking at the attempts to codify these practices in the form of a definition, as well as to a minor extent take Becker’s study as an exemplar of what qualitative researchers actually do, and what the characteristic of being ‘qualitative’ denotes and implies. We claim that qualitative researchers, if there is such a thing as “qualitative research,” should be able to codify their practices in a condensed, yet general way expressed in language.
Lingering problems of “generalizability” and “how many cases do I need” (Small 2009 ) are blocking advancement – in this line of work qualitative approaches are said to differ considerably from quantitative ones, while some of the former unsuccessfully mimic principles related to the latter (Small 2009 ). Additionally, quantitative researchers sometimes unfairly criticize the first based on their own quality criteria. Scholars like Goertz and Mahoney ( 2012 ) have successfully focused on the different norms and practices beyond what they argue are essentially two different cultures: those working with either qualitative or quantitative methods. Instead, similarly to Becker ( 2017 ) who has recently questioned the usefulness of the distinction between qualitative and quantitative research, we focus on similarities.
The current situation also impedes both students and researchers in focusing their studies and understanding each other’s work (Lazarsfeld and Barton 1982 :239). A third consequence is providing an opening for critiques by scholars operating within different traditions (Valsiner 2000 :101). A fourth issue is that the “implicit use of methods in qualitative research makes the field far less standardized than the quantitative paradigm” (Goertz and Mahoney 2012 :9). Relatedly, the National Science Foundation in the US organized two workshops in 2004 and 2005 to address the scientific foundations of qualitative research involving strategies to improve it and to develop standards of evaluation in qualitative research. However, a specific focus on its distinguishing feature of being “qualitative” while being implicitly acknowledged, was discussed only briefly (for example, Best 2004 ).
In 2014 a theme issue was published in this journal on “Methods, Materials, and Meanings: Designing Cultural Analysis,” discussing central issues in (cultural) qualitative research (Berezin 2014 ; Biernacki 2014 ; Glaeser 2014 ; Lamont and Swidler 2014 ; Spillman 2014). We agree with many of the arguments put forward, such as the risk of methodological tribalism, and that we should not waste energy on debating methods separated from research questions. Nonetheless, a clarification of the relation to what is called “quantitative research” is of outmost importance to avoid misunderstandings and misguided debates between “qualitative” and “quantitative” researchers. Our strategy means that researchers, “qualitative” or “quantitative” they may be, in their actual practice may combine qualitative work and quantitative work.
In this article we accomplish three tasks. First, we systematically survey the literature for meanings of qualitative research by looking at how researchers have defined it. Drawing upon existing knowledge we find that the different meanings and ideas of qualitative research are not yet coherently integrated into one satisfactory definition. Next, we advance our contribution by offering a definition of qualitative research and illustrate its meaning and use partially by expanding on the brief example introduced earlier related to Becker’s work ( 1963 ). We offer a systematic analysis of central themes of what researchers consider to be the core of “qualitative,” regardless of style of work. These themes – which we summarize in terms of four keywords: distinction, process, closeness, improved understanding – constitute part of our literature review, in which each one appears, sometimes with others, but never all in the same definition. They serve as the foundation of our contribution. Our categories are overlapping. Their use is primarily to organize the large amount of definitions we have identified and analyzed, and not necessarily to draw a clear distinction between them. Finally, we continue the elaboration discussed above on the advantages of a clear definition of qualitative research.
In a hermeneutic fashion we propose that there is something meaningful that deserves to be labelled “qualitative research” (Gadamer 1990 ). To approach the question “What is qualitative in qualitative research?” we have surveyed the literature. In conducting our survey we first traced the word’s etymology in dictionaries, encyclopedias, handbooks of the social sciences and of methods and textbooks, mainly in English, which is common to methodology courses. It should be noted that we have zoomed in on sociology and its literature. This discipline has been the site of the largest debate and development of methods that can be called “qualitative,” which suggests that this field should be examined in great detail.
In an ideal situation we should expect that one good definition, or at least some common ideas, would have emerged over the years. This common core of qualitative research should be so accepted that it would appear in at least some textbooks. Since this is not what we found, we decided to pursue an inductive approach to capture maximal variation in the field of qualitative research; we searched in a selection of handbooks, textbooks, book chapters, and books, to which we added the analysis of journal articles. Our sample comprises a total of 89 references.
In practice we focused on the discipline that has had a clear discussion of methods, namely sociology. We also conducted a broad search in the JSTOR database to identify scholarly sociology articles published between 1998 and 2017 in English with a focus on defining or explaining qualitative research. We specifically zoom in on this time frame because we would have expect that this more mature period would have produced clear discussions on the meaning of qualitative research. To find these articles we combined a number of keywords to search the content and/or the title: qualitative (which was always included), definition, empirical, research, methodology, studies, fieldwork, interview and observation .
As a second phase of our research we searched within nine major sociological journals ( American Journal of Sociology , Sociological Theory , American Sociological Review , Contemporary Sociology , Sociological Forum , Sociological Theory , Qualitative Research , Qualitative Sociology and Qualitative Sociology Review ) for articles also published during the past 19 years (1998–2017) that had the term “qualitative” in the title and attempted to define qualitative research.
Lastly we picked two additional journals, Qualitative Research and Qualitative Sociology , in which we could expect to find texts addressing the notion of “qualitative.” From Qualitative Research we chose Volume 14, Issue 6, December 2014, and from Qualitative Sociology we chose Volume 36, Issue 2, June 2017. Within each of these we selected the first article; then we picked the second article of three prior issues. Again we went back another three issues and investigated article number three. Finally we went back another three issues and perused article number four. This selection criteria was used to get a manageable sample for the analysis.
The coding process of the 89 references we gathered in our selected review began soon after the first round of material was gathered, and we reduced the complexity created by our maximum variation sampling (Snow and Anderson 1993 :22) to four different categories within which questions on the nature and properties of qualitative research were discussed. We call them: Qualitative and Quantitative Research, Qualitative Research, Fieldwork, and Grounded Theory. This – which may appear as an illogical grouping – merely reflects the “context” in which the matter of “qualitative” is discussed. If the selection process of the material – books and articles – was informed by pre-knowledge, we used an inductive strategy to code the material. When studying our material, we identified four central notions related to “qualitative” that appear in various combinations in the literature which indicate what is the core of qualitative research. We have labeled them: “distinctions”, “process,” “closeness,” and “improved understanding.” During the research process the categories and notions were improved, refined, changed, and reordered. The coding ended when a sense of saturation in the material arose. In the presentation below all quotations and references come from our empirical material of texts on qualitative research.
Analysis – What is Qualitative Research?
In this section we describe the four categories we identified in the coding, how they differently discuss qualitative research, as well as their overall content. Some salient quotations are selected to represent the type of text sorted under each of the four categories. What we present are examples from the literature.
Qualitative and Quantitative
This analytic category comprises quotations comparing qualitative and quantitative research, a distinction that is frequently used (Brown 2010 :231); in effect this is a conceptual pair that structures the discussion and that may be associated with opposing interests. While the general goal of quantitative and qualitative research is the same – to understand the world better – their methodologies and focus in certain respects differ substantially (Becker 1966 :55). Quantity refers to that property of something that can be determined by measurement. In a dictionary of Statistics and Methodology we find that “(a) When referring to *variables, ‘qualitative’ is another term for *categorical or *nominal. (b) When speaking of kinds of research, ‘qualitative’ refers to studies of subjects that are hard to quantify, such as art history. Qualitative research tends to be a residual category for almost any kind of non-quantitative research” (Stiles 1998:183). But it should be obvious that one could employ a quantitative approach when studying, for example, art history.
The same dictionary states that quantitative is “said of variables or research that can be handled numerically, usually (too sharply) contrasted with *qualitative variables and research” (Stiles 1998:184). From a qualitative perspective “quantitative research” is about numbers and counting, and from a quantitative perspective qualitative research is everything that is not about numbers. But this does not say much about what is “qualitative.” If we turn to encyclopedias we find that in the 1932 edition of the Encyclopedia of the Social Sciences there is no mention of “qualitative.” In the Encyclopedia from 1968 we can read:
Qualitative Analysis. For methods of obtaining, analyzing, and describing data, see [the various entries:] CONTENT ANALYSIS; COUNTED DATA; EVALUATION RESEARCH, FIELD WORK; GRAPHIC PRESENTATION; HISTORIOGRAPHY, especially the article on THE RHETORIC OF HISTORY; INTERVIEWING; OBSERVATION; PERSONALITY MEASUREMENT; PROJECTIVE METHODS; PSYCHOANALYSIS, article on EXPERIMENTAL METHODS; SURVEY ANALYSIS, TABULAR PRESENTATION; TYPOLOGIES. (Vol. 13:225)
Some, like Alford, divide researchers into methodologists or, in his words, “quantitative and qualitative specialists” (Alford 1998 :12). Qualitative research uses a variety of methods, such as intensive interviews or in-depth analysis of historical materials, and it is concerned with a comprehensive account of some event or unit (King et al. 1994 :4). Like quantitative research it can be utilized to study a variety of issues, but it tends to focus on meanings and motivations that underlie cultural symbols, personal experiences, phenomena and detailed understanding of processes in the social world. In short, qualitative research centers on understanding processes, experiences, and the meanings people assign to things (Kalof et al. 2008 :79).
Others simply say that qualitative methods are inherently unscientific (Jovanović 2011 :19). Hood, for instance, argues that words are intrinsically less precise than numbers, and that they are therefore more prone to subjective analysis, leading to biased results (Hood 2006 :219). Qualitative methodologies have raised concerns over the limitations of quantitative templates (Brady et al. 2004 :4). Scholars such as King et al. ( 1994 ), for instance, argue that non-statistical research can produce more reliable results if researchers pay attention to the rules of scientific inference commonly stated in quantitative research. Also, researchers such as Becker ( 1966 :59; 1970 :42–43) have asserted that, if conducted properly, qualitative research and in particular ethnographic field methods, can lead to more accurate results than quantitative studies, in particular, survey research and laboratory experiments.
Some researchers, such as Kalof, Dan, and Dietz ( 2008 :79) claim that the boundaries between the two approaches are becoming blurred, and Small ( 2009 ) argues that currently much qualitative research (especially in North America) tries unsuccessfully and unnecessarily to emulate quantitative standards. For others, qualitative research tends to be more humanistic and discursive (King et al. 1994 :4). Ragin ( 1994 ), and similarly also Becker, ( 1996 :53), Marchel and Owens ( 2007 :303) think that the main distinction between the two styles is overstated and does not rest on the simple dichotomy of “numbers versus words” (Ragin 1994 :xii). Some claim that quantitative data can be utilized to discover associations, but in order to unveil cause and effect a complex research design involving the use of qualitative approaches needs to be devised (Gilbert 2009 :35). Consequently, qualitative data are useful for understanding the nuances lying beyond those processes as they unfold (Gilbert 2009 :35). Others contend that qualitative research is particularly well suited both to identify causality and to uncover fine descriptive distinctions (Fine and Hallett 2014 ; Lichterman and Isaac Reed 2014 ; Katz 2015 ).
There are other ways to separate these two traditions, including normative statements about what qualitative research should be (that is, better or worse than quantitative approaches, concerned with scientific approaches to societal change or vice versa; Snow and Morrill 1995 ; Denzin and Lincoln 2005 ), or whether it should develop falsifiable statements; Best 2004 ).
We propose that quantitative research is largely concerned with pre-determined variables (Small 2008 ); the analysis concerns the relations between variables. These categories are primarily not questioned in the study, only their frequency or degree, or the correlations between them (cf. Franzosi 2016 ). If a researcher studies wage differences between women and men, he or she works with given categories: x number of men are compared with y number of women, with a certain wage attributed to each person. The idea is not to move beyond the given categories of wage, men and women; they are the starting point as well as the end point, and undergo no “qualitative change.” Qualitative research, in contrast, investigates relations between categories that are themselves subject to change in the research process. Returning to Becker’s study ( 1963 ), we see that he questioned pre-dispositional theories of deviant behavior working with pre-determined variables such as an individual’s combination of personal qualities or emotional problems. His take, in contrast, was to understand marijuana consumption by developing “variables” as part of the investigation. Thereby he presented new variables, or as we would say today, theoretical concepts, but which are grounded in the empirical material.
Qualitative Research
This category contains quotations that refer to descriptions of qualitative research without making comparisons with quantitative research. Researchers such as Denzin and Lincoln, who have written a series of influential handbooks on qualitative methods (1994; Denzin and Lincoln 2003 ; 2005 ), citing Nelson et al. (1992:4), argue that because qualitative research is “interdisciplinary, transdisciplinary, and sometimes counterdisciplinary” it is difficult to derive one single definition of it (Jovanović 2011 :3). According to them, in fact, “the field” is “many things at the same time,” involving contradictions, tensions over its focus, methods, and how to derive interpretations and findings ( 2003 : 11). Similarly, others, such as Flick ( 2007 :ix–x) contend that agreeing on an accepted definition has increasingly become problematic, and that qualitative research has possibly matured different identities. However, Best holds that “the proliferation of many sorts of activities under the label of qualitative sociology threatens to confuse our discussions” ( 2004 :54). Atkinson’s position is more definite: “the current state of qualitative research and research methods is confused” ( 2005 :3–4).
Qualitative research is about interpretation (Blumer 1969 ; Strauss and Corbin 1998 ; Denzin and Lincoln 2003 ), or Verstehen [understanding] (Frankfort-Nachmias and Nachmias 1996 ). It is “multi-method,” involving the collection and use of a variety of empirical materials (Denzin and Lincoln 1998; Silverman 2013 ) and approaches (Silverman 2005 ; Flick 2007 ). It focuses not only on the objective nature of behavior but also on its subjective meanings: individuals’ own accounts of their attitudes, motivations, behavior (McIntyre 2005 :127; Creswell 2009 ), events and situations (Bryman 1989) – what people say and do in specific places and institutions (Goodwin and Horowitz 2002 :35–36) in social and temporal contexts (Morrill and Fine 1997). For this reason, following Weber ([1921-22] 1978), it can be described as an interpretative science (McIntyre 2005 :127). But could quantitative research also be concerned with these questions? Also, as pointed out below, does all qualitative research focus on subjective meaning, as some scholars suggest?
Others also distinguish qualitative research by claiming that it collects data using a naturalistic approach (Denzin and Lincoln 2005 :2; Creswell 2009 ), focusing on the meaning actors ascribe to their actions. But again, does all qualitative research need to be collected in situ? And does qualitative research have to be inherently concerned with meaning? Flick ( 2007 ), referring to Denzin and Lincoln ( 2005 ), mentions conversation analysis as an example of qualitative research that is not concerned with the meanings people bring to a situation, but rather with the formal organization of talk. Still others, such as Ragin ( 1994 :85), note that qualitative research is often (especially early on in the project, we would add) less structured than other kinds of social research – a characteristic connected to its flexibility and that can lead both to potentially better, but also worse results. But is this not a feature of this type of research, rather than a defining description of its essence? Wouldn’t this comment also apply, albeit to varying degrees, to quantitative research?
In addition, Strauss ( 2003 ), along with others, such as Alvesson and Kärreman ( 2011 :10–76), argue that qualitative researchers struggle to capture and represent complex phenomena partially because they tend to collect a large amount of data. While his analysis is correct at some points – “It is necessary to do detailed, intensive, microscopic examination of the data in order to bring out the amazing complexity of what lies in, behind, and beyond those data” (Strauss 2003 :10) – much of his analysis concerns the supposed focus of qualitative research and its challenges, rather than exactly what it is about. But even in this instance we would make a weak case arguing that these are strictly the defining features of qualitative research. Some researchers seem to focus on the approach or the methods used, or even on the way material is analyzed. Several researchers stress the naturalistic assumption of investigating the world, suggesting that meaning and interpretation appear to be a core matter of qualitative research.
We can also see that in this category there is no consensus about specific qualitative methods nor about qualitative data. Many emphasize interpretation, but quantitative research, too, involves interpretation; the results of a regression analysis, for example, certainly have to be interpreted, and the form of meta-analysis that factor analysis provides indeed requires interpretation However, there is no interpretation of quantitative raw data, i.e., numbers in tables. One common thread is that qualitative researchers have to get to grips with their data in order to understand what is being studied in great detail, irrespective of the type of empirical material that is being analyzed. This observation is connected to the fact that qualitative researchers routinely make several adjustments of focus and research design as their studies progress, in many cases until the very end of the project (Kalof et al. 2008 ). If you, like Becker, do not start out with a detailed theory, adjustments such as the emergence and refinement of research questions will occur during the research process. We have thus found a number of useful reflections about qualitative research scattered across different sources, but none of them effectively describe the defining characteristics of this approach.
Although qualitative research does not appear to be defined in terms of a specific method, it is certainly common that fieldwork, i.e., research that entails that the researcher spends considerable time in the field that is studied and use the knowledge gained as data, is seen as emblematic of or even identical to qualitative research. But because we understand that fieldwork tends to focus primarily on the collection and analysis of qualitative data, we expected to find within it discussions on the meaning of “qualitative.” But, again, this was not the case.
Instead, we found material on the history of this approach (for example, Frankfort-Nachmias and Nachmias 1996 ; Atkinson et al. 2001), including how it has changed; for example, by adopting a more self-reflexive practice (Heyl 2001), as well as the different nomenclature that has been adopted, such as fieldwork, ethnography, qualitative research, naturalistic research, participant observation and so on (for example, Lofland et al. 2006 ; Gans 1999 ).
We retrieved definitions of ethnography, such as “the study of people acting in the natural courses of their daily lives,” involving a “resocialization of the researcher” (Emerson 1988 :1) through intense immersion in others’ social worlds (see also examples in Hammersley 2018 ). This may be accomplished by direct observation and also participation (Neuman 2007 :276), although others, such as Denzin ( 1970 :185), have long recognized other types of observation, including non-participant (“fly on the wall”). In this category we have also isolated claims and opposing views, arguing that this type of research is distinguished primarily by where it is conducted (natural settings) (Hughes 1971:496), and how it is carried out (a variety of methods are applied) or, for some most importantly, by involving an active, empathetic immersion in those being studied (Emerson 1988 :2). We also retrieved descriptions of the goals it attends in relation to how it is taught (understanding subjective meanings of the people studied, primarily develop theory, or contribute to social change) (see for example, Corte and Irwin 2017 ; Frankfort-Nachmias and Nachmias 1996 :281; Trier-Bieniek 2012 :639) by collecting the richest possible data (Lofland et al. 2006 ) to derive “thick descriptions” (Geertz 1973 ), and/or to aim at theoretical statements of general scope and applicability (for example, Emerson 1988 ; Fine 2003 ). We have identified guidelines on how to evaluate it (for example Becker 1996 ; Lamont 2004 ) and have retrieved instructions on how it should be conducted (for example, Lofland et al. 2006 ). For instance, analysis should take place while the data gathering unfolds (Emerson 1988 ; Hammersley and Atkinson 2007 ; Lofland et al. 2006 ), observations should be of long duration (Becker 1970 :54; Goffman 1989 ), and data should be of high quantity (Becker 1970 :52–53), as well as other questionable distinctions between fieldwork and other methods:
Field studies differ from other methods of research in that the researcher performs the task of selecting topics, decides what questions to ask, and forges interest in the course of the research itself . This is in sharp contrast to many ‘theory-driven’ and ‘hypothesis-testing’ methods. (Lofland and Lofland 1995 :5)
But could not, for example, a strictly interview-based study be carried out with the same amount of flexibility, such as sequential interviewing (for example, Small 2009 )? Once again, are quantitative approaches really as inflexible as some qualitative researchers think? Moreover, this category stresses the role of the actors’ meaning, which requires knowledge and close interaction with people, their practices and their lifeworld.
It is clear that field studies – which are seen by some as the “gold standard” of qualitative research – are nonetheless only one way of doing qualitative research. There are other methods, but it is not clear why some are more qualitative than others, or why they are better or worse. Fieldwork is characterized by interaction with the field (the material) and understanding of the phenomenon that is being studied. In Becker’s case, he had general experience from fields in which marihuana was used, based on which he did interviews with actual users in several fields.
Grounded Theory
Another major category we identified in our sample is Grounded Theory. We found descriptions of it most clearly in Glaser and Strauss’ ([1967] 2010 ) original articulation, Strauss and Corbin ( 1998 ) and Charmaz ( 2006 ), as well as many other accounts of what it is for: generating and testing theory (Strauss 2003 :xi). We identified explanations of how this task can be accomplished – such as through two main procedures: constant comparison and theoretical sampling (Emerson 1998:96), and how using it has helped researchers to “think differently” (for example, Strauss and Corbin 1998 :1). We also read descriptions of its main traits, what it entails and fosters – for instance, an exceptional flexibility, an inductive approach (Strauss and Corbin 1998 :31–33; 1990; Esterberg 2002 :7), an ability to step back and critically analyze situations, recognize tendencies towards bias, think abstractly and be open to criticism, enhance sensitivity towards the words and actions of respondents, and develop a sense of absorption and devotion to the research process (Strauss and Corbin 1998 :5–6). Accordingly, we identified discussions of the value of triangulating different methods (both using and not using grounded theory), including quantitative ones, and theories to achieve theoretical development (most comprehensively in Denzin 1970 ; Strauss and Corbin 1998 ; Timmermans and Tavory 2012 ). We have also located arguments about how its practice helps to systematize data collection, analysis and presentation of results (Glaser and Strauss [1967] 2010 :16).
Grounded theory offers a systematic approach which requires researchers to get close to the field; closeness is a requirement of identifying questions and developing new concepts or making further distinctions with regard to old concepts. In contrast to other qualitative approaches, grounded theory emphasizes the detailed coding process, and the numerous fine-tuned distinctions that the researcher makes during the process. Within this category, too, we could not find a satisfying discussion of the meaning of qualitative research.
Defining Qualitative Research
In sum, our analysis shows that some notions reappear in the discussion of qualitative research, such as understanding, interpretation, “getting close” and making distinctions. These notions capture aspects of what we think is “qualitative.” However, a comprehensive definition that is useful and that can further develop the field is lacking, and not even a clear picture of its essential elements appears. In other words no definition emerges from our data, and in our research process we have moved back and forth between our empirical data and the attempt to present a definition. Our concrete strategy, as stated above, is to relate qualitative and quantitative research, or more specifically, qualitative and quantitative work. We use an ideal-typical notion of quantitative research which relies on taken for granted and numbered variables. This means that the data consists of variables on different scales, such as ordinal, but frequently ratio and absolute scales, and the representation of the numbers to the variables, i.e. the justification of the assignment of numbers to object or phenomenon, are not questioned, though the validity may be questioned. In this section we return to the notion of quality and try to clarify it while presenting our contribution.
Broadly, research refers to the activity performed by people trained to obtain knowledge through systematic procedures. Notions such as “objectivity” and “reflexivity,” “systematic,” “theory,” “evidence” and “openness” are here taken for granted in any type of research. Next, building on our empirical analysis we explain the four notions that we have identified as central to qualitative work: distinctions, process, closeness, and improved understanding. In discussing them, ultimately in relation to one another, we make their meaning even more precise. Our idea, in short, is that only when these ideas that we present separately for analytic purposes are brought together can we speak of qualitative research.
Distinctions
We believe that the possibility of making new distinctions is one the defining characteristics of qualitative research. It clearly sets it apart from quantitative analysis which works with taken-for-granted variables, albeit as mentioned, meta-analyses, for example, factor analysis may result in new variables. “Quality” refers essentially to distinctions, as already pointed out by Aristotle. He discusses the term “qualitative” commenting: “By a quality I mean that in virtue of which things are said to be qualified somehow” (Aristotle 1984:14). Quality is about what something is or has, which means that the distinction from its environment is crucial. We see qualitative research as a process in which significant new distinctions are made to the scholarly community; to make distinctions is a key aspect of obtaining new knowledge; a point, as we will see, that also has implications for “quantitative research.” The notion of being “significant” is paramount. New distinctions by themselves are not enough; just adding concepts only increases complexity without furthering our knowledge. The significance of new distinctions is judged against the communal knowledge of the research community. To enable this discussion and judgements central elements of rational discussion are required (cf. Habermas [1981] 1987 ; Davidsson [ 1988 ] 2001) to identify what is new and relevant scientific knowledge. Relatedly, Ragin alludes to the idea of new and useful knowledge at a more concrete level: “Qualitative methods are appropriate for in-depth examination of cases because they aid the identification of key features of cases. Most qualitative methods enhance data” (1994:79). When Becker ( 1963 ) studied deviant behavior and investigated how people became marihuana smokers, he made distinctions between the ways in which people learned how to smoke. This is a classic example of how the strategy of “getting close” to the material, for example the text, people or pictures that are subject to analysis, may enable researchers to obtain deeper insight and new knowledge by making distinctions – in this instance on the initial notion of learning how to smoke. Others have stressed the making of distinctions in relation to coding or theorizing. Emerson et al. ( 1995 ), for example, hold that “qualitative coding is a way of opening up avenues of inquiry,” meaning that the researcher identifies and develops concepts and analytic insights through close examination of and reflection on data (Emerson et al. 1995 :151). Goodwin and Horowitz highlight making distinctions in relation to theory-building writing: “Close engagement with their cases typically requires qualitative researchers to adapt existing theories or to make new conceptual distinctions or theoretical arguments to accommodate new data” ( 2002 : 37). In the ideal-typical quantitative research only existing and so to speak, given, variables would be used. If this is the case no new distinction are made. But, would not also many “quantitative” researchers make new distinctions?
Process does not merely suggest that research takes time. It mainly implies that qualitative new knowledge results from a process that involves several phases, and above all iteration. Qualitative research is about oscillation between theory and evidence, analysis and generating material, between first- and second -order constructs (Schütz 1962 :59), between getting in contact with something, finding sources, becoming deeply familiar with a topic, and then distilling and communicating some of its essential features. The main point is that the categories that the researcher uses, and perhaps takes for granted at the beginning of the research process, usually undergo qualitative changes resulting from what is found. Becker describes how he tested hypotheses and let the jargon of the users develop into theoretical concepts. This happens over time while the study is being conducted, exemplifying what we mean by process.
In the research process, a pilot-study may be used to get a first glance of, for example, the field, how to approach it, and what methods can be used, after which the method and theory are chosen or refined before the main study begins. Thus, the empirical material is often central from the start of the project and frequently leads to adjustments by the researcher. Likewise, during the main study categories are not fixed; the empirical material is seen in light of the theory used, but it is also given the opportunity to kick back, thereby resisting attempts to apply theoretical straightjackets (Becker 1970 :43). In this process, coding and analysis are interwoven, and thus are often important steps for getting closer to the phenomenon and deciding what to focus on next. Becker began his research by interviewing musicians close to him, then asking them to refer him to other musicians, and later on doubling his original sample of about 25 to include individuals in other professions (Becker 1973:46). Additionally, he made use of some participant observation, documents, and interviews with opiate users made available to him by colleagues. As his inductive theory of deviance evolved, Becker expanded his sample in order to fine tune it, and test the accuracy and generality of his hypotheses. In addition, he introduced a negative case and discussed the null hypothesis ( 1963 :44). His phasic career model is thus based on a research design that embraces processual work. Typically, process means to move between “theory” and “material” but also to deal with negative cases, and Becker ( 1998 ) describes how discovering these negative cases impacted his research design and ultimately its findings.
Obviously, all research is process-oriented to some degree. The point is that the ideal-typical quantitative process does not imply change of the data, and iteration between data, evidence, hypotheses, empirical work, and theory. The data, quantified variables, are, in most cases fixed. Merging of data, which of course can be done in a quantitative research process, does not mean new data. New hypotheses are frequently tested, but the “raw data is often the “the same.” Obviously, over time new datasets are made available and put into use.
Another characteristic that is emphasized in our sample is that qualitative researchers – and in particular ethnographers – can, or as Goffman put it, ought to ( 1989 ), get closer to the phenomenon being studied and their data than quantitative researchers (for example, Silverman 2009 :85). Put differently, essentially because of their methods qualitative researchers get into direct close contact with those being investigated and/or the material, such as texts, being analyzed. Becker started out his interview study, as we noted, by talking to those he knew in the field of music to get closer to the phenomenon he was studying. By conducting interviews he got even closer. Had he done more observations, he would undoubtedly have got even closer to the field.
Additionally, ethnographers’ design enables researchers to follow the field over time, and the research they do is almost by definition longitudinal, though the time in the field is studied obviously differs between studies. The general characteristic of closeness over time maximizes the chances of unexpected events, new data (related, for example, to archival research as additional sources, and for ethnography for situations not necessarily previously thought of as instrumental – what Mannay and Morgan ( 2015 ) term the “waiting field”), serendipity (Merton and Barber 2004 ; Åkerström 2013 ), and possibly reactivity, as well as the opportunity to observe disrupted patterns that translate into exemplars of negative cases. Two classic examples of this are Becker’s finding of what medical students call “crocks” (Becker et al. 1961 :317), and Geertz’s ( 1973 ) study of “deep play” in Balinese society.
By getting and staying so close to their data – be it pictures, text or humans interacting (Becker was himself a musician) – for a long time, as the research progressively focuses, qualitative researchers are prompted to continually test their hunches, presuppositions and hypotheses. They test them against a reality that often (but certainly not always), and practically, as well as metaphorically, talks back, whether by validating them, or disqualifying their premises – correctly, as well as incorrectly (Fine 2003 ; Becker 1970 ). This testing nonetheless often leads to new directions for the research. Becker, for example, says that he was initially reading psychological theories, but when facing the data he develops a theory that looks at, you may say, everything but psychological dispositions to explain the use of marihuana. Especially researchers involved with ethnographic methods have a fairly unique opportunity to dig up and then test (in a circular, continuous and temporal way) new research questions and findings as the research progresses, and thereby to derive previously unimagined and uncharted distinctions by getting closer to the phenomenon under study.
Let us stress that getting close is by no means restricted to ethnography. The notion of hermeneutic circle and hermeneutics as a general way of understanding implies that we must get close to the details in order to get the big picture. This also means that qualitative researchers can literally also make use of details of pictures as evidence (cf. Harper 2002). Thus, researchers may get closer both when generating the material or when analyzing it.
Quantitative research, we maintain, in the ideal-typical representation cannot get closer to the data. The data is essentially numbers in tables making up the variables (Franzosi 2016 :138). The data may originally have been “qualitative,” but once reduced to numbers there can only be a type of “hermeneutics” about what the number may stand for. The numbers themselves, however, are non-ambiguous. Thus, in quantitative research, interpretation, if done, is not about the data itself—the numbers—but what the numbers stand for. It follows that the interpretation is essentially done in a more “speculative” mode without direct empirical evidence (cf. Becker 2017 ).
Improved Understanding
While distinction, process and getting closer refer to the qualitative work of the researcher, improved understanding refers to its conditions and outcome of this work. Understanding cuts deeper than explanation, which to some may mean a causally verified correlation between variables. The notion of explanation presupposes the notion of understanding since explanation does not include an idea of how knowledge is gained (Manicas 2006 : 15). Understanding, we argue, is the core concept of what we call the outcome of the process when research has made use of all the other elements that were integrated in the research. Understanding, then, has a special status in qualitative research since it refers both to the conditions of knowledge and the outcome of the process. Understanding can to some extent be seen as the condition of explanation and occurs in a process of interpretation, which naturally refers to meaning (Gadamer 1990 ). It is fundamentally connected to knowing, and to the knowing of how to do things (Heidegger [1927] 2001 ). Conceptually the term hermeneutics is used to account for this process. Heidegger ties hermeneutics to human being and not possible to separate from the understanding of being ( 1988 ). Here we use it in a broader sense, and more connected to method in general (cf. Seiffert 1992 ). The abovementioned aspects – for example, “objectivity” and “reflexivity” – of the approach are conditions of scientific understanding. Understanding is the result of a circular process and means that the parts are understood in light of the whole, and vice versa. Understanding presupposes pre-understanding, or in other words, some knowledge of the phenomenon studied. The pre-understanding, even in the form of prejudices, are in qualitative research process, which we see as iterative, questioned, which gradually or suddenly change due to the iteration of data, evidence and concepts. However, qualitative research generates understanding in the iterative process when the researcher gets closer to the data, e.g., by going back and forth between field and analysis in a process that generates new data that changes the evidence, and, ultimately, the findings. Questioning, to ask questions, and put what one assumes—prejudices and presumption—in question, is central to understand something (Heidegger [1927] 2001 ; Gadamer 1990 :368–384). We propose that this iterative process in which the process of understanding occurs is characteristic of qualitative research.
Improved understanding means that we obtain scientific knowledge of something that we as a scholarly community did not know before, or that we get to know something better. It means that we understand more about how parts are related to one another, and to other things we already understand (see also Fine and Hallett 2014 ). Understanding is an important condition for qualitative research. It is not enough to identify correlations, make distinctions, and work in a process in which one gets close to the field or phenomena. Understanding is accomplished when the elements are integrated in an iterative process.
It is, moreover, possible to understand many things, and researchers, just like children, may come to understand new things every day as they engage with the world. This subjective condition of understanding – namely, that a person gains a better understanding of something –is easily met. To be qualified as “scientific,” the understanding must be general and useful to many; it must be public. But even this generally accessible understanding is not enough in order to speak of “scientific understanding.” Though we as a collective can increase understanding of everything in virtually all potential directions as a result also of qualitative work, we refrain from this “objective” way of understanding, which has no means of discriminating between what we gain in understanding. Scientific understanding means that it is deemed relevant from the scientific horizon (compare Schütz 1962 : 35–38, 46, 63), and that it rests on the pre-understanding that the scientists have and must have in order to understand. In other words, the understanding gained must be deemed useful by other researchers, so that they can build on it. We thus see understanding from a pragmatic, rather than a subjective or objective perspective. Improved understanding is related to the question(s) at hand. Understanding, in order to represent an improvement, must be an improvement in relation to the existing body of knowledge of the scientific community (James [ 1907 ] 1955). Scientific understanding is, by definition, collective, as expressed in Weber’s famous note on objectivity, namely that scientific work aims at truths “which … can claim, even for a Chinese, the validity appropriate to an empirical analysis” ([1904] 1949 :59). By qualifying “improved understanding” we argue that it is a general defining characteristic of qualitative research. Becker‘s ( 1966 ) study and other research of deviant behavior increased our understanding of the social learning processes of how individuals start a behavior. And it also added new knowledge about the labeling of deviant behavior as a social process. Few studies, of course, make the same large contribution as Becker’s, but are nonetheless qualitative research.
Understanding in the phenomenological sense, which is a hallmark of qualitative research, we argue, requires meaning and this meaning is derived from the context, and above all the data being analyzed. The ideal-typical quantitative research operates with given variables with different numbers. This type of material is not enough to establish meaning at the level that truly justifies understanding. In other words, many social science explanations offer ideas about correlations or even causal relations, but this does not mean that the meaning at the level of the data analyzed, is understood. This leads us to say that there are indeed many explanations that meet the criteria of understanding, for example the explanation of how one becomes a marihuana smoker presented by Becker. However, we may also understand a phenomenon without explaining it, and we may have potential explanations, or better correlations, that are not really understood.
We may speak more generally of quantitative research and its data to clarify what we see as an important distinction. The “raw data” that quantitative research—as an idealtypical activity, refers to is not available for further analysis; the numbers, once created, are not to be questioned (Franzosi 2016 : 138). If the researcher is to do “more” or “change” something, this will be done by conjectures based on theoretical knowledge or based on the researcher’s lifeworld. Both qualitative and quantitative research is based on the lifeworld, and all researchers use prejudices and pre-understanding in the research process. This idea is present in the works of Heidegger ( 2001 ) and Heisenberg (cited in Franzosi 2010 :619). Qualitative research, as we argued, involves the interaction and questioning of concepts (theory), data, and evidence.
Ragin ( 2004 :22) points out that “a good definition of qualitative research should be inclusive and should emphasize its key strengths and features, not what it lacks (for example, the use of sophisticated quantitative techniques).” We define qualitative research as an iterative process in which improved understanding to the scientific community is achieved by making new significant distinctions resulting from getting closer to the phenomenon studied. Qualitative research, as defined here, is consequently a combination of two criteria: (i) how to do things –namely, generating and analyzing empirical material, in an iterative process in which one gets closer by making distinctions, and (ii) the outcome –improved understanding novel to the scholarly community. Is our definition applicable to our own study? In this study we have closely read the empirical material that we generated, and the novel distinction of the notion “qualitative research” is the outcome of an iterative process in which both deduction and induction were involved, in which we identified the categories that we analyzed. We thus claim to meet the first criteria, “how to do things.” The second criteria cannot be judged but in a partial way by us, namely that the “outcome” —in concrete form the definition-improves our understanding to others in the scientific community.
We have defined qualitative research, or qualitative scientific work, in relation to quantitative scientific work. Given this definition, qualitative research is about questioning the pre-given (taken for granted) variables, but it is thus also about making new distinctions of any type of phenomenon, for example, by coining new concepts, including the identification of new variables. This process, as we have discussed, is carried out in relation to empirical material, previous research, and thus in relation to theory. Theory and previous research cannot be escaped or bracketed. According to hermeneutic principles all scientific work is grounded in the lifeworld, and as social scientists we can thus never fully bracket our pre-understanding.
We have proposed that quantitative research, as an idealtype, is concerned with pre-determined variables (Small 2008 ). Variables are epistemically fixed, but can vary in terms of dimensions, such as frequency or number. Age is an example; as a variable it can take on different numbers. In relation to quantitative research, qualitative research does not reduce its material to number and variables. If this is done the process of comes to a halt, the researcher gets more distanced from her data, and it makes it no longer possible to make new distinctions that increase our understanding. We have above discussed the components of our definition in relation to quantitative research. Our conclusion is that in the research that is called quantitative there are frequent and necessary qualitative elements.
Further, comparative empirical research on researchers primarily working with ”quantitative” approaches and those working with ”qualitative” approaches, we propose, would perhaps show that there are many similarities in practices of these two approaches. This is not to deny dissimilarities, or the different epistemic and ontic presuppositions that may be more or less strongly associated with the two different strands (see Goertz and Mahoney 2012 ). Our point is nonetheless that prejudices and preconceptions about researchers are unproductive, and that as other researchers have argued, differences may be exaggerated (e.g., Becker 1996 : 53, 2017 ; Marchel and Owens 2007 :303; Ragin 1994 ), and that a qualitative dimension is present in both kinds of work.
Several things follow from our findings. The most important result is the relation to quantitative research. In our analysis we have separated qualitative research from quantitative research. The point is not to label individual researchers, methods, projects, or works as either “quantitative” or “qualitative.” By analyzing, i.e., taking apart, the notions of quantitative and qualitative, we hope to have shown the elements of qualitative research. Our definition captures the elements, and how they, when combined in practice, generate understanding. As many of the quotations we have used suggest, one conclusion of our study holds that qualitative approaches are not inherently connected with a specific method. Put differently, none of the methods that are frequently labelled “qualitative,” such as interviews or participant observation, are inherently “qualitative.” What matters, given our definition, is whether one works qualitatively or quantitatively in the research process, until the results are produced. Consequently, our analysis also suggests that those researchers working with what in the literature and in jargon is often called “quantitative research” are almost bound to make use of what we have identified as qualitative elements in any research project. Our findings also suggest that many” quantitative” researchers, at least to some extent, are engaged with qualitative work, such as when research questions are developed, variables are constructed and combined, and hypotheses are formulated. Furthermore, a research project may hover between “qualitative” and “quantitative” or start out as “qualitative” and later move into a “quantitative” (a distinct strategy that is not similar to “mixed methods” or just simply combining induction and deduction). More generally speaking, the categories of “qualitative” and “quantitative,” unfortunately, often cover up practices, and it may lead to “camps” of researchers opposing one another. For example, regardless of the researcher is primarily oriented to “quantitative” or “qualitative” research, the role of theory is neglected (cf. Swedberg 2017 ). Our results open up for an interaction not characterized by differences, but by different emphasis, and similarities.
Let us take two examples to briefly indicate how qualitative elements can fruitfully be combined with quantitative. Franzosi ( 2010 ) has discussed the relations between quantitative and qualitative approaches, and more specifically the relation between words and numbers. He analyzes texts and argues that scientific meaning cannot be reduced to numbers. Put differently, the meaning of the numbers is to be understood by what is taken for granted, and what is part of the lifeworld (Schütz 1962 ). Franzosi shows how one can go about using qualitative and quantitative methods and data to address scientific questions analyzing violence in Italy at the time when fascism was rising (1919–1922). Aspers ( 2006 ) studied the meaning of fashion photographers. He uses an empirical phenomenological approach, and establishes meaning at the level of actors. In a second step this meaning, and the different ideal-typical photographers constructed as a result of participant observation and interviews, are tested using quantitative data from a database; in the first phase to verify the different ideal-types, in the second phase to use these types to establish new knowledge about the types. In both of these cases—and more examples can be found—authors move from qualitative data and try to keep the meaning established when using the quantitative data.
A second main result of our study is that a definition, and we provided one, offers a way for research to clarify, and even evaluate, what is done. Hence, our definition can guide researchers and students, informing them on how to think about concrete research problems they face, and to show what it means to get closer in a process in which new distinctions are made. The definition can also be used to evaluate the results, given that it is a standard of evaluation (cf. Hammersley 2007 ), to see whether new distinctions are made and whether this improves our understanding of what is researched, in addition to the evaluation of how the research was conducted. By making what is qualitative research explicit it becomes easier to communicate findings, and it is thereby much harder to fly under the radar with substandard research since there are standards of evaluation which make it easier to separate “good” from “not so good” qualitative research.
To conclude, our analysis, which ends with a definition of qualitative research can thus both address the “internal” issues of what is qualitative research, and the “external” critiques that make it harder to do qualitative research, to which both pressure from quantitative methods and general changes in society contribute.
Acknowledgements
Financial Support for this research is given by the European Research Council, CEV (263699). The authors are grateful to Susann Krieglsteiner for assistance in collecting the data. The paper has benefitted from the many useful comments by the three reviewers and the editor, comments by members of the Uppsala Laboratory of Economic Sociology, as well as Jukka Gronow, Sebastian Kohl, Marcin Serafin, Richard Swedberg, Anders Vassenden and Turid Rødne.
Biographies
is professor of sociology at the Department of Sociology, Uppsala University and Universität St. Gallen. His main focus is economic sociology, and in particular, markets. He has published numerous articles and books, including Orderly Fashion (Princeton University Press 2010), Markets (Polity Press 2011) and Re-Imagining Economic Sociology (edited with N. Dodd, Oxford University Press 2015). His book Ethnographic Methods (in Swedish) has already gone through several editions.
is associate professor of sociology at the Department of Media and Social Sciences, University of Stavanger. His research has been published in journals such as Social Psychology Quarterly, Sociological Theory, Teaching Sociology, and Music and Arts in Action. As an ethnographer he is working on a book on he social world of big-wave surfing.
Publisher’s Note
Springer Nature remains neutral with regard to jurisdictional claims in published maps and institutional affiliations.
Contributor Information
Patrik Aspers, Email: [email protected] .
Ugo Corte, Email: [email protected] .
- Åkerström M. Curiosity and serendipity in qualitative research. Qualitative Sociology Review. 2013; 9 (2):10–18. [ Google Scholar ]
- Alford, Robert R. 1998. The craft of inquiry. Theories, methods, evidence . Oxford: Oxford University Press.
- Alvesson M, Kärreman D. Qualitative research and theory development . Mystery as method . London: SAGE Publications; 2011. [ Google Scholar ]
- Aspers, Patrik. 2006. Markets in Fashion, A Phenomenological Approach. London Routledge.
- Atkinson P. Qualitative research. Unity and diversity. Forum: Qualitative Social Research. 2005; 6 (3):1–15. [ Google Scholar ]
- Becker HS. Outsiders. Studies in the sociology of deviance . New York: The Free Press; 1963. [ Google Scholar ]
- Becker HS. Whose side are we on? Social Problems. 1966; 14 (3):239–247. [ Google Scholar ]
- Becker HS. Sociological work. Method and substance. New Brunswick: Transaction Books; 1970. [ Google Scholar ]
- Becker HS. The epistemology of qualitative research. In: Richard J, Anne C, Shweder RA, editors. Ethnography and human development. Context and meaning in social inquiry. Chicago: University of Chicago Press; 1996. pp. 53–71. [ Google Scholar ]
- Becker HS. Tricks of the trade. How to think about your research while you're doing it. Chicago: University of Chicago Press; 1998. [ Google Scholar ]
- Becker, Howard S. 2017. Evidence . Chigaco: University of Chicago Press.
- Becker H, Geer B, Hughes E, Strauss A. Boys in White, student culture in medical school. New Brunswick: Transaction Publishers; 1961. [ Google Scholar ]
- Berezin M. How do we know what we mean? Epistemological dilemmas in cultural sociology. Qualitative Sociology. 2014; 37 (2):141–151. [ Google Scholar ]
- Best, Joel. 2004. Defining qualitative research. In Workshop on Scientific Foundations of Qualitative Research , eds . Charles, Ragin, Joanne, Nagel, and Patricia White, 53-54. http://www.nsf.gov/pubs/2004/nsf04219/nsf04219.pdf .
- Biernacki R. Humanist interpretation versus coding text samples. Qualitative Sociology. 2014; 37 (2):173–188. [ Google Scholar ]
- Blumer H. Symbolic interactionism: Perspective and method. Berkeley: University of California Press; 1969. [ Google Scholar ]
- Brady H, Collier D, Seawright J. Refocusing the discussion of methodology. In: Henry B, David C, editors. Rethinking social inquiry. Diverse tools, shared standards. Lanham: Rowman and Littlefield; 2004. pp. 3–22. [ Google Scholar ]
- Brown AP. Qualitative method and compromise in applied social research. Qualitative Research. 2010; 10 (2):229–248. [ Google Scholar ]
- Charmaz K. Constructing grounded theory. London: Sage; 2006. [ Google Scholar ]
- Corte, Ugo, and Katherine Irwin. 2017. “The Form and Flow of Teaching Ethnographic Knowledge: Hands-on Approaches for Learning Epistemology” Teaching Sociology 45(3): 209-219.
- Creswell JW. Research design. Qualitative, quantitative, and mixed method approaches. 3. Thousand Oaks: SAGE Publications; 2009. [ Google Scholar ]
- Davidsson D. The myth of the subjective. In: Davidsson D, editor. Subjective, intersubjective, objective. Oxford: Oxford University Press; 1988. pp. 39–52. [ Google Scholar ]
- Denzin NK. The research act: A theoretical introduction to Ssociological methods. Chicago: Aldine Publishing Company Publishers; 1970. [ Google Scholar ]
- Denzin NK, Lincoln YS. Introduction. The discipline and practice of qualitative research. In: Denzin NK, Lincoln YS, editors. Collecting and interpreting qualitative materials. Thousand Oaks: SAGE Publications; 2003. pp. 1–45. [ Google Scholar ]
- Denzin NK, Lincoln YS. Introduction. The discipline and practice of qualitative research. In: Denzin NK, Lincoln YS, editors. The Sage handbook of qualitative research. Thousand Oaks: SAGE Publications; 2005. pp. 1–32. [ Google Scholar ]
- Emerson RM, editor. Contemporary field research. A collection of readings. Prospect Heights: Waveland Press; 1988. [ Google Scholar ]
- Emerson RM, Fretz RI, Shaw LL. Writing ethnographic fieldnotes. Chicago: University of Chicago Press; 1995. [ Google Scholar ]
- Esterberg KG. Qualitative methods in social research. Boston: McGraw-Hill; 2002. [ Google Scholar ]
- Fine, Gary Alan. 1995. Review of “handbook of qualitative research.” Contemporary Sociology 24 (3): 416–418.
- Fine, Gary Alan. 2003. “ Toward a Peopled Ethnography: Developing Theory from Group Life.” Ethnography . 4(1):41-60.
- Fine GA, Hancock BH. The new ethnographer at work. Qualitative Research. 2017; 17 (2):260–268. [ Google Scholar ]
- Fine GA, Hallett T. Stranger and stranger: Creating theory through ethnographic distance and authority. Journal of Organizational Ethnography. 2014; 3 (2):188–203. [ Google Scholar ]
- Flick U. Qualitative research. State of the art. Social Science Information. 2002; 41 (1):5–24. [ Google Scholar ]
- Flick U. Designing qualitative research. London: SAGE Publications; 2007. [ Google Scholar ]
- Frankfort-Nachmias C, Nachmias D. Research methods in the social sciences. 5. London: Edward Arnold; 1996. [ Google Scholar ]
- Franzosi R. Sociology, narrative, and the quality versus quantity debate (Goethe versus Newton): Can computer-assisted story grammars help us understand the rise of Italian fascism (1919- 1922)? Theory and Society. 2010; 39 (6):593–629. [ Google Scholar ]
- Franzosi R. From method and measurement to narrative and number. International journal of social research methodology. 2016; 19 (1):137–141. [ Google Scholar ]
- Gadamer, Hans-Georg. 1990. Wahrheit und Methode, Grundzüge einer philosophischen Hermeneutik . Band 1, Hermeneutik. Tübingen: J.C.B. Mohr.
- Gans H. Participant Observation in an Age of “Ethnography” Journal of Contemporary Ethnography. 1999; 28 (5):540–548. [ Google Scholar ]
- Geertz C. The interpretation of cultures. New York: Basic Books; 1973. [ Google Scholar ]
- Gilbert N. Researching social life. 3. London: SAGE Publications; 2009. [ Google Scholar ]
- Glaeser A. Hermeneutic institutionalism: Towards a new synthesis. Qualitative Sociology. 2014; 37 :207–241. [ Google Scholar ]
- Glaser, Barney G., and Anselm L. Strauss. [1967] 2010. The discovery of grounded theory. Strategies for qualitative research. Hawthorne: Aldine.
- Goertz G, Mahoney J. A tale of two cultures: Qualitative and quantitative research in the social sciences. Princeton: Princeton University Press; 2012. [ Google Scholar ]
- Goffman E. On fieldwork. Journal of Contemporary Ethnography. 1989; 18 (2):123–132. [ Google Scholar ]
- Goodwin J, Horowitz R. Introduction. The methodological strengths and dilemmas of qualitative sociology. Qualitative Sociology. 2002; 25 (1):33–47. [ Google Scholar ]
- Habermas, Jürgen. [1981] 1987. The theory of communicative action . Oxford: Polity Press.
- Hammersley M. The issue of quality in qualitative research. International Journal of Research & Method in Education. 2007; 30 (3):287–305. [ Google Scholar ]
- Hammersley, Martyn. 2013. What is qualitative research? Bloomsbury Publishing.
- Hammersley M. What is ethnography? Can it survive should it? Ethnography and Education. 2018; 13 (1):1–17. [ Google Scholar ]
- Hammersley M, Atkinson P. Ethnography . Principles in practice . London: Tavistock Publications; 2007. [ Google Scholar ]
- Heidegger M. Sein und Zeit. Tübingen: Max Niemeyer Verlag; 2001. [ Google Scholar ]
- Heidegger, Martin. 1988. 1923. Ontologie. Hermeneutik der Faktizität, Gesamtausgabe II. Abteilung: Vorlesungen 1919-1944, Band 63, Frankfurt am Main: Vittorio Klostermann.
- Hempel CG. Philosophy of the natural sciences. Upper Saddle River: Prentice Hall; 1966. [ Google Scholar ]
- Hood JC. Teaching against the text. The case of qualitative methods. Teaching Sociology. 2006; 34 (3):207–223. [ Google Scholar ]
- James W. Pragmatism. New York: Meredian Books; 1907. [ Google Scholar ]
- Jovanović G. Toward a social history of qualitative research. History of the Human Sciences. 2011; 24 (2):1–27. [ Google Scholar ]
- Kalof L, Dan A, Dietz T. Essentials of social research. London: Open University Press; 2008. [ Google Scholar ]
- Katz J. Situational evidence: Strategies for causal reasoning from observational field notes. Sociological Methods & Research. 2015; 44 (1):108–144. [ Google Scholar ]
- King G, Keohane RO, Sidney S, Verba S. Scientific inference in qualitative research. Princeton: Princeton University Press; 1994. Designing social inquiry. [ Google Scholar ]
- Lamont M. Evaluating qualitative research: Some empirical findings and an agenda. In: Lamont M, White P, editors. Report from workshop on interdisciplinary standards for systematic qualitative research. Washington, DC: National Science Foundation; 2004. pp. 91–95. [ Google Scholar ]
- Lamont M, Swidler A. Methodological pluralism and the possibilities and limits of interviewing. Qualitative Sociology. 2014; 37 (2):153–171. [ Google Scholar ]
- Lazarsfeld P, Barton A. Some functions of qualitative analysis in social research. In: Kendall P, editor. The varied sociology of Paul Lazarsfeld. New York: Columbia University Press; 1982. pp. 239–285. [ Google Scholar ]
- Lichterman, Paul, and Isaac Reed I (2014), Theory and Contrastive Explanation in Ethnography. Sociological methods and research. Prepublished 27 October 2014; 10.1177/0049124114554458.
- Lofland J, Lofland L. Analyzing social settings. A guide to qualitative observation and analysis. 3. Belmont: Wadsworth; 1995. [ Google Scholar ]
- Lofland J, Snow DA, Anderson L, Lofland LH. Analyzing social settings. A guide to qualitative observation and analysis. 4. Belmont: Wadsworth/Thomson Learning; 2006. [ Google Scholar ]
- Long AF, Godfrey M. An evaluation tool to assess the quality of qualitative research studies. International Journal of Social Research Methodology. 2004; 7 (2):181–196. [ Google Scholar ]
- Lundberg G. Social research: A study in methods of gathering data. New York: Longmans, Green and Co.; 1951. [ Google Scholar ]
- Malinowski B. Argonauts of the Western Pacific: An account of native Enterprise and adventure in the archipelagoes of Melanesian New Guinea. London: Routledge; 1922. [ Google Scholar ]
- Manicas P. A realist philosophy of science: Explanation and understanding. Cambridge: Cambridge University Press; 2006. [ Google Scholar ]
- Marchel C, Owens S. Qualitative research in psychology. Could William James get a job? History of Psychology. 2007; 10 (4):301–324. [ PubMed ] [ Google Scholar ]
- McIntyre LJ. Need to know. Social science research methods. Boston: McGraw-Hill; 2005. [ Google Scholar ]
- Merton RK, Barber E. The travels and adventures of serendipity . A Study in Sociological Semantics and the Sociology of Science. Princeton: Princeton University Press; 2004. [ Google Scholar ]
- Mannay D, Morgan M. Doing ethnography or applying a qualitative technique? Reflections from the ‘waiting field‘ Qualitative Research. 2015; 15 (2):166–182. [ Google Scholar ]
- Neuman LW. Basics of social research. Qualitative and quantitative approaches. 2. Boston: Pearson Education; 2007. [ Google Scholar ]
- Ragin CC. Constructing social research. The unity and diversity of method. Thousand Oaks: Pine Forge Press; 1994. [ Google Scholar ]
- Ragin, Charles C. 2004. Introduction to session 1: Defining qualitative research. In Workshop on Scientific Foundations of Qualitative Research , 22, ed. Charles C. Ragin, Joane Nagel, Patricia White. http://www.nsf.gov/pubs/2004/nsf04219/nsf04219.pdf
- Rawls, Anne. 2018. The Wartime narrative in US sociology, 1940–7: Stigmatizing qualitative sociology in the name of ‘science,’ European Journal of Social Theory (Online first).
- Schütz A. Collected papers I: The problem of social reality. The Hague: Nijhoff; 1962. [ Google Scholar ]
- Seiffert H. Einführung in die Hermeneutik. Tübingen: Franke; 1992. [ Google Scholar ]
- Silverman D. Doing qualitative research. A practical handbook. 2. London: SAGE Publications; 2005. [ Google Scholar ]
- Silverman D. A very short, fairly interesting and reasonably cheap book about qualitative research. London: SAGE Publications; 2009. [ Google Scholar ]
- Silverman D. What counts as qualitative research? Some cautionary comments. Qualitative Sociology Review. 2013; 9 (2):48–55. [ Google Scholar ]
- Small ML. “How many cases do I need?” on science and the logic of case selection in field-based research. Ethnography. 2009; 10 (1):5–38. [ Google Scholar ]
- Small, Mario L 2008. Lost in translation: How not to make qualitative research more scientific. In Workshop on interdisciplinary standards for systematic qualitative research, ed in Michelle Lamont, and Patricia White, 165–171. Washington, DC: National Science Foundation.
- Snow DA, Anderson L. Down on their luck: A study of homeless street people. Berkeley: University of California Press; 1993. [ Google Scholar ]
- Snow DA, Morrill C. New ethnographies: Review symposium: A revolutionary handbook or a handbook for revolution? Journal of Contemporary Ethnography. 1995; 24 (3):341–349. [ Google Scholar ]
- Strauss AL. Qualitative analysis for social scientists. 14. Chicago: Cambridge University Press; 2003. [ Google Scholar ]
- Strauss AL, Corbin JM. Basics of qualitative research. Techniques and procedures for developing grounded theory. 2. Thousand Oaks: Sage Publications; 1998. [ Google Scholar ]
- Swedberg, Richard. 2017. Theorizing in sociological research: A new perspective, a new departure? Annual Review of Sociology 43: 189–206.
- Swedberg R. The new 'Battle of Methods'. Challenge January–February. 1990; 3 (1):33–38. [ Google Scholar ]
- Timmermans S, Tavory I. Theory construction in qualitative research: From grounded theory to abductive analysis. Sociological Theory. 2012; 30 (3):167–186. [ Google Scholar ]
- Trier-Bieniek A. Framing the telephone interview as a participant-centred tool for qualitative research. A methodological discussion. Qualitative Research. 2012; 12 (6):630–644. [ Google Scholar ]
- Valsiner J. Data as representations. Contextualizing qualitative and quantitative research strategies. Social Science Information. 2000; 39 (1):99–113. [ Google Scholar ]
- Weber, Max. 1904. 1949. Objectivity’ in social Science and social policy. Ed. Edward A. Shils and Henry A. Finch, 49–112. New York: The Free Press.
- What is qualitative data?
Last updated
8 February 2023
Reviewed by
Miroslav Damyanov
If you're wondering how qualitative data can help your business or innovative research efforts, keep reading. This is the ultimate guide to understanding what it is, how it works, and where to begin in collecting and analyzing data.
Analyze all your qualitative data
Analyze qualitative data faster and surface more actionable insights with Dovetail
For those who want to leverage the power of qualitative data, it's essential to understand the basic definitions first.
When working with qualitative data, you're collecting and reviewing metrics that characterize and approximate. You source this data through observation, including interviews, surveys , and focus groups .
With responses and analytics in hand, you can categorize your findings in terms of feelings, attributes, or properties.
- Qualitative vs. quantitative data
You've likely heard of quantitative data. It's equally important to understand the distinctions between quantitative and qualitative datasets. While qualitative data measures feelings, behaviors, and properties, quantitative data are measures of values expressed as numbers.
Quantitative data answers questions like how much or how often . Qualitative data will answer questions like what type or why . Because qualitative data evaluate deeper sentiments, they're best at illustrating thought processes and behaviors behind the raw quantitative data.
- Importance of qualitative data
Qualitative data plays a more pivotal role in research and business today, especially because it helps unravel the characteristics and behavioral motivations. This data allows researchers and managers to "qualify" the environment or ecosystem they're studying. These analytics allow you to dive deeper into understanding people’s emotional or perceptual motivations.
These metrics provide insights about target audiences and customer decision-making preferences in business. These datasets are great for solving problems and paving the way for innovations.
Properly collecting and analyzing qualitative data will provide all the insights you need to prioritize your focus, address challenges, and resolve issues.
- Types of qualitative data
Since qualitative data is more of an exploratory process, it involves a more in-depth look at behaviors and concepts. And there are various types of qualitative data to explore.
How you collect qualitative data and the channels you use will help you organize your results. Most data fits into one of three pillar categories: Nominal, binary, and ordinal. But all initiatives should answer a primary research question.
Most qualitative data methods aim to:
Gain behavioral insights
Understand reasoning
Explore motivations
Identify emotional connections
- Qualitative data examples
It may be helpful to see qualitative data examples to better understand how to apply these collection and analysis methods to your model. Here are a few simplified samples to illustrate the value of these metrics in business and research.
Measuring characteristics
If you're studying a group of women, you might dive deeper to measure their characteristics. For example, you can collect qualitative data about the various hair colors or current jobs of the women in your study group. These qualitative metrics are great for developing marketing personas in business applications.
Measuring behaviors
Imagine you're studying a group of children in a room full of different toys. Measuring qualitative data might include observing which toys those children choose to play with first. Studying these behaviors will help you better understand the behavior behind what attracts a child to a particular type of toy, which is great for product innovation initiatives.
Measuring motivations
Consumers buying products or services will make their purchasing decisions according to personal motivations. Qualitative data surveys can help you study particular groups to evaluate why those consumers decided to buy. Knowing if your target audience is more motivated by price point, free shipping, or customer service will help you change how you engage them.
- Qualitative data collection methods
When you're ready to explore collecting and analyzing qualitative data, there are several collection methods to consider. Surveys and focus groups are great tools, but there are other methods, too. Each method is uniquely beneficial to specific metrics and research goals.
One-on-one interviews
One of the most common data collection methods, this qualitative research effort provides a more personal approach to determining sentiments and behaviors. Interviews with open-ended questions net the most in-depth responses and results.
Focus groups
Usually limited to ten or fewer participants, this method assigns a moderator to initiate a group discussion of ideas and sentiments. Members may all have something in common, but their responses will contribute to your qualitative datasets.
Case studies
These methods are ideal for collecting specific in-depth data using a combination of qualitative data sources to gain contextual knowledge about a specific real-world phenomenon.
Longitudinal studies
This research approach is where you collect data from the same participants repeatedly. It's an observational model for dynamically comparing a captured group's results over days or even decades.
Record keeping
With this method, you can use existing sources of information to inspire new research. Much like visiting a library, you can study reference material to discover new qualitative data you should be studying.
Observation
This method involves a researcher immersing into a setting to watch and take notes of participants. Documentation might be in the form of note-taking, video observation, photography, or audio recording.
- What is qualitative data analysis?
Once you've collected the qualitative data, it's time to evaluate and analyze it to produce inferences and actionable applications. There are no hard or fast rules for interpreting the data you collect. However, there are two primary approaches to understanding the qualitative data you've assembled for review.
Deductive approach
If you've already outlined a structure as part of your data collection process, you're using a deductive approach to analyzing data. You use this analysis method when you already have an idea about the responses in the dataset. This approach is often easier to execute since you establish much of the groundwork during the data collection stage.
Inductive approach
This method involves analyzing qualitative data when you have no idea what the responses, results, or research phenomenon will be. It's more time-consuming to study data this way, but it can also be where researchers find revolutionary anomalies and lightbulb, a-ha solutions.
- How do you begin analyzing qualitative data?
To help you begin analyzing your recently collected qualitative data, you can loosely follow these basic steps to initiate your analysis.
Arrange your data into systems or categories into a software platform or analysis tool that allows you to easily visualize what you've collected.
Organize your qualitative data according to your research objectives using tables, spreadsheets, or visually appealing graphics.
Assigning proper codes for your data will help you compress vast libraries of information. Coding really translates to categories but takes it a step further by assigning properties and patterns. Codes will help you draw conclusions later.
Start validating your qualitative data to identify viable collection samples and eliminate any flawed or misconstrued datasets. Verify the accuracy of the collection methods and confirm the reliability and accuracy of the data you’ve collected.
Conclude your data with systematic presentation in a condensed report. In this step, you'll outline the methods you used in collection, the researchers involved, and the approach. You'll share the positives, negatives, and limitations of your study. Here, you draw inferences about your findings and offer suggestions for action or future research.
- Sharing qualitative analysis
When you're ready to share your qualitative analysis findings, you can choose a variety of formats to suit your presentation audience. These might include:
Digital or physical reports
Images or infographics
Audio or visual materials
Scanned historical documents
Observation dictations
Field notes
When explaining your analysis, it's best to share the purpose and parameters of your study. Then you can offer immediate results. Your qualitative analysis process will allow you to draw conclusions, apply judgment, and determine the next steps based on your unique scenario.
- Advantages of qualitative data
There are inherent advantages to applying qualitative data collection and analysis methods to business and research projects. These are the three most pivotal benefits of tapping into regular qualitative data initiatives ongoing:
Get in-depth data beyond the numbers.
Understand participants or consumer behaviors more intuitively.
Discover rich data you can use and reuse well into the future.
- Disadvantages of qualitative data
A few disadvantages of qualitative data practices are worth noting. Before adopting these approaches, consider these potential setbacks:
Proper qualitative data collection and analysis is time-consuming.
The data can be hard to generalize, especially with fewer participants.
The researcher’s data analysis skills and the results are directly correlated.
- Qualitative analysis tools
As complex as qualitative data analysis can be, you don't have to go it alone or recreate the wheel. Many incredible tools and resources are available to simplify each step of the research process.
Explore software solutions that streamline how you collect data, like online survey tools or customer questionnaires. Discover the design and data management software solutions out there intended to help you categorize and organize your data.
Software packages for qualitative data analysis are your best friend when entering the analysis stage and looking for patterns and conclusions.
Start leveraging the many benefits of qualitative data analysis for your business or research project. Remember to reference this guide as you develop your studies and determine your methods.
Should you be using a customer insights hub?
Do you want to discover previous research faster?
Do you share your research findings with others?
Do you analyze research data?
Start for free today, add your research, and get to key insights faster
Editor’s picks
Last updated: 18 April 2023
Last updated: 27 February 2023
Last updated: 22 August 2024
Last updated: 5 February 2023
Last updated: 16 August 2024
Last updated: 9 March 2023
Last updated: 30 April 2024
Last updated: 12 December 2023
Last updated: 11 March 2024
Last updated: 4 July 2024
Last updated: 6 March 2024
Last updated: 5 March 2024
Last updated: 13 May 2024
Latest articles
Related topics, .css-je19u9{-webkit-align-items:flex-end;-webkit-box-align:flex-end;-ms-flex-align:flex-end;align-items:flex-end;display:-webkit-box;display:-webkit-flex;display:-ms-flexbox;display:flex;-webkit-flex-direction:row;-ms-flex-direction:row;flex-direction:row;-webkit-box-flex-wrap:wrap;-webkit-flex-wrap:wrap;-ms-flex-wrap:wrap;flex-wrap:wrap;-webkit-box-pack:center;-ms-flex-pack:center;-webkit-justify-content:center;justify-content:center;row-gap:0;text-align:center;max-width:671px;}@media (max-width: 1079px){.css-je19u9{max-width:400px;}.css-je19u9>span{white-space:pre;}}@media (max-width: 799px){.css-je19u9{max-width:400px;}.css-je19u9>span{white-space:pre;}} decide what to .css-1kiodld{max-height:56px;display:-webkit-box;display:-webkit-flex;display:-ms-flexbox;display:flex;-webkit-align-items:center;-webkit-box-align:center;-ms-flex-align:center;align-items:center;}@media (max-width: 1079px){.css-1kiodld{display:none;}} build next, decide what to build next, log in or sign up.
Get started for free
- Privacy Policy

Home » Qualitative Research – Methods, Analysis Types and Guide
Qualitative Research – Methods, Analysis Types and Guide
Table of Contents

Qualitative Research
Qualitative research is a type of research methodology that focuses on exploring and understanding people’s beliefs, attitudes, behaviors, and experiences through the collection and analysis of non-numerical data. It seeks to answer research questions through the examination of subjective data, such as interviews, focus groups, observations, and textual analysis.
Qualitative research aims to uncover the meaning and significance of social phenomena, and it typically involves a more flexible and iterative approach to data collection and analysis compared to quantitative research. Qualitative research is often used in fields such as sociology, anthropology, psychology, and education.
Qualitative Research Methods

Qualitative Research Methods are as follows:
One-to-One Interview
This method involves conducting an interview with a single participant to gain a detailed understanding of their experiences, attitudes, and beliefs. One-to-one interviews can be conducted in-person, over the phone, or through video conferencing. The interviewer typically uses open-ended questions to encourage the participant to share their thoughts and feelings. One-to-one interviews are useful for gaining detailed insights into individual experiences.
Focus Groups
This method involves bringing together a group of people to discuss a specific topic in a structured setting. The focus group is led by a moderator who guides the discussion and encourages participants to share their thoughts and opinions. Focus groups are useful for generating ideas and insights, exploring social norms and attitudes, and understanding group dynamics.
Ethnographic Studies
This method involves immersing oneself in a culture or community to gain a deep understanding of its norms, beliefs, and practices. Ethnographic studies typically involve long-term fieldwork and observation, as well as interviews and document analysis. Ethnographic studies are useful for understanding the cultural context of social phenomena and for gaining a holistic understanding of complex social processes.
Text Analysis
This method involves analyzing written or spoken language to identify patterns and themes. Text analysis can be quantitative or qualitative. Qualitative text analysis involves close reading and interpretation of texts to identify recurring themes, concepts, and patterns. Text analysis is useful for understanding media messages, public discourse, and cultural trends.
This method involves an in-depth examination of a single person, group, or event to gain an understanding of complex phenomena. Case studies typically involve a combination of data collection methods, such as interviews, observations, and document analysis, to provide a comprehensive understanding of the case. Case studies are useful for exploring unique or rare cases, and for generating hypotheses for further research.
Process of Observation
This method involves systematically observing and recording behaviors and interactions in natural settings. The observer may take notes, use audio or video recordings, or use other methods to document what they see. Process of observation is useful for understanding social interactions, cultural practices, and the context in which behaviors occur.
Record Keeping
This method involves keeping detailed records of observations, interviews, and other data collected during the research process. Record keeping is essential for ensuring the accuracy and reliability of the data, and for providing a basis for analysis and interpretation.
This method involves collecting data from a large sample of participants through a structured questionnaire. Surveys can be conducted in person, over the phone, through mail, or online. Surveys are useful for collecting data on attitudes, beliefs, and behaviors, and for identifying patterns and trends in a population.
Qualitative data analysis is a process of turning unstructured data into meaningful insights. It involves extracting and organizing information from sources like interviews, focus groups, and surveys. The goal is to understand people’s attitudes, behaviors, and motivations
Qualitative Research Analysis Methods
Qualitative Research analysis methods involve a systematic approach to interpreting and making sense of the data collected in qualitative research. Here are some common qualitative data analysis methods:
Thematic Analysis
This method involves identifying patterns or themes in the data that are relevant to the research question. The researcher reviews the data, identifies keywords or phrases, and groups them into categories or themes. Thematic analysis is useful for identifying patterns across multiple data sources and for generating new insights into the research topic.
Content Analysis
This method involves analyzing the content of written or spoken language to identify key themes or concepts. Content analysis can be quantitative or qualitative. Qualitative content analysis involves close reading and interpretation of texts to identify recurring themes, concepts, and patterns. Content analysis is useful for identifying patterns in media messages, public discourse, and cultural trends.
Discourse Analysis
This method involves analyzing language to understand how it constructs meaning and shapes social interactions. Discourse analysis can involve a variety of methods, such as conversation analysis, critical discourse analysis, and narrative analysis. Discourse analysis is useful for understanding how language shapes social interactions, cultural norms, and power relationships.
Grounded Theory Analysis
This method involves developing a theory or explanation based on the data collected. Grounded theory analysis starts with the data and uses an iterative process of coding and analysis to identify patterns and themes in the data. The theory or explanation that emerges is grounded in the data, rather than preconceived hypotheses. Grounded theory analysis is useful for understanding complex social phenomena and for generating new theoretical insights.
Narrative Analysis
This method involves analyzing the stories or narratives that participants share to gain insights into their experiences, attitudes, and beliefs. Narrative analysis can involve a variety of methods, such as structural analysis, thematic analysis, and discourse analysis. Narrative analysis is useful for understanding how individuals construct their identities, make sense of their experiences, and communicate their values and beliefs.
Phenomenological Analysis
This method involves analyzing how individuals make sense of their experiences and the meanings they attach to them. Phenomenological analysis typically involves in-depth interviews with participants to explore their experiences in detail. Phenomenological analysis is useful for understanding subjective experiences and for developing a rich understanding of human consciousness.
Comparative Analysis
This method involves comparing and contrasting data across different cases or groups to identify similarities and differences. Comparative analysis can be used to identify patterns or themes that are common across multiple cases, as well as to identify unique or distinctive features of individual cases. Comparative analysis is useful for understanding how social phenomena vary across different contexts and groups.
Applications of Qualitative Research
Qualitative research has many applications across different fields and industries. Here are some examples of how qualitative research is used:
- Market Research: Qualitative research is often used in market research to understand consumer attitudes, behaviors, and preferences. Researchers conduct focus groups and one-on-one interviews with consumers to gather insights into their experiences and perceptions of products and services.
- Health Care: Qualitative research is used in health care to explore patient experiences and perspectives on health and illness. Researchers conduct in-depth interviews with patients and their families to gather information on their experiences with different health care providers and treatments.
- Education: Qualitative research is used in education to understand student experiences and to develop effective teaching strategies. Researchers conduct classroom observations and interviews with students and teachers to gather insights into classroom dynamics and instructional practices.
- Social Work : Qualitative research is used in social work to explore social problems and to develop interventions to address them. Researchers conduct in-depth interviews with individuals and families to understand their experiences with poverty, discrimination, and other social problems.
- Anthropology : Qualitative research is used in anthropology to understand different cultures and societies. Researchers conduct ethnographic studies and observe and interview members of different cultural groups to gain insights into their beliefs, practices, and social structures.
- Psychology : Qualitative research is used in psychology to understand human behavior and mental processes. Researchers conduct in-depth interviews with individuals to explore their thoughts, feelings, and experiences.
- Public Policy : Qualitative research is used in public policy to explore public attitudes and to inform policy decisions. Researchers conduct focus groups and one-on-one interviews with members of the public to gather insights into their perspectives on different policy issues.
How to Conduct Qualitative Research
Here are some general steps for conducting qualitative research:
- Identify your research question: Qualitative research starts with a research question or set of questions that you want to explore. This question should be focused and specific, but also broad enough to allow for exploration and discovery.
- Select your research design: There are different types of qualitative research designs, including ethnography, case study, grounded theory, and phenomenology. You should select a design that aligns with your research question and that will allow you to gather the data you need to answer your research question.
- Recruit participants: Once you have your research question and design, you need to recruit participants. The number of participants you need will depend on your research design and the scope of your research. You can recruit participants through advertisements, social media, or through personal networks.
- Collect data: There are different methods for collecting qualitative data, including interviews, focus groups, observation, and document analysis. You should select the method or methods that align with your research design and that will allow you to gather the data you need to answer your research question.
- Analyze data: Once you have collected your data, you need to analyze it. This involves reviewing your data, identifying patterns and themes, and developing codes to organize your data. You can use different software programs to help you analyze your data, or you can do it manually.
- Interpret data: Once you have analyzed your data, you need to interpret it. This involves making sense of the patterns and themes you have identified, and developing insights and conclusions that answer your research question. You should be guided by your research question and use your data to support your conclusions.
- Communicate results: Once you have interpreted your data, you need to communicate your results. This can be done through academic papers, presentations, or reports. You should be clear and concise in your communication, and use examples and quotes from your data to support your findings.
Examples of Qualitative Research
Here are some real-time examples of qualitative research:
- Customer Feedback: A company may conduct qualitative research to understand the feedback and experiences of its customers. This may involve conducting focus groups or one-on-one interviews with customers to gather insights into their attitudes, behaviors, and preferences.
- Healthcare : A healthcare provider may conduct qualitative research to explore patient experiences and perspectives on health and illness. This may involve conducting in-depth interviews with patients and their families to gather information on their experiences with different health care providers and treatments.
- Education : An educational institution may conduct qualitative research to understand student experiences and to develop effective teaching strategies. This may involve conducting classroom observations and interviews with students and teachers to gather insights into classroom dynamics and instructional practices.
- Social Work: A social worker may conduct qualitative research to explore social problems and to develop interventions to address them. This may involve conducting in-depth interviews with individuals and families to understand their experiences with poverty, discrimination, and other social problems.
- Anthropology : An anthropologist may conduct qualitative research to understand different cultures and societies. This may involve conducting ethnographic studies and observing and interviewing members of different cultural groups to gain insights into their beliefs, practices, and social structures.
- Psychology : A psychologist may conduct qualitative research to understand human behavior and mental processes. This may involve conducting in-depth interviews with individuals to explore their thoughts, feelings, and experiences.
- Public Policy: A government agency or non-profit organization may conduct qualitative research to explore public attitudes and to inform policy decisions. This may involve conducting focus groups and one-on-one interviews with members of the public to gather insights into their perspectives on different policy issues.
Purpose of Qualitative Research
The purpose of qualitative research is to explore and understand the subjective experiences, behaviors, and perspectives of individuals or groups in a particular context. Unlike quantitative research, which focuses on numerical data and statistical analysis, qualitative research aims to provide in-depth, descriptive information that can help researchers develop insights and theories about complex social phenomena.
Qualitative research can serve multiple purposes, including:
- Exploring new or emerging phenomena : Qualitative research can be useful for exploring new or emerging phenomena, such as new technologies or social trends. This type of research can help researchers develop a deeper understanding of these phenomena and identify potential areas for further study.
- Understanding complex social phenomena : Qualitative research can be useful for exploring complex social phenomena, such as cultural beliefs, social norms, or political processes. This type of research can help researchers develop a more nuanced understanding of these phenomena and identify factors that may influence them.
- Generating new theories or hypotheses: Qualitative research can be useful for generating new theories or hypotheses about social phenomena. By gathering rich, detailed data about individuals’ experiences and perspectives, researchers can develop insights that may challenge existing theories or lead to new lines of inquiry.
- Providing context for quantitative data: Qualitative research can be useful for providing context for quantitative data. By gathering qualitative data alongside quantitative data, researchers can develop a more complete understanding of complex social phenomena and identify potential explanations for quantitative findings.
When to use Qualitative Research
Here are some situations where qualitative research may be appropriate:
- Exploring a new area: If little is known about a particular topic, qualitative research can help to identify key issues, generate hypotheses, and develop new theories.
- Understanding complex phenomena: Qualitative research can be used to investigate complex social, cultural, or organizational phenomena that are difficult to measure quantitatively.
- Investigating subjective experiences: Qualitative research is particularly useful for investigating the subjective experiences of individuals or groups, such as their attitudes, beliefs, values, or emotions.
- Conducting formative research: Qualitative research can be used in the early stages of a research project to develop research questions, identify potential research participants, and refine research methods.
- Evaluating interventions or programs: Qualitative research can be used to evaluate the effectiveness of interventions or programs by collecting data on participants’ experiences, attitudes, and behaviors.
Characteristics of Qualitative Research
Qualitative research is characterized by several key features, including:
- Focus on subjective experience: Qualitative research is concerned with understanding the subjective experiences, beliefs, and perspectives of individuals or groups in a particular context. Researchers aim to explore the meanings that people attach to their experiences and to understand the social and cultural factors that shape these meanings.
- Use of open-ended questions: Qualitative research relies on open-ended questions that allow participants to provide detailed, in-depth responses. Researchers seek to elicit rich, descriptive data that can provide insights into participants’ experiences and perspectives.
- Sampling-based on purpose and diversity: Qualitative research often involves purposive sampling, in which participants are selected based on specific criteria related to the research question. Researchers may also seek to include participants with diverse experiences and perspectives to capture a range of viewpoints.
- Data collection through multiple methods: Qualitative research typically involves the use of multiple data collection methods, such as in-depth interviews, focus groups, and observation. This allows researchers to gather rich, detailed data from multiple sources, which can provide a more complete picture of participants’ experiences and perspectives.
- Inductive data analysis: Qualitative research relies on inductive data analysis, in which researchers develop theories and insights based on the data rather than testing pre-existing hypotheses. Researchers use coding and thematic analysis to identify patterns and themes in the data and to develop theories and explanations based on these patterns.
- Emphasis on researcher reflexivity: Qualitative research recognizes the importance of the researcher’s role in shaping the research process and outcomes. Researchers are encouraged to reflect on their own biases and assumptions and to be transparent about their role in the research process.
Advantages of Qualitative Research
Qualitative research offers several advantages over other research methods, including:
- Depth and detail: Qualitative research allows researchers to gather rich, detailed data that provides a deeper understanding of complex social phenomena. Through in-depth interviews, focus groups, and observation, researchers can gather detailed information about participants’ experiences and perspectives that may be missed by other research methods.
- Flexibility : Qualitative research is a flexible approach that allows researchers to adapt their methods to the research question and context. Researchers can adjust their research methods in real-time to gather more information or explore unexpected findings.
- Contextual understanding: Qualitative research is well-suited to exploring the social and cultural context in which individuals or groups are situated. Researchers can gather information about cultural norms, social structures, and historical events that may influence participants’ experiences and perspectives.
- Participant perspective : Qualitative research prioritizes the perspective of participants, allowing researchers to explore subjective experiences and understand the meanings that participants attach to their experiences.
- Theory development: Qualitative research can contribute to the development of new theories and insights about complex social phenomena. By gathering rich, detailed data and using inductive data analysis, researchers can develop new theories and explanations that may challenge existing understandings.
- Validity : Qualitative research can offer high validity by using multiple data collection methods, purposive and diverse sampling, and researcher reflexivity. This can help ensure that findings are credible and trustworthy.
Limitations of Qualitative Research
Qualitative research also has some limitations, including:
- Subjectivity : Qualitative research relies on the subjective interpretation of researchers, which can introduce bias into the research process. The researcher’s perspective, beliefs, and experiences can influence the way data is collected, analyzed, and interpreted.
- Limited generalizability: Qualitative research typically involves small, purposive samples that may not be representative of larger populations. This limits the generalizability of findings to other contexts or populations.
- Time-consuming: Qualitative research can be a time-consuming process, requiring significant resources for data collection, analysis, and interpretation.
- Resource-intensive: Qualitative research may require more resources than other research methods, including specialized training for researchers, specialized software for data analysis, and transcription services.
- Limited reliability: Qualitative research may be less reliable than quantitative research, as it relies on the subjective interpretation of researchers. This can make it difficult to replicate findings or compare results across different studies.
- Ethics and confidentiality: Qualitative research involves collecting sensitive information from participants, which raises ethical concerns about confidentiality and informed consent. Researchers must take care to protect the privacy and confidentiality of participants and obtain informed consent.
Also see Research Methods
About the author
Muhammad Hassan
Researcher, Academic Writer, Web developer
You may also like

Exploratory Research – Types, Methods and...

Phenomenology – Methods, Examples and Guide

Mixed Methods Research – Types & Analysis

Focus Groups – Steps, Examples and Guide

Survey Research – Types, Methods, Examples

One-to-One Interview – Methods and Guide
- Skip to main content
- Skip to primary sidebar
- Skip to footer
- QuestionPro

- Solutions Industries Gaming Automotive Sports and events Education Government Travel & Hospitality Financial Services Healthcare Cannabis Technology Use Case AskWhy Communities Audience Contactless surveys Mobile LivePolls Member Experience GDPR Positive People Science 360 Feedback Surveys
- Resources Blog eBooks Survey Templates Case Studies Training Help center

Home Market Research
Qualitative Data Collection: What it is + Methods to do it
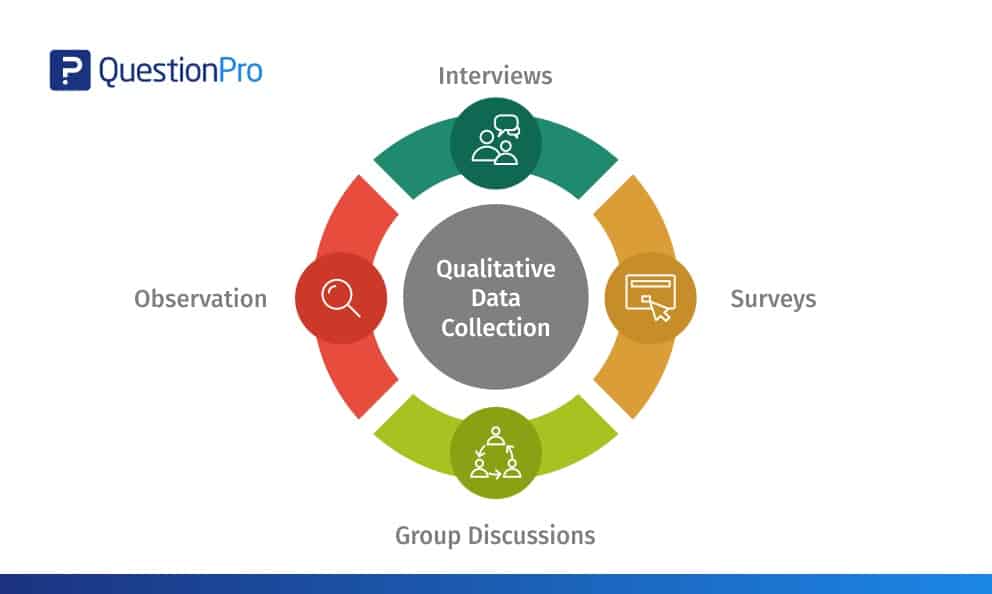
Qualitative data collection is vital in qualitative research. It helps researchers understand individuals’ attitudes, beliefs, and behaviors in a specific context.
Several methods are used to collect qualitative data, including interviews, surveys, focus groups, and observations. Understanding the various methods used for gathering qualitative data is essential for successful qualitative research.
In this post, we will discuss qualitative data and its collection methods of it.
Content Index
What is Qualitative Data?
What is qualitative data collection, what is the need for qualitative data collection, effective qualitative data collection methods, qualitative data analysis, advantages of qualitative data collection.
Qualitative data is defined as data that approximates and characterizes. It can be observed and recorded.
This data type is non-numerical in nature. This type of data is collected through methods of observations, one-to-one interviews, conducting focus groups, and similar methods.
Qualitative data in statistics is also known as categorical data – data that can be arranged categorically based on the attributes and properties of a thing or a phenomenon.
It’s pretty easy to understand the difference between qualitative and quantitative data. Qualitative data does not include numbers in its definition of traits, whereas quantitative research data is all about numbers.
- The cake is orange, blue, and black in color (qualitative).
- Females have brown, black, blonde, and red hair (qualitative).
Qualitative data collection is gathering non-numerical information, such as words, images, and observations, to understand individuals’ attitudes, behaviors, beliefs, and motivations in a specific context. It is an approach used in qualitative research. It seeks to understand social phenomena through in-depth exploration and analysis of people’s perspectives, experiences, and narratives. In statistical analysis , distinguishing between categorical data and numerical data is essential, as categorical data involves distinct categories or labels, while numerical data consists of measurable quantities.
The data collected through qualitative methods are often subjective, open-ended, and unstructured and can provide a rich and nuanced understanding of complex social phenomena.
Qualitative research is a type of study carried out with a qualitative approach to understand the exploratory reasons and to assay how and why a specific program or phenomenon operates in the way it is working. A researcher can access numerous qualitative data collection methods that he/she feels are relevant.
LEARN ABOUT: Best Data Collection Tools
Qualitative data collection methods serve the primary purpose of collecting textual data for research and analysis , like the thematic analysis. The collected research data is used to examine:
- Knowledge around a specific issue or a program, experience of people.
- Meaning and relationships.
- Social norms and contextual or cultural practices demean people or impact a cause.
The qualitative data is textual or non-numerical. It covers mostly the images, videos, texts, and written or spoken words by the people. You can opt for any digital data collection methods , like structured or semi-structured surveys, or settle for the traditional approach comprising individual interviews, group discussions, etc.
Data at hand leads to a smooth process ensuring all the decisions made are for the business’s betterment. You will be able to make informed decisions only if you have relevant data.
Well! With quality data, you will improve the quality of decision-making. But you will also enhance the quality of the results expected from any endeavor.
Qualitative data collection methods are exploratory. Those are usually more focused on gaining insights and understanding the underlying reasons by digging deeper.
Although quantitative data cannot be quantified, measuring it or analyzing qualitative data might become an issue. Due to the lack of measurability, collection methods of qualitative data are primarily unstructured or structured in rare cases – that too to some extent.
Let’s explore the most common methods used for the collection of qualitative data:
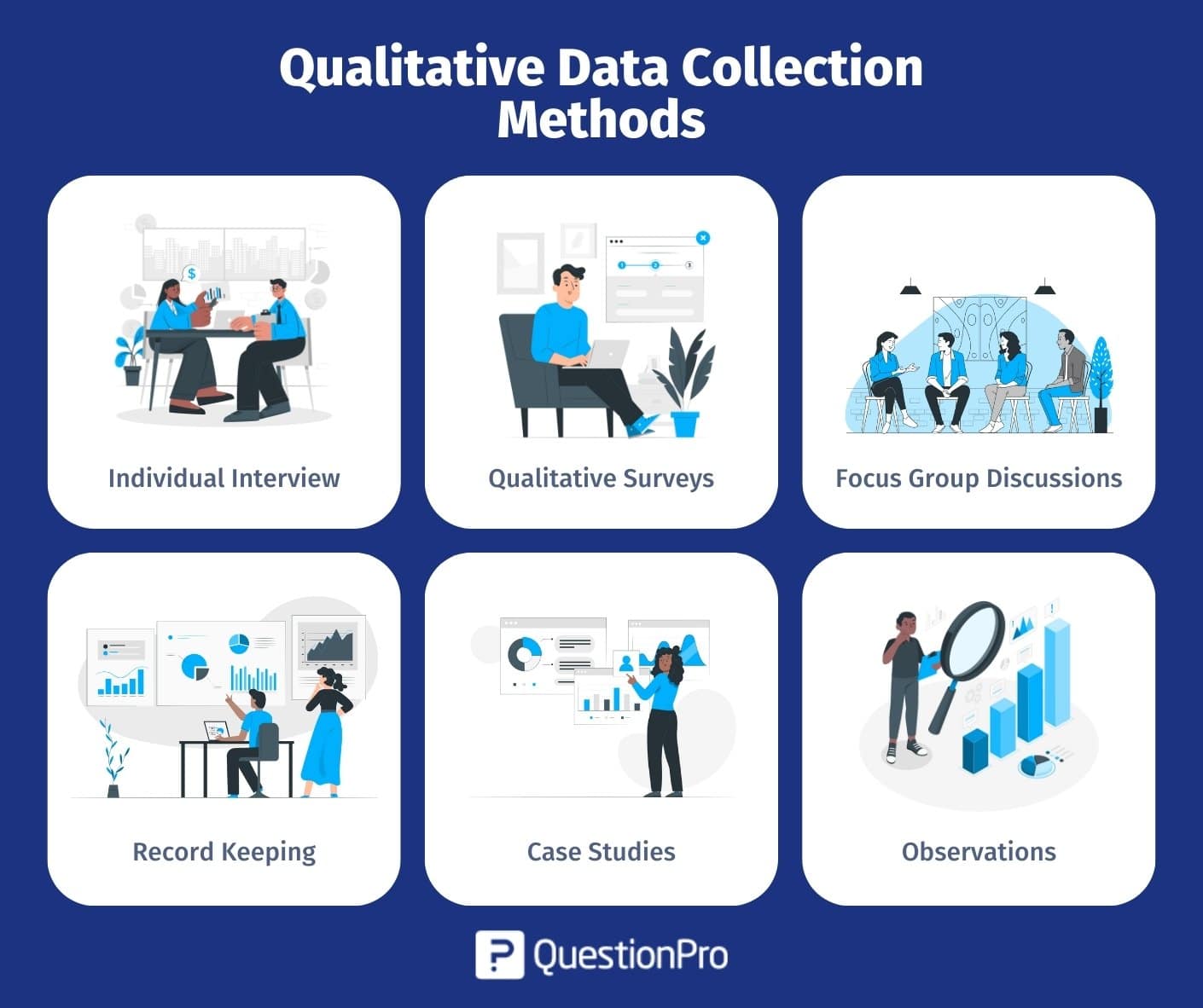
Individual interview
It is one of the most trusted, widely used, and familiar qualitative data collection methods primarily because of its approach. An individual or face-to-face interview is a direct conversation between two people with a specific structure and purpose.
The interview questionnaire is designed in the manner to elicit the interviewee’s knowledge or perspective related to a topic, program, or issue.
At times, depending on the interviewer’s approach, the conversation can be unstructured or informal but focused on understanding the individual’s beliefs, values, understandings, feelings, experiences, and perspectives on an issue.
More often, the interviewer chooses to ask open-ended questions in individual interviews. If the interviewee selects answers from a set of given options, it becomes a structured, fixed response or a biased discussion.
The individual interview is an ideal qualitative data collection method. Particularly when the researchers want highly personalized information from the participants. The individual interview is a notable method if the interviewer decides to probe further and ask follow-up questions to gain more insights.
Qualitative surveys
To develop an informed hypothesis, many researchers use qualitative research surveys for data collection or to collect a piece of detailed information about a product or an issue. If you want to create questionnaires for collecting textual or qualitative data, then ask more open-ended questions .
LEARN ABOUT: Research Process Steps
To answer such qualitative research questions , the respondent has to write his/her opinion or perspective concerning a specific topic or issue. Unlike other collection methods, online surveys have a wider reach. People can provide you with quality data that is highly credible and valuable.
Paper surveys
Online surveys, focus group discussions.
Focus group discussions can also be considered a type of interview, but it is conducted in a group discussion setting. Usually, the focus group consists of 8 – 10 people (the size may vary depending on the researcher’s requirement). The researchers ensure appropriate space is given to the participants to discuss a topic or issue in a context. The participants are allowed to either agree or disagree with each other’s comments.
With a focused group discussion, researchers know how a particular group of participants perceives the topic. Researchers analyze what participants think of an issue, the range of opinions expressed, and the ideas discussed. The data is collected by noting down the variations or inconsistencies (if any exist) in the participants, especially in terms of belief, experiences, and practice.
The participants of focused group discussions are selected based on the topic or issues for which the researcher wants actionable insights. For example, if the research is about the recovery of college students from drug addiction. The participants have to be college students studying and recovering from drug addiction.
Other parameters such as age, qualification, financial background, social presence, and demographics are also considered, but not primarily, as the group needs diverse participants. Frequently, the qualitative data collected through focused group discussion is more descriptive and highly detailed.
Record keeping
This method uses reliable documents and other sources of information that already exist as the data source. This information can help with the new study. It’s a lot like going to the library. There, you can look through books and other sources to find information that can be used in your research.
Case studies
In this method, data is collected by looking at case studies in detail. This method’s flexibility is shown by the fact that it can be used to analyze both simple and complicated topics. This method’s strength is how well it draws conclusions from a mix of one or more qualitative data collection methods.
Observations
Observation is one of the traditional methods of qualitative data collection. It is used by researchers to gather descriptive analysis data by observing people and their behavior at events or in their natural settings. In this method, the researcher is completely immersed in watching people by taking a participatory stance to take down notes.
There are two main types of observation:
- Covert: In this method, the observer is concealed without letting anyone know that they are being observed. For example, a researcher studying the rituals of a wedding in nomadic tribes must join them as a guest and quietly see everything.
- Overt: In this method, everyone is aware that they are being watched. For example, A researcher or an observer wants to study the wedding rituals of a nomadic tribe. To proceed with the research, the observer or researcher can reveal why he is attending the marriage and even use a video camera to shoot everything around him.
Observation is a useful method of qualitative data collection, especially when you want to study the ongoing process, situation, or reactions on a specific issue related to the people being observed.
When you want to understand people’s behavior or their way of interaction in a particular community or demographic, you can rely on the observation data. Remember, if you fail to get quality data through surveys, qualitative interviews , or group discussions, rely on observation.
It is the best and most trusted collection method of qualitative data to generate qualitative data as it requires equal to no effort from the participants.
LEARN ABOUT: Behavioral Research
You invested time and money acquiring your data, so analyze it. It’s necessary to avoid being in the dark after all your hard work. Qualitative data analysis starts with knowing its two basic techniques, but there are no rules.
- Deductive Approach: The deductive data analysis uses a researcher-defined structure to analyze qualitative data. This method is quick and easy when a researcher knows what the sample population will say.
- Inductive Approach: The inductive technique has no structure or framework. When a researcher knows little about the event, an inductive approach is applied.
Whether you want to analyze qualitative data from a one-on-one interview or a survey, these simple steps will ensure a comprehensive qualitative data analysis.
Step 1: Arrange your Data
After collecting all the data, it is mostly unstructured and sometimes unclear. Arranging your data is the first stage in qualitative data analysis. So, researchers must transcribe data before analyzing it.
Step 2: Organize all your Data
After transforming and arranging your data, the next step is to organize it. One of the best ways to organize the data is to think back to your research goals and then organize the data based on the research questions you asked.
Step 3: Set a Code to the Data Collected
Setting up appropriate codes for the collected data gets you one step closer. Coding is one of the most effective methods for compressing a massive amount of data. It allows you to derive theories from relevant research findings.
Step 4: Validate your Data
Qualitative data analysis success requires data validation. Data validation should be done throughout the research process, not just once. There are two sides to validating data:
- The accuracy of your research design or methods.
- Reliability—how well the approaches deliver accurate data.
Step 5: Concluding the Analysis Process
Finally, conclude your data in a presentable report. The report should describe your research methods, their pros and cons, and research limitations. Your report should include findings, inferences, and future research.
QuestionPro is a comprehensive online survey software that offers a variety of qualitative data analysis tools to help businesses and researchers in making sense of their data. Users can use many different qualitative analysis methods to learn more about their data.
Users of QuestionPro can see their data in different charts and graphs, which makes it easier to spot patterns and trends. It can help researchers and businesses learn more about their target audience, which can lead to better decisions and better results.
LEARN ABOUT: Steps in Qualitative Research
Qualitative data collection has several advantages, including:
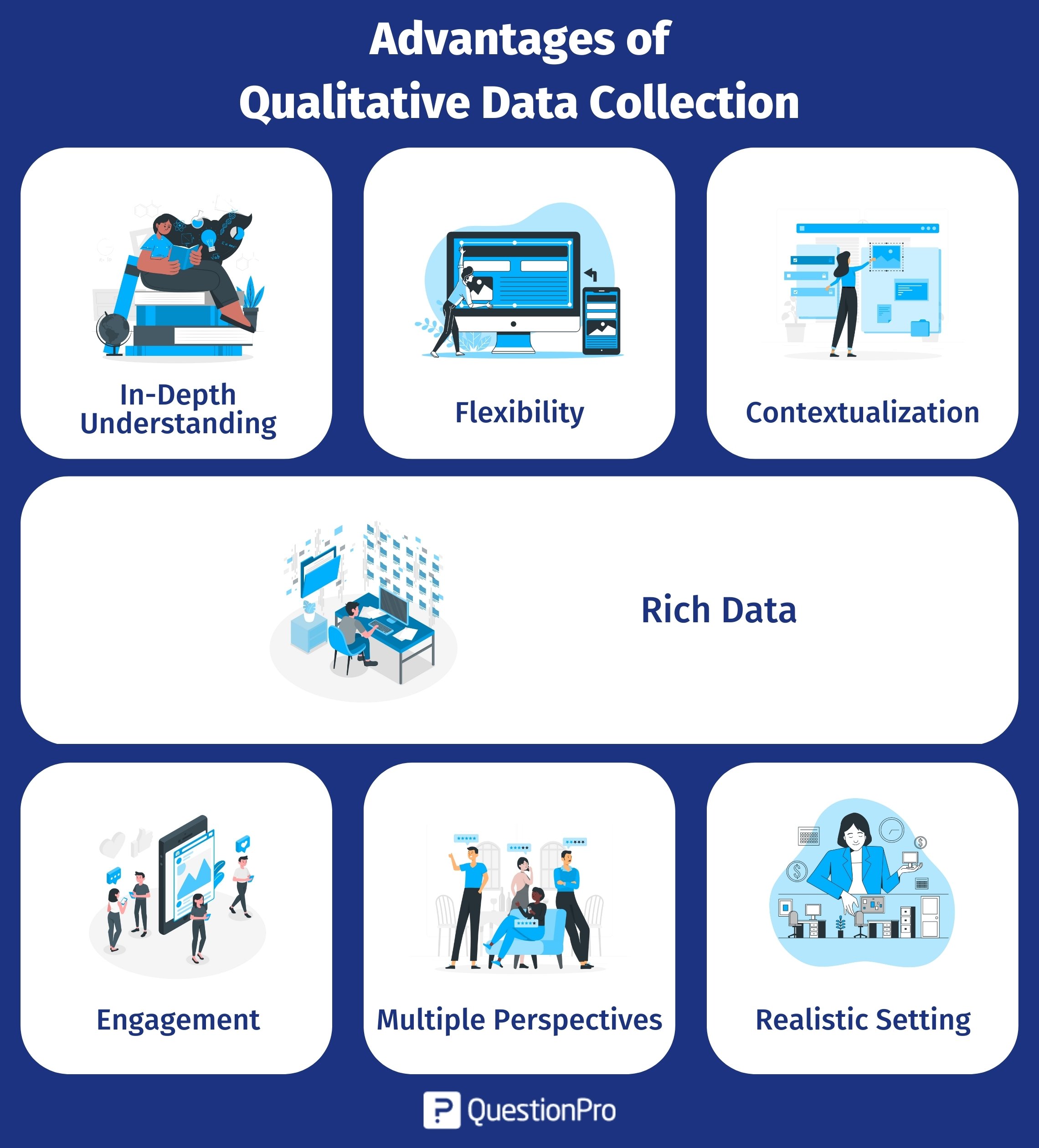
- In-depth understanding: It provides in-depth information about attitudes and behaviors, leading to a deeper understanding of the research.
- Flexibility: The methods allow researchers to modify questions or change direction if new information emerges.
- Contextualization: Qualitative research data is in context, which helps to provide a deep understanding of the experiences and perspectives of individuals.
- Rich data: It often produces rich, detailed, and nuanced information that cannot capture through numerical data.
- Engagement: The methods, such as interviews and focus groups, involve active meetings with participants, leading to a deeper understanding.
- Multiple perspectives: This can provide various views and a rich array of voices, adding depth and complexity.
- Realistic setting: It often occurs in realistic settings, providing more authentic experiences and behaviors.
LEARN ABOUT: 12 Best Tools for Researchers
Qualitative research is one of the best methods for identifying the behavior and patterns governing social conditions, issues, or topics. It spans a step ahead of quantitative data as it fails to explain the reasons and rationale behind a phenomenon, but qualitative data quickly does.
Qualitative research is one of the best tools to identify behaviors and patterns governing social conditions. It goes a step beyond quantitative data by providing the reasons and rationale behind a phenomenon that cannot be explored quantitatively.
With QuestionPro, you can use it for qualitative data collection through various methods. Using Our robust suite correctly, you can enhance the quality and integrity of the collected data.
LEARN MORE FREE TRIAL
MORE LIKE THIS
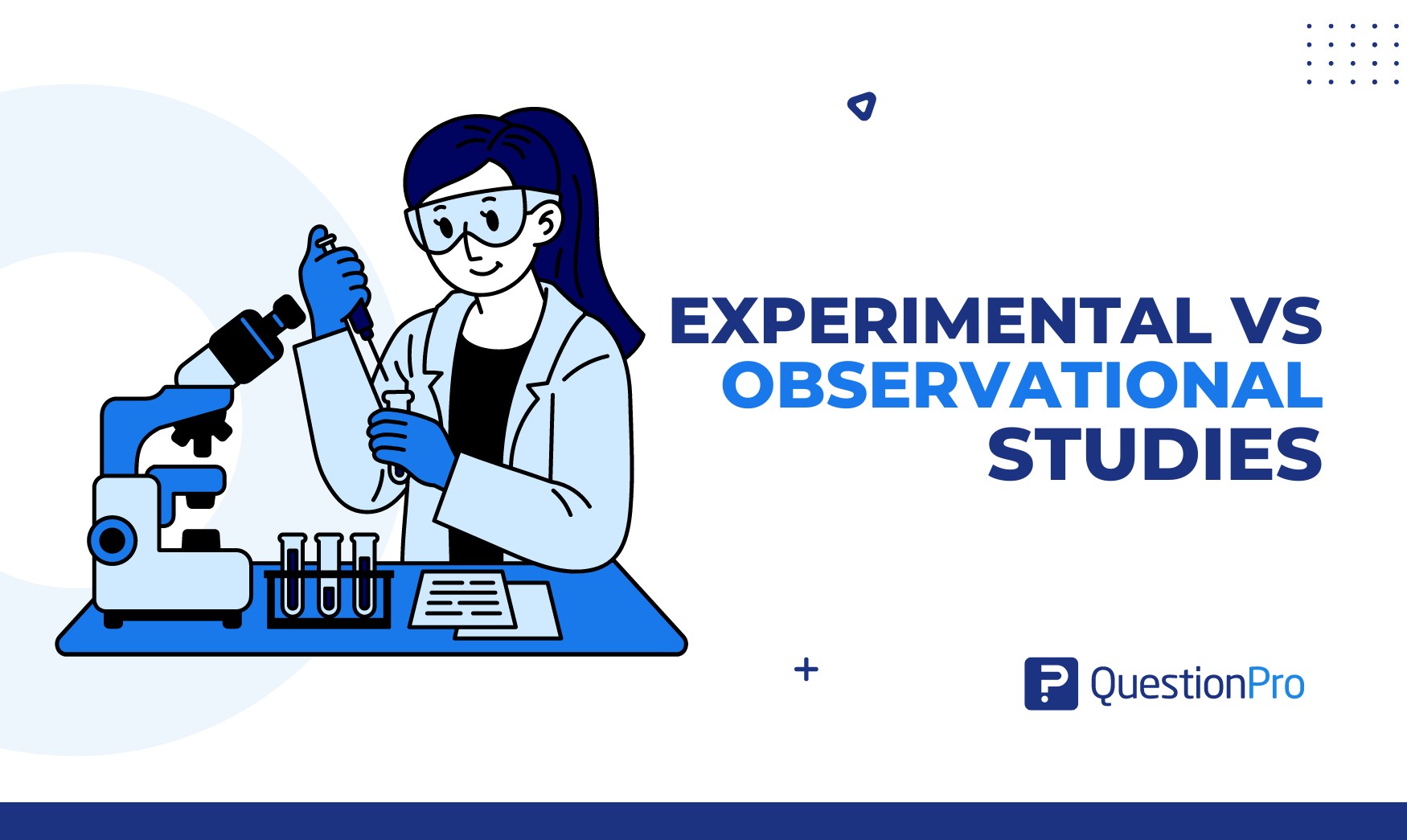
Experimental vs Observational Studies: Differences & Examples
Sep 5, 2024
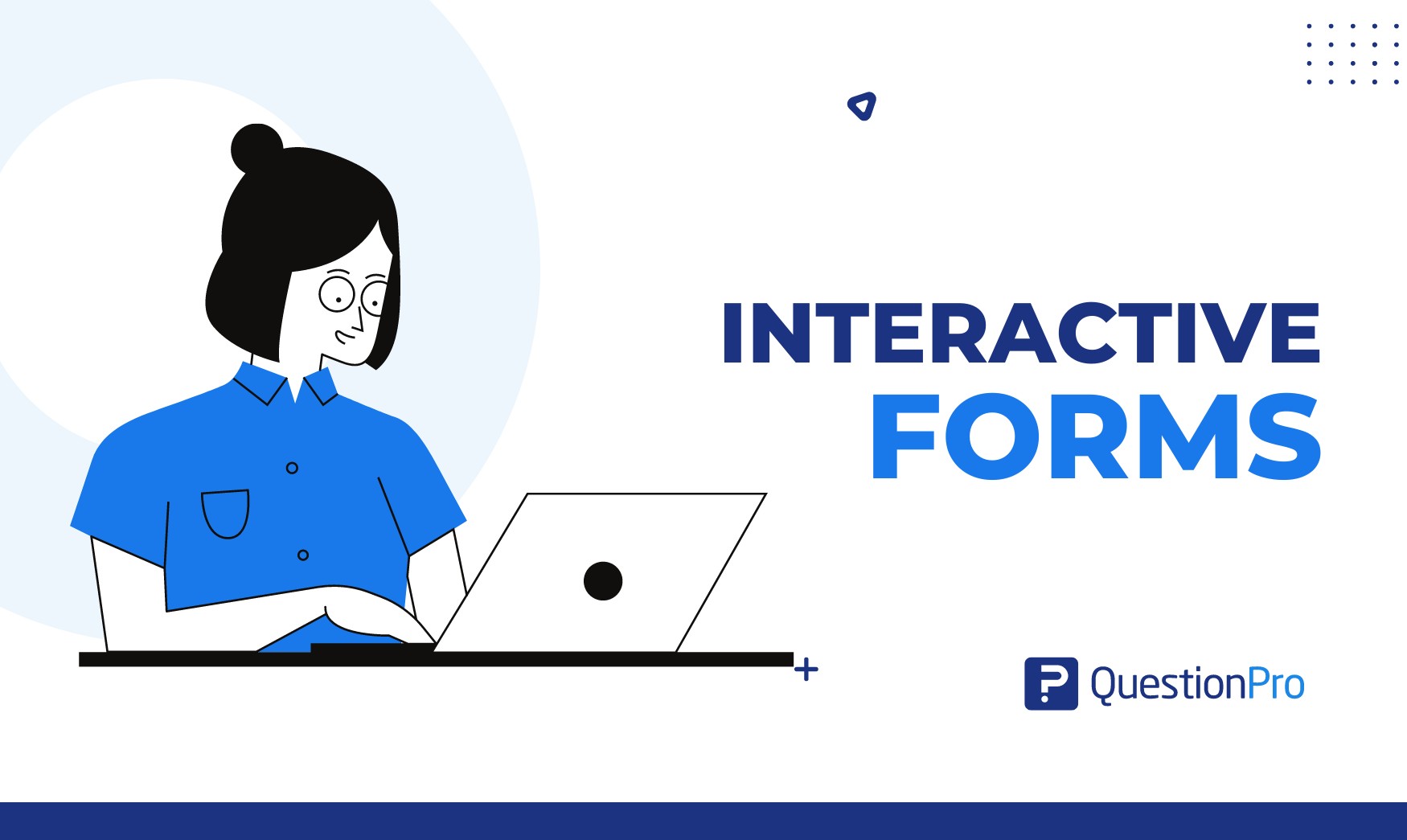
Interactive Forms: Key Features, Benefits, Uses + Design Tips
Sep 4, 2024
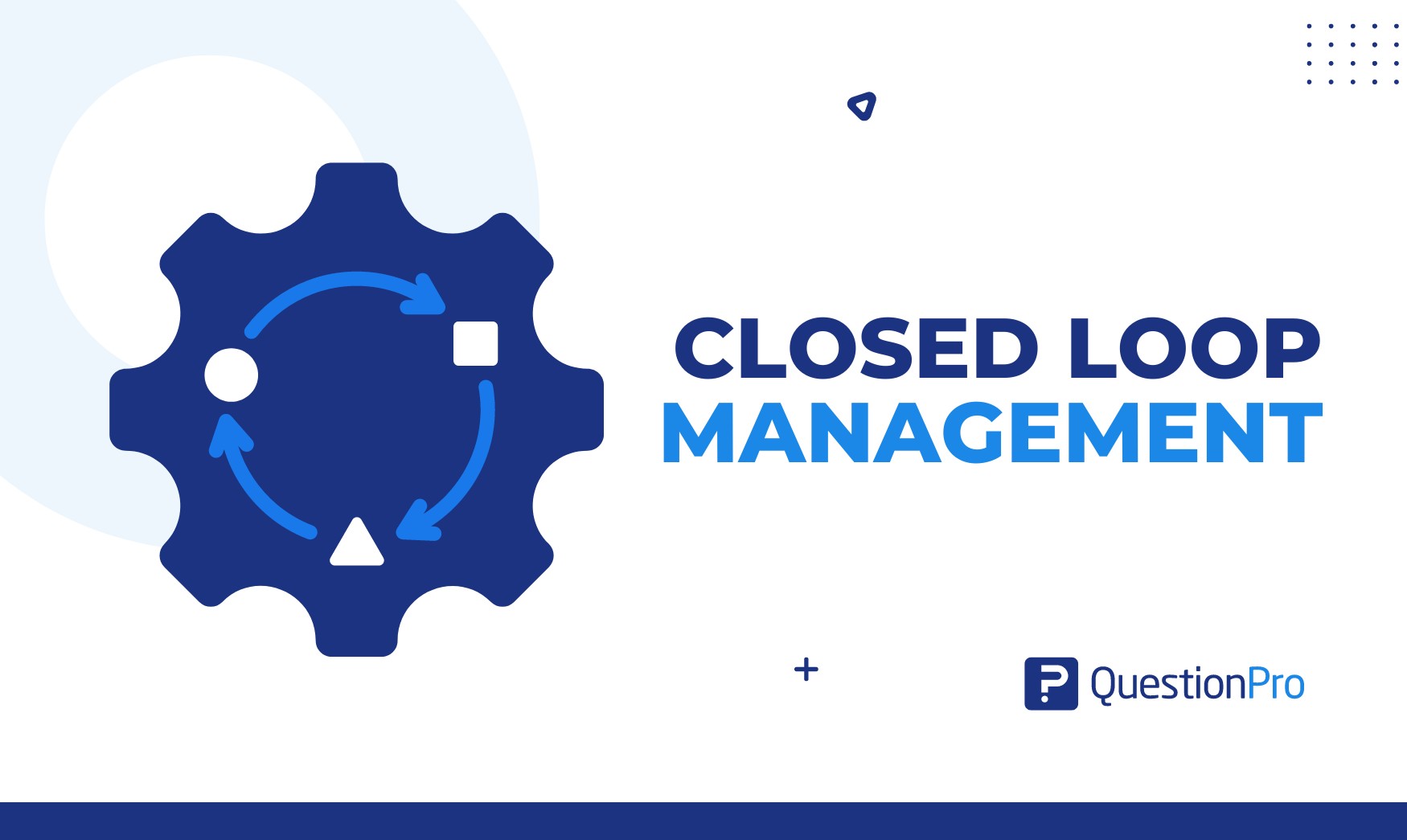
Closed-Loop Management: The Key to Customer Centricity
Sep 3, 2024
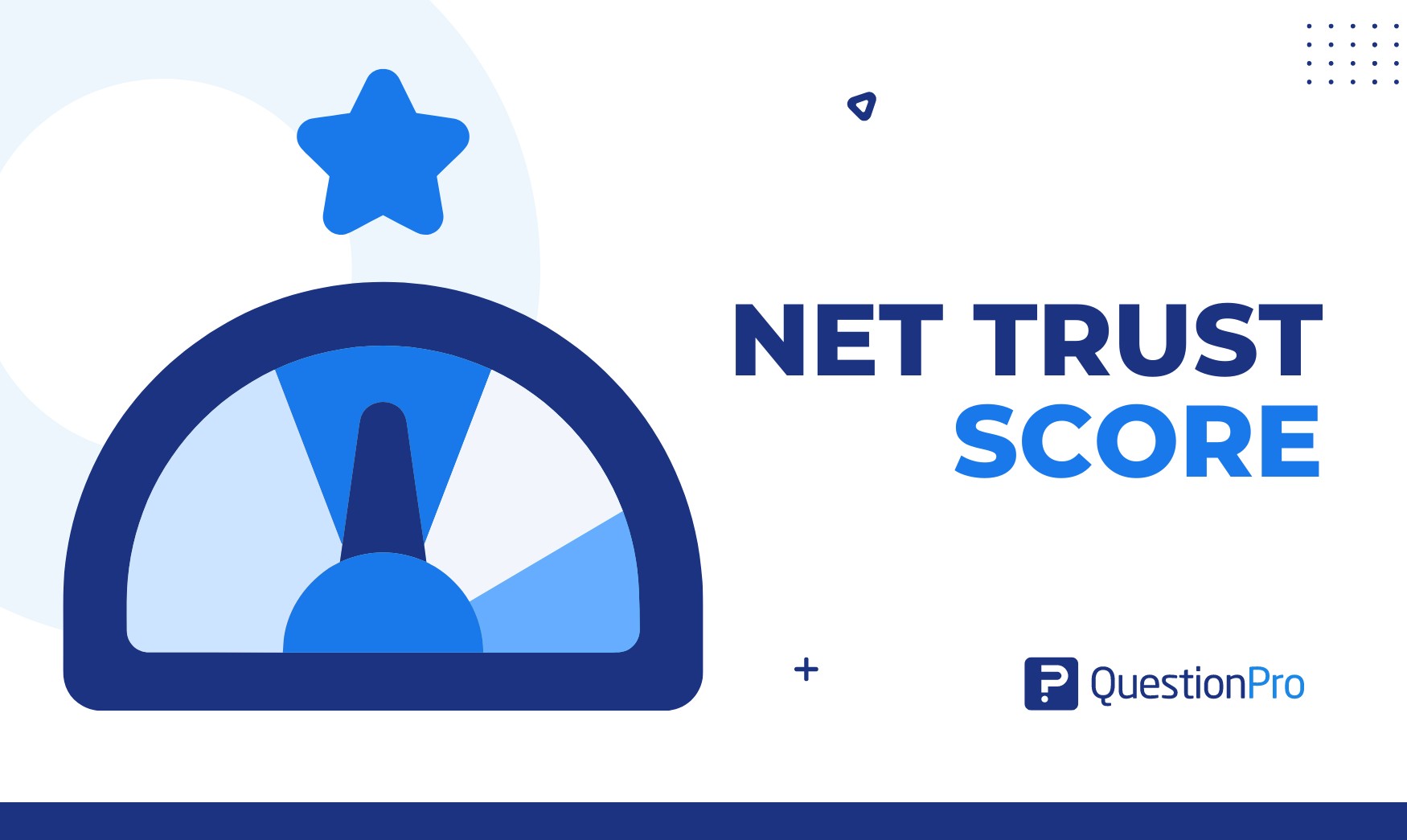
Net Trust Score: Tool for Measuring Trust in Organization
Sep 2, 2024
Other categories
- Academic Research
- Artificial Intelligence
- Assessments
- Brand Awareness
- Case Studies
- Communities
- Consumer Insights
- Customer effort score
- Customer Engagement
- Customer Experience
- Customer Loyalty
- Customer Research
- Customer Satisfaction
- Employee Benefits
- Employee Engagement
- Employee Retention
- Friday Five
- General Data Protection Regulation
- Insights Hub
- Life@QuestionPro
- Market Research
- Mobile diaries
- Mobile Surveys
- New Features
- Online Communities
- Question Types
- Questionnaire
- QuestionPro Products
- Release Notes
- Research Tools and Apps
- Revenue at Risk
- Survey Templates
- Training Tips
- Tuesday CX Thoughts (TCXT)
- Uncategorized
- What’s Coming Up
- Workforce Intelligence
Thank you for visiting nature.com. You are using a browser version with limited support for CSS. To obtain the best experience, we recommend you use a more up to date browser (or turn off compatibility mode in Internet Explorer). In the meantime, to ensure continued support, we are displaying the site without styles and JavaScript.
- View all journals
- Explore content
- About the journal
- Publish with us
- Sign up for alerts
- Published: 22 March 2008
Methods of data collection in qualitative research: interviews and focus groups
- P. Gill 1 ,
- K. Stewart 2 ,
- E. Treasure 3 &
- B. Chadwick 4
British Dental Journal volume 204 , pages 291–295 ( 2008 ) Cite this article
1.70m Accesses
1066 Citations
46 Altmetric
Metrics details
Interviews and focus groups are the most common methods of data collection used in qualitative healthcare research
Interviews can be used to explore the views, experiences, beliefs and motivations of individual participants
Focus group use group dynamics to generate qualitative data
Qualitative research in dentistry
Conducting qualitative interviews with school children in dental research
Analysing and presenting qualitative data
This paper explores the most common methods of data collection used in qualitative research: interviews and focus groups. The paper examines each method in detail, focusing on how they work in practice, when their use is appropriate and what they can offer dentistry. Examples of empirical studies that have used interviews or focus groups are also provided.
You have full access to this article via your institution.
Similar content being viewed by others
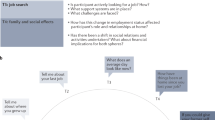
Interviews in the social sciences
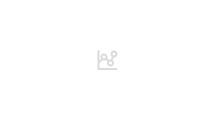
A review of technical and quality assessment considerations of audio-visual and web-conferencing focus groups in qualitative health research
Professionalism in dentistry: deconstructing common terminology, introduction.
Having explored the nature and purpose of qualitative research in the previous paper, this paper explores methods of data collection used in qualitative research. There are a variety of methods of data collection in qualitative research, including observations, textual or visual analysis (eg from books or videos) and interviews (individual or group). 1 However, the most common methods used, particularly in healthcare research, are interviews and focus groups. 2 , 3
The purpose of this paper is to explore these two methods in more detail, in particular how they work in practice, the purpose of each, when their use is appropriate and what they can offer dental research.
Qualitative research interviews
There are three fundamental types of research interviews: structured, semi-structured and unstructured. Structured interviews are, essentially, verbally administered questionnaires, in which a list of predetermined questions are asked, with little or no variation and with no scope for follow-up questions to responses that warrant further elaboration. Consequently, they are relatively quick and easy to administer and may be of particular use if clarification of certain questions are required or if there are likely to be literacy or numeracy problems with the respondents. However, by their very nature, they only allow for limited participant responses and are, therefore, of little use if 'depth' is required.
Conversely, unstructured interviews do not reflect any preconceived theories or ideas and are performed with little or no organisation. 4 Such an interview may simply start with an opening question such as 'Can you tell me about your experience of visiting the dentist?' and will then progress based, primarily, upon the initial response. Unstructured interviews are usually very time-consuming (often lasting several hours) and can be difficult to manage, and to participate in, as the lack of predetermined interview questions provides little guidance on what to talk about (which many participants find confusing and unhelpful). Their use is, therefore, generally only considered where significant 'depth' is required, or where virtually nothing is known about the subject area (or a different perspective of a known subject area is required).
Semi-structured interviews consist of several key questions that help to define the areas to be explored, but also allows the interviewer or interviewee to diverge in order to pursue an idea or response in more detail. 2 This interview format is used most frequently in healthcare, as it provides participants with some guidance on what to talk about, which many find helpful. The flexibility of this approach, particularly compared to structured interviews, also allows for the discovery or elaboration of information that is important to participants but may not have previously been thought of as pertinent by the research team.
For example, in a recent dental public heath study, 5 school children in Cardiff, UK were interviewed about their food choices and preferences. A key finding that emerged from semi-structured interviews, which was not previously thought to be as highly influential as the data subsequently confirmed, was the significance of peer-pressure in influencing children's food choices and preferences. This finding was also established primarily through follow-up questioning (eg probing interesting responses with follow-up questions, such as 'Can you tell me a bit more about that?') and, therefore, may not have emerged in the same way, if at all, if asked as a predetermined question.
The purpose of research interviews
The purpose of the research interview is to explore the views, experiences, beliefs and/or motivations of individuals on specific matters (eg factors that influence their attendance at the dentist). Qualitative methods, such as interviews, are believed to provide a 'deeper' understanding of social phenomena than would be obtained from purely quantitative methods, such as questionnaires. 1 Interviews are, therefore, most appropriate where little is already known about the study phenomenon or where detailed insights are required from individual participants. They are also particularly appropriate for exploring sensitive topics, where participants may not want to talk about such issues in a group environment.
Examples of dental studies that have collected data using interviews are 'Examining the psychosocial process involved in regular dental attendance' 6 and 'Exploring factors governing dentists' treatment philosophies'. 7 Gibson et al . 6 provided an improved understanding of factors that influenced people's regular attendance with their dentist. The study by Kay and Blinkhorn 7 provided a detailed insight into factors that influenced GDPs' decision making in relation to treatment choices. The study found that dentists' clinical decisions about treatments were not necessarily related to pathology or treatment options, as was perhaps initially thought, but also involved discussions with patients, patients' values and dentists' feelings of self esteem and conscience.
There are many similarities between clinical encounters and research interviews, in that both employ similar interpersonal skills, such as questioning, conversing and listening. However, there are also some fundamental differences between the two, such as the purpose of the encounter, reasons for participating, roles of the people involved and how the interview is conducted and recorded. 8
The primary purpose of clinical encounters is for the dentist to ask the patient questions in order to acquire sufficient information to inform decision making and treatment options. However, the constraints of most consultations are such that any open-ended questioning needs to be brought to a conclusion within a fairly short time. 2 In contrast, the fundamental purpose of the research interview is to listen attentively to what respondents have to say, in order to acquire more knowledge about the study topic. 9 Unlike the clinical encounter, it is not to intentionally offer any form of help or advice, which many researchers have neither the training nor the time for. Research interviewing therefore requires a different approach and a different range of skills.
The interview
When designing an interview schedule it is imperative to ask questions that are likely to yield as much information about the study phenomenon as possible and also be able to address the aims and objectives of the research. In a qualitative interview, good questions should be open-ended (ie, require more than a yes/no answer), neutral, sensitive and understandable. 2 It is usually best to start with questions that participants can answer easily and then proceed to more difficult or sensitive topics. 2 This can help put respondents at ease, build up confidence and rapport and often generates rich data that subsequently develops the interview further.
As in any research, it is often wise to first pilot the interview schedule on several respondents prior to data collection proper. 8 This allows the research team to establish if the schedule is clear, understandable and capable of answering the research questions, and if, therefore, any changes to the interview schedule are required.
The length of interviews varies depending on the topic, researcher and participant. However, on average, healthcare interviews last 20-60 minutes. Interviews can be performed on a one-off or, if change over time is of interest, repeated basis, 4 for example exploring the psychosocial impact of oral trauma on participants and their subsequent experiences of cosmetic dental surgery.
Developing the interview
Before an interview takes place, respondents should be informed about the study details and given assurance about ethical principles, such as anonymity and confidentiality. 2 This gives respondents some idea of what to expect from the interview, increases the likelihood of honesty and is also a fundamental aspect of the informed consent process.
Wherever possible, interviews should be conducted in areas free from distractions and at times and locations that are most suitable for participants. For many this may be at their own home in the evenings. Whilst researchers may have less control over the home environment, familiarity may help the respondent to relax and result in a more productive interview. 9 Establishing rapport with participants prior to the interview is also important as this can also have a positive effect on the subsequent development of the interview.
When conducting the actual interview it is prudent for the interviewer to familiarise themselves with the interview schedule, so that the process appears more natural and less rehearsed. However, to ensure that the interview is as productive as possible, researchers must possess a repertoire of skills and techniques to ensure that comprehensive and representative data are collected during the interview. 10 One of the most important skills is the ability to listen attentively to what is being said, so that participants are able to recount their experiences as fully as possible, without unnecessary interruptions.
Other important skills include adopting open and emotionally neutral body language, nodding, smiling, looking interested and making encouraging noises (eg, 'Mmmm') during the interview. 2 The strategic use of silence, if used appropriately, can also be highly effective at getting respondents to contemplate their responses, talk more, elaborate or clarify particular issues. Other techniques that can be used to develop the interview further include reflecting on remarks made by participants (eg, 'Pain?') and probing remarks ('When you said you were afraid of going to the dentist what did you mean?'). 9 Where appropriate, it is also wise to seek clarification from respondents if it is unclear what they mean. The use of 'leading' or 'loaded' questions that may unduly influence responses should always be avoided (eg, 'So you think dental surgery waiting rooms are frightening?' rather than 'How do you find the waiting room at the dentists?').
At the end of the interview it is important to thank participants for their time and ask them if there is anything they would like to add. This gives respondents an opportunity to deal with issues that they have thought about, or think are important but have not been dealt with by the interviewer. 9 This can often lead to the discovery of new, unanticipated information. Respondents should also be debriefed about the study after the interview has finished.
All interviews should be tape recorded and transcribed verbatim afterwards, as this protects against bias and provides a permanent record of what was and was not said. 8 It is often also helpful to make 'field notes' during and immediately after each interview about observations, thoughts and ideas about the interview, as this can help in data analysis process. 4 , 8
Focus groups
Focus groups share many common features with less structured interviews, but there is more to them than merely collecting similar data from many participants at once. A focus group is a group discussion on a particular topic organised for research purposes. This discussion is guided, monitored and recorded by a researcher (sometimes called a moderator or facilitator). 11 , 12
Focus groups were first used as a research method in market research, originating in the 1940s in the work of the Bureau of Applied Social Research at Columbia University. Eventually the success of focus groups as a marketing tool in the private sector resulted in its use in public sector marketing, such as the assessment of the impact of health education campaigns. 13 However, focus group techniques, as used in public and private sectors, have diverged over time. Therefore, in this paper, we seek to describe focus groups as they are used in academic research.
When focus groups are used
Focus groups are used for generating information on collective views, and the meanings that lie behind those views. They are also useful in generating a rich understanding of participants' experiences and beliefs. 12 Suggested criteria for using focus groups include: 13
As a standalone method, for research relating to group norms, meanings and processes
In a multi-method design, to explore a topic or collect group language or narratives to be used in later stages
To clarify, extend, qualify or challenge data collected through other methods
To feedback results to research participants.
Morgan 12 suggests that focus groups should be avoided according to the following criteria:
If listening to participants' views generates expectations for the outcome of the research that can not be fulfilled
If participants are uneasy with each other, and will therefore not discuss their feelings and opinions openly
If the topic of interest to the researcher is not a topic the participants can or wish to discuss
If statistical data is required. Focus groups give depth and insight, but cannot produce useful numerical results.
Conducting focus groups: group composition and size
The composition of a focus group needs great care to get the best quality of discussion. There is no 'best' solution to group composition, and group mix will always impact on the data, according to things such as the mix of ages, sexes and social professional statuses of the participants. What is important is that the researcher gives due consideration to the impact of group mix (eg, how the group may interact with each other) before the focus group proceeds. 14
Interaction is key to a successful focus group. Sometimes this means a pre-existing group interacts best for research purposes, and sometimes stranger groups. Pre-existing groups may be easier to recruit, have shared experiences and enjoy a comfort and familiarity which facilitates discussion or the ability to challenge each other comfortably. In health settings, pre-existing groups can overcome issues relating to disclosure of potentially stigmatising status which people may find uncomfortable in stranger groups (conversely there may be situations where disclosure is more comfortable in stranger groups). In other research projects it may be decided that stranger groups will be able to speak more freely without fear of repercussion, and challenges to other participants may be more challenging and probing, leading to richer data. 13
Group size is an important consideration in focus group research. Stewart and Shamdasani 14 suggest that it is better to slightly over-recruit for a focus group and potentially manage a slightly larger group, than under-recruit and risk having to cancel the session or having an unsatisfactory discussion. They advise that each group will probably have two non-attenders. The optimum size for a focus group is six to eight participants (excluding researchers), but focus groups can work successfully with as few as three and as many as 14 participants. Small groups risk limited discussion occurring, while large groups can be chaotic, hard to manage for the moderator and frustrating for participants who feel they get insufficient opportunities to speak. 13
Preparing an interview schedule
Like research interviews, the interview schedule for focus groups is often no more structured than a loose schedule of topics to be discussed. However, in preparing an interview schedule for focus groups, Stewart and Shamdasani 14 suggest two general principles:
Questions should move from general to more specific questions
Question order should be relative to importance of issues in the research agenda.
There can, however, be some conflict between these two principles, and trade offs are often needed, although often discussions will take on a life of their own, which will influence or determine the order in which issues are covered. Usually, less than a dozen predetermined questions are needed and, as with research interviews, the researcher will also probe and expand on issues according to the discussion.
Moderating a focus group looks easy when done well, but requires a complex set of skills, which are related to the following principles: 15
Participants have valuable views and the ability to respond actively, positively and respectfully. Such an approach is not simply a courtesy, but will encourage fruitful discussions
Moderating without participating: a moderator must guide a discussion rather than join in with it. Expressing one's own views tends to give participants cues as to what to say (introducing bias), rather than the confidence to be open and honest about their own views
Be prepared for views that may be unpalatably critical of a topic which may be important to you
It is important to recognise that researchers' individual characteristics mean that no one person will always be suitable to moderate any kind of group. Sometimes the characteristics that suit a moderator for one group will inhibit discussion in another
Be yourself. If the moderator is comfortable and natural, participants will feel relaxed.
The moderator should facilitate group discussion, keeping it focussed without leading it. They should also be able to prevent the discussion being dominated by one member (for example, by emphasising at the outset the importance of hearing a range of views), ensure that all participants have ample opportunity to contribute, allow differences of opinions to be discussed fairly and, if required, encourage reticent participants. 13
Other relevant factors
The venue for a focus group is important and should, ideally, be accessible, comfortable, private, quiet and free from distractions. 13 However, while a central location, such as the participants' workplace or school, may encourage attendance, the venue may affect participants' behaviour. For example, in a school setting, pupils may behave like pupils, and in clinical settings, participants may be affected by any anxieties that affect them when they attend in a patient role.
Focus groups are usually recorded, often observed (by a researcher other than the moderator, whose role is to observe the interaction of the group to enhance analysis) and sometimes videotaped. At the start of a focus group, a moderator should acknowledge the presence of the audio recording equipment, assure participants of confidentiality and give people the opportunity to withdraw if they are uncomfortable with being taped. 14
A good quality multi-directional external microphone is recommended for the recording of focus groups, as internal microphones are rarely good enough to cope with the variation in volume of different speakers. 13 If observers are present, they should be introduced to participants as someone who is just there to observe, and sit away from the discussion. 14 Videotaping will require more than one camera to capture the whole group, as well as additional operational personnel in the room. This is, therefore, very obtrusive, which can affect the spontaneity of the group and in a focus group does not usually yield enough additional information that could not be captured by an observer to make videotaping worthwhile. 15
The systematic analysis of focus group transcripts is crucial. However, the transcription of focus groups is more complex and time consuming than in one-to-one interviews, and each hour of audio can take up to eight hours to transcribe and generate approximately 100 pages of text. Recordings should be transcribed verbatim and also speakers should be identified in a way that makes it possible to follow the contributions of each individual. Sometimes observational notes also need to be described in the transcripts in order for them to make sense.
The analysis of qualitative data is explored in the final paper of this series. However, it is important to note that the analysis of focus group data is different from other qualitative data because of their interactive nature, and this needs to be taken into consideration during analysis. The importance of the context of other speakers is essential to the understanding of individual contributions. 13 For example, in a group situation, participants will often challenge each other and justify their remarks because of the group setting, in a way that perhaps they would not in a one-to-one interview. The analysis of focus group data must therefore take account of the group dynamics that have generated remarks.
Focus groups in dental research
Focus groups are used increasingly in dental research, on a diverse range of topics, 16 illuminating a number of areas relating to patients, dental services and the dental profession. Addressing a special needs population difficult to access and sample through quantitative measures, Robinson et al . 17 used focus groups to investigate the oral health-related attitudes of drug users, exploring the priorities, understandings and barriers to care they encounter. Newton et al . 18 used focus groups to explore barriers to services among minority ethnic groups, highlighting for the first time differences between minority ethnic groups. Demonstrating the use of the method with professional groups as subjects in dental research, Gussy et al . 19 explored the barriers to and possible strategies for developing a shared approach in prevention of caries among pre-schoolers. This mixed method study was very important as the qualitative element was able to explain why the clinical trial failed, and this understanding may help researchers improve on the quantitative aspect of future studies, as well as making a valuable academic contribution in its own right.
Interviews and focus groups remain the most common methods of data collection in qualitative research, and are now being used with increasing frequency in dental research, particularly to access areas not amendable to quantitative methods and/or where depth, insight and understanding of particular phenomena are required. The examples of dental studies that have employed these methods also help to demonstrate the range of research contexts to which interview and focus group research can make a useful contribution. The continued employment of these methods can further strengthen many areas of dentally related work.
Silverman D . Doing qualitative research . London: Sage Publications, 2000.
Google Scholar
Britten N . Qualitative interviews in healthcare. In Pope C, Mays N (eds) Qualitative research in health care . 2nd ed. pp 11–19. London: BMJ Books, 1999.
Legard R, Keegan J, Ward K . In-depth interviews. In Ritchie J, Lewis J (eds) Qualitative research practice: a guide for social science students and researchers . pp 139–169. London: Sage Publications, 2003.
May K M . Interview techniques in qualitative research: concerns and challenges. In Morse J M (ed) Qualitative nursing research . pp 187–201. Newbury Park: Sage Publications, 1991.
Stewart K, Gill P, Treasure E, Chadwick B . Understanding about food among 6-11 year olds in South Wales. Food Culture Society 2006; 9 : 317–333.
Article Google Scholar
Gibson B, Drenna J, Hanna S, Freeman R . An exploratory qualitative study examining the social and psychological processes involved in regular dental attendance. J Public Health Dent 2000; 60 : 5–11.
Kay E J, Blinkhorn A S . A qualitative investigation of factors governing dentists' treatment philosophies. Br Dent J 1996; 180 : 171–176.
Pontin D . Interviews. In Cormack D F S (ed) The research process in nursing . 4th ed. pp 289–298. Oxford: Blackwell Science, 2000.
Kvale S . Interviews . Thousand Oaks: Sage Publications, 1996.
Hammersley M, Atkinson P . Ethnography: principles in practice . 2nd ed. London: Routledge, 1995.
Kitzinger J . The methodology of focus groups: the importance of interaction between research participants. Sociol Health Illn 1994; 16 : 103–121.
Morgan D L . The focus group guide book . London: Sage Publications, 1998.
Book Google Scholar
Bloor M, Frankland J, Thomas M, Robson K . Focus groups in social research . London: Sage Publications, 2001.
Stewart D W, Shamdasani P M . Focus groups. Theory and practice . London: Sage Publications, 1990.
Krueger R A . Moderating focus groups . London: Sage Publications, 1998.
Chestnutt I G, Robson K F. Focus groups – what are they? Dent Update 2002; 28 : 189–192.
Robinson P G, Acquah S, Gibson B . Drug users: oral health related attitudes and behaviours. Br Dent J 2005; 198 : 219–224.
Newton J T, Thorogood N, Bhavnani V, Pitt J, Gibbons D E, Gelbier S . Barriers to the use of dental services by individuals from minority ethnic communities living in the United Kingdom: findings from focus groups. Primary Dent Care 2001; 8 : 157–161.
Gussy M G, Waters E, Kilpatrick M . A qualitative study exploring barriers to a model of shared care for pre-school children's oral health. Br Dent J 2006; 201 : 165–170.
Download references
Author information
Authors and affiliations.
Senior Research Fellow, Faculty of Health, Sport and Science, University of Glamorgan, Pontypridd, CF37 1DL,
Research Fellow, Academic Unit of Primary Care, University of Bristol, Bristol, BS8 2AA,
Dean and Professor of Dental Public Health, School of Dentistry, Dental Health and Biological Sciences, School of Dentistry, Cardiff University, Heath Park, Cardiff, CF14 4XY,
E. Treasure
Professor of Paediatric Dentistry, School of Dentistry, Dental Health and Biological Sciences, School of Dentistry, Cardiff University, Heath Park, Cardiff, CF14 4XY,
B. Chadwick
You can also search for this author in PubMed Google Scholar
Corresponding author
Correspondence to P. Gill .
Additional information
Refereed paper
Rights and permissions
Reprints and permissions
About this article
Cite this article.
Gill, P., Stewart, K., Treasure, E. et al. Methods of data collection in qualitative research: interviews and focus groups. Br Dent J 204 , 291–295 (2008). https://doi.org/10.1038/bdj.2008.192
Download citation
Published : 22 March 2008
Issue Date : 22 March 2008
DOI : https://doi.org/10.1038/bdj.2008.192
Share this article
Anyone you share the following link with will be able to read this content:
Sorry, a shareable link is not currently available for this article.
Provided by the Springer Nature SharedIt content-sharing initiative
This article is cited by
Imprisonment for south ethiopian people living with hiv presents a double health burden: lived experiences of prisoners.
- Terefe Gone Fuge
- George Tsourtos
- Emma R Miller
BMC Health Services Research (2024)
Employees’ experiences of a large-scale implementation in a public care setting: a novel mixed-method approach to content analysis
- My Säfström
- Ulrika Löfkvist
Translating brand reputation into equity from the stakeholder’s theory: an approach to value creation based on consumer’s perception & interactions
- Olukorede Adewole
International Journal of Corporate Social Responsibility (2024)
Nurses’ and patients’ perceptions of physical health screening for patients with schizophrenia spectrum disorders: a qualitative study
- Camilla Långstedt
- Daniel Bressington
- Maritta Välimäki
BMC Nursing (2024)
Heteronormative biases and distinctive experiences with prostate cancer among men who have sex with men: a qualitative focus group study
- Evan Panken
- Noah Frydenlund
- Channa Amarasekera
BMC Urology (2024)
Quick links
- Explore articles by subject
- Guide to authors
- Editorial policies

Log in using your username and password
- Search More Search for this keyword Advanced search
- Latest content
- Current issue
- Write for Us
- BMJ Journals
You are here
- Volume 21, Issue 3
- Data collection in qualitative research
- Article Text
- Article info
- Citation Tools
- Rapid Responses
- Article metrics

- David Barrett 1 ,
- http://orcid.org/0000-0003-1130-5603 Alison Twycross 2
- 1 Faculty of Health Sciences , University of Hull , Hull , UK
- 2 School of Health and Social Care , London South Bank University , London , UK
- Correspondence to Dr David Barrett, Faculty of Health Sciences, University of Hull, Hull HU6 7RX, UK; D.I.Barrett{at}hull.ac.uk
https://doi.org/10.1136/eb-2018-102939
Statistics from Altmetric.com
Request permissions.
If you wish to reuse any or all of this article please use the link below which will take you to the Copyright Clearance Center’s RightsLink service. You will be able to get a quick price and instant permission to reuse the content in many different ways.
Qualitative research methods allow us to better understand the experiences of patients and carers; they allow us to explore how decisions are made and provide us with a detailed insight into how interventions may alter care. To develop such insights, qualitative research requires data which are holistic, rich and nuanced, allowing themes and findings to emerge through careful analysis. This article provides an overview of the core approaches to data collection in qualitative research, exploring their strengths, weaknesses and challenges.
Collecting data through interviews with participants is a characteristic of many qualitative studies. Interviews give the most direct and straightforward approach to gathering detailed and rich data regarding a particular phenomenon. The type of interview used to collect data can be tailored to the research question, the characteristics of participants and the preferred approach of the researcher. Interviews are most often carried out face-to-face, though the use of telephone interviews to overcome geographical barriers to participant recruitment is becoming more prevalent. 1
A common approach in qualitative research is the semistructured interview, where core elements of the phenomenon being studied are explicitly asked about by the interviewer. A well-designed semistructured interview should ensure data are captured in key areas while still allowing flexibility for participants to bring their own personality and perspective to the discussion. Finally, interviews can be much more rigidly structured to provide greater control for the researcher, essentially becoming questionnaires where responses are verbal rather than written.
Deciding where to place an interview design on this ‘structural spectrum’ will depend on the question to be answered and the skills of the researcher. A very structured approach is easy to administer and analyse but may not allow the participant to express themselves fully. At the other end of the spectrum, an open approach allows for freedom and flexibility, but requires the researcher to walk an investigative tightrope that maintains the focus of an interview without forcing participants into particular areas of discussion.
Example of an interview schedule 3
What do you think is the most effective way of assessing a child’s pain?
Have you come across any issues that make it difficult to assess a child’s pain?
What pain-relieving interventions do you find most useful and why?
When managing pain in children what is your overall aim?
Whose responsibility is pain management?
What involvement do you think parents should have in their child’s pain management?
What involvement do children have in their pain management?
Is there anything that currently stops you managing pain as well as you would like?
What would help you manage pain better?
Interviews present several challenges to researchers. Most interviews are recorded and will need transcribing before analysing. This can be extremely time-consuming, with 1 hour of interview requiring 5–6 hours to transcribe. 4 The analysis itself is also time-consuming, requiring transcriptions to be pored over word-for-word and line-by-line. Interviews also present the problem of bias the researcher needs to take care to avoid leading questions or providing non-verbal signals that might influence the responses of participants.
Focus groups
The focus group is a method of data collection in which a moderator/facilitator (usually a coresearcher) speaks with a group of 6–12 participants about issues related to the research question. As an approach, the focus group offers qualitative researchers an efficient method of gathering the views of many participants at one time. Also, the fact that many people are discussing the same issue together can result in an enhanced level of debate, with the moderator often able to step back and let the focus group enter into a free-flowing discussion. 5 This provides an opportunity to gather rich data from a specific population about a particular area of interest, such as barriers perceived by student nurses when trying to communicate with patients with cancer. 6
From a participant perspective, the focus group may provide a more relaxing environment than a one-to-one interview; they will not need to be involved with every part of the discussion and may feel more comfortable expressing views when they are shared by others in the group. Focus groups also allow participants to ‘bounce’ ideas off each other which sometimes results in different perspectives emerging from the discussion. However, focus groups are not without their difficulties. As with interviews, focus groups provide a vast amount of data to be transcribed and analysed, with discussions often lasting 1–2 hours. Moderators also need to be highly skilled to ensure that the discussion can flow while remaining focused and that all participants are encouraged to speak, while ensuring that no individuals dominate the discussion. 7
Observation
Participant and non-participant observation are powerful tools for collecting qualitative data, as they give nurse researchers an opportunity to capture a wide array of information—such as verbal and non-verbal communication, actions (eg, techniques of providing care) and environmental factors—within a care setting. Another advantage of observation is that the researcher gains a first-hand picture of what actually happens in clinical practice. 8 If the researcher is adopting a qualitative approach to observation they will normally record field notes . Field notes can take many forms, such as a chronological log of what is happening in the setting, a description of what has been observed, a record of conversations with participants or an expanded account of impressions from the fieldwork. 9 10
As with other qualitative data collection techniques, observation provides an enormous amount of data to be captured and analysed—one approach to helping with collection and analysis is to digitally record observations to allow for repeated viewing. 11 Observation also provides the researcher with some unique methodological and ethical challenges. Methodologically, the act of being observed may change the behaviour of the participant (often referred to as the ‘Hawthorne effect’), impacting on the value of findings. However, most researchers report a process of habitation taking place where, after a relatively short period of time, those being observed revert to their normal behaviour. Ethically, the researcher will need to consider when and how they should intervene if they view poor practice that could put patients at risk.
The three core approaches to data collection in qualitative research—interviews, focus groups and observation—provide researchers with rich and deep insights. All methods require skill on the part of the researcher, and all produce a large amount of raw data. However, with careful and systematic analysis 12 the data yielded with these methods will allow researchers to develop a detailed understanding of patient experiences and the work of nurses.
- Twycross AM ,
- Williams AM ,
- Huang MC , et al
- Onwuegbuzie AJ ,
- Dickinson WB ,
- Leech NL , et al
- Twycross A ,
- Emerson RM ,
- Meriläinen M ,
- Ala-Kokko T
Competing interests None declared.
Patient consent Not required.
Provenance and peer review Commissioned; internally peer reviewed.
Read the full text or download the PDF:
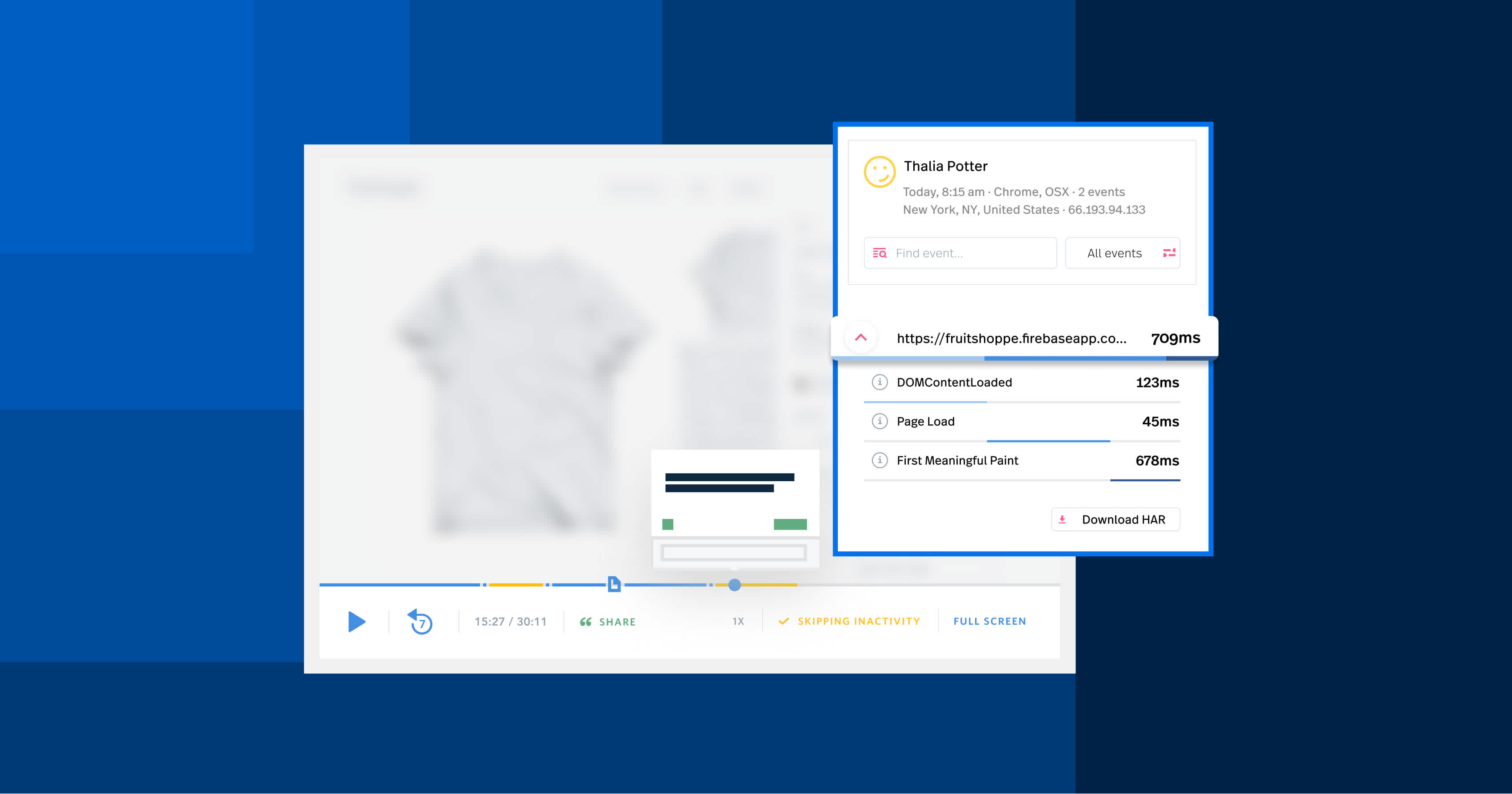
What is qualitative data? How to understand, collect, and analyze it
A comprehensive guide to qualitative data, how it differs from quantitative data, and why it's a valuable tool for solving problems.
What is qualitative research?
Importance of qualitative data.
- Differences between qualitative and quantitative data
Characteristics of qualitative data
Types of qualitative data.
- Pros and cons
- Collection methods
- Return to top
Everything that’s done digitally—from surfing the web to conducting a transaction—creates a data trail. And data analysts are constantly exploring and examining that trail, trying to find out ways to use data to make better decisions.
Different types of data define more and more of our interactions online—one of the most common and well-known being qualitative data or data that can be expressed in descriptions and feelings.
This guide takes a deep look at what qualitative data is, what it can be used for, how it’s collected, and how it’s important to you.
Key takeaways:
Qualitative data gives insights into people's thoughts and feelings through detailed descriptions from interviews, observations, and visual materials.
The three main types of qualitative data are binary, nominal, and ordinal.
There are many different types of qualitative data, like data in research, work, and statistics.
Both qualitative and quantitative research are conducted through surveys and interviews, among other methods.
What is qualitative data?
Qualitative data is descriptive information that captures observable qualities and characteristics not quantifiable by numbers. It is collected from interviews, focus groups, observations, and documents offering insights into experiences, perceptions, and behaviors.
Qualitative data analysis cannot be counted or measured because it describes the data. It refers to the words or labels used to describe certain characteristics or traits.
This type of data answers the "why" or "how" behind the analysis . It’s often used to conduct open-ended studies, allowing those partaking to show their true feelings and actions without direction.
Think of qualitative data as the type of data you’d get if you were to ask someone why they did something—what was their reasoning?
Qualitative research not only helps to collect data, it also gives the researcher a chance to understand the trends and meanings of natural actions.
This type of data research focuses on the qualities of users—the actions behind the numbers. Qualitative research is the descriptive and subjective research that helps bring context to quantitative data.
It’s flexible and iterative. For example:
The music had a light tone that filled the kitchen.
Every blue button had white lettering, while the red buttons had yellow.
The little girl had red hair with a white hat.
Qualitative data is important in determining the frequency of traits or characteristics.
Understanding your data can help you understand your customers, users, or visitors better. And, when you understand your audience better, you can make them happier. First-party data , which is collected directly from your own audience, is especially valuable as it provides the most accurate and relevant insights for your specific needs.
Qualitative data helps the market researcher answer questions like what issues or problems they are facing, what motivates them, and what improvements can be made.
Examples of qualitative data
You’ve most likely used qualitative data today. This type of data is found in your everyday work and in statistics all over the web. Here are some examples of qualitative data in descriptions, research, work, and statistics.
Qualitative data in descriptions
Analysis of qualitative data requires descriptive context in order to support its theories and hypothesis. Here are some core examples of descriptive qualitative data:
The extremely short woman has curly hair and brilliant blue eyes.
A bright white light pierced the small dark space.
The plump fish jumped out of crystal-clear waters.
The fluffy brown dog jumped over the tall white fence.
A soft cloud floated by an otherwise bright blue sky.
Qualitative data in research
Qualitative data research methods allow analysts to use contextual information to create theories and models. These open- and closed-ended questions can be helpful to understand the reasoning behind motivations, frustrations, and actions —in any type of case.
Some examples of qualitative data collection in research:
What country do you work in?
What is your most recent job title?
How do you rank in the search engines?
How do you rate your purchase: good, bad, or exceptional?
Qualitative data at work
Professionals in various industries use qualitative observations in their work and research. Examples of this type of data in the workforce include:
A manager gives an employee constructive criticism on their skills. "Your efforts are solid and you understand the product knowledge well, just have patience."
A judge shares the verdict with the courtroom. "The man was found not guilty and is free to go."
A sales associate collects feedback from customers. "The customer said the check-out button did not work.”
A teacher gives feedback to their student. "I gave you an A on this project because of your dedication and commitment to the cause."
A digital marketer watches a session replay to get an understand of how users use their platform.
Qualitative data in statistics
Qualitative data can provide important statistics about any industry, any group of users, and any products. Here are some examples of qualitative data set collections in statistics:
The age, weight, and height of a group of body types to determine clothing size charts.
The origin, gender, and location for a census reading.
The name, title, and profession of people attending a conference to aid in follow-up emails.
Difference between qualitative and quantitative data
Qualitative and quantitative data are much different, but bring equal value to any data analysis. When it comes to understanding data research, there are different analysis methods, collection types and uses.
Here are the differences between qualitative and quantitative data :
Qualitative data is individualized, descriptive, and relating to emotions.
Quantitative data is countable, measurable and relating to numbers.
Qualitative data helps us understand why, or how something occurred behind certain behaviors .
Quantitative data helps us understand how many, how much, or how often something occurred.
Qualitative data is subjective and personalized.
Quantitative data is fixed and ubiquitous.
Qualitative research methods are conducted through observations or in-depth interviews.
Quantitative research methods are conducted through surveys and factual measuring.
Qualitative data is analyzed by grouping the data into classifications and topics.
Quantitative data is analyzed using statistical analysis.
Both provide a ton of value for any data collection and are key to truly understanding trending use cases and patterns in behavior . Dig deeper into quantitative data examples .
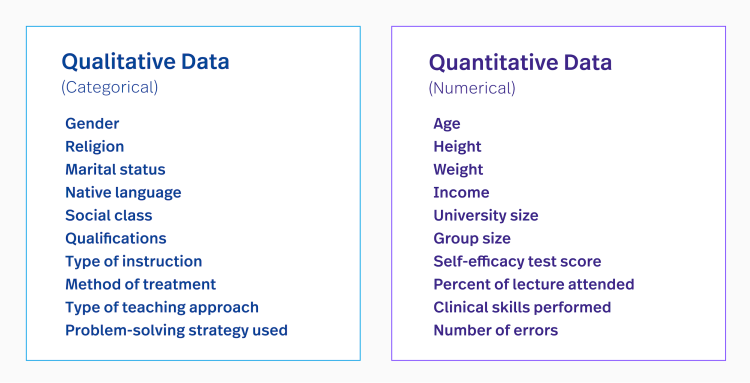
The characteristics of qualitative data are vast. There are a few traits that stand out amongst other data that should be understood for successful data analysis.
Descriptive : describing or classifying in an objective and nonjudgmental way.
Detailed : to give an account in words with full particulars.
Open-ended : having no determined limit or boundary.
Non-numerical : not containing numbers.
Subjective : based on or influenced by personal feelings, tastes, or opinions.
With qualitative data samples, these traits can help you understand the meaning behind the equation—or for lack of a better term, what’s behind the results.
As we narrow down the importance of qualitative data, you should understand that there are different data types. Data analysts often categorize qualitative data into three types:
1. Binary data
Binary data is numerically represented by a combination of zeros and ones. Binary data is the only category of data that can be directly understood and executed by a computer.
Data analysts use binary data to create statistical models that predict how often the study subject is likely to be positive or negative, up or down, right or wrong—based on a zero scale.
2. Nominal data
Nominal data , also referred to as “named, labeled data” or “nominal scaled data,” is any type of data used to label something without giving it a numerical value.
Data analysts use nominal data to determine statistically significant differences between sets of qualitative data.
For example, a multiple-choice test to profile participants’ skills in a study.
3. Ordinal data
Ordinal data is qualitative data categorized in a particular order or on a ranging scale. When researchers use ordinal data, the order of the qualitative information matters more than the difference between each category. Data analysts might use ordinal data when creating charts, while researchers might use it to classify groups, such as age, gender, or class.
For example, a Net Promoter Score ( NPS ) survey has results that are on a 0-10 satisfaction scale.
When should you use qualitative research?
One of the important things to learn about qualitative data is when to use it.
Qualitative data is used when you need to determine the particular trends of traits or characteristics or to form parameters for larger data sets to be observed. Qualitative data provides the means by which analysts can quantify the world around them.
You would use qualitative data to help answer questions like who your customers are, what issues or problems they’re facing, and where they need to focus their attention, so you can better solve those issues.
Qualitative data is widely used to understand language consumers speak—so apply it where necessary.
Pros and cons of qualitative data
Qualitative data is a detailed, deep understanding of a topic through observing and interviewing a sample of people. There are both benefits and drawbacks to this type of data.
Pros of qualitative data
Qualitative research is affordable and requires a small sample size.
Qualitative data provides a predictive element and provides specific insight into development.
Qualitative research focuses on the details of personal choice and uses these individual choices as workable data.
Qualitative research works to remove bias from its collected data by using an open-ended response process.
Qualitative data research provides useful content in any thematic analysis.

Cons of qualitative data
Qualitative data can be time-consuming to collect and can be difficult to scale out to a larger population.
Qualitative research creates subjective information points.
Qualitative research can involve significant levels of repetition and is often difficult to replicate.
Qualitative research relies on the knowledge of the researchers.
Qualitative research does not offer statistical analysis, for that, you have to turn to quantitative data.
Qualitative data collection methods
Here are the main approaches and collection methods of qualitative studies and data:
1. Interviews
Personal interviews are one of the most commonly used deductive data collection methods for qualitative research, because of its personal approach.
The interview may be informal and unstructured and is often conversational in nature. The interviewer or the researcher collects data directly from the interviewee one-to-one. Mostly the open-ended questions are asked spontaneously, with the interviewer allowing the flow of the interview to dictate the questions and answers.
The point of the interview is to obtain how the interviewee feels about the subject.
2. Focus groups
Focus groups are held in a discussion-style setting with 6 to 10 people. The moderator is assigned to monitor and dictate the discussion based on focus questions.
Depending on the qualitative data that is needed, the members of the group may have something in common. For example, a researcher conducting a study on dog sled runners understands dogs, sleds, and snow and would have sufficient knowledge of the subject matter.
3. Data records
Data doesn’t start with your collection, it has most likely been obtained in the past.
Using already existing reliable data and similar sources of information as the data source is a surefire way to obtain qualitative research. Much like going to a library, you can review books and other reference material to collect relevant data that can be used in the research.
For example, if you were to study the trends of dictionaries, you would want to know the past history of every dictionary made, starting with the very first one.
4. Observation
Observation is a longstanding qualitative data collection method, where the researcher simply observes behaviors in a participant's natural setting. They keep a keen eye on the participants and take down transcript notes to find out innate responses and reactions without prompting.
Typically observation is an inductive approach, which is used when a researcher has very little or no idea of the research phenomenon.
Other documentation methods, such as video recordings, audio recordings, and photo imagery, may be used to obtain qualitative data.
Further reading: Site observations through heatmaps
5. Case studies
Case studies are an intensive analysis of an individual person or community with a stress on developmental factors in relation to the environment.
In this method, data is gathered by an in-depth analysis and is used to understand both simple and complex subjects. The goal of a case study is to see how using a product or service has positively impacted the subject, showcasing a solution to a problem or the like.
6. Longitudinal studies
A longitudinal study is where people who share a single characteristic are studied over a period of time.
This data collection method is performed on the same subject repeatedly over an extended period. It is an observational research method that goes on for a few years and, in some cases, decades. The goal is to find correlations of subjects with common traits.
For example, medical researchers conduct longitudinal studies to ascertain the effects of a drug or the symptoms related.
Qualitative data analysis tools
And, as with anything—you aren’t able to be successful without the right tools. Here are a few qualitative data analysis tools to have in your toolbox:
MAXQDA —A qualitative and mixed-method data analysis software
Fullstory —A behavioral data and analysis platform
ATLAS.ti —A powerful qualitative data tool that offers AI-based functions
Quirkos —Qualitative data analysis software for the simple learner
Dedoose —A project management and analysis tool for collaboration and teamwork
Taguette —A free, open-source, data analysis and organization platform
MonkeyLearn —AI-powered, qualitative text analysis, and visualization tool
Qualtrics —Experience management software
Frequently asked questions about qualitative data
Is qualitative data subjective.
Yes, categorical data or qualitative data is information that cannot generally be proven. For instance, the statement “the chair is too small” depends on what it is used for and by whom it is being used.
Who uses qualitative data?
If you’re interested in the following, you should use qualitative data:
Understand emotional connections to your brand
Identify obstacles in any funnel, for example with session replay
Uncover confusion about your messaging
Locate product feature gaps
Improve usability of your website, app, or experience
Observe how people talk, think, and feel about your brand
Learn how an organization selects vendors and partners
What are the steps for qualitative data?
1. Transcribe your data : Once you’ve collected all the data, you need to transcribe it. The first step in analyzing your data is arranging it systematically. Arranging data means converting all the data into a text format.
2. Organize your data : Go back to your research objectives and organize the data based on the questions asked. Arrange your research objective in a table, so it appears visually clear. Avoid working with unorganized data, there will be no conclusive results obtained.
3. Categorize and assign the data : The coding process of qualitative data means categorizing and assigning variables, properties, and patterns. Coding is an important step in qualitative data analysis, as you can derive theories from relevant research findings. You can then begin to gain in-depth insight into the data that help make informed decisions.
4. Validate your data : Data validation is a recurring step that should be followed throughout the research process. There are two sides to validating data: the accuracy and reliability of your research methods, which is the extent to which the methods produce accurate data consistently.
5. Conclude the data analysis : Present your data in a report that shares the method used to conduct the research studies, the outcomes, and the projected hypothesis of your findings in any related areas.
Is qualitative data better than quantitative data?
One is not better than the other, rather they work cohesively to create a better overall data analysis experience. Understanding the importance of both qualitative and quantitative data is going to produce the best possible data content analysis outcome for any study.
Further reading : Qualitative vs. quantitative data — what's the difference?
Related posts
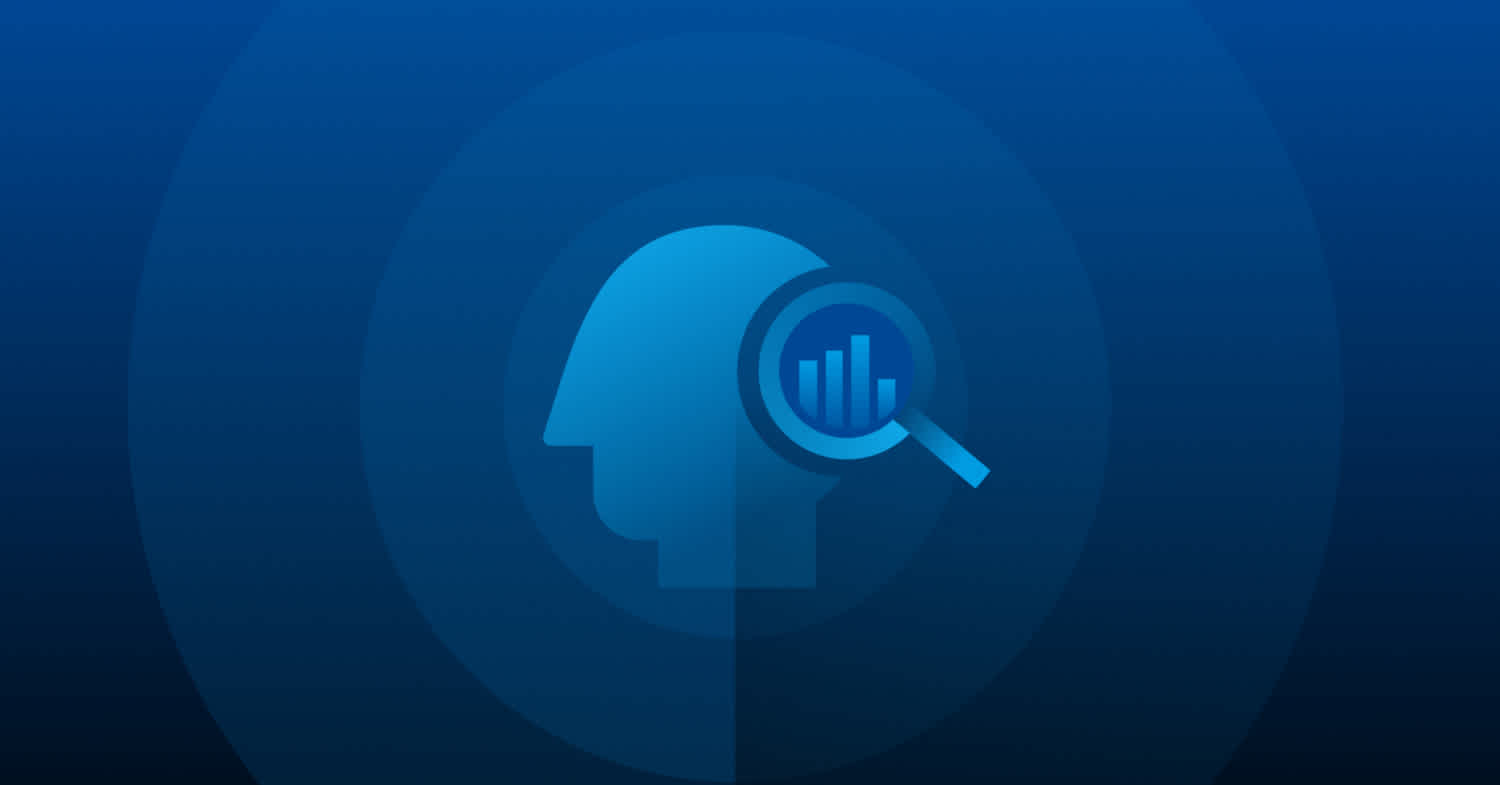
Learn how to analyze qualitative data. We show examples of how to collect, organize, and analyze qualitative data to gain insights.
Here's how you can quantitatively analyze your qualitative digital experience data to unlock an entirely new workflow.
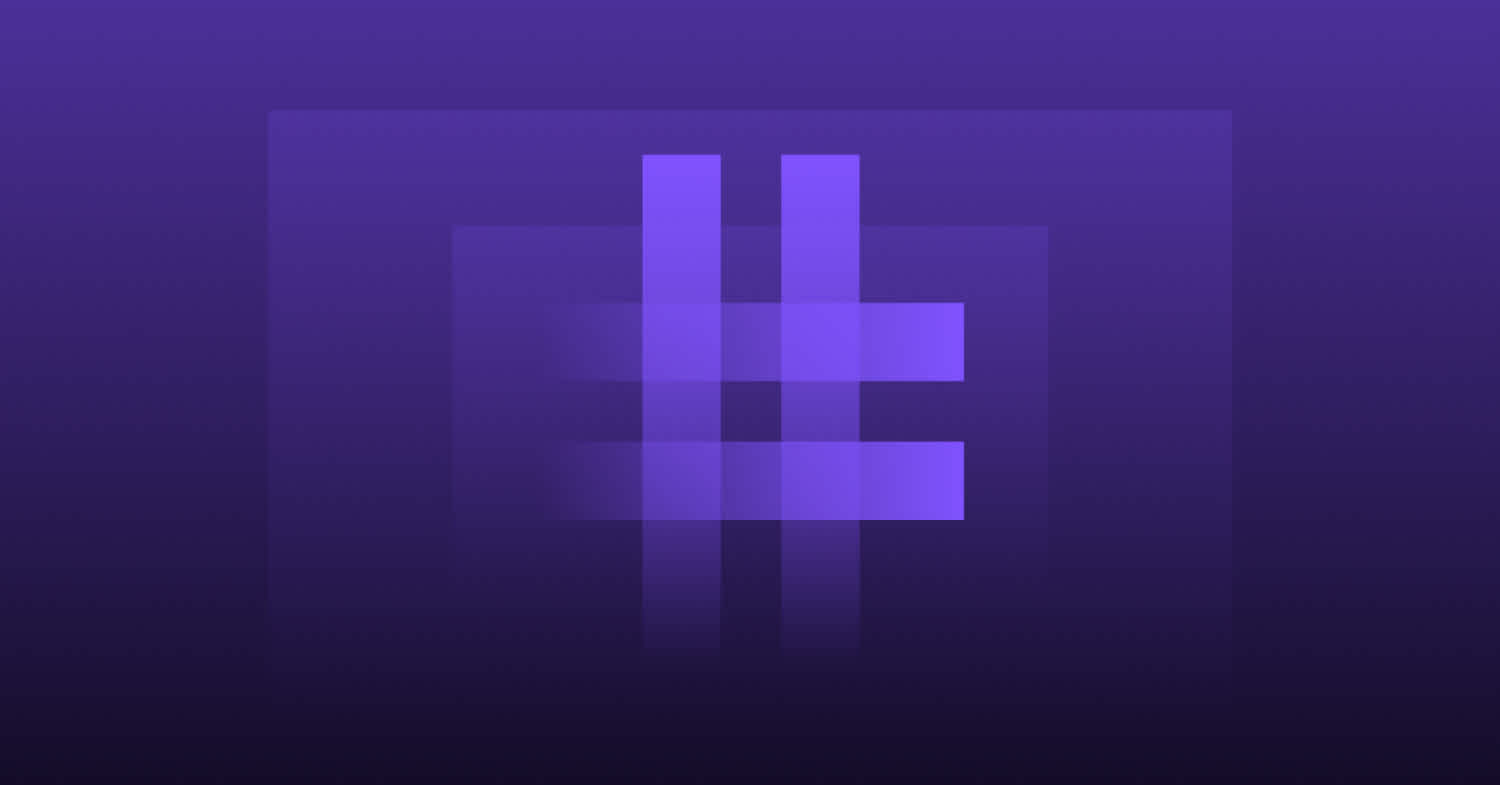
Quantitative data is used for calculations or obtaining numerical results. Learn about the different types of quantitative data uses cases and more.
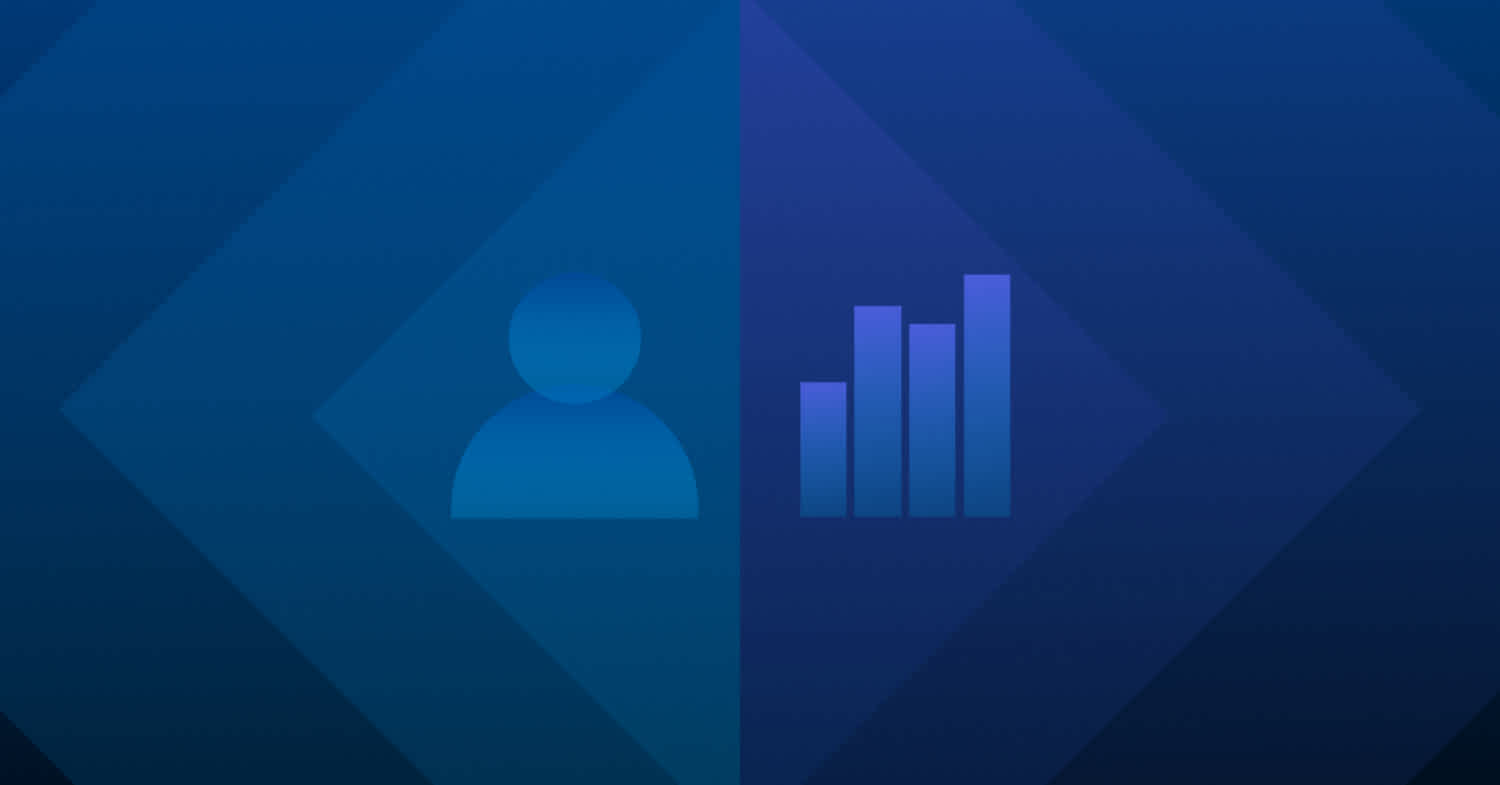
Qualitative and quantitative data differ on what they emphasize—qualitative focuses on meaning, and quantitative emphasizes statistical analysis.
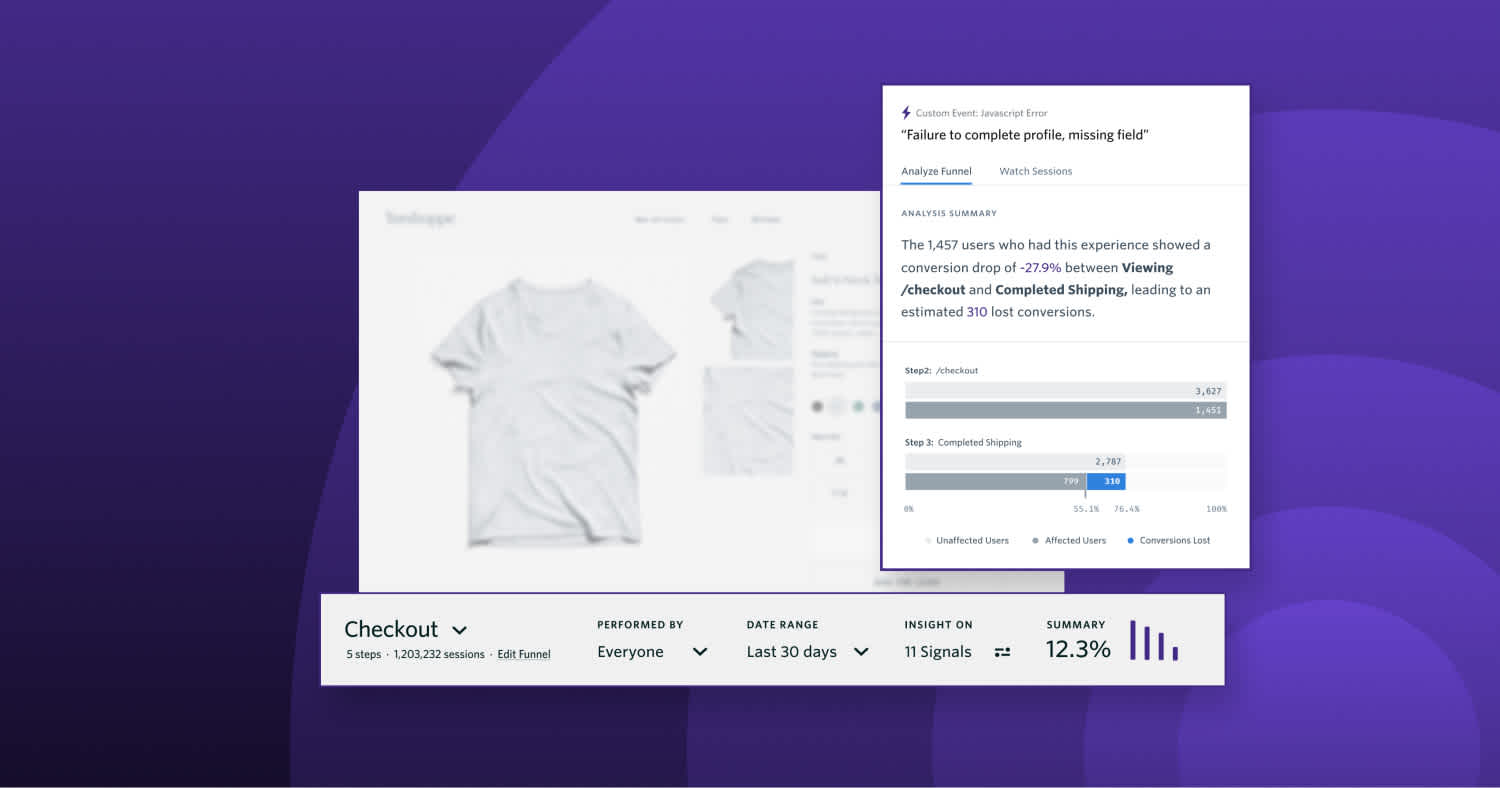
A comprehensive guide to product analysis and analytics platforms, how important they are, and why they’re a valuable asset for your bottom line.
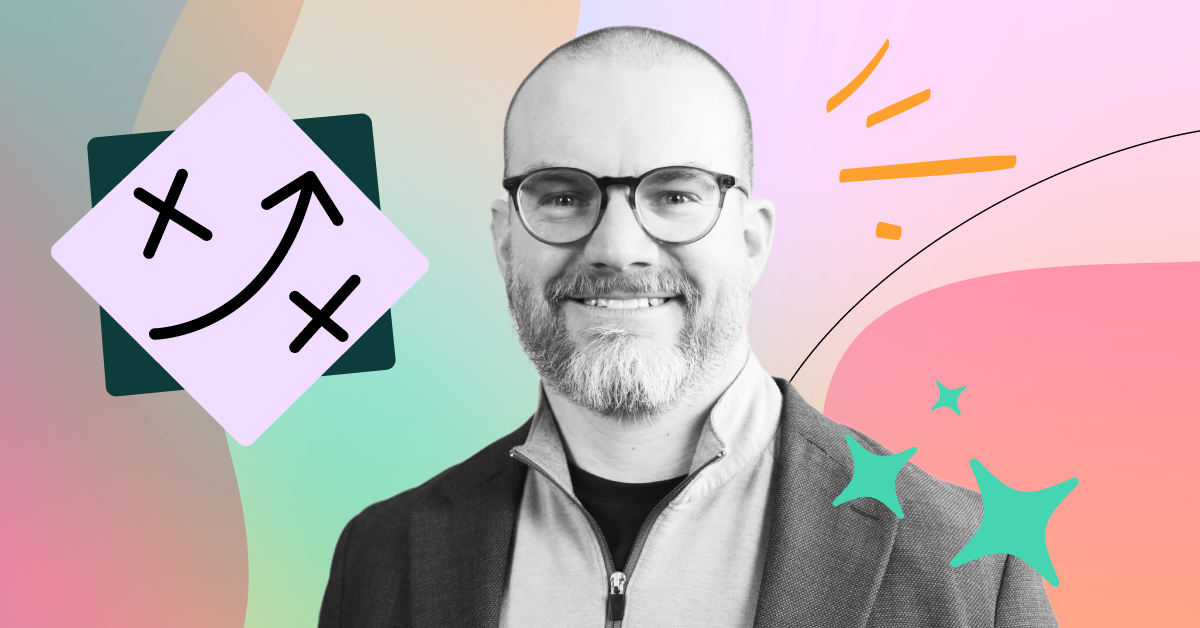
Jordan Morrow shares how AI-driven decision-making can revolutionize your business by harnessing data and enhancing your decision-making processes.
- Search this journal
- Search all journals
- View access options
- View profile
- Create profile
Add email alerts
You are adding the following journal to your email alerts
New content | |
---|---|
Human Resource Development Review |
Learning to Do Qualitative Data Analysis: A Starting Point
Biographies, cite article, share options, information, rights and permissions, metrics and citations, get full access to this article.
View all access and purchase options for this article.
Download to reference manager
If you have citation software installed, you can download article citation data to the citation manager of your choice
Share this article
Share with email, share on social media, share access to this article.
Sharing links are not relevant where the article is open access and not available if you do not have a subscription.
For more information view the Sage Journals article sharing page.
Information
Published in.

- qualitative research
- qualitative data analysis
Rights and permissions
Affiliations, journals metrics.
This article was published in Human Resource Development Review .
Article usage *
Total views and downloads: 168515
* Article usage tracking started in December 2016
Articles citing this one
Receive email alerts when this article is cited
Web of Science: 144 view articles Opens in new tab
Crossref: 80
- The Landscape of Research Method Rigor in the Field of Human Resource ... Go to citation Crossref Google Scholar
- What is Known About Development-Oriented Performance Management Practi... Go to citation Crossref Google Scholar
- “The gay clubs are it”: An analysis of straight women’s motivations fo... Go to citation Crossref Google Scholar
- Understanding the Epistemic Diversity and Essential Components of the ... Go to citation Crossref Google Scholar
- Enabling Collaborative Research in Project Management by Creating Gioi... Go to citation Crossref Google Scholar
- Office gossip related to gays and lesbians: An ‘otherness’ perspective Go to citation Crossref Google Scholar
- COVID-19 and Collective Transformation: Changes in Online Nursing Facu... Go to citation Crossref Google Scholar
- Engagement, Interaction, and Socialization of Islamic Education Pre-se... Go to citation Crossref Google Scholar
- Premature Closure of Analysis in Qualitative Research: Identifying Fea... Go to citation Crossref Google Scholar
- Barriers and enablers to accessing child health resources and services... Go to citation Crossref Google Scholar
- Navigating learning and teaching in expanding culturally diverse highe... Go to citation Crossref Google Scholar
- Nature-based interventions in social work practice and education: Insi... Go to citation Crossref Google Scholar
- Interim Finance in Creditor-Oriented Bankruptcy Codes: A Study in the ... Go to citation Crossref Google Scholar
- Evaluating the Results of PISA Assessment: Are There Gaps Between the ... Go to citation Crossref Google Scholar
- A Study on Politeness Strategies among Generation X and Y in Islamic E... Go to citation Crossref Google Scholar
- Economic-Related Reasons as a Motive to Avoid Sanction during Pandemic... Go to citation Crossref Google Scholar
- Understanding Computer-Assisted Qualitative Data Analysis Software as ... Go to citation Crossref Google Scholar
- The ‘Rich Pictures’ Method: Its Use and Value, and the Implications fo... Go to citation Crossref Google Scholar
- Racism as a vehicle for the overrepresentation of Black youth in child... Go to citation Crossref Google Scholar
- Deployment of physical access control (PAC) devices in university sett... Go to citation Crossref Google Scholar
- Development and validation of an individual entrepreneurial potential ... Go to citation Crossref Google Scholar
- Views on Augmented Reality, Virtual Reality, and 3D Printing in Modern... Go to citation Crossref Google Scholar
- A text-based approach to developing EFL learners’ intercultural awaren... Go to citation Crossref Google Scholar
- Promoting Policy Perspectives on Self-Directed Learning as a Transform... Go to citation Crossref Google Scholar
- Anaesthesia global health programmes in North America: organisational ... Go to citation Crossref Google Scholar
- A Qualitative Study on Factors Influencing Technology Adoption in the ... Go to citation Crossref Google Scholar
- An evaluation of funding challenges in the Malawian public healthcare ... Go to citation Crossref Google Scholar
- Severed connections and timely reflections: A collaborative autoethnog... Go to citation Crossref Google Scholar
- Gender Issues Behind Bars: Insights for a Sustainable Wellbeing Plan Go to citation Crossref Google Scholar
- Teachers’ perceptions of the impact of teenage pregnancy on learners’ ... Go to citation Crossref Google Scholar
- Thinking Diffractively with Data in Human Resource Development Go to citation Crossref Google Scholar
- Using Empathy Interviews and Qualitative Evidence to Improve Human Res... Go to citation Crossref Google Scholar
- Using Qualitative Data Analysis Software to Support Digital Research W... Go to citation Crossref Google Scholar
- Conducting and Writing a Structured Literature Review in Human Resourc... Go to citation Crossref Google Scholar
- Navigating Multiple Approaches to Qualitative Research in HRD Go to citation Crossref Google Scholar
- Introduction to Special Issue: Qualitative Research Methodologies and ... Go to citation Crossref Google Scholar
- Disputes and Claim Management during the COVID-19 Crisis: The Lessons ... Go to citation Crossref Google Scholar
- Analyzing fast and slow: Combining traditional and rapid qualitative a... Go to citation Crossref Google Scholar
- Developing critical thinking in classrooms: Teacher responses to a Rea... Go to citation Crossref Google Scholar
- How Leaders Regulate Emotions Experienced During Organization Change E... Go to citation Crossref Google Scholar
- Use of Framework Matrix and Thematic Coding Methods in Qualitative Ana... Go to citation Crossref Google Scholar
- “Underprepared” Principals Leading Curriculum Reform in Lesotho Go to citation Crossref Google Scholar
- Experiences of B. Ed Students on the use of E-Learning as a Vehicle to... Go to citation Crossref Google Scholar
- How people who are deaf, Deaf, and hard of hearing use technology in c... Go to citation Crossref Google Scholar
- Maternal Experiences with Discussing Complementary Feeding in Primary ... Go to citation Crossref Google Scholar
- Examining Erasmus student social integration at two Croatian universit... Go to citation Crossref Google Scholar
- The journey of embracing life: Mothers' perspectives of living with th... Go to citation Crossref Google Scholar
- At-distance neurocognitive rehabilitation during COVID-19 pandemic: A ... Go to citation Crossref Google Scholar
- Leadership for reducing medical errors via organizational culture: a l... Go to citation Crossref Google Scholar
- Data-driven quality improvement program to prevent hospitalisation and... Go to citation Crossref Google Scholar
- Roles of general practitioners in shared decision‐making for patients ... Go to citation Crossref Google Scholar
- Illocutionary Acts of the Prime Minister of Singapore in Curbing the T... Go to citation Crossref Google Scholar
- “Connecting With Good People and Good Plants”: Community Gardener Expe... Go to citation Crossref Google Scholar
- Religion and Belonging: Voices of Lahore’s Khawajasiras' Community Go to citation Crossref Google Scholar
- From Crisis to Resilience Go to citation Crossref Google Scholar
- Lecturers’ Experiences of Administering Online Examinations at a South... Go to citation Crossref Google Scholar
- Collaborative Zoom Coding—A Novel Approach to Qualitative Analysis Go to citation Crossref Google Scholar
- Qualitative Analyses of Couples’ Text Messages: Methods Reflection Go to citation Crossref Google Scholar
- Collaborative Coding in Multi-National Teams: Benefits, Challenges and... Go to citation Crossref Google Scholar
- Exploring the performance of shared-value banking at discovery bank: a... Go to citation Crossref Google Scholar
- Self-Regulated Writing of English Learners: Intervention Development Go to citation Crossref Google Scholar
- Considerations for Meeting Students' Mental Health Needs at a U.S. Uni... Go to citation Crossref Google Scholar
- Knowing When and How to Trust Superiors’ Decisions: Toward a Conceptua... Go to citation Crossref Google Scholar
- An Explorative Study into the Importance of Defining and Classifying C... Go to citation Crossref Google Scholar
- Leadership Creativity in Entrepreneuring People With Special Abilities... Go to citation Crossref Google Scholar
- “The Light at the End of the Tunnel”: Experiences of LGBTQ+ Adults in ... Go to citation Crossref Google Scholar
- Needed Methodological Emancipation: Qualitative Coding and the Institu... Go to citation Crossref Google Scholar
- What Is Instructor’s Corner in Human Resource Development Review? Go to citation Crossref Google Scholar
- An updated survey of beliefs and practices related to faking in indivi... Go to citation Crossref Google Scholar
- The Italian School Psychologists’ Role: A Qualitative Study about Prof... Go to citation Crossref Google Scholar
- Enhancing resilience in the Covid-19 crisis: lessons from human resour... Go to citation Crossref Google Scholar
- Use of Indicators in Strategic Environmental Assessments of Urban-Plan... Go to citation Crossref Google Scholar
- Youth Inclusion in Forest Policy Dialogue: Contemplating Human–Forest ... Go to citation Crossref Google Scholar
- Tending the grapevine: a qualitative analysis of responses to a psycho... Go to citation Crossref Google Scholar
- Factors Limiting the Development of the Organic Food Sector—Perspectiv... Go to citation Crossref Google Scholar
- Barriers towards Resilient Performance among Public Critical Infrastru... Go to citation Crossref Google Scholar
- Introduction to Special Issue Quality in Qualitative Approaches: Celeb... Go to citation Crossref Google Scholar
- Sustainable Natural Resources and Environmental Management Systems: Ap... Go to citation Crossref Google Scholar
- Measurement of the MOOC Phenomenon by Pre-Service Teachers: A Descript... Go to citation Crossref Google Scholar
- Rethinking the Impact of HRD: New Year, New Opportunities Go to citation Crossref Google Scholar
Figures and tables
Figures & media, view options, access options.
If you have access to journal content via a personal subscription, university, library, employer or society, select from the options below:
I am signed in as:
I can access personal subscriptions, purchases, paired institutional access and free tools such as favourite journals, email alerts and saved searches.
Login failed. Please check you entered the correct user name and password.
Access personal subscriptions, purchases, paired institutional or society access and free tools such as email alerts and saved searches.
loading institutional access options
Click the button below for the full-text content
AHRD members can access this journal content using society membership credentials.
Alternatively, view purchase options below:
Purchase 24 hour online access to view and download content.
Access journal content via a DeepDyve subscription or find out more about this option.
View options
You currently have no access to this content. Visit the access options page to authenticate.
Also from Sage
- CQ Library Elevating debate opens in new tab
- Sage Data Uncovering insight opens in new tab
- Sage Business Cases Shaping futures opens in new tab
- Sage Campus Unleashing potential opens in new tab
- Sage Knowledge Multimedia learning resources opens in new tab
- Sage Research Methods Supercharging research opens in new tab
- Sage Video Streaming knowledge opens in new tab
- Technology from Sage Library digital services opens in new tab
- Our strategy for 2023-2025
- Diversity, equity and inclusion
- Ethics and safeguarding
- Impact and financial reports
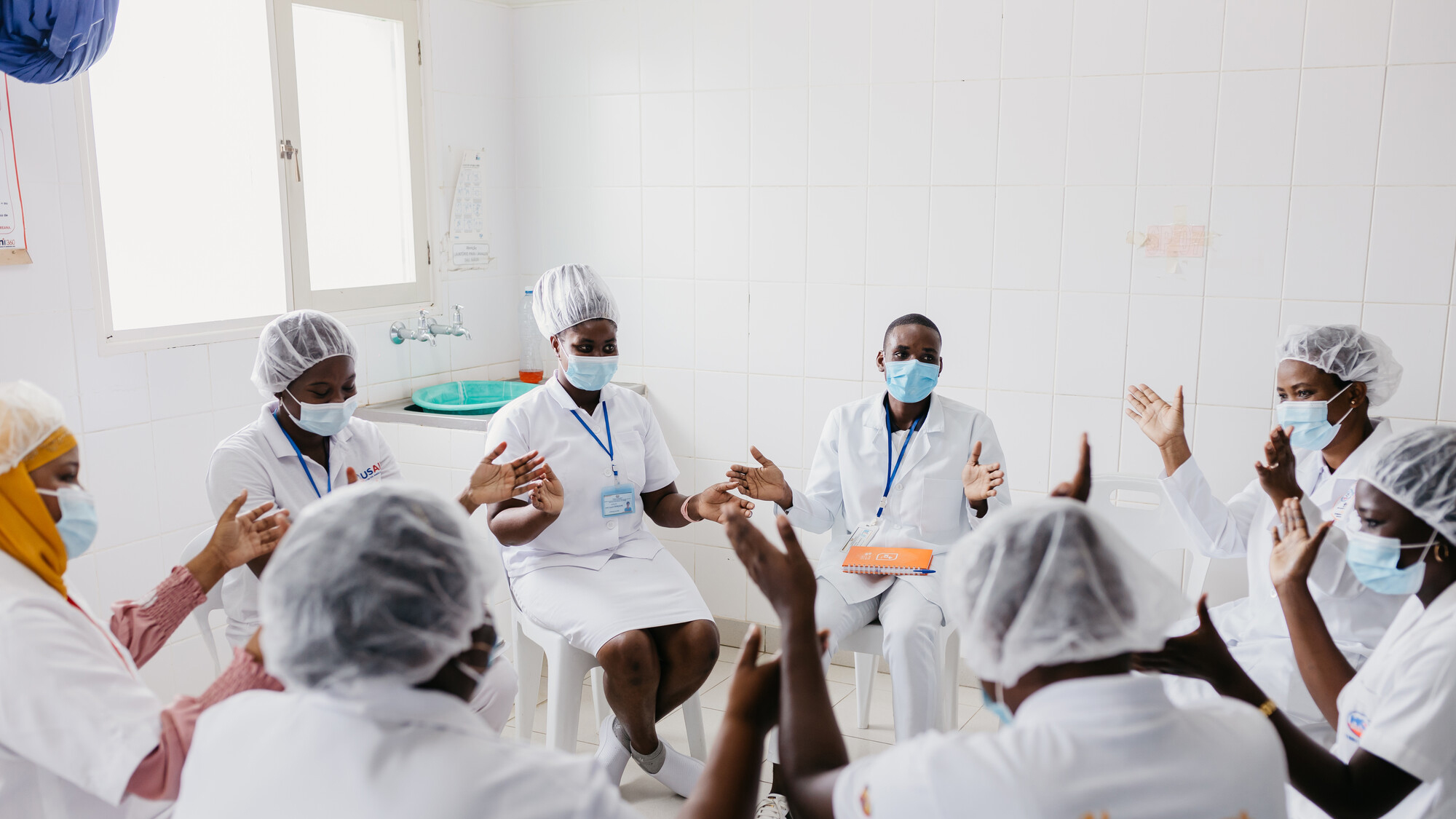
Our strategy
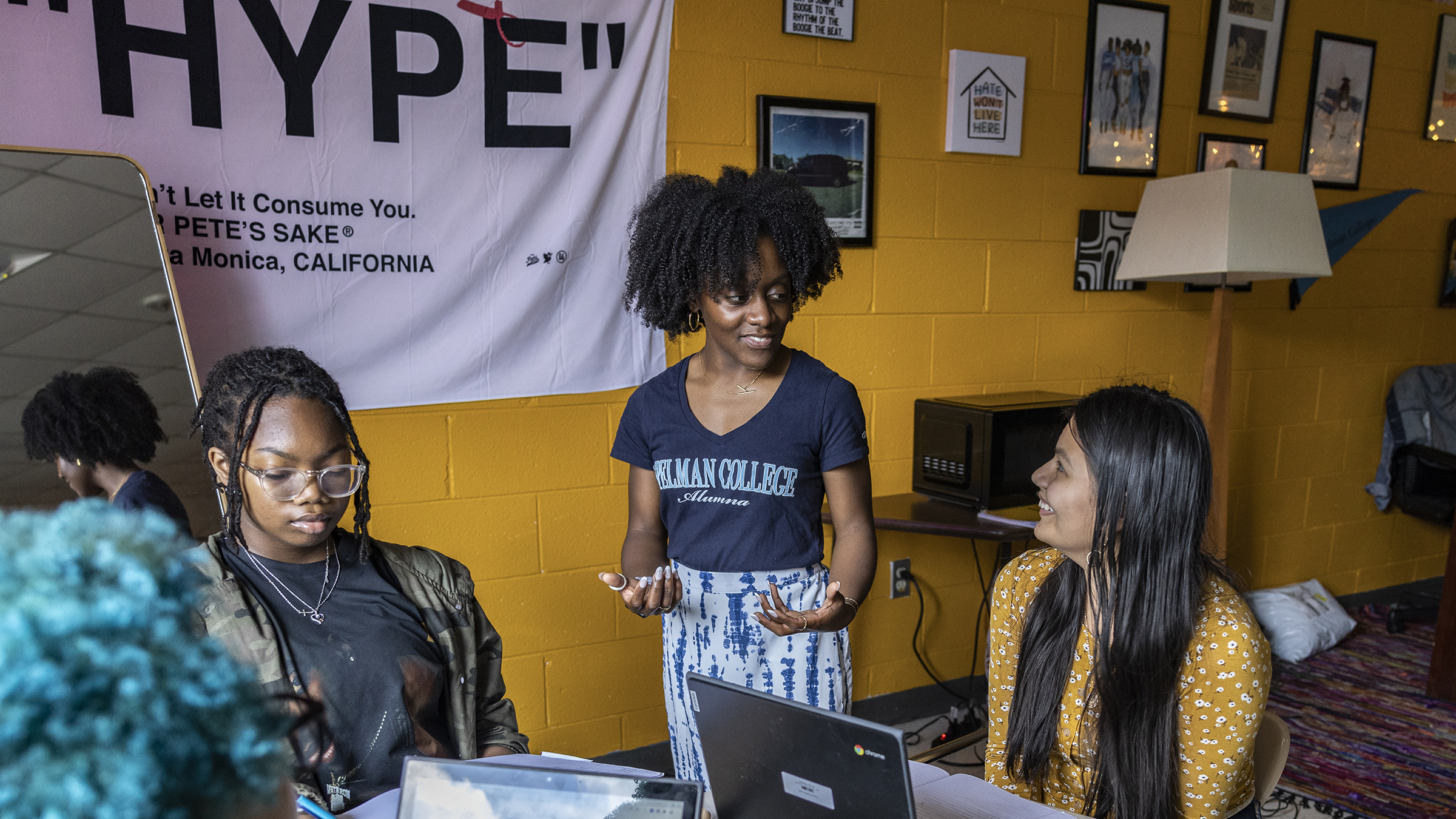
Democracy and governance
Economic opportunity
Environment and climate action
Humanitarian response
- Nutrition and food security
- Explore our expertise
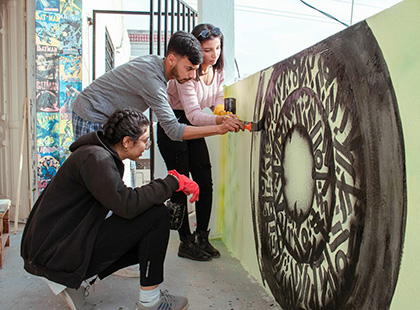
Nutrition and food security
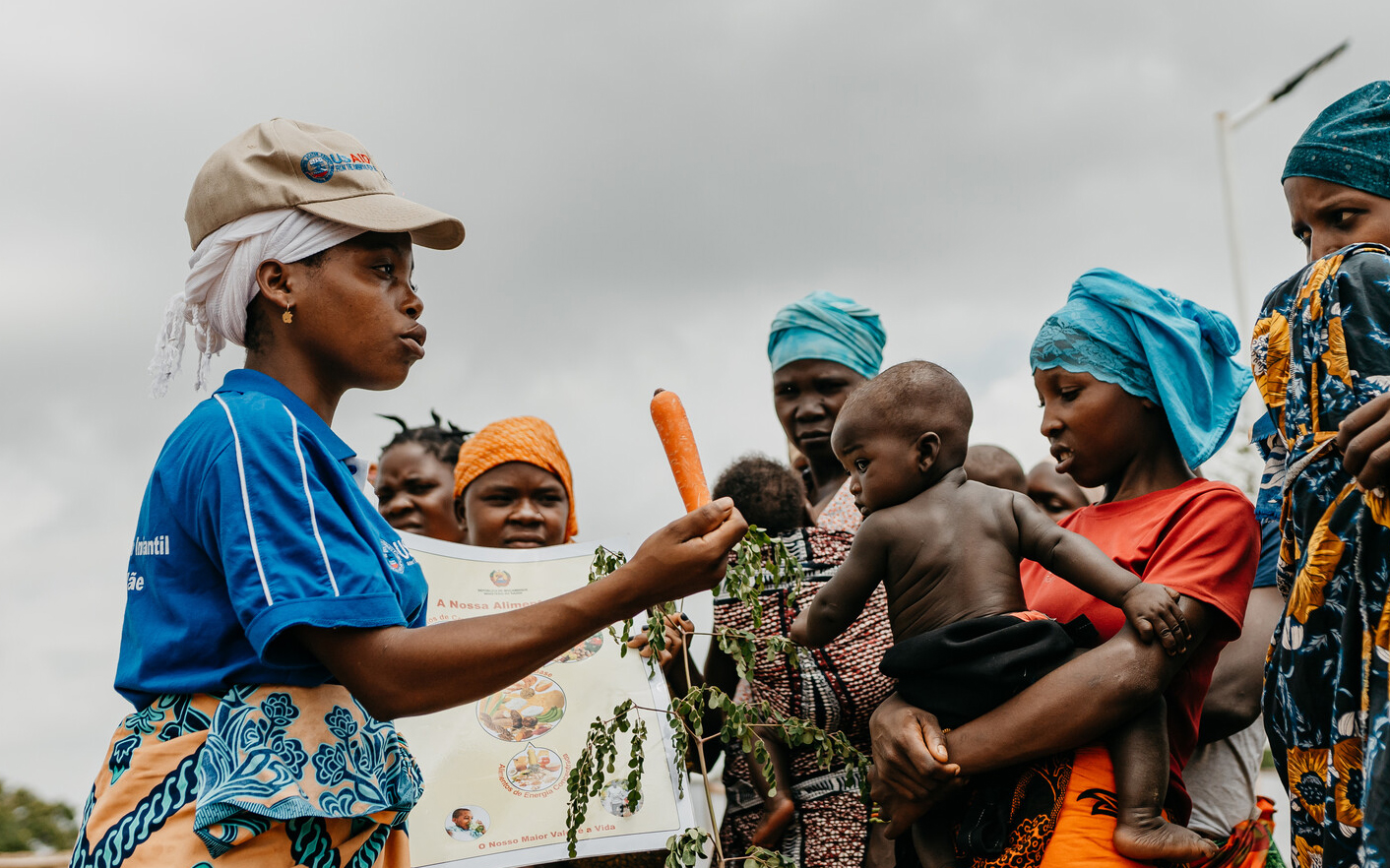
- Asia Pacific
- Central Africa
- East Africa
- West Africa
- Southern Africa
- Middle East and North Africa
- Europe and Central Asia
- Latin America and the Caribbean
- United States
Around the world
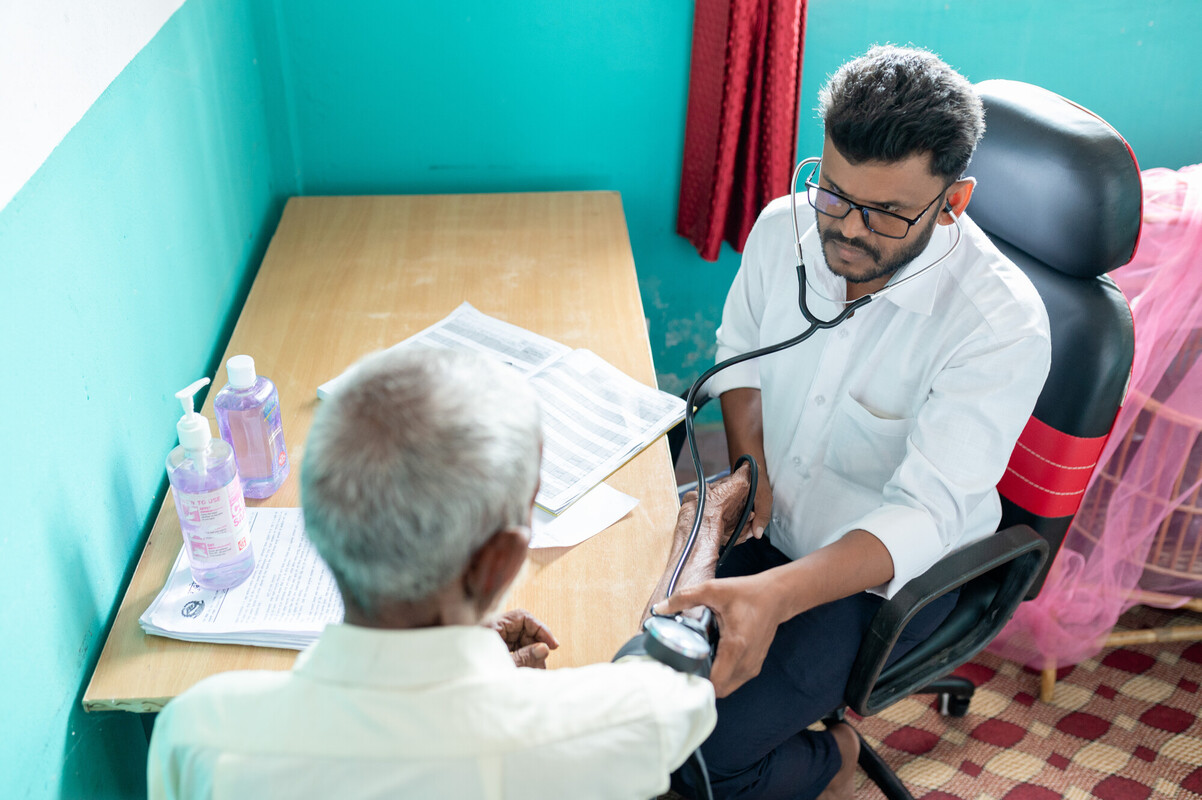
In the United States
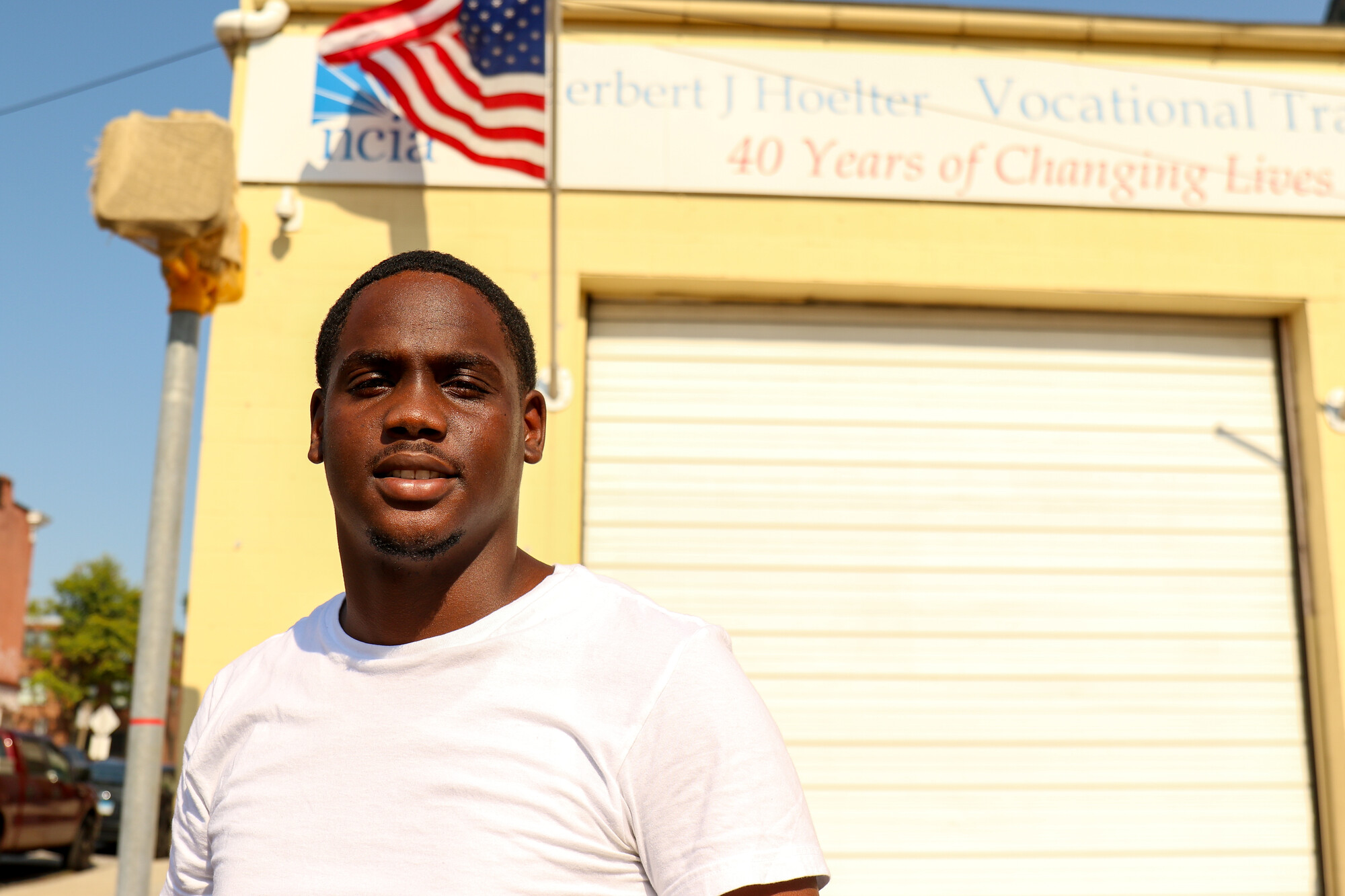
Join our team
Partner with us
- Our FHI 360 network
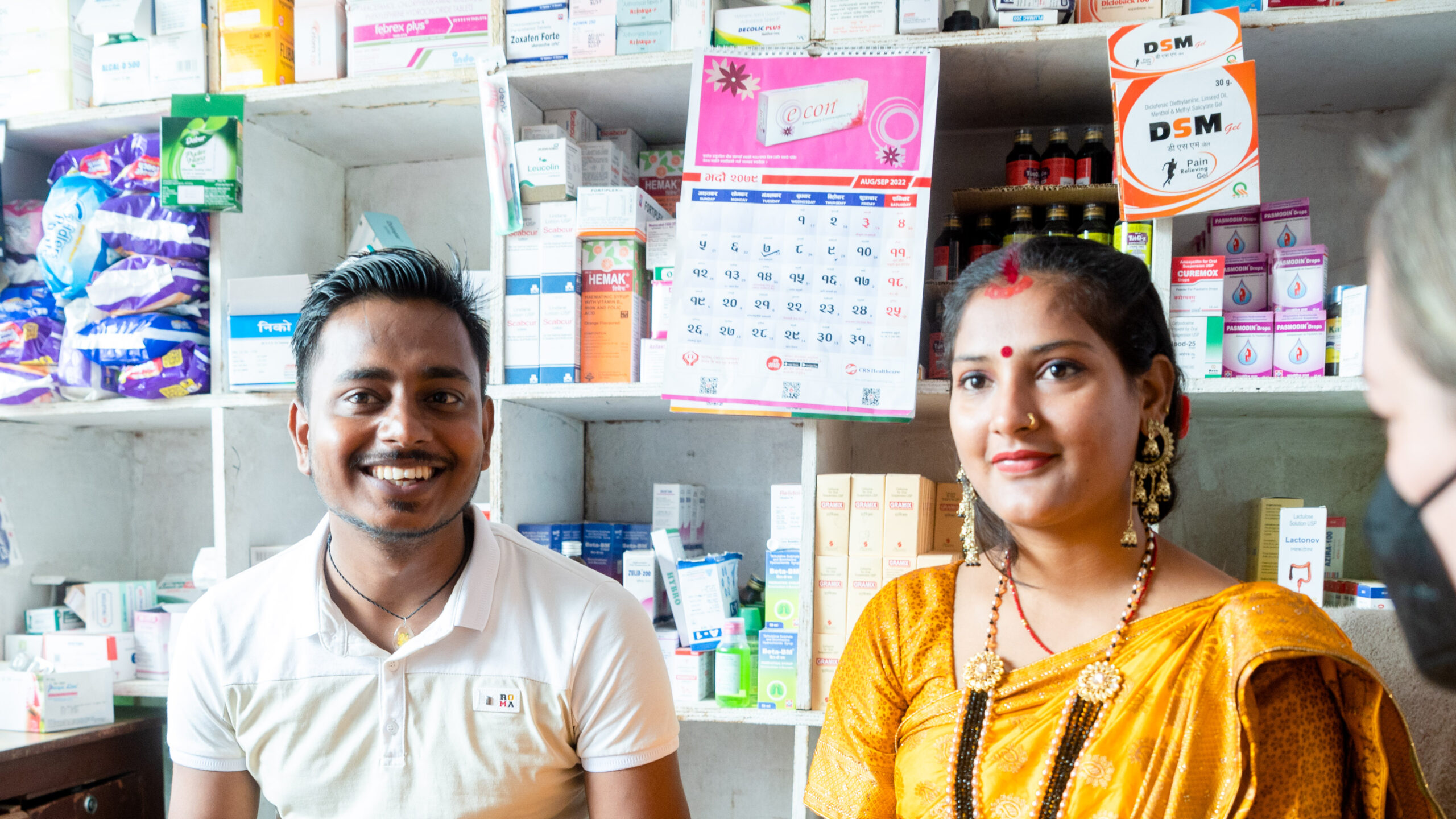
- Videos (YouTube)
- Get the latest news
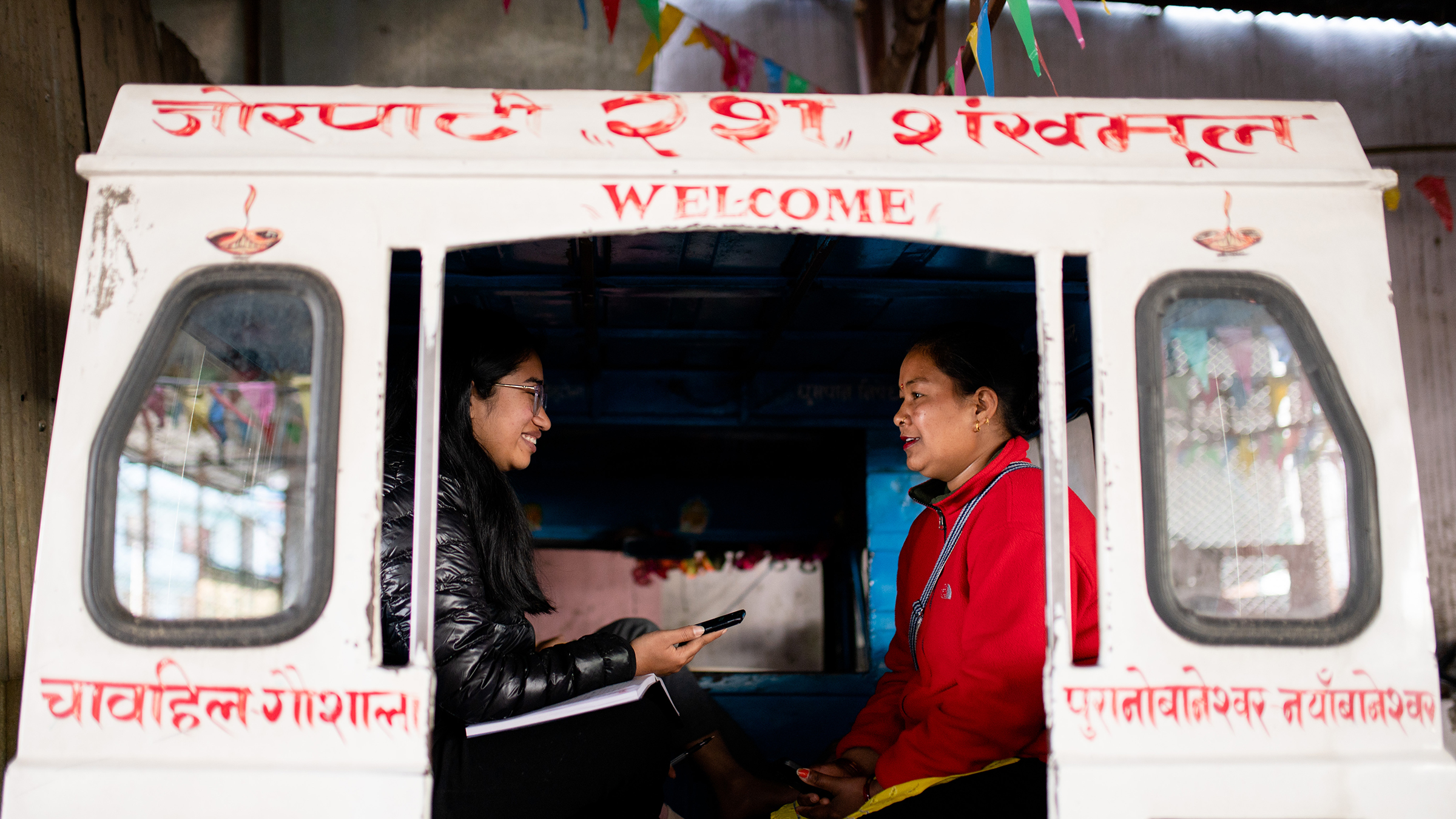
Published research
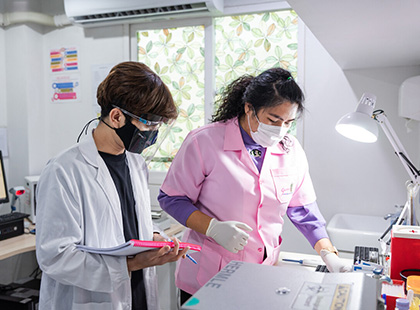
Qualitative Research Methods: A Data Collector’s Field Guide
2005 — This how-to guide covers the mechanics of data collection for applied qualitative research. It is appropriate for novice and experienced researchers alike. It can be used as both a training tool and a daily reference manual for field team members. The question and answer format and modular design make it easy for readers to find information on a particular topic quickly. The Data Collector’s Field Guide includes:
- Self-contained modules for each research method (including participant observation, in-depth interviews and focus group discussions)
- Ethical guidelines for each method
- Case study samples with notes, forms and transcripts
- Tips for taking field notes for specific methods
- Steps and checklists for what to do and bring for each type of data collection
- Exercises for training data collectors in each method
- Tools for data managers
- Suggested readings
Documents / Downloads
- Qualitative Research Methods: A Data Collector's Field Guide
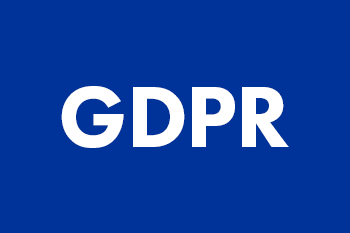
- Privacy overview
- Essential cookies
- Non-essential cookies
This website uses cookies so that we can provide you with the best user experience possible. Cookie information is stored in your browser and performs functions such as recognising you when you return to our website and helping our team to understand which sections of the website you find most interesting and useful.
Essential cookies should be enabled at all times so that we can save your preferences for cookie settings. However, they can be enabled or disabled using the button below.
If you disable all cookies, we will not be able to save your preferences. This means that every time you visit this website you will need to enable or disable essential cookies again.
This website uses Google Analytics to collect anonymous information such as the number of visitors to the site, and the most popular pages.
Keeping this cookie enabled helps us to improve our website.
Please enable essential cookies first so that we can save your preferences!

Official websites use .gov
A .gov website belongs to an official government organization in the United States.
Secure .gov websites use HTTPS
A lock ( ) or https:// means you've safely connected to the .gov website. Share sensitive information only on official, secure websites.

Collecting and Analyzing Qualitative Data
Brent Wolff, Frank Mahoney, Anna Leena Lohiniva, and Melissa Corkum
- Choosing When to Apply Qualitative Methods
- Commonly Used Qualitative Methods in Field Investigations
- Sampling and Recruitment for Qualitative Research
- Managing, Condensing, Displaying, and Interpreting Qualitative Data
- Coding and Analysis Requirements
Qualitative research methods are a key component of field epidemiologic investigations because they can provide insight into the perceptions, values, opinions, and community norms where investigations are being conducted ( 1,2 ). Open-ended inquiry methods, the mainstay of qualitative interview techniques, are essential in formative research for exploring contextual factors and rationales for risk behaviors that do not fit neatly into predefined categories. For example, during the 2014–2015 Ebola virus disease outbreaks in parts of West Africa, understanding the cultural implications of burial practices within different communities was crucial to designing and monitoring interventions for safe burials ( Box 10.1 ). In program evaluations, qualitative methods can assist the investigator in diagnosing what went right or wrong as part of a process evaluation or in troubleshooting why a program might not be working as well as expected. When designing an intervention, qualitative methods can be useful in exploring dimensions of acceptability to increase the chances of intervention acceptance and success. When performed in conjunction with quantitative studies, qualitative methods can help the investigator confirm, challenge, or deepen the validity of conclusions than either component might have yielded alone ( 1,2 ).
Qualitative research was used extensively in response to the Ebola virus disease outbreaks in parts of West Africa to understand burial practices and to design culturally appropriate strategies to ensure safe burials. Qualitative studies were also used to monitor key aspects of the response.
In October 2014, Liberia experienced an abrupt and steady decrease in case counts and deaths in contrast with predicted disease models of an increased case count. At the time, communities were resistant to entering Ebola treatment centers, raising the possibility that patients were not being referred for care and communities might be conducting occult burials.
To assess what was happening at the community level, the Liberian Emergency Operations Center recruited epidemiologists from the US Department of Health and Human Services/Centers for Disease Control and Prevention and the African Union to investigate the problem.
Teams conducted in-depth interviews and focus group discussions with community leaders, local funeral directors, and coffin makers and learned that communities were not conducting occult burials and that the overall number of burials was less than what they had experienced in previous years. Other key findings included the willingness of funeral directors to cooperate with disease response efforts, the need for training of funeral home workers, and considerable community resistance to cremation practices. These findings prompted the Emergency Operations Center to open a burial ground for Ebola decedents, support enhanced testing of burials in the private sector, and train private-sector funeral workers regarding safe burial practices.
Source: Melissa Corkum, personal communication.
Similar to quantitative approaches, qualitative research seeks answers to specific questions by using rigorous approaches to collecting and compiling information and producing findings that can be applicable beyond the study population. The fundamental difference in approaches lies in how they translate real-life complexities of initial observations into units of analysis. Data collected in qualitative studies typically are in the form of text or visual images, which provide rich sources of insight but also tend to be bulky and time-consuming to code and analyze. Practically speaking, qualitative study designs tend to favor small, purposively selected samples ideal for case studies or in-depth analysis ( 1 ). The combination of purposive sampling and open-ended question formats deprive qualitative study designs of the power to quantify and generalize conclusions, one of the key limitations of this approach.
Qualitative scientists might argue, however, that the generalizability and precision possible through probabilistic sampling and categorical outcomes are achieved at the cost of enhanced validity, nuance, and naturalism that less structured approaches offer ( 3 ). Open-ended techniques are particularly useful for understanding subjective meanings and motivations underlying behavior. They enable investigators to be equally adept at exploring factors observed and unobserved, intentions as well as actions, internal meanings as well as external consequences, options considered but not taken, and unmeasurable as well as measurable outcomes. These methods are important when the source of or solution to a public health problem is rooted in local perceptions rather than objectively measurable characteristics selected by outside observers ( 3 ). Ultimately, such approaches have the ability to go beyond quantifying questions of how much or how many to take on questions of how or why from the perspective and in the words of the study subjects themselves ( 1,2 ).
Another key advantage of qualitative methods for field investigations is their flexibility ( 4 ). Qualitative designs not only enable but also encourage flexibility in the content and flow of questions to challenge and probe for deeper meanings or follow new leads if they lead to deeper understanding of an issue (5). It is not uncommon for topic guides to be adjusted in the course of fieldwork to investigate emerging themes relevant to answering the original study question. As discussed herein, qualitative study designs allow flexibility in sample size to accommodate the need for more or fewer interviews among particular groups to determine the root cause of an issue (see the section on Sampling and Recruitment in Qualitative Research). In the context of field investigations, such methods can be extremely useful for investigating complex or fast-moving situations where the dimensions of analysis cannot be fully anticipated.
Ultimately, the decision whether to include qualitative research in a particular field investigation depends mainly on the nature of the research question itself. Certain types of research topics lend themselves more naturally to qualitative rather than other approaches ( Table 10.1 ). These include exploratory investigations when not enough is known about a problem to formulate a hypothesis or develop a fixed set of questions and answer codes. They include research questions where intentions matter as much as actions and “why?” or “why not?” questions matter as much as precise estimation of measured outcomes. Qualitative approaches also work well when contextual influences, subjective meanings, stigma, or strong social desirability biases lower faith in the validity of responses coming from a relatively impersonal survey questionnaire interview.
The availability of personnel with training and experience in qualitative interviewing or observation is critical for obtaining the best quality data but is not absolutely required for rapid assessment in field settings. Qualitative interviewing requires a broader set of skills than survey interviewing. It is not enough to follow a topic guide like a questionnaire, in order, from top to bottom. A qualitative interviewer must exercise judgment to decide when to probe and when to move on, when to encourage, challenge, or follow relevant leads even if they are not written in the topic guide. Ability to engage with informants, connect ideas during the interview, and think on one’s feet are common characteristics of good qualitative interviewers. By far the most important qualification in conducting qualitative fieldwork is a firm grasp of the research objectives; with this qualification, a member of the research team armed with curiosity and a topic guide can learn on the job with successful results.
Exploratory research | The relevant questions or answer options are unknown in advance | In-depth case studies Situation analyses by viewing a problem from multiple perspectives Hypothesis generation |
Understanding the role of context | Risk exposure or care-seeking behavior is embedded in particular social or physical environments | Key barriers or enablers to effective response Competing concerns that might interfere with each other Environmental behavioral interactions |
Understanding the role of perceptions and subjective meaning | Different perception or meaning of the same observable facts influence risk exposure or behavioral response | Why or why not questions Understanding how persons make health decisions Exploring options considered but not taken |
Understanding context and meaning of hidden, sensitive, or illegal behaviors | Legal barriers or social desirability biases prevent candid reporting by using conventional interviewing methods | Risky sexual or drug use behaviors Quality-of-care questions Questions that require a higher degree of trust between respondent and interviewer to obtain valid answers |
Evaluating how interventions work in practice | Evaluating What went right or, more commonly, what went wrong with a public health response Process or outcome evaluations Who benefited in what way from what perceived change in practice | ‘How’ questions Why interventions fail Unintended consequences of programs Patient–provider interactions |
Semi-Structured Interviews
Semi-structured interviews can be conducted with single participants (in-depth or individual key informants) or with groups (focus group discussions [FGDs] or key informant groups). These interviews follow a suggested topic guide rather than a fixed questionnaire format. Topic guides typically consist of a limited number ( 10– 15 ) of broad, open-ended questions followed by bulleted points to facilitate optional probing. The conversational back-and-forth nature of a semi-structured format puts the researcher and researched (the interview participants) on more equal footing than allowed by more structured formats. Respondents, the term used in the case of quantitative questionnaire interviews, become informants in the case of individual semi-structured in-depth interviews (IDIs) or participants in the case of FGDs. Freedom to probe beyond initial responses enables interviewers to actively engage with the interviewee to seek clarity, openness, and depth by challenging informants to reach below layers of self-presentation and social desirability. In this respect, interviewing is sometimes compared with peeling an onion, with the first version of events accessible to the public, including survey interviewers, and deeper inner layers accessible to those who invest the time and effort to build rapport and gain trust. (The theory of the active interview suggests that all interviews involve staged social encounters where the interviewee is constantly assessing interviewer intentions and adjusting his or her responses accordingly [ 1 ]. Consequently good rapport is important for any type of interview. Survey formats give interviewers less freedom to divert from the preset script of questions and formal probes.)
Individual In-Depth Interviews and Key-Informant Interviews
The most common forms of individual semi-structured interviews are IDIs and key informant interviews (KIIs). IDIs are conducted among informants typically selected for first-hand experience (e.g., service users, participants, survivors) relevant to the research topic. These are typically conducted as one-on-one face-to-face interviews (two-on-one if translators are needed) to maximize rapport-building and confidentiality. KIIs are similar to IDIs but focus on individual persons with special knowledge or influence (e.g., community leaders or health authorities) that give them broader perspective or deeper insight into the topic area ( Box 10.2 ). Whereas IDIs tend to focus on personal experiences, context, meaning, and implications for informants, KIIs tend to steer away from personal questions in favor of expert insights or community perspectives. IDIs enable flexible sampling strategies and represent the interviewing reference standard for confidentiality, rapport, richness, and contextual detail. However, IDIs are time-and labor-intensive to collect and analyze. Because confidentiality is not a concern in KIIs, these interviews might be conducted as individual or group interviews, as required for the topic area.
Focus Group Discussions and Group Key Informant Interviews
FGDs are semi-structured group interviews in which six to eight participants, homogeneous with respect to a shared experience, behavior, or demographic characteristic, are guided through a topic guide by a trained moderator ( 6 ). (Advice on ideal group interview size varies. The principle is to convene a group large enough to foster an open, lively discussion of the topic, and small enough to ensure all participants stay fully engaged in the process.) Over the course of discussion, the moderator is expected to pose questions, foster group participation, and probe for clarity and depth. Long a staple of market research, focus groups have become a widely used social science technique with broad applications in public health, and they are especially popular as a rapid method for assessing community norms and shared perceptions.
Focus groups have certain useful advantages during field investigations. They are highly adaptable, inexpensive to arrange and conduct, and often enjoyable for participants. Group dynamics effectively tap into collective knowledge and experience to serve as a proxy informant for the community as a whole. They are also capable of recreating a microcosm of social norms where social, moral, and emotional dimensions of topics are allowed to emerge. Skilled moderators can also exploit the tendency of small groups to seek consensus to bring out disagreements that the participants will work to resolve in a way that can lead to deeper understanding. There are also limitations on focus group methods. Lack of confidentiality during group interviews means they should not be used to explore personal experiences of a sensitive nature on ethical grounds. Participants may take it on themselves to volunteer such information, but moderators are generally encouraged to steer the conversation back to general observations to avoid putting pressure on other participants to disclose in a similar way. Similarly, FGDs are subject by design to strong social desirability biases. Qualitative study designs using focus groups sometimes add individual interviews precisely to enable participants to describe personal experiences or personal views that would be difficult or inappropriate to share in a group setting. Focus groups run the risk of producing broad but shallow analyses of issues if groups reach comfortable but superficial consensus around complex topics. This weakness can be countered by training moderators to probe effectively and challenge any consensus that sounds too simplistic or contradictory with prior knowledge. However, FGDs are surprisingly robust against the influence of strongly opinionated participants, highly adaptable, and well suited to application in study designs where systematic comparisons across different groups are called for.
Like FGDs, group KIIs rely on positive chemistry and the stimulating effects of group discussion but aim to gather expert knowledge or oversight on a particular topic rather than lived experience of embedded social actors. Group KIIs have no minimum size requirements and can involve as few as two or three participants.
Egypt’s National Infection Prevention and Control (IPC) program undertook qualitative research to gain an understanding of the contextual behaviors and motivations of healthcare workers in complying with IPC guidelines. The study was undertaken to guide the development of effective behavior change interventions in healthcare settings to improve IPC compliance.
Key informant interviews and focus group discussions were conducted in two governorates among cleaning staff, nursing staff, and physicians in different types of healthcare facilities. The findings highlighted social and cultural barriers to IPC compliance, enabling the IPC program to design responses. For example,
- Informants expressed difficulty in complying with IPC measures that forced them to act outside their normal roles in an ingrained hospital culture. Response: Role models and champions were introduced to help catalyze change.
- Informants described fatalistic attitudes that undermined energy and interest in modifying behavior. Response: Accordingly, interventions affirming institutional commitment to change while challenging fatalistic assumptions were developed.
- Informants did not perceive IPC as effective. Response: Trainings were amended to include scientific evidence justifying IPC practices.
- Informants perceived hygiene as something they took pride in and were judged on. Response: Public recognition of optimal IPC practice was introduced to tap into positive social desirability and professional pride in maintaining hygiene in the work environment.
Qualitative research identified sources of resistance to quality clinical practice in Egypt’s healthcare settings and culturally appropriate responses to overcome that resistance.
____________________ Source: Anna Leena Lohiniva, personal communication.
Visualization Methods
Visualization methods have been developed as a way to enhance participation and empower interviewees relative to researchers during group data collection ( 7 ). Visualization methods involve asking participants to engage in collective problem- solving of challenges expressed through group production of maps, diagrams, or other images. For example, participants from the community might be asked to sketch a map of their community and to highlight features of relevance to the research topic (e.g., access to health facilities or sites of risk concentrations). Body diagramming is another visualization tool in which community members are asked to depict how and where a health threat affects the human body as a way of understanding folk conceptions of health, disease, treatment, and prevention. Ensuing debate and dialogue regarding construction of images can be recorded and analyzed in conjunction with the visual image itself. Visualization exercises were initially designed to accommodate groups the size of entire communities, but they can work equally well with smaller groups corresponding to the size of FGDs or group KIIs.
Selecting a Sample of Study Participants
Fundamental differences between qualitative and quantitative approaches to research emerge most clearly in the practice of sampling and recruitment of study participants. Qualitative samples are typically small and purposive. In-depth interview informants are usually selected on the basis of unique characteristics or personal experiences that make them exemplary for the study, if not typical in other respects. Key informants are selected for their unique knowledge or influence in the study domain. Focus group mobilization often seeks participants who are typical with respect to others in the community having similar exposure or shared characteristics. Often, however, participants in qualitative studies are selected because they are exceptional rather than simply representative. Their value lies not in their generalizability but in their ability to generate insight into the key questions driving the study.
Determining Sample Size
Sample size determination for qualitative studies also follows a different logic than that used for probability sample surveys. For example, whereas some qualitative methods specify ideal ranges of participants that constitute a valid observation (e.g., focus groups), there are no rules on how many observations it takes to attain valid results. In theory, sample size in qualitative designs should be determined by the saturation principle , where interviews are conducted until additional interviews yield no additional insights into the topic of research ( 8 ). Practically speaking, designing a study with a range in number of interviews is advisable for providing a level of flexibility if additional interviews are needed to reach clear conclusions.
Recruiting Study Participants
Recruitment strategies for qualitative studies typically involve some degree of participant self-selection (e.g., advertising in public spaces for interested participants) and purposive selection (e.g., identification of key informants). Purposive selection in community settings often requires authorization from local authorities and assistance from local mobilizers before the informed consent process can begin. Clearly specifying eligibility criteria is crucial for minimizing the tendency of study mobilizers to apply their own filters regarding who reflects the community in the best light. In addition to formal eligibility criteria, character traits (e.g., articulate and interested in participating) and convenience (e.g., not too far away) are legitimate considerations for whom to include in the sample. Accommodations to personality and convenience help to ensure the small number of interviews in a typical qualitative design yields maximum value for minimum investment. This is one reason why random sampling of qualitative informants is not only unnecessary but also potentially counterproductive.
Analysis of qualitative data can be divided into four stages: data management, data condensation, data display, and drawing and verifying conclusions ( 9 ).
Managing Qualitative Data
From the outset, developing a clear organization system for qualitative data is important. Ideally, naming conventions for original data files and subsequent analysis should be recorded in a data dictionary file that includes dates, locations, defining individual or group characteristics, interviewer characteristics, and other defining features. Digital recordings of interviews or visualization products should be reviewed to ensure fidelity of analyzed data to original observations. If ethics agreements require that no names or identifying characteristics be recorded, all individual names must be removed from final transcriptions before analysis begins. If data are analyzed by using textual data analysis software, maintaining careful version control over the data files is crucial, especially when multiple coders are involved.
Condensing Qualitative Data
Condensing refers to the process of selecting, focusing, simplifying, and abstracting the data available at the time of the original observation, then transforming the condensed data into a data set that can be analyzed. In qualitative research, most of the time investment required to complete a study comes after the fieldwork is complete. A single hour of taped individual interview can take a full day to transcribe and additional time to translate if necessary. Group interviews can take even longer because of the difficulty of transcribing active group input. Each stage of data condensation involves multiple decisions that require clear rules and close supervision. A typical challenge is finding the right balance between fidelity to the rhythm and texture of original language and clarity of the translated version in the language of analysis. For example, discussions among groups with little or no education should not emerge after the transcription (and translation) process sounding like university graduates. Judgment must be exercised about which terms should be translated and which terms should be kept in vernacular because there is no appropriate term in English to capture the richness of its meaning.
Displaying Qualitative Data
After the initial condensation, qualitative analysis depends on how the data are displayed. Decisions regarding how data are summarized and laid out to facilitate comparison influence the depth and detail of the investigation’s conclusions. Displays might range from full verbatim transcripts of interviews to bulleted summaries or distilled summaries of interview notes. In a field setting, a useful and commonly used display format is an overview chart in which key themes or research questions are listed in rows in a word processer table or in a spreadsheet and individual informant or group entry characteristics are listed across columns. Overview charts are useful because they allow easy, systematic comparison of results.
Drawing and Verifying Conclusions
Analyzing qualitative data is an iterative and ideally interactive process that leads to rigorous and systematic interpretation of textual or visual data. At least four common steps are involved:
- Reading and rereading. The core of qualitative analysis is careful, systematic, and repeated reading of text to identify consistent themes and interconnections emerging from the data. The act of repeated reading inevitably yields new themes, connections, and deeper meanings from the first reading. Reading the full text of interviews multiple times before subdividing according to coded themes is key to appreciating the full context and flow of each interview before subdividing and extracting coded sections of text for separate analysis.
- Coding. A common technique in qualitative analysis involves developing codes for labeling sections of text for selective retrieval in later stages of analysis and verification. Different approaches can be used for textual coding. One approach, structural coding , follows the structure of the interview guide. Another approach, thematic coding , labels common themes that appear across interviews, whether by design of the topic guide or emerging themes assigned based on further analysis. To avoid the problem of shift and drift in codes across time or multiple coders, qualitative investigators should develop a standard codebook with written definitions and rules about when codes should start and stop. Coding is also an iterative process in which new codes that emerge from repeated reading are layered on top of existing codes. Development and refinement of the codebook is inseparably part of the analysis.
- Analyzing and writing memos. As codes are being developed and refined, answers to the original research question should begin to emerge. Coding can facilitate that process through selective text retrieval during which similarities within and between coding categories can be extracted and compared systematically. Because no p values can be derived in qualitative analyses to mark the transition from tentative to firm conclusions, standard practice is to write memos to record evolving insights and emerging patterns in the data and how they relate to the original research questions. Writing memos is intended to catalyze further thinking about the data, thus initiating new connections that can lead to further coding and deeper understanding.
- Verifying conclusions. Analysis rigor depends as much on the thoroughness of the cross-examination and attempt to find alternative conclusions as on the quality of original conclusions. Cross-examining conclusions can occur in different ways. One way is encouraging regular interaction between analysts to challenge conclusions and pose alternative explanations for the same data. Another way is quizzing the data (i.e., retrieving coded segments by using Boolean logic to systematically compare code contents where they overlap with other codes or informant characteristics). If alternative explanations for initial conclusions are more difficult to justify, confidence in those conclusions is strengthened.
Above all, qualitative data analysis requires sufficient time and immersion in the data. Computer textual software programs can facilitate selective text retrieval and quizzing the data, but discerning patterns and arriving at conclusions can be done only by the analysts. This requirement involves intensive reading and rereading, developing codebooks and coding, discussing and debating, revising codebooks, and recoding as needed until clear patterns emerge from the data. Although quality and depth of analysis is usually proportional to the time invested, a number of techniques, including some mentioned earlier, can be used to expedite analysis under field conditions.
- Detailed notes instead of full transcriptions. Assigning one or two note-takers to an interview can be considered where the time needed for full transcription and translation is not feasible. Even if plans are in place for full transcriptions after fieldwork, asking note-takers to submit organized summary notes is a useful technique for getting real-time feedback on interview content and making adjustments to topic guides or interviewer training as needed.
- Summary overview charts for thematic coding. (See discussion under “Displaying Data.”) If there is limited time for full transcription and/or systematic coding of text interviews using textual analysis software in the field, an overview chart is a useful technique for rapid manual coding.
- Thematic extract files. This is a slightly expanded version of manual thematic coding that is useful when full transcriptions of interviews are available. With use of a word processing program, files can be sectioned according to themes, or separate files can be created for each theme. Relevant extracts from transcripts or analyst notes can be copied and pasted into files or sections of files corresponding to each theme. This is particularly useful for storing appropriate quotes that can be used to illustrate thematic conclusions in final reports or manuscripts.
- Teamwork. Qualitative analysis can be performed by a single analyst, but it is usually beneficial to involve more than one. Qualitative conclusions involve subjective judgment calls. Having more than one coder or analyst working on a project enables more interactive discussion and debate before reaching consensus on conclusions.
- Systematic coding.
- Selective retrieval of coded segments.
- Verifying conclusions (“quizzing the data”).
- Working on larger data sets with multiple separate files.
- Working in teams with multiple coders to allow intercoder reliability to be measured and monitored.
The most widely used software packages (e.g., NVivo [QSR International Pty. Ltd., Melbourne, VIC, Australia] and ATLAS.ti [Scientific Software Development GmbH, Berlin, Germany]) evolved to include sophisticated analytic features covering a wide array of applications but are relatively expensive in terms of license cost and initial investment in time and training. A promising development is the advent of free or low-cost Web-based services (e.g., Dedoose [Sociocultural Research Consultants LLC, Manhattan Beach, CA]) that have many of the same analytic features on a more affordable subscription basis and that enable local research counterparts to remain engaged through the analysis phase (see Teamwork criteria). The start-up costs of computer-assisted analysis need to be weighed against their analytic benefits, which tend to decline with the volume and complexity of data to be analyzed. For rapid situational analyses or small scale qualitative studies (e.g. fewer than 30 observations as an informal rule of thumb), manual coding and analysis using word processing or spreadsheet programs is faster and sufficient to enable rigorous analysis and verification of conclusions.
Qualitative methods belong to a branch of social science inquiry that emphasizes the importance of context, subjective meanings, and motivations in understanding human behavior patterns. Qualitative approaches definitionally rely on open-ended, semistructured, non-numeric strategies for asking questions and recording responses. Conclusions are drawn from systematic visual or textual analysis involving repeated reading, coding, and organizing information into structured and emerging themes. Because textual analysis is relatively time-and skill-intensive, qualitative samples tend to be small and purposively selected to yield the maximum amount of information from the minimum amount of data collection. Although qualitative approaches cannot provide representative or generalizable findings in a statistical sense, they can offer an unparalleled level of detail, nuance, and naturalistic insight into the chosen subject of study. Qualitative methods enable investigators to “hear the voice” of the researched in a way that questionnaire methods, even with the occasional open-ended response option, cannot.
Whether or when to use qualitative methods in field epidemiology studies ultimately depends on the nature of the public health question to be answered. Qualitative approaches make sense when a study question about behavior patterns or program performance leads with why, why not , or how . Similarly, they are appropriate when the answer to the study question depends on understanding the problem from the perspective of social actors in real-life settings or when the object of study cannot be adequately captured, quantified, or categorized through a battery of closed-ended survey questions (e.g., stigma or the foundation of health beliefs). Another justification for qualitative methods occurs when the topic is especially sensitive or subject to strong social desirability biases that require developing trust with the informant and persistent probing to reach the truth. Finally, qualitative methods make sense when the study question is exploratory in nature, where this approach enables the investigator the freedom and flexibility to adjust topic guides and probe beyond the original topic guides.
Given that the conditions just described probably apply more often than not in everyday field epidemiology, it might be surprising that such approaches are not incorporated more routinely into standard epidemiologic training. Part of the answer might have to do with the subjective element in qualitative sampling and analysis that seems at odds with core scientific values of objectivity. Part of it might have to do with the skill requirements for good qualitative interviewing, which are generally more difficult to find than those required for routine survey interviewing.
For the field epidemiologist unfamiliar with qualitative study design, it is important to emphasize that obtaining important insights from applying basic approaches is possible, even without a seasoned team of qualitative researchers on hand to do the work. The flexibility of qualitative methods also tends to make them forgiving with practice and persistence. Beyond the required study approvals and ethical clearances, the basic essential requirements for collecting qualitative data in field settings start with an interviewer having a strong command of the research question, basic interactive and language skills, and a healthy sense of curiosity, armed with a simple open-ended topic guide and a tape recorder or note-taker to capture the key points of the discussion. Readily available manuals on qualitative study design, methods, and analysis can provide additional guidance to improve the quality of data collection and analysis.
- Patton MQ. Qualitative research and evaluation methods: integrating theory and practice . 4th ed. Thousand Oaks, CA: Sage; 2015.
- Hennink M, Hutter I, Bailey A. Qualitative research methods . Thousand Oaks, CA: Sage; 2010.
- Lincoln YS, Guba EG. The constructivist credo . Walnut Creek, CA: Left Coast Press; 2013.
- Mack N, Woodsong C, MacQueen KM, Guest G, Namey E. Qualitative research methods: a data collectors field guide. https://www.fhi360.org/sites/default/files/media/documents/Qualitative%20Research%20Methods%20-%20A%20Data%20Collector%27s%20Field%20Guide.pdf
- Kvale S, Brinkmann S. Interviews: learning the craft of qualitative research . Thousand Oaks, CA: Sage; 2009:230–43.
- Krueger RA, Casey MA. Focus groups: a practical guide for applied research . Thousand Oaks, CA: Sage; 2014.
- Margolis E, Pauwels L. The Sage handbook of visual research methods . Thousand Oaks, CA: Sage; 2011.
- Mason M. Sample size and saturation in PhD studies using qualitative interviews. Forum : Qualitative Social Research/Sozialforschung. 2010;11(3).
- Miles MB, Huberman AM, Saldana J. Qualitative data analysis: a methods sourcebook . 3rd ed. Thousand Oaks, CA: Sage; 2014.
- Silver C, Lewins A. Using software in qualitative research: a step-by-step guide . Thousand Oaks, CA; Sage: 2014.
< Previous Chapter 9: Optimizing Epidemiology– Laboratory Collaborations
Next Chapter 11: Developing Interventions >
The fellowship application period and host site application period are closed.
For questions about the EIS program, please contact us directly at [email protected] .
- Laboratory Leadership Service (LLS)
- Fellowships and Training Opportunities
- Division of Workforce Development
- Methodology
- Research Methodology
- Qualitative Research
Data Collection for Qualitative Research
- January 2020
- In book: Research Methods in Business Studies (pp.95-128)

- University of Birmingham
- This person is not on ResearchGate, or hasn't claimed this research yet.
Discover the world's research
- 25+ million members
- 160+ million publication pages
- 2.3+ billion citations

- Guilherme Marques Pereira
- Recruit researchers
- Join for free
- Login Email Tip: Most researchers use their institutional email address as their ResearchGate login Password Forgot password? Keep me logged in Log in or Continue with Google Welcome back! Please log in. Email · Hint Tip: Most researchers use their institutional email address as their ResearchGate login Password Forgot password? Keep me logged in Log in or Continue with Google No account? Sign up
Extract insights from Interviews. At Scale.
Forms of data analysis in qualitative research: methods explained.
Home » Forms of data analysis in qualitative research: Methods explained
Qualitative Analysis Methods serve as essential tools in the exploration of human experiences and perceptions, providing rich insights that quantitative data often overlook. In qualitative research, the emphasis lies not solely on numbers but on understanding the deeper meanings behind participants' responses. This approach allows researchers to capture the nuances of human behavior, making it invaluable in fields like social sciences, psychology, and market research.
Many researchers face challenges in analyzing qualitative data due to its complex nature. Traditional analytical methods may fall short, particularly when dealing with vast amounts of unstructured data. Effective qualitative analysis requires an adaptable framework that encourages critical thinking and creativity. By mastering various qualitative analysis methods, researchers can transform raw data into actionable insights that can drive informed decision-making and strategy.
Popular Qualitative Analysis Methods
Qualitative analysis methods are essential for transforming raw data into meaningful insights. Researchers employ various approaches to unravel intricate patterns and themes within qualitative data. Popular qualitative analysis methods include thematic analysis, grounded theory, and discourse analysis, among others.
Thematic analysis is a widely used method that identifies and interprets patterns across qualitative data. It allows researchers to extract important themes that arise from interviews, focus groups, or open-ended survey responses. Grounded theory, on the other hand, emphasizes generating theories through the systematic gathering and analysis of data, ensuring that findings are grounded in the participants' perspectives. Lastly, discourse analysis focuses on the language and communication used in interactions, providing insights into the social context and power dynamics present in conversations. Understanding these methods equips researchers with the tools needed to analyze qualitative data effectively, leading to richer interpretations and actionable insights.
Thematic Analysis in Qualitative Research
Thematic analysis is a pivotal method in qualitative research, enabling researchers to systematically identify and analyze themes within data. This analytical approach allows for a deeper understanding of patterns and experiences expressed in qualitative data, such as interviews or focus groups. By focusing on recurring themes, researchers can extract significant insights that reflect participants' viewpoints and social contexts.
To conduct effective thematic analysis, researchers often follow a structured process. First, they familiarize themselves with the data through careful reading and re-reading. Next, they generate initial codes by highlighting important features related to the research question. Following this, they search for themes by collating codes into groups, then review themes for coherence and relevance. Finally, they define and name each theme, providing a comprehensive report that illustrates the findings clearly.
This approach serves as a robust qualitative analysis method, offering vital insights into complex behavioral and emotional dynamics. Its versatility allows it to be adapted across various fields, making it essential for researchers seeking depth in their qualitative inquiries.
Content Analysis in Qualitative Studies
Content analysis is a systematic approach used in qualitative studies to interpret and understand textual data. Researchers explore documents, interviews, and various forms of media to identify patterns, themes, and meaningful insights. This method can reveal underlying meanings and categorize data, making it an essential tool in qualitative analysis methods.
To conduct effective content analysis, researchers generally follow these key steps. First, they define the research question clearly to guide their analysis. Next, they select relevant texts or media that will provide rich data. Third, they create coding schemes that categorize the data, helping to organize findings. Finally, researchers interpret the results, drawing conclusions that aid in understanding the broader context of the data. By engaging in content analysis, scholars and practitioners can make informed decisions grounded in well-analyzed qualitative data.
Advanced Qualitative Analysis Methods
Advanced qualitative analysis methods signify a shift towards more sophisticated approaches in understanding qualitative data. These methods enable researchers to interpret complex patterns and derive deeper insights from extensive narrative data. Regardless of the specific qualitative analysis methods employed, their common goal is to enhance the understanding of human behavior and societal trends.
A few advanced qualitative analysis methods include thematic analysis, grounded theory, and content analysis. Thematic analysis focuses on identifying themes across qualitative data, offering a framework to interpret information systematically. Grounded theory is an iterative process that develops theories based on data collected, allowing for a rich understanding of contexts over time. Content analysis systematically categorizes verbal or textual data, providing quantifiable insights along with qualitative depth. By applying these methods effectively, researchers can create a more comprehensive narrative that informs decision-making and strategy development in various fields.
Grounded Theory Approach
The Grounded Theory Approach is a systematic methodology used in qualitative analysis methods that allows researchers to generate theories based on collected data. This technique considers data collection, coding, and analysis as interrelated processes, helping researchers identify patterns and develop insights that emerge from the data. Instead of testing a pre-existing theory, this method builds theories from the ground up, reflecting the realities of the participants' experiences.
In this context, the approach involves several key steps. First, researchers should begin with open coding, which involves breaking down data into distinct parts to identify themes. Next, they use axial coding to connect these themes, exploring how they interact. Finally, selective coding helps researchers consolidate their findings into a coherent theory. By employing this iterative process, the Grounded Theory Approach facilitates a deep understanding of complex phenomena in qualitative research.
Phenomenological Analysis
Phenomenological analysis focuses on understanding the essence of individuals' lived experiences. This qualitative analysis method emphasizes the subjective perception of experiences, allowing researchers to capture deep insights that quantitative methods often overlook. By exploring how individuals interpret their experiences, phenomenological analysis provides a nuanced understanding of complex phenomena.
To conduct phenomenological analysis effectively, researchers typically follow several key steps. First, they immerse themselves in the data by engaging with participants' narratives through interviews or open-ended responses. Next, they identify significant statements that highlight essential themes within the shared experiences. After categorizing these themes, researchers interpret the findings to reveal underlying meanings relevant to the study's objectives. This structured approach enables a rich exploration of human experiences, enhancing the validity and depth of qualitative analysis methods used in research.
Conclusion on Forms of Qualitative Analysis Methods
Qualitative Analysis Methods provide a rich understanding of human experiences and social phenomena. They emphasize depth over breadth, allowing researchers to explore nuanced insights that quantitative methods may overlook. Through various strategies, such as interviews and focus groups, these methods glean valuable data from participants, shedding light on their emotions, behaviors, and perspectives.
In conclusion, employing qualitative analysis enhances the breadth of data interpretation, unveiling complex themes and patterns. As researchers continue to utilize these methods, they reveal that human-centric insights are critical for informed decision-making. Ultimately, embracing qualitative analysis fosters a deeper connection with the subject, enriching both research outcomes and practical applications.
Turn interviews into actionable insights
On this Page
Top 5 Call Monitoring Services for Quality Assurance: What to Consider
You may also like, generative ai consulting market: key insights.
Generative AI for product development: Top trends
Generative ai for hr: best tools to consider.
Unlock Insights from Interviews 10x faster
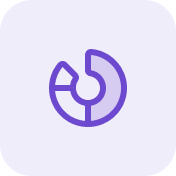
- Request demo
- Get started for free
Promoting higher education students’ self-regulated learning through learning analytics: A qualitative study
- Open access
- Published: 07 September 2024
Cite this article
You have full access to this open access article
- Riina Kleimola ORCID: orcid.org/0000-0003-2091-2798 1 ,
- Laura Hirsto ORCID: orcid.org/0000-0002-8963-3036 2 &
- Heli Ruokamo ORCID: orcid.org/0000-0002-8679-781X 1
Learning analytics provides a novel means to support the development and growth of students into self-regulated learners, but little is known about student perspectives on its utilization. To address this gap, the present study proposed the following research question: what are the perceptions of higher education students on the utilization of a learning analytics dashboard to promote self-regulated learning? More specifically, this can be expressed via the following threefold sub-question: how do higher education students perceive the use of a learning analytics dashboard and its development as promoting the (1) forethought, (2) performance, and (3) reflection phase processes of self-regulated learning? Data for the study were collected from students ( N = 16) through semi-structured interviews and analyzed using a qualitative content analysis. Results indicated that the students perceived the use of the learning analytics dashboard as an opportunity for versatile learning support, providing them with a means to control and observe their studies and learning, while facilitating various performance phase processes. Insights from the analytics data could also be used in targeting the students’ development areas as well as in reflecting on their studies and learning, both individually and jointly with their educators, thus contributing to the activities of forethought and reflection phases. However, in order for the learning analytics dashboard to serve students more profoundly across myriad studies, its further development was deemed necessary. The findings of this investigation emphasize the need to integrate the use and development of learning analytics into versatile learning processes and mechanisms of comprehensive support and guidance.
Explore related subjects
- Artificial Intelligence
- Digital Education and Educational Technology
Avoid common mistakes on your manuscript.
1 Introduction
Promoting students to become autonomous, self-regulated learners is a fundamental goal of education (Lodge et al., 2019 ; Puustinen & Pulkkinen, 2001 ). The importance of doing so is particularly highlighted in higher education (HE) contexts that strive to prepare its students for highly demanding and autonomous expert tasks (Virtanen, 2019 ). In order to perform successfully in diverse educational and professional settings, students need to take an active, self-initiated role in managing their learning processes, thereby assuming primary responsibility for their educational pursuits. Self-regulated learning (SRL) invites students to actively monitor, control, and regulate their cognition, motivation, and behavior in relation to their learning goals and contextual conditions (Pintrich, 2000 ). In an effort to create a favorable foundation for the development of SRL, many HE institutions have begun to explore and exploit the potential of emerging educational technologies, such as learning analytics (LA).
Despite the growing interest in adopting LA for educational purposes (Van Leeuwen et al., 2022 ), little is known about students’ perspectives on its utilization (Jivet et al., 2020 ; Wise et al., 2016 ). Additionally, there is only limited evidence on using LA to support SRL (Heikkinen et al., 2022 ; Jivet et al., 2018 ; Matcha et al., 2020 ; Viberg et al., 2020 ). Thus, more research is inevitably needed to better understand how students themselves consider the potential of analytics applications from the perspective of SRL. Involving students in the development of LA is particularly important, as they represent primary stakeholders targeted to benefit from its utilization (Dollinger & Lodge, 2018 ; West et al., 2020 ). LA should not only be developed for users but also with them in order to adapt its potential to their needs and expectations (Dollinger & Lodge, 2018 ; Klein et al., 2019 ).
LA is thought to provide a promising means to enhance student SRL by harnessing the massive amount of data stored in educational systems and facilitating appropriate means of support (Lodge et al., 2019 ). It is generally defined as “the measurement, collection, analysis and reporting of data about learners and their contexts, for purposes of understanding and optimising learning and the environments in which it occurs” (Conole et al., 2011 , para. 4). The reporting of such data is typically conducted through learning analytics dashboards (LADs) that aggregate diverse types of indicators about learners and learning processes in a visualized form (Corrin & De Barba, 2014 ; Park & Jo, 2015 ; Schwendimann et al., 2017 ). Recently, there has been a rapid movement into LADs that present analytics data directly to students themselves (Schwendimann et al., 2017 ; Teasley, 2017 ; Van Leeuwen et al., 2022 ). Such analytics applications generally aim to provide students with insights into their study progress as well as support for optimizing learning outcomes (Molenaar et al., 2019 ; Sclater et al., 2016 ; Susnjak et al., 2022 ).
The purpose of this qualitative study is to examine how HE students perceive the use and development of an LAD to promote the different phases and processes of SRL. Instead of taking a course-level approach, this study addresses a less-examined study path perspective that covers the entirety of studies, from the start of an HE degree to its completion. A specific emphasis is placed on such an LAD that students could use both independently across studies and together with their tutor teachers as a component of educational guidance. As analytics applications are largely still under development (Sclater et al., 2016 ), and mainly in the exploratory phase (Schwendimann et al., 2017 ; Susnjak et al., 2022 ), it is essential to gain an understanding of how students perceive the use of these applications as a form of learning support. Preparing students to become efficient self-regulated learners is increasingly—and simultaneously—a matter of helping them develop into efficient users of analytics data.
2 Theoretical framework
2.1 enhancing srl in he.
SRL, which has been the subject of wide research interest over the last two decades (Panadero, 2017 ), is referred to as “self-generated thoughts, feelings, and actions that are planned and cyclically adapted to the attainment of personal goals” (Zimmerman, 1999 , p. 14). Self-regulated students are proactive in their endeavors to learn, and they engage in diverse, personally initiated metacognitive, motivational, and behavioral processes to achieve their goals (Zimmerman, 1999 ). They master their learning through covert, cognitive means but also through behavioral, social, and environmental approaches that are reciprocally interdependent and interrelated (Zimmerman, 1999 , 2015 ), thus emphasizing the sociocognitive views on SRL (Bandura, 1986 ).
When describing and modelling SRL, researchers have widely agreed on its cyclical nature and its organization into several distinct phases and processes (Panadero, 2017 ; Puustinen & Pulkkinen, 2001 ). In the well-established model by Zimmerman and Moylan ( 2009 ), SRL occurs in the cyclic phases of forethought, performance, and self-reflection that take place before, during, and after students’ efforts to learn. In the forethought phase, students prepare themselves for learning and approach the learning tasks through the processes of planning and goal setting, and the activation of self-motivation beliefs, such as self-efficacy perceptions, outcome expectations, and personal interests. Next, in the performance phase, they carry out the actual learning tasks and make use of self-control processes and strategies, such as self-instruction, time management, help-seeking, and interest enhancement. Moreover, they keep records of their performance and monitor their learning, while promoting the achievement of desired goals. In the final self-reflection phase, students participate in the processes of evaluating their learning and reflecting on the perceived causes of their successes and failures, which typically results in different types of cognitive and affective self-reactions as responses to such activity. This phase also forms the basis for the approaches to be adjusted for and applied in the subsequent forethought phase, thereby completing the SRL cycle. The model suggests that the processes in each phase influence the following ones in a cyclical and interactive manner and provide feedback for subsequent learning efforts (Zimmerman & Moylan, 2009 ; Zimmerman, 2011 ). Participation in these processes allows students to become self-aware, competent, and decisive in their learning approaches (Kramarski & Michalsky, 2009 ).
Although several other prevalent SRL models with specific emphases also exist (e.g., Pintrich, 2000 ; Winne & Hadwin, 1998 ; for a review, see Panadero, 2017 ), the one presented above provides a comprehensive yet straightforward framework for identifying and examining the key phases and processes related to SRL (Panadero & Alonso-Tapia, 2014 ). Developing thorough insights into the student SRL is especially needed in an HE context, where the increase in digitized educational settings and tools requires students to manage their learning in a way that is autonomous and self-initiated. When pursuing an HE degree, students are expected to engage in the cyclical phases and processes of SRL as a continuous effort throughout their studies. Involvement in SRL is needed not only to successfully perform a single study module, course, or task but also to actively promote the entirety of studies throughout semesters and academic years. It therefore plays a central role in the successful completion of HE studies.
From the study path perspective, the forethought phase requires HE students to be active in the directing and planning of their studies and learning—that is, setting achievable goals, making detailed plans, finding personal interests, and trusting in their abilities to complete the degree. The performance phase, in turn, invites students to participate in the control and observation of their studies and learning. While completing their studies, they must regularly track study performance, visualize relevant study information, create functional study environments, maintain motivation and interest, and seek and receive productive guidance. The reflection phase, on the other hand, involves students in evaluating and reflecting on their studies and learning—that is, analyzing their learning achievements and processing resulting responses. These activities typically occur as overlapping, cyclic, and connected processes and as a continuum across studies. Additionally, the phases may appear simultaneously, as students strive to learn and receive feedback from different processes (Pintrich, 2000 ). The processes may also emerge in more than one phase (Panadero & Alonso-Tapia, 2014 ), and boundaries between the phases are not always that precise.
SRL is shown to benefit HE students in various ways. Research has evidenced, for instance, that online students who use their time efficiently, are aware of their learning behavior, think critically, and show efforts to learn despite challenges are likely to achieve academic success when studying in online settings (Broadbent & Poon, 2015 ). SRL is also shown to contribute to many non-academic outcomes in HE blended environments (for a review, see Anthonysamy et al., 2020 ). Despite this importance, research (e.g., Azevedo et al., 2004 ; Barnard-Brak et al., 2010 ) has indicated that students differ in their ways to self-regulate, and not all are competent self-regulated learners by default. As such, many students would require and benefit from support to develop their SRL (Moos, 2018 ; Wong et al., 2019 ).
Supporting student SRL is generally considered the responsibility of a teaching staff (Callan et al., 2022 ; Kramarski, 2018 ). It can also be a specific task given to tutor teachers assigned to each student or to a group of students for particular academic years. Sometimes referred to as advisors, they are often teachers of study programs who aim to help students in decision-making, study planning, and career reflection (De Laet et al., 2020 ), while offering them guidance and support for the better management of learning. In recent years, efforts have also been made to promote student SRL with educational technologies such as LA (e.g., Marzouk et al., 2016 ; Wise et al., 2016 ). LA is used to deliver insights for students themselves to better self-regulate their learning (e.g., Jivet et al., 2021 ; Molenaar et al., 2019 ), and also to facilitate the interaction between students and guidance personnel (e.g., Charleer et al., 2018 ). It is generally thought to promote the development of future competences needed by students in education and working life (Kleimola & Leppisaari, 2022 ), and to offer novel insights into their motivational drivers (Kleimola et al., 2023 ).
2.2 LA as a potential tool to promote SRL
Much of the recent development in the field of LA has focused on the design and implementation of LADs. In general, their purpose is to support sensemaking and encourage students and teachers to make informed decisions about learning and teaching processes (Jivet et al., 2020 ; Verbert et al., 2020 ). Schwendimann and colleagues ( 2017 ) refer to an LAD as a “display that aggregates different indicators about learner(s), learning process(es) and/or learning context(s) into one or multiple visualizations” (p. 37). Such indicators may provide information, for instance, about student actions and use of learning contents on a learning platform, or the results of one’s learning performance, such as grades (Schwendimann et al., 2017 ). Data can also be extracted from educational institutions’ student information systems to provide students with snapshots of their study progress and access to learning support (Elouazizi, 2014 ). While visualizations enable intuitive and quick interpretations of educational data (Papamitsiou & Economides, 2015 ), they additionally require careful preparation, as not all users may necessarily interpret them uniformly (Aguilar, 2018 ).
LADs can target various stakeholders, and recently there has been a growing interest in their development for students’ personal use (Van Leeuwen et al., 2022 ). Such displays, also known as student-facing dashboards, are thought to increase students’ knowledge of themselves and to assist them in achieving educational goals (Eickholt et al., 2022 ). They are also believed to promote student autonomy by encouraging students to take control of their learning and by supporting their intrinsic motivation to succeed (Bodily & Verbert, 2017 ). However, simply making analytics applications available to students does not guarantee that they will be used productively in terms of learning (Wise, 2014 ; Wise et al., 2016 ). Moreover, they may not necessarily cover or address the relevant aspects of learning (Clow, 2013 ). Thus, to promote the widespread acceptance and adoption of LADs, it is crucial to consider students’ perspectives on their use as a means of learning support (Divjak et al., 2023 ; Schumacher & Ifenthaler, 2018 ). If students’ needs are not adequately examined and met, such analytics applications may fail to encourage or even hinder the process of SRL (Schumacher & Ifenthaler, 2018 ).
Although previous research on students’ perceptions of LA to enhance their SRL appears to be limited, some studies have addressed such perspectives. Schumacher and Ifenthaler ( 2018 ) found that HE students appreciated LADs that help them plan and initiate their learning activities with supporting elements such as reminders, to-do lists, motivational prompts, learning objectives, and adaptive recommendations, thus promoting the forethought phase of SRL. The students in their study also expected such analytics applications to support the performance phase by providing analyses of their current situation and progress towards goals, materials to meet their individual learning needs, and opportunities for learning exploration and social interaction. To promote the self-reflection phase, the students anticipated LADs to allow for self-assessment, real-time feedback, and future recommendations but were divided as to whether they should receive comparative information about their own or their peers’ performance (Schumacher & Ifenthaler, 2018 ). Additionally, the students desired analytics applications to be holistic and advanced, as well as adaptable to individual needs (Schumacher & Ifenthaler, 2018 ).
Somewhat similar notions were made by Divjak and colleagues ( 2023 ), who discovered that students welcomed LADs that promote short-term planning and organization of learning but were wary of making comparisons or competing with peers, as they might demotivate learners. Correspondingly, De Barba et al. ( 2022 ) noted that students perceived goal setting and monitoring of progress from a multiple-goals approach as key features in LADs, but they were hesitant to view peer comparisons, as they could promote unproductive competition between students and challenge data privacy. In a similar vein, Rets et al. ( 2021 ) reported that students favored LADs that provide them with study recommendations but did not favor peer comparison unless additional information was included. Roberts et al. ( 2017 ), in turn, stressed that LADs should be customizable by students and offer them some level of control to support their SRL. Silvola et al. ( 2023 ) found that students perceived LADs as supportive for their study planning and monitoring at a study path level but also associated some challenges with them in terms of SRL. Further, Bennett ( 2018 ) found that students’ responses to receiving analytics data varied and were highly individual. There were different views, for instance, on the potential of analytics to motivate students: although it seemed to inspire most students, not all students felt the same way (Bennett, 2018 ; see also Corrin & De Barba, 2014 ; Schumacher & Ifenthaler, 2018 ). Moreover, LADs were reported to evoke varying affective responses in students (Bennett, 2018 ; Lim et al., 2021 ).
To promote student SRL, it is imperative that LADs comprehensively address and support all phases of SRL (Schumacher & Ifenthaler, 2018 ). However, a systematic literature review conducted by Jivet et al. ( 2017 ) indicated that students were often offered only limited support for goal setting and planning, and comprehensive self-monitoring, as very few of the LADs included in their study enabled the management of self-set learning goals or the tracking of study progress over time. According to Jivet et al. ( 2017 ), this might indicate that most LADs were mainly harnessed to support the reflection and self-evaluation phase of SRL, as the other phases were mostly ignored. Somewhat contradictory results were obtained by Viberg et al. ( 2020 ), whose literature review revealed that most studies aiming to measure or support SRL with LA were primarily focused on the forethought and performance phases and less on the reflection phase. Heikkinen et al. ( 2022 ) discovered that not many of the studies combining analytics-based interventions and SRL processes covered all phases of SRL.
It appears that further development is inevitably required for LADs to better promote student SRL as a whole. Similarly, there is a demand for their tight integration into pedagogical practices and learning processes to encourage their productive use (Wise, 2014 ; Wise et al., 2016 ). One such strategy is to use these analytics applications as a part of guidance activity and as a joint tool for both students and guidance personnel. In the study by Charleer et al. ( 2018 ), the LAD was shown to trigger conversations and to facilitate dialogue between students and study advisors, improve the personalization of guidance, and provide insights into factual data for further interpretation and reflection. However, offering students access to an LAD only during the guidance meeting may not be sufficient to meet their requirements for the entire duration of their studies. For instance, Charleer and colleagues ( 2018 ) found that the students were also interested in using the LAD independently, outside of the guidance context. Also, it seems that encouraging students to actively advance their studies with such analytics applications necessitates a student-centered approach and holistic development through research. According to Rets et al. ( 2021 ), there is a particular call for qualitative insights, as many previous LAD studies that included students have primarily used quantitative approaches (e.g., Beheshitha et al., 2016 ; Divjak et al., 2023 ; Kim et al., 2016 ).
2.3 Research questions
The purpose of this qualitative study is to examine how HE students perceive the utilization of an LAD in SRL. A specific emphasis was placed on its utilization as part of the forethought, performance, and reflection phase processes, considered central to student SRL. The main research question (RQ) and the threefold sub-question are as follows:
RQ: What are the perceptions of HE students on the utilization of an LAD to promote SRL?
How do HE students perceive the use of an LAD and its development as promoting the (1) forethought, (2) performance, and (3) reflection phase processes of SRL?
3.1 Context
The study was conducted in a university of applied sciences (UAS) in Finland that had launched an initial version of an LAD to be piloted together with its students and tutor teachers as a part of the guidance process. The LAD was descriptive in nature and consisted of commonly available analytics data and simple analytics indicators showing an individual student’s study progress and success in a study path. As is typical for descriptive analytics, it offered insights to better understand the past and present (Costas-Jauregui et al., 2021 ) while informing the future action (Van Leeuwen et al., 2022 ). The data were extracted from the UAS’ student information system and presented using Microsoft Power BI tools. No predictive or comparative information was included. The main display of the LAD consisted of three data visualizations and an information bar (see Fig. 1 , a–d), all presented originally in Finnish. Each visualization could also be expanded into a single display for more accurate viewing.
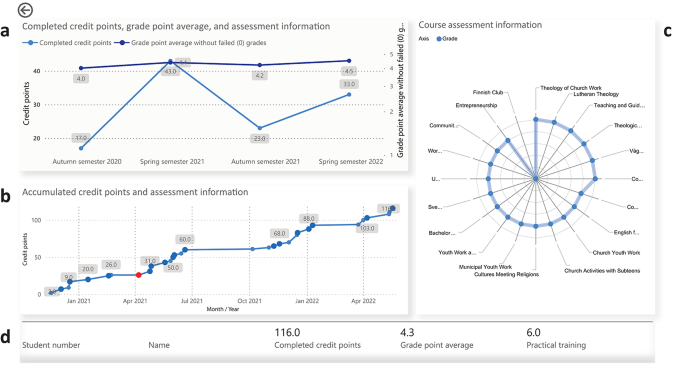
An example of the main display of the piloted LAD with data visualizations ( a – c ) and an information bar ( d )
First, the LAD included a data visualization that illustrated a student’s study progress and success per semester using a line chart (Fig. 1 , a). It displayed the scales for total number of credit points (left) and grade point averages (right) for courses completed on a semester timeline. Data points on the chart displayed an individual student’s study performance with respect to these indicators in each semester and were connected to each other with a line. Pointing to one of these data points also opened a data box that indicated the student name and information about courses (course name, scope, grade, assessment date) from which the credit points and grade point averages were obtained.
Second, the LAD contained another type of line chart that indicated a student’s individual study progress over time in more detail (Fig. 1 , b). The chart displayed a timeline with three-month periods and illustrated a scale for the accumulated credit points. Data points on the chart indicated the accumulated number of credit points obtained from the courses and appeared in blue if the student had passed the course(s) and in red if the student had failed the course(s) at that time. As with the line chart above it, the data points in this chart also provided more detailed information about the courses behind the credit points and were intertwined with a line.
Third, the LAD offered information related to a student’s study success through a radar chart (Fig. 1 , c). The chart represented the courses taken by the student and displayed a scale for the grades received from them. The lowest grade was placed in the center of the chart and the highest one on its outer circle. The grades in between were scaled on the chart accordingly, and the courses performed with a similar grade were displayed close to each other. Data points on the chart represented the grades obtained from numerically evaluated courses and were merged with a line. Each data point also had a data box with the course name and the grade obtained.
Fourth, the LAD included an information bar (Fig. 1 , d) that displayed the student number and the student name (removed from the figure), the total number of accumulated credit points, the grade point average for passed courses, and the amount of credit points obtained from practical training.
The LAD was piloted in authentic guidance meetings in which a tutor teacher and a student discussed topical issues related to the completion of studies. Such meetings were a part of the UAS’ standard guidance discussions that were typically held 1–2 times during the academic year, or more often if needed. In the studied meetings, the students and tutor teachers collectively reviewed the LAD to support the discussion. Only the tutor teachers were commonly able to access the LAD, as it was still under development and in the pilot phase. However, the students could examine its use as presented by the tutor teacher. In addition to the LAD, the meeting focused on reviewing the student’s personal study plan, which contained information about their studies to be completed and could be viewed through the student information system. Most of the meetings were organized online, and their duration varied according to an individual student’s needs. A researcher (first author) attended the meetings as an observer.
3.2 Participants and procedures
Participants were HE students ( N = 16) pursuing a bachelor’s degree at the Finnish University of Applied Sciences (UAS), ranging from 21 to 49 years of age (mean = 30.38, median = 29.5); 11 (68.75%) were female, and 5 (31.25%) were male. HE studies commenced between 2016 and 2020, and comprised different academic fields, including business administration, culture, engineering, humanities and education, and social services and health care. Depending on the degree, study scope ranged from 210 to 240 ECTS credit points, which take approximately three and a half to four years to complete. However, the students could also proceed at a faster or slower pace under certain conditions. The students were selected to represent different study fields and study stages, and to have studied for more than one academic year. Informed consent to participate in the study was obtained from all students, and their participation was voluntary. The research design was approved by the respective UAS.
Data for this qualitative study was collected through semi-structured, individual student interviews conducted in April–September 2022. To address certain topics in each interview, an interview guide was used. The interview questions incorporated into the guide were tested in two student test interviews to simulate a real interview situation and to assure intelligibility, as also suggested by Chenail ( 2011 ). Findings indicated that the questions were largely usable, functional, and understandable, but some had to be slightly refined to ensure their conciseness and to improve clarity and familiarity of expressions vis-à-vis the target group. Also, the order of questions was partly reshaped to support the flow of discussion.
In the interviews, the students were asked to provide information about their demographic and educational backgrounds as well as their overall opinions of educational practices and the use of LA. In particular, they were invited to share their views on the use of the piloted LAD and its development as promoting different phases and processes of SRL. Students’ perceptions were generally based on the assumption that they could use the LAD both independently during their studies and collectively with their tutor teachers as a component of the guidance process.
Interviews were conducted immediately or shortly after the guidance meeting. Interview duration ranged from 42 to 70 min. The graphical presentation of the LAD was commonly shown to the students to provide stimuli and evoke discussion, as suggested by Kwasnicka et al. ( 2015 ). The interviews were conducted by the same researcher (first author) who observed the guidance meetings. They were primarily held online, and only one was organized face-to-face. All interviews were video recorded for subsequent analysis.
3.3 Data analysis
Interview recordings were transcribed verbatim, accumulating a total of 187 pages of textual material for analysis (Times New Roman, 12-point font, line spacing 1). A qualitative content analysis method was used to analyze the data (see Mayring, 2000 ; Schreier, 2014 ) to enhance in-depth understanding of the research phenomenon and to inform practical actions (Krippendorf, 2019 ). Also, data were approached both deductively and inductively (see Elo & Kyngäs, 2008 ; Merriam & Tisdell, 2016 ), and the analysis was supported using the ATLAS.ti program.
Analysis began with a thorough familiarization with the data in order to develop a general understanding of the students’ perspectives. First, the data were deductively coded using Zimmerman and Moylan’s ( 2009 ) SRL model as a theoretical guide for analysis and as applied to the study path perspective. All relevant units of analysis—such as paragraphs, sentences, or phrases that addressed the use of the LAD or its development in relation to the processes of SRL presented in the model—were initially identified from the data, and then sorted into meaningful units with specific codes. The focus was placed on instances in the data that were applicable and similar to the processes represented in the model, but the analysis was not limited to those that fully corresponded to them. The preliminary analysis involved several rounds of coding that ultimately led to the formation of main categories, grouped into the phases of SRL. The forethought phase consisted of processes that emphasized the planning and directing of studies and learning with the LAD. The performance phase, in turn, involved processes that addressed the control and observation of studies and learning through the LAD. Finally, the reflection phase included processes that focused on evaluating and reflecting on studies and learning with the LAD.
Second, the data were approached inductively by examining the use of the LAD and its development as distinct aspects within each phase and process of SRL (i.e., the main categories). The aim was, on the one hand, to identify how the use of the LAD was considered to serve the students in the phases and processes of SRL in its current form, and on the other hand, how it should be improved to better support them. The analysis not only focused on the characteristics of the LAD but also on the practices that surrounded its use and development. The units of analysis were first condensed from the data and then organized into subcategories for similar units. As suggested by Schreier ( 2014 ), the process was continued until a saturation point was reached—that is, no additional categories could be found. As a result, subcategories for all of the main categories were identified.
Following Schreier’s ( 2014 ) recommendation, the categories were named and described with specific data examples. Additionally, some guidelines were added to highlight differences between categories and to avoid overlap. Using parts of this categorization framework as a coding scheme, a portion of the data (120 text segments) was independently coded into the main categories by the first and second authors. The results were then compared, and all disagreements were resolved through negotiation until a shared consensus was reached. After minor changes were made to the coding scheme, the first author recoded all data. The number of students who had provided responses to each subcategory was counted and added to provide details on the study. For study integrity, the results are supported by data examples with the students’ aliases and the study fields they represented. The quotations were translated from Finnish to English.
The results are reported by first answering the threefold sub-question, that is, how do HE students perceive the use of an LAD and its development as promoting the (1) forethought, (2) performance, and (3) reflection phase processes of SRL. The subsequent results are then summarized to address the main RQ, that is, what are the main findings on HE students’ perceptions on the utilization of an LAD to promote SRL.
4.1 LAD as a part of the forethought phase processes
The students perceived the use of the LAD and its development as related to the forethought phase processes of SRL through the categorization presented in Table 1 below.
Regarding the process of goal setting , almost all students ( n = 15) emphasized that the use of the LAD promoted the targeting of goal-oriented study completion and competence development. Analytics indicators—such as grades, grade point averages, and accumulated credit points—adequately informed the students of areas they should aim for, further improve, or put more effort into. Only one student ( n = 1) considered the analytics data too general for establishing goals. However, some students ( n = 7) specifically mentioned their desire to set and enter individual goals in the LAD. The students were considered to have individual intentions, which should also be made visible in the LAD:
For example, someone might complete [their studies] in four years, someone might do [them] even faster, so maybe in a way that there is the possibility…to set…that, well, I want or my goal is to graduate in this time, and then it would kind of show in it. (Sophia, Humanities and Education student)
Moreover, some students ( n = 6) wanted to obtain information on the degree program’s overall target times, study requirements, or pace recommendations through the LAD.
In relation to the process of study planning , the use of the LAD provided many students ( n = 8) grounds to plan and structure the promotion and completion of their studies, such as which courses and types of studies to choose, and what kind of study pace and schedule to follow. However, an even greater set of students ( n = 12) hoped that the LAD could provide them with more sophisticated tools for planning. For instance, it could inform them about studies to be completed, analyze their study performance in detail, or make predictions for the future. Moreover, it should offer them opportunities to choose courses, make enrollments, set schedules, get reminders, and take notes. One example of such an advanced analytics application was described as follows: ‟It would be a bit like a conversational tool with the student as well, that you would first put…your studies in the program, so it would [then] remind you regularly that hey, do this” (James, Humanities and Education student).
When discussing the use of the LAD, most students ( n = 12) emphasized the critical role of personal interests and preferences , which was found to not only guide studying and learning in general but to also drive and shape the utilization of the LAD. According to the students, using such an analytics application could particularly benefit those students who, for instance, prefer monitoring of study performance, perceive information in a visualized form, are interested in analytics or themselves, or find it relevant for their studies. Prior familiarization was also considered useful: ‟Of course, there are those who use this kind of thing more and those who use this kind of thing in daily life, so they could especially benefit from this, probably more than I do” (Olivia, Social Services and Health Care student). Even though the LAD was considered to offer pertinent insights for many types of learners, it might not be suitable for all. For instance, it could be challenging for some students to comprehend analytics data or to make effective use of them in their studies. In the development of the LAD, such personal aspects should be noted. The students ( n = 7) believed the LAD might better adapt to students’ individual needs if it allows them to customize its features and displays or to use it voluntarily based on one’s personal interests and needs.
When describing the use of LAD, half of the students ( n = 8) discussed its connections with self-efficacy . Making use of analytics data appeared to strengthen the students’ beliefs in their abilities to study and learn in a targeted manner, even if their own feelings suggested otherwise. As one of the students stated:
It’s nice to see that progress, that it has happened although it feels that it hasn’t. So, you can probably set goals based on [an idea] that you’re likely to progress, you could set [them] that you could graduate sometime. (Emma, Engineering student)
On the other hand, the use of the LAD also seemed to require students to have sufficient self-efficacy. It was perceived as vital especially when the analytics data showed unfavorable study performance, such as failed or incomplete courses, or gaps in the study performance with respect to peers. One student ( n = 1) suggested that the LAD could include praises as evidence of and support for appropriate study performance. Such incentives may help improve the students’ self-confidence as learners. Apart from this, however, the students had no other recommendations for developing the use of the LAD to support self-efficacy.
4.2 LAD as a part of the performance phase processes
The students discussed the use of the LAD and its development in relation to the performance phase processes of SRL according to the categories described in Table 2 below.
The students ( n = 16) widely agreed that using the LAD benefited them in the process of metacognitive monitoring. By indicating the progress and success of study performance, the LAD was thought to be well suited for observing the course of studies and the development of competences. Moreover, it helped the students to gain awareness of their individual strengths and weaknesses, as well as successes and failures, in a study path. Tracking individual study performance was also found to contribute to purposeful study completion, as the following data example demonstrates:
It’s important especially when there is a target time to graduate, so of course you must follow and stay on track in many ways as there are many such pitfalls to easily fall into, [and] as I’ve fallen quite many times, it’s good [to monitor]. (Sarah, Culture student)
Additionally, the insights of monitoring could be used in future job searches to provide information about acquired competences to potential employers. The successful promotion of studies was generally perceived to require regular monitoring by both students and their educators. However, one of the students considered it a particular responsibility of the students themselves, as the studies were completed at an HE level and were thus voluntary for them. To provide more in-depth insights, many students ( n = 12) recommended the incorporation of a course-level monitoring opportunity in the LAD. More detailed information was needed, for instance, about course descriptions, assignments completed, and grades received. The rest of the students ( n = 4), however, wanted to keep the course-level monitoring within the learning management system. One of them stated that it could also be a place through which the students could use the LAD. Some students ( n = 6) emphasized the need to reconsider current assessment practices to enable better tracking of study performance. Specifically, assessments could be made in greater detail and grades given immediately after course completion. The variation in scales and time points of assessments between the courses and degree programs posed potential challenges for monitoring, thus prompting the need to unify educational practices at the organizational level.
As an activity closely related to metacognitive monitoring, the process of imaging and visualizing was emphasized by the students as helping them to advance in their educational pursuits. Most students ( n = 15) mentioned that using the LAD allowed them to easily image their study path and clarify their study situation. As one of them stated, ‟This is quite clear, this like, that you can see the overall situation with a quick glance” (Anna, Business Administration student). The visualizations were perceived as informative, tangible, and understandable. However, they were also thought to carry the risk of students neglecting some other relevant aspects of studying and learning in the course of attracting such focused attention. Although the visualizations were generally considered clear, some students ( n = 11) noted that they could be further improved to better organize the analytics data. For instance, the students suggested the attractive use of colors and the categorization of different types of courses. Visual symbols, in turn, may be particularly effective in course-level data. Technical aspects should also be carefully considered to avoid false visualizations.
Regarding the process of environmental structuring , the LAD appeared to be a welcome addition to the study toolkit and overall study environment. A few students ( n = 4) considered it appropriate to utilize the LAD as a separate PowerBI application alongside other (Microsoft O365) study tools, but they also felt that it could be utilized through other systems if necessary. However, one student ( n = 1) raised the need for overall system integrations and some students ( n = 8) expressed a specific wish to use the LAD as an integrated part of the student information system that was thought to improve its accessibility. A few students ( n = 6) also wanted to receive some additional analytics data as related to the information stored in such a system. For instance, the students could be informed about their study progress or offered feedback on their overall performance in relation to the personal study plan. Other students ( n = 10), in turn, did not consider the need for this or did not mention it. It was generally emphasized that the LAD should remain sufficiently clear and simple, as too much information can make its use ineffective:
I think there is just enough information in this. Of course, if you would want to add something small, you could, but I don’t know how much, because I feel that when there is too much information, so it’s a bit like you can’t get as much out of it as you could get. (Olivia, Social Services and Health Care student)
Moreover, the analytics data must be kept private and protected. The students generally desired personal access to the LAD; if given such an opportunity, almost all ( n = 15) believed they would utilize it in the future, and only one ( n = 1) was unsure about this prospect. The analytics data were believed to be of particular use when studies were actively promoted. Hence they should be made available to the students from the start of their studies.
Regarding the process of interest and motivation enhancement , all students ( n = 16) mentioned that using the LAD stimulated their interest or enhanced their motivation, although to varying degrees. For some students, a general tracking of studies was enough to encourage them to continue their pursuits, while others were particularly inspired by seeing either high or low study performance. The development of motivation and interest was generally thought to be a hindrance if the students perceived the analytics data as unfavorable or lacking essential information. As one of students mentioned, ‟If your [chart] line was downward, and if there were only ones and zeros or something like that, it could in a way decrease the motivation” (Helen, Humanities and Education student). It appeared that enhancing interest and motivation was mainly dependent on the students’ own efforts to succeed in their course of study and thus to generate favorable analytics data. However, some students ( n = 7) felt that it could be additionally enhanced by diversifying and improving the analytics tools in the LAD. For example, the opportunities for more detailed analyses and future study planning or comparisons of study performance with that of peers might further increase these students’ motivation and interest in their studies. Even so, it was also considered possible that especially comparisons between students might have the opposite, demotivating and discouraging effect.
All students ( n = 16) mentioned that using the LAD facilitated the process of seeking and accessing help . It enabled the identification of potential support needs—for instance, if several courses were failed or left unfinished. As noted, they were perceived as alarming signals for the students themselves to seek help and for the guidance personnel to provide targeted support. As one of the students emphasized, it was important that not only ‟a teacher [tutor] gets interested in looking at what the situation is but also that a student would understand to communicate regarding the promotion of studies and situations” (Emily, Social Services and Health Care student). Some students ( n = 9) suggested that the students, tutor teachers, or both could receive automated alerts if concerns were to arise. On the other hand, the impact of such automated notifications on changing the course of study was considered somewhat questionable. Above all, the students ( n = 16) preferred human contact and personal support by the guidance personnel, who would use a sensitive approach to address possibly delicate issues. Support would be important to include in existing practices, as the tutor teachers should not be overburdened. One of the students also stated that the automated alerts could be sufficient if they just worked effectively.
4.3 LAD as a part of the reflection phase and processes
The students addressed the use of the LAD and its development as a part of the reflection phase processes of SRL through categories outlined in Table 3 .
The students widely appreciated the support provided by the use of the LAD for the process of evaluation and reflection. The majority ( n = 15) mentioned that it allowed them to individually reflect on the underlying aspects of their study performance, such as what kind of learners they are, what type of teaching or learning methods suit them, and what factors impact their learning. Similarly, the students ( n = 16) valued the possibility of examining the analytics data together with the guidance personnel, such as tutor teachers, and commonly expressed a desire to revisit the LAD in future guidance meetings. It was thought to promote the interpretation of analytics data and to facilitate collective reflection on the reasons behind one’s study success or failure. However, this might require a certain orientation from the guidance personnel, as the student describes below:
I feel that it’s possible to address such themes that what may perhaps cause this. Of course, a lot depends on how amenable the teacher [tutor] is, like are we focusing on how the studies are going but in a way, not so much on what may cause it. (Sophia, Humanities and Education student)
Some students ( n = 8) proposed incorporating familiarization with analytics insights into course implementations of the degree programs. Additionally, many students ( n = 11) expressed a desire to examine the student group’s general progress in tutoring classes together with the tutor teacher and peers, particularly if the results were properly targeted and anonymized, and presented in a discreet manner. However, some students ( n = 5) found this irrelevant. The students were generally wary to evaluate and compare an individual student’s study performance in relation to the peer average through the LAD. While some students ( n = 4) welcomed such an opportunity, others ( n = 6) considered it unnecessary. A few students ( n = 5) emphasized that such comparisons between students should be optional and visible if desired, and one student ( n = 1) did not have a definite view about it. Rather than competing with others, the students stressed the importance of challenging themselves and evaluating study performance against their own goals or previous achievements.
According to the students ( n = 16), the use of the LAD was associated with a wide range of affective reactions . Positive responses such as joy, relief, and satisfaction were considered to emerge if the analytics data displayed by the LAD was perceived as favorable and expected, and supportive of future learning. Similarly, negative responses such as anxiety, pressure, or stress were likely to occur if such data indicated poor performance, thus challenging the learning process. On the other hand, such self-reactions could also appear as neutral or indifferent, depending on the student and the situation. Individual responses were related not only to the current version of the LAD but also to its further development targets. Some students ( n = 3) pointed out the importance of guidance and support, through which the affective reactions could be processed together with professionals. As one of the students underlined, it is important “that there is also that support for the studies, that it isn’t just like you have this chart, and it looks bad, that try to manage. Perhaps there is that support, support in a significant role as well” (Sophia, Humanities and Education student). It seemed critical that the students were not left alone with the LAD but rather were given assistance to deal with the various responses its use may elicit.
4.4 Summary of findings on LAD utilization to promote SRL among HE students
In summary, HE students’ perceptions on the utilization of an LAD to promote SRL phases and processes were largely congruent, but nonetheless partly varied. In particular, the students agreed on the support provided by the LAD during the performance phase and for the purpose of metacognitive monitoring. Such activity was thought to not only enable the students to observe their studies and learning, but to also create the basis for the emergence of all other processes, which were facilitated by the monitoring. That is, while the students familiarized themselves with the course of their studies via the analytics data, they could further apply these insights—for instance, to visualize study situations, enhance motivation, and identify possible support needs. Monitoring with the LAD was also perceived to partly promote the students to the forethought and reflection phases and processes by giving them grounds to target their development areas as well as to reflect on their studies and learning individually and jointly with their tutor teachers. However, it was clear that less emphasis was placed on using the LAD for study planning, addressing individual interests, activating self-efficacy, and supporting environmental structuring, thus giving incentives for their further investigation and future improvement.
Although the LAD used in this study seemed to serve many functions as such, its holistic development was deemed necessary for more thorough SRL support. In particular, the students agreed on the need to improve such an analytics application to further strengthen the performance phase processes—particularly monitoring—by, for instance, developing it for the students’ independent use, and by integrating it with instructional and guidance practices provided by their educators. Moreover, the students commonly wished for more advanced analytics tools that could more directly contribute to the planning of studies and joint reflection of group-level analytics data. To better support the various processes of SRL, new features were generally welcomed into the LAD, although the students’ views and emphases on them also varied. Mixed perspectives were related, for instance, to the need to enrich data or compare students within the LAD. Thus, it seemed important to develop the LAD to conform to the preferences of its users. Along with improving the LAD, students also paid attention to the development of pedagogical practices and guidance processes that together could create appropriate conditions for the emergence of SRL.
5 Discussion
The purpose of this study was to gain insights into HE students’ perceptions on the utilization of an LAD to promote their SRL. The investigation extended the previous research by offering in-depth descriptions of the specific phases and processes of SRL associated with the use of an LAD and its development targets. By applying a study path perspective, it also provided novel insights into how to promote students to become self-regulated learners and effective users of analytics data as an integral part of their studies in HE.
The students’ perspectives on the use of LAD and its development were initially explored as a part of the forethought phase processes of SRL, with a particular focus on the planning and directing of studies and learning. In line with previous research (e.g., Divjak et al., 2023 ; Schumacher & Ifenthaler, 2018 ; Silvola et al., 2023 ), the students in this study appreciated an analytics application that helped them prepare for their future learning endeavors—that is, the initial phase of the SRL cycle (see Zimmerman & Moylan, 2009 ). Using the LAD specifically allowed the students to recognize their development areas and offered a basis to organize their future coursework. However, improvements to allow students to set individual goals and make plans directly within the LAD, as well as to increase awareness of general degree goals, were also desired. These seem to be pertinent avenues for development, as goals may inspire the students not only to invest greater efforts in learning but also to track their achievements against these goals (Wise, 2014 ; Wise et al., 2016 ). While education is typically entered with individual starting points, it is important to allow the students to set personal targets and routes for their learning (Wise, 2014 ; Wise et al., 2016 ).
The results of this study indicate that the use of LADs is primarily driven and shaped by students’ personal interests and preferences, which commonly play a crucial role in the development of SRL (see Zimmerman & Moylan, 2009 ; Panadero & Alonso-Tapia, 2014 ). It might particularly benefit those students for whom analytics-related activities are characteristic and of interest, and who consider them personally meaningful for their studies. It has been argued that if students consider analytics applications serve their learning, they are also willing to use them (Schumacher & Ifenthaler, 2018 ; Wise et al., 2016 ). On the other hand, it has also been stated that not all students are necessarily able to maximize its possible benefits on their own and might need support in understanding its purpose (Wise, 2014 ) and in finding personal relevance for its use. The findings of this study suggest that a more individual fit of LADs could be promoted by allowing students to customize its functionalities and displays. Comparable results have also been obtained from other studies (e.g., Bennett, 2018 ; Rets et al., 2021 ; Roberts et al., 2017 ; Schumacher & Ifenthaler, 2018 ), thus highlighting the need to develop customized LADs that better meet the needs of diverse students and that empower them to control their analytics data. More attention may also be needed to promote the use and development of LADs to support self-efficacy, as it appeared to be an unrecognized potential still for many students in this study. According to Rets et al. ( 2021 ), using LADs for such a purpose might particularly benefit online learners and part-time students, who often face various requirements and thus may forget the efforts put into learning and giving themselves enough credit. By facilitating students’ self-confidence, it could also promote the necessary changes in study behavior, at least for those students with low self-efficacy (Rets et al., 2021 ).
Second, the students’ views on the use of the LAD and its development were investigated in terms of the performance phase processes of SRL, with an emphasis on the control and observation of studies and learning. In line with the results of other studies (De Barba et al., 2022 ; Rets et al., 2021 ; Schumacher & Ifenthaler, 2018 ; Silvola et al., 2023 ), the students preferred using the LAD to monitor their study performance—they wanted to follow their progress and success over time and keep themselves and their educators up to date. According to Jivet et al. ( 2017 ), such functionality directly promotes the performance phase of SRL. Moreover, it seemed to serve as a basis for other activities under SRL, all of which were heavily dependent and built on the monitoring. The results of this study, however, imply that monitoring opportunities should be further expanded to provide even more detailed insights. Moreover, they indicate the need to develop and refine pedagogical practices at the organizational level in order to better serve student monitoring. As monitoring plays a crucial role in SRL (Zimmerman & Moylan, 2009 ), it is essential to examine how it is related to other SRL processes and how it can be effectively promoted with analytics applications (Viberg et al., 2020 ).
In this study, the students used the LAD not only to monitor but also to image and visualize their learning. In accordance with the views of Papamitsiou and Economides ( 2015 ), the visualizations transformed the analytics data into an easily interpretable visual form. The visualizations were not considered to generate information overload, although such a concern has sometimes been associated with the use of LADs (e.g., Susnjak et al., 2022 ). However, the students widely preferred even more descriptive and nuanced illustrations to clarify and structure the analytics data. At the same time, care must be taken to ensure that the visualizations do not divert too much attention from other relevant aspects of learning, as was also found important in prior research (e.g., Charleer et al., 2018 ; Wise, 2014 ). It seems critical that an LAD inform but not overwhelm its users (Susnjak et al., 2022 ). As argued by Klein et al. ( 2019 ), confusing visualizations may not only generate mistrust but also lead to their complete nonuse.
Although the LAD piloted in the study was considered to be a relatively functional application, it could be even more accessible and usable if it was incorporated into the student information system and enriched with the data from it. Even then, however, the LAD should remain simple to use and its data privacy ensured. It has been argued that more information is not always better (Aguilar, 2018 ), and the analytics indicators must be carefully considered to truly optimize learning (Clow, 2013 ). While developing their SRL, students would particularly benefit from a well-structured environment with fewer distractions and more facilitators for learning (Panadero & Alonso-Tapia, 2014 ). The smooth promotion of studies also seems to require personal access to the analytics data. Similar to the learners in Charleer and colleagues’ ( 2018 ) study, the students in this study desired to take advantage of the LAD autonomously, beyond the guidance context. It was believed to be especially used when they were actively promoting their studies. This is seen as a somewhat expected finding given the significant role of study performance indicators in the LAD. However, the question is also raised as to whether such an analytics application would be used mainly by those students who progress diligently but would be ignored by those who advance only a little or not at all. Ideally, the LAD would serve students in different situations and at various stages of studies.
Using the LAD offered the students a promising means to enhance motivation and interest in their studies through the monitoring of analytics data. However, not all students were inspired in the same manner or similar analytics data displayed by the LAD. Although the LAD was seen as inspiring and interesting in many ways, it also had the potential to demotivate or even discourage. This finding corroborates the results of other studies reporting mixed results on the power of LADs to motivate students (e.g., Bennett, 2018 ; Corrin & de Barba, 2014 ; Schumacher & Ifenthaler, 2018 ). As such, it would be essential that the analytics applications consider and address students with different performance levels and motivational factors (Jivet et al., 2017 ). Based on the results of this study, diversifying the tools included in the LAD might also be necessary. On the other hand, the enhancement of motivation was also found to be the responsibility of the students themselves—that is, if the students wish the analytics application to display favorable analytics data and thus motivate them, they must first display concomitant effort in their studies.
The use of the LAD provided a convenient way to intervene if the students’ study performance did not meet expectations. With the LAD, both the students and their tutor teachers could detect signs of possible support needs and address them with guidance. In the future, such needs could also be reported through automated alerts. Overall, however, the students in this study preferred human contact and personal support over automated interventions, contrary to the findings obtained by Roberts and colleagues ( 2017 ). Being identified to their educators did not seem to be a particular concern for them, although it has been found to worry students in other contexts (e.g., Roberts et al., 2017 ). Rather, the students felt they would benefit more from personal support that was specifically targeted to them and sensitive in its approach. The students generally demanded delicate, ethical consideration when acting upon analytics data and in the provision of support, which was also found to be important in prior research (e.g., Kleimola & Leppisaari, 2022 ). Additionally, Wise and colleagues ( 2016 ) underlined the need to foster student agency and to prevent students from becoming overly reliant on analytics-based interventions: if all of the students’ mistakes are pointed out to them, they may no longer learn to recognize mistakes on their own. Therefore, to support SRL, it is essential to know when to intervene and when to let students solve challenges independently (Kramarski & Michalsky, 2009 ).
Lastly, the students’ perceptions on the use and development of the LAD were examined from the perspective of the reflection phase processes of SRL, with particular attention given to evaluation and reflection on studies and learning. The use of the LAD provided the students with a basis to individually reflect on the potential causes behind their study performance, for better or worse. Moreover, they could address such issues together with guidance personnel and thus make better sense of the analytics data. Corresponding to the results of Charleer et al.’s ( 2018 ) study, collective reflection on analytical data provided the students with new insights and supported their understanding. Engaging in such reflective practices offered the students the opportunity to complete the SRL cycle and draw the necessary conclusions regarding their performance for subsequent actions (see Zimmerman & Moylan, 2009 ). In the future, analytics-based reflection could also be implemented in joint tutoring classes and courses included in the degree programs. This would likely promote the integration of LADs into the activity flow of educational environments, as recommended by Wise and colleagues ( 2016 ). In sum, using LADs should be a regular part of pedagogical practices and learning processes (Wise et al., 2016 ).
When evaluating and reflecting on their studies and learning, the students preferred to focus on themselves and their own development as learners. Similar to earlier findings (e.g., Divjak et al., 2023 ; Rets et al., 2021 ; Roberts et al., 2017 ; Schumacher & Ifenthaler, 2018 ), the students felt differently about the need to develop LADs to compare their study performance with that of other students. Although this function could help some of the students to position themselves in relation to their peers, others thought it should be optional or completely avoided. In agreement with the findings of Divjak et al. ( 2023 ), it seemed that the students wanted to avoid mutual competition comparisons; however, it might not be harmful for everyone and in every case. Consequently, care is required when considering the kind of features in the LAD that offer real value to students in a particular context (Divjak et al., 2023 ). Rather than limiting the point of reference only to peers, it might be useful to also offer students other targets for comparative activity, such as individual students’ previous progress or goals set for the activity (Wise, 2014 ; Wise et al., 2016 ; see also Bandura, 1986 ). In addition, it is important that students not be left alone to face and cope with the various reactions that may be elicited by such evaluation and reflection with analytics data (Kleimola & Leppisaari, 2022 ). As the results of this study and those of others (e.g., Bennett, 2018 ; Lim et al., 2021 ) generally indicate, affective responses evoked by LADs may vary and are not always exclusively positive. Providing a safe environment for students to reflect on successes and failures and to process the resulting responses might not only encourage necessary changes in future studies but also promote the use of an LAD as a learning support.
In summary, the results of this study imply that making an effective use of an analytics application—even with a limited amount of analytics data and functionality available—may facilitate the growth of students into self-regulated learners. That is, even if the LAD principally addresses some particular phase or process of SRL, it can act as a catalyst to encourage students in the development of SRL on a wider scale. This finding also emphasizes the interdependent and interactive nature of SRL (see Zimmerman, 2011 ; Zimmerman & Moylan, 2009 ) that similarly seems to characterize the use of an LAD. However, the potential of LADs to promote SRL may be lost unless students themselves are (pro)active in initiating and engaging with such activity or receive appropriate pedagogical support for it. There appears to be a specific need for guidance that is sensitive to the students’ affective reactions and would help students learn and develop with analytics data. Providing the students with adequate support is particularly critical if their studies have not progressed favorably or as planned. It seems important that the LAD would not only target those students who are already self-regulated learners but, with appropriate support and guidance, would also serve those students who are gradually growing in that direction.
5.1 Limitations and further research
This study has some limitations. First, it involved a relatively small number of HE students who were examined in a pilot setting. Although the sample was sufficient to provide in-depth insights and the saturation point was reached, it might be useful in further research to use quantitative approaches and diverse groups of students to improve the generalizability of results to a larger student population. Also, addressing the perspectives of guidance personnel, specifically tutor teachers, could provide additional insights into the use and development of LADs to promote SRL.
Second, the LAD piloted and investigated in this study was not yet widely in use or accessible by the students. Moreover, it was examined for a relatively brief time, so the students’ perceptions were shaped not only by their experiences but also by their expectations of its potential. Future research on students and tutor teachers with more extensive user experience could build an even more profound picture of the possibilities and limitations of the LAD from a study path perspective. Such investigation might also benefit from trace data collected from the students’ and tutor teachers’ interactions with the LAD. It would be valuable to examine how the students and tutor teachers make use of the LAD in the long term and how it is integrated into learning activities and pedagogical practices.
Third, due to the emphasis on an HE institution and the analytics application used in this specific context, the transferability of results may be limited. However, the results of this study offer many important and applicable perspectives to consider in various educational environments where LADs are implemented and aimed at supporting students across their studies.
6 Conclusions
The results of this study offer useful insights for the creation of LADs that are closely related to the theoretical aspects of learning and that meet the particular needs of their users. In particular, the study increases the understanding of how such analytics applications should be connected to the entirety of studies—that is, what kind of learning processes and pedagogical support are needed alongside them to best serve students in their learning. Consequently, it encourages a comprehensive consideration and promotion of pedagogy, educational technology, and related practices in HE. The role of LA in supporting learning and guidance seems significant, so investments must be made in its appropriate use and development. In particular, the voice of the students must be listened to, as it promotes their commitment to the joint development process and fosters the productive use of analytics applications in learning. At its best, LA becomes an integral part of HE settings, one that helps students to complete their studies and contributes to their development into self-regulated learners.
Data availability
Not applicable.
Abbreviations
Higher education
- Learning analytics
- Learning analytics dashboard
Research question
- Self-regulated learning
Aguilar, S. J. (2018). Examining the relationship between comparative and self-focused academic data visualizations in at-risk college students’ academic motivation. Journal of Research on Technology in Education , 50 (1), 84–103. https://doi.org/10.1080/15391523.2017.1401498
Article Google Scholar
Anthonysamy, L., Koo, A-C., & Hew, S-H. (2020). Self-regulated learning strategies and non-academic outcomes in higher education blended learning environments: A one decade review. Education and Information Technologies , 25 (5), 3677–3704. https://doi.org/10.1007/s10639-020-10134-2
Azevedo, R., Guthrie, J. T., & Seibert, D. (2004). The role of self-regulated learning in fostering students’ conceptual understanding of complex systems with hypermedia. Journal of Educational Computing Research , 30 (1–2), 87–111. https://doi.org/10.2190/DVWX-GM1T-6THQ-5WC7
Bandura, A. (1986). Social foundations of thought and action: A social cognitive theory . Prentice-Hall.
Barnard-Brak, L., Paton, V. O., & Lan, W. Y. (2010). Profiles in self-regulated learning in the online learning environment. The International Review of Research in Open and Distributed Learning , 11 (1), 61–80. https://doi.org/10.19173/irrodl.v11i1.769
Beheshitha, S. S., Hatala, M., Gašević, D., & Joksimović, S. (2016). The role of achievement goal orientations when studying effect of learning analytics visualizations. Proceedings of the 6th International Conference on Learning Analytics and Knowledge (pp. 54–63). Association for Computing Machinery. https://doi.org/10.1145/2883851.2883904
Bennett, E. (2018). Students’ learning responses to receiving dashboard data: Research report . Huddersfield Centre for Research in Education and Society, University of Huddersfield.
Bodily, R., & Verbert, K. (2017). Review of research on student-facing learning analytics dashboards and educational recommender systems. IEEE Transactions on Learning Technologies , 10 (4), 405–418. https://doi.org/10.1109/TLT.2017.2740172
Broadbent, J., & Poon, W. L. (2015). Self-regulated learning strategies & academic achievement in online higher education learning environments: A systematic review. The Internet and Higher Education , 27 , 1–13. https://doi.org/10.1016/j.iheduc.2015.04.007
Callan, G., Longhurst, D., Shim, S., & Ariotti, A. (2022). Identifying and predicting teachers’ use of practices that support SRL. Psychology in the Schools , 59 (11), 2327–2344. https://doi.org/10.1002/pits.22712
Charleer, S., Moere, A. V., Klerkx, J., Verbert, K., & De Laet, T. (2018). Learning analytics dashboards to support adviser-student dialogue. IEEE Transactions on Learning Technologies , 11 (3), 389–399. https://doi.org/10.1109/TLT.2017.2720670
Chenail, R. J. (2011). Interviewing the investigator: Strategies for addressing instrumentation and researcher bias concerns in qualitative research. The Qualitative Report , 16 (1), 255–262. https://doi.org/10.46743/2160-3715/2011.1051
Clow, D. (2013). An overview of learning analytics. Teaching in Higher Education , 18 (6), 683–695. https://doi.org/10.1080/13562517.2013.827653
Conole, G., Gašević, D., Long, P., & Siemens, G. (2011). Message from the LAK 2011 general & program chairs. Proceedings of the 1st International Conference on Learning Analytics and Knowledge . Association for Computing Machinery. https://doi.org/10.1145/2090116
Corrin, L., & De Barba, P. (2014). Exploring students’ interpretation of feedback delivered through learning analytics dashboards. In B. Hegarty, J. McDonald, & S-K. Loke (Eds.), ASCILITE 2014 conference proceedings—Rhetoric and reality: Critical perspectives on educational technology (pp. 629–633). Australasian Society for Computers in Learning in Tertiary Education (ASCILITE). https://www.ascilite.org/conferences/dunedin2014/files/concisepapers/223-Corrin.pdf
Costas-Jauregui, V., Oyelere, S. S., Caussin-Torrez, B., Barros-Gavilanes, G., Agbo, F. J., Toivonen, T., Motz, R., & Tenesaca, J. B. (2021). Descriptive analytics dashboard for an inclusive learning environment. 2021 IEEE Frontiers in Education Conference (FIE) (pp. 1–9). IEEE. https://doi.org/10.1109/FIE49875.2021.9637388
De Barba, P., Oliveira, E. A., & Hu, X. (2022). Same graph, different data: A usability study of a student-facing dashboard based on self-regulated learning theory. In S. Wilson, N. Arthars, D. Wardak, P. Yeoman, E. Kalman, & D. Y. T. Liu (Eds.), ASCILITE 2022 conference proceedings: Reconnecting relationships through technology (Article e22168). Australasian Society for Computers in Learning in Tertiary Education (ASCILITE). https://doi.org/10.14742/apubs.2022.168
De Laet, T., Millecamp, M., Ortiz-Rojas, M., Jimenez, A., Maya, R., & Verbert, K. (2020). Adoption and impact of a learning analytics dashboard supporting the advisor: Student dialogue in a higher education institute in Latin America. British Journal of Educational Technology , 51 (4), 1002–1018. https://doi.org/10.1111/bjet.12962
Divjak, B., Svetec, B., & Horvat, D. (2023). Learning analytics dashboards: What do students actually ask for? Proceedings of the 13th International Learning Analytics and Knowledge Conference (pp. 44–56). Association for Computing Machinery. https://doi.org/10.1145/3576050.3576141
Dollinger, M., & Lodge, J. M. (2018). Co-creation strategies for learning analytics. Proceedings of the 8th International Conference on Learning Analytics and Knowledge (pp. 97–101). Association for Computing Machinery. https://doi.org/10.1145/3170358.3170372
Eickholt, J., Weible, J. L., & Teasley, S. D. (2022). Student-facing learning analytics dashboard: Profiles of student use. IEEE Frontiers in Education Conference (FIE) (1–9). IEEE. https://doi.org/10.1109/FIE56618.2022.9962531
Elo, S., & Kyngäs, H. (2008). The qualitative content analysis process. Journal of Advanced Nursing , 62 (1), 107–115. https://doi.org/10.1111/j.1365-2648.2007.04569.x
Elouazizi, N. (2014). Critical factors in data governance for learning analytics. Journal of Learning Analytics , 1 (3), 211–222. https://doi.org/10.18608/jla.2014.13.25
Heikkinen, S., Saqr, M., Malmberg, J., & Tedre, M. (2022). Supporting self-regulated learning with learning analytics interventions: A systematic literature review. Education and Information Technologies , 28 (3), 3059–3088. https://doi.org/10.1007/s10639-022-11281-4
Jivet, I., Scheffel, M., Drachsler, H., & Specht, M. (2017). Awareness is not enough: Pitfalls of learning analytics dashboards in the educational practice. In É. Lavoué, H. Drachsler, K. Verbert, J. Broisin, & M. Pérez-Sanagustín (Eds.), Lecture notes in computer science: Vol. 10474. Data driven approaches in digital education (pp. 82–96). Springer. https://doi.org/10.1007/978-3-319-66610-5_7
Jivet, I., Scheffel, M., Specht, M., & Drachsler, H. (2018). License to evaluate: Preparing learning analytics dashboards for educational practice. Proceedings of the 8th International Conference on Learning Analytics and Knowledge (pp. 31–40). Association for Computing Machinery. https://doi.org/10.1145/3170358.3170421
Jivet, I., Scheffel, M., Schmitz, M., Robbers, S., Specht, M., & Drachsler, H. (2020). From students with love: An empirical study on learner goals, self-regulated learning and sense-making of learning analytics in higher education. The Internet and Higher Education , 47 , 100758. https://doi.org/10.1016/j.iheduc.2020.100758
Jivet, I., Wong, J., Scheffel, M., Valle Torre, M., Specht, M., & Drachsler, H. (2021). Quantum of choice: How learners’ feedback monitoring decisions, goals and self-regulated learning skills are related. Proceedings of 11th International Conference on Learning Analytics and Knowledge (pp. 416–427). Association for Computing Machinery. https://doi.org/10.1145/3448139.3448179
Kim, J., Jo, I-H., & Park, Y. (2016). Effects of learning analytics dashboard: Analyzing the relations among dashboard utilization, satisfaction, and learning achievement. Asia Pacific Education Review , 17 (1), 13–24. https://doi.org/10.1007/s12564-015-9403-8
Kleimola, R., & Leppisaari, I. (2022). Learning analytics to develop future competences in higher education: A case study. International Journal of Educational Technology in Higher Education , 19 (1), 17. https://doi.org/10.1186/s41239-022-00318-w
Kleimola, R., López-Pernas, S., Väisänen, S., Saqr, M., Sointu, E., & Hirsto, L. (2023). Learning analytics to explore the motivational profiles of non-traditional practical nurse students: A mixed-methods approach. Empirical Research in Vocational Education and Training , 15 (1), 11. https://doi.org/10.1186/s40461-023-00150-0
Klein, C., Lester, J., Rangwala, H., & Johri, A. (2019). Technological barriers and incentives to learning analytics adoption in higher education: Insights from users. Journal of Computing in Higher Education , 31 (3), 604–625. https://doi.org/10.1007/s12528-019-09210-5
Kramarski, B. (2018). Teachers as agents in promoting students’ SRL and performance: Applications for teachers’ dual-role training program. In D. H. Schunk, & J. A. Greene (Eds.), Handbook of self-regulation of learning and performance (2nd ed., pp. 223–239). Routledge/Taylor & Francis Group. https://doi.org/10.4324/9781315697048-15
Kramarski, B., & Michalsky, T. (2009). Investigating preservice teachers’ professional growth in self-regulated learning environments. Journal of Educational Psychology , 101 (1), 161–175. https://doi.org/10.1037/a0013101
Krippendorff, K. (2019). Content analysis (4th ed.). SAGE Publications. https://doi.org/10.4135/9781071878781
Kwasnicka, D., Dombrowski, S. U., White, M., & Sniehotta, F. F. (2015). Data-prompted interviews: Using individual ecological data to stimulate narratives and explore meanings. Health Psychology , 34 (12), 1191–1194. https://doi.org/10.1037/hea0000234
Lim, L-A., Dawson, S., Gašević, D., Joksimović, S., Pardo, A., Fudge, A., & Gentili, S. (2021). Students’ perceptions of, and emotional responses to, personalised learning analytics-based feedback: An exploratory study of four courses. Assessment & Evaluation in Higher Education , 46 (3), 339–359. https://doi.org/10.1080/02602938.2020.1782831
Lodge, J. M., Panadero, E., Broadbent, J., & De Barba, P. G. (2019). Supporting self-regulated learning with learning analytics. In J. M. Lodge, J. Horvath, & L. Corrin (Eds.), Learning analytics in the classroom: Translating learning analytics research for teachers (pp. 45–55). Routledge. https://doi.org/10.4324/9781351113038-4
Marzouk, Z., Rakovic, M., Liaqat, A., Vytasek, J., Samadi, D., Stewart-Alonso, J., Ram, I., Woloshen, S., Winne, P. H., & Nesbit, J. C. (2016). What if learning analytics were based on learning science? Australasian Journal of Educational Technology , 32 (6), 1–18. https://doi.org/10.14742/ajet.3058
Matcha, W., Uzir, N. A., Gašević, D., & Pardo, A. (2020). A systematic review of empirical studies on learning analytics dashboards: A self-regulated learning perspective. IEEE Transactions on Learning Technologies , 13 (2), 226–245. https://doi.org/10.1109/TLT.2019.2916802
Mayring, P. (2000). Qualitative content analysis. Forum Qualitative Sozialforschung Forum: Qualitative Social Research , 1 (2). https://doi.org/10.17169/fqs-1.2.1089
Merriam, S. B., & Tisdell, E. J. (2016). Qualitative research: A guide to design and implementation (4th ed.). JosseyBass.
Molenaar, I., Horvers, A., & Baker, R. S. (2019). Towards hybrid human-system regulation: Understanding children’ SRL support needs in blended classrooms. Proceedings of the 9th International Conference on Learning Analytics and Knowledge (pp. 471–480). Association for Computing Machinery. https://doi.org/10.1145/3303772.3303780
Moos, D. C. (2018). Emerging classroom technology: Using self-regulation principles as a guide for effective implementation. In D. H. Schunk, & J. A. Greene (Eds.), Handbook of self-regulation of learning and performance (2nd ed., pp. 243–253). Routledge. https://doi.org/10.4324/9781315697048-16
Panadero, E. (2017). A review of self-regulated learning: Six models and four directions for research. Frontiers in Psychology , 8 ., Article 422. https://doi.org/10.3389/fpsyg.2017.00422
Panadero, E., & Alonso-Tapia, J. (2014). How do students self-regulate? Review of Zimmerman’s cyclical model of self-regulated learning. Anales De Psicología , 30 (2), 450–462. https://doi.org/10.6018/analesps.30.2.167221
Papamitsiou, Z., & Economides, A. A. (2015). Temporal learning analytics visualizations for increasing awareness during assessment. RUSC Universities and Knowledge Society Journal , 12 (3), 129–147. https://doi.org/10.7238/rusc.v12i3.2519
Park, Y., & Jo, I. (2015). Development of the learning analytics dashboard to support students’ learning performance. Journal of Universal Computer Science , 21 (1), 110–133. https://doi.org/10.3217/jucs-021-01-0110
Pintrich, P. R. (2000). The role of goal orientation in self-regulated learning. In M. Boekaerts, P. R. Pintrich, & M. Zeidner (Eds.), Handbook of self-regulation (pp. 451–502). Academic Press. https://doi.org/10.1016/B978-012109890-2/50043-3
Puustinen, M., & Pulkkinen, L. (2001). Models of self-regulated learning: A review. Scandinavian Journal of Educational Research , 45 (3), 269–286. https://doi.org/10.1080/00313830120074206
Rets, I., Herodotou, C., Bayer, V., Hlosta, M., & Rienties, B. (2021). Exploring critical factors of the perceived usefulness of a learning analytics dashboard for distance university students. International Journal of Educational Technology in Higher Education , 18 (1). https://doi.org/10.1186/s41239-021-00284-9
Roberts, L. D., Howell, J. A., & Seaman, K. (2017). Give me a customizable dashboard: Personalized learning analytics dashboards in higher education. Technology Knowledge and Learning , 22 (3), 317–333. https://doi.org/10.1007/s10758-017-9316-1
Schreier, M. (2014). Qualitative content analysis. In U. Flick (Ed.), The SAGE handbook of qualitative data analysis (pp. 170–183). SAGE. https://doi.org/10.4135/9781446282243
Schumacher, C., & Ifenthaler, D. (2018). Features students really expect from learning analytics. Computers in Human Behavior , 78 , 397–407. https://doi.org/10.1016/j.chb.2017.06.030
Schwendimann, B. A., Rodríguez-Triana, M. J., Vozniuk, A., Prieto, L. P., Boroujeni, M. S., Holzer, A., Gillet, D., & Dillenbourg, P. (2017). Perceiving learning at a glance: A systematic literature review of learning dashboard research. IEEE Transactions on Learning Technologies , 10 (1), 30–41. https://doi.org/10.1109/TLT.2016.2599522
Sclater, N., Peasgood, A., & Mullan, J. (2016). Learning analytics in higher education—A review of UK and international practice: Full report . Jisc. https://www.jisc.ac.uk/sites/default/files/learning-analytics-in-he-v2_0.pdf
Silvola, A., Sjöblom, A., Näykki, P., Gedrimiene, E., & Muukkonen, H. (2023). Learning analytics for academic paths: Student evaluations of two dashboards for study planning and monitoring. Frontline Learning Research , 11 (2), 78–98. https://doi.org/10.14786/flr.v11i2.1277
Susnjak, T., Ramaswami, G. S., & Mathrani, A. (2022). Learning analytics dashboard: A tool for providing actionable insights to learners. International Journal of Educational Technology in Higher Education , 19 (1). https://doi.org/10.1186/s41239-021-00313-7
Teasley, S. D. (2017). Student facing dashboards: One size fits all? Technology Knowledge and Learning , 22 (3), 377–384. https://doi.org/10.1007/s10758-017-9314-3
Van Leeuwen, A., Teasley, S., & Wise, A. (2022). Teacher and student facing analytics. In C. Lang, G. Siemens, A. Wise, D. Gašević, & A. Merceron (Eds.), Handbook of learning analytics (2nd ed., pp. 130–140). Society for Learning Analytics Research. https://doi.org/10.18608/hla22.013
Verbert, K., Ochoa, X., De Croon, R., Dourado, R. A., & De Laet, T. (2020). Learning analytics dashboards: The past, the present and the future. Proceedings of the 10th International Conference on Learning Analytics and Knowledge (pp. 35–40). Association for Computing Machinery. https://doi.org/10.1145/3375462.3375504
Viberg, O., Khalil, M., & Baars, M. (2020). Self-regulated learning and learning analytics in online learning environments: A review of empirical research. Proceedings of the 10th International Conference on Learning Analytics and Knowledge (pp. 524–533). Association for Computing Machinery. https://doi.org/10.1145/3375462.3375483
Virtanen, P. (2019). Self-regulated learning in higher education: Basic dimensions, individual differences, and relationship with academic achievement (Helsinki Studies in Education, 1798–8322) [Doctoral dissertation, University of Helsinki]. University of Helsinki Open Repository. https://urn.fi/URN:ISBN:978-951-51-5681-5
West, D., Luzeckyj, A., Toohey, D., Vanderlelie, J., & Searle, B. (2020). Do academics and university administrators really know better? The ethics of positioning student perspectives in learning analytics. Australasian Journal of Educational Technology , 36 (2), 60–70. https://doi.org/10.14742/ajet.4653
Winne, P. H., & Hadwin, A. F. (1998). Studying as self-regulated learning. In D. Hacker, J. Dunlosky, & A. Graesser (Eds.), Metacognition in educational theory and practice (pp. 277–304). Erlbaum.
Wise, A. F. (2014). Designing pedagogical interventions to support student use of learning analytics. Proceedings of the 4th International Conference on Learning Analytics and Knowledge (pp. 203–211). Association for Computing Machinery. https://doi.org/10.1145/2567574.2567588
Wise, A. F., Vytasek, J. M., Hausknecht, S., & Zhao, Y. (2016). Developing learning analytics design knowledge in the middle space: The student tuning model and align design framework for learning analytics use. Online Learning , 20 (2), 155–182. https://doi.org/10.24059/olj.v20i2.783
Wong, J., Baars, M., Davis, D., Van Der Zee, T., Houben, G-J., & Paas, F. (2019). Supporting self-regulated learning in online learning environments and MOOCs: A systematic review. International Journal of Human–Computer Interaction , 35 (4–5), 356–373. https://doi.org/10.1080/10447318.2018.1543084
Zimmerman, B. J. (1999). Attaining self-regulation: A social cognitive perspective. In M. Boekaerts, P. R. Pintrich, & M. Zeidner (Eds.), Handbook of self-regulation (pp. 13–39). Academic Press. https://doi.org/10.1016/B978-012109890-2/50031-7
Zimmerman, B. J. (2011). Motivational sources and outcomes of self-regulated learning and performance. In B. J. Zimmerman, & D. H. Schunk (Eds.), Handbook of self-regulation of learning and performance (pp. 49–64). Routledge/Taylor & Francis Group.
Zimmerman, B. J. (2015). Self-regulated learning: Theories, measures, and outcomes. In J. D. Wright (Ed.), International Encyclopedia of the Social & Behavioral Sciences (2nd ed., pp. 541–546), Elsevier. https://doi.org/10.1016/B978-0-08-097086-8.26060-1
Zimmerman, B. J., & Moylan, A. R. (2009). Self-regulation: Where metacognition and motivation intersect. In D. J. Hacker, J. Dunlosky, & A. C. Graesser (Eds.), Handbook of metacognition in education (pp. 299–315). Routledge/Taylor & Francis Group.
Download references
Acknowledgements
Language process: In the preparation process of the manuscript, the Quillbot Paraphraser tool was used to improve language clarity in some parts of the text (e.g., word choice). The manuscript was also proofread by a professional. After using this tool and service, the authors reviewed and revised the text as necessary, taking full responsibility for the content of this manuscript.
The authors also thank the communications and information technology specialists of the UAS under study for their support in editing Fig. 1 for publication.
This research was partly funded by Business Finland through the European Regional Development Fund (ERDF) project “Utilization of learning analytics in the various educational levels for supporting self-regulated learning (OAHOT)” (Grant no. 5145/31/2019). The article was completed with grants from the Finnish Cultural Foundation’s Central Ostrobothnia Regional Fund (Grant no. 25221232) and The Emil Aaltonen Foundation (Grant no. 230078), which were awarded to the first author.
Open Access funding provided by University of Lapland.
Author information
Authors and affiliations.
Faculty of Education, University of Lapland, Rovaniemi, Finland
Riina Kleimola & Heli Ruokamo
School of Applied Educational Science and Teacher Education, University of Eastern Finland, Joensuu, Finland
Laura Hirsto
You can also search for this author in PubMed Google Scholar
Contributions
Conceptualization: RK, LH. Data collection: RK. Formal analysis: RK, LH. Writing—original draft: RK. Writing—review and editing: RK, LH, HR. Supervision: LH, HR. All authors read and approved the final manuscript.
Corresponding author
Correspondence to Riina Kleimola .
Ethics declarations
Competing interests.
The authors declare that they have no competing interests.
Additional information
Publisher’s note.
Springer Nature remains neutral with regard to jurisdictional claims in published maps and institutional affiliations.
Rights and permissions
Open Access This article is licensed under a Creative Commons Attribution 4.0 International License, which permits use, sharing, adaptation, distribution and reproduction in any medium or format, as long as you give appropriate credit to the original author(s) and the source, provide a link to the Creative Commons licence, and indicate if changes were made. The images or other third party material in this article are included in the article’s Creative Commons licence, unless indicated otherwise in a credit line to the material. If material is not included in the article’s Creative Commons licence and your intended use is not permitted by statutory regulation or exceeds the permitted use, you will need to obtain permission directly from the copyright holder. To view a copy of this licence, visit http://creativecommons.org/licenses/by/4.0/ .
Reprints and permissions
About this article
Kleimola, R., Hirsto, L. & Ruokamo, H. Promoting higher education students’ self-regulated learning through learning analytics: A qualitative study. Educ Inf Technol (2024). https://doi.org/10.1007/s10639-024-12978-4
Download citation
Received : 14 February 2024
Accepted : 09 August 2024
Published : 07 September 2024
DOI : https://doi.org/10.1007/s10639-024-12978-4
Share this article
Anyone you share the following link with will be able to read this content:
Sorry, a shareable link is not currently available for this article.
Provided by the Springer Nature SharedIt content-sharing initiative
- Higher education student
- Qualitative study
- Find a journal
- Publish with us
- Track your research
- Open access
- Published: 07 September 2024
Iranian experts’ perspectives on facilitators and barriers influencing the infectious disease knowledge network: A qualitative study
- Mina Mahami-Oskouei ORCID: orcid.org/0000-0001-8561-8042 1 ,
- Leila Nemati-Anaraki ORCID: orcid.org/0000-0002-9436-2533 2 ,
- Sirous Panahi ORCID: orcid.org/0000-0001-7610-906X 3 &
- Shadi Asadzandi ORCID: orcid.org/0000-0002-1350-9629 3
BMC Health Services Research volume 24 , Article number: 1040 ( 2024 ) Cite this article
Metrics details
Knowledge networks, such as Communities of Practice (CoP), are essential elements of knowledge management. They play a crucial role in assimilating various knowledge domains and converting individual knowledge into collective knowledge. This study aimed to assess the concept of knowledge networks and identify facilitators and barriers influencing knowledge sharing in infectious diseases, according to Iranian experts.
This qualitative study employed content analysis and used purposive and snowball sampling. The data were collected via online or face-to-face interviews with 25 participants with diverse expertise in infectious diseases (both clinical and non-clinical), epidemiology, knowledge management, and knowledge-based business management in Iran. The thematic analysis technique was used to code the interviews, and the collected data were analyzed using MAXQDA 20 software.
Thematic analysis of the interviews led to 437 codes. These codes were categorized into two groups: facilitators and barriers. The facilitators shaping the knowledge network for infectious diseases were classified into three main categories: individual factors, organizational factors, and communication mechanisms. Individual factors involved two themes: strengthening knowledge exchange between experts in infectious diseases and personal characteristics such as the criteria for network membership. Organizational factors comprised three themes: organizational and trans-organizational factors, management strategies, and interactions with non-governmental sectors. Communication mechanisms included two themes: the use of information technology and knowledge brokers. In addition, three important challenges were identified as barriers influencing the knowledge network: administration and policy-making, organizational and trans-organizational, and personal challenges.
Conclusions
Several facilitators and barriers influence the formation of an infectious disease knowledge network, which must be addressed to ensure its effectiveness, development, and long-term sustainability. Addressing these factors will enable the network to effectively integrate diverse knowledge and contribute to advancing infectious disease management.
Peer Review reports
Introduction
Knowledge networks are networks that appear in various forms, such as Communities of Practice (CoP). These networks bring together scientists from different disciplines, fostering tacit knowledge sharing and exchange between specialists in scientific fields. These networks can be created at the organizational and inter-organizational levels and even beyond organizational boundaries. They also offer opportunities to break down professional barriers, address complex systemic challenges, and support professionals. These networks are of utmost importance in the synthesis of diverse knowledge streams from various contributors in knowledge management [ 1 , 2 , 3 , 4 ]. These structures facilitate the fluid exchange of knowledge and expertise among individuals and groups, surpassing organizational boundaries to convert individual insights into collective comprehension. These interactive environments cultivate the generation of innovative information, enhancement of pre-existing knowledge, and efficient resolution of intricate challenges [ 5 , 6 ].
Reliance on knowledge networks is significant in the constantly evolving field of healthcare [ 7 ]. Health organizations worldwide prioritize enhancing the caliber, efficacy, and productivity of their services [ 8 ]. A crucial element of this commitment involves embracing research-based and evidence-driven decision-making processes [ 9 ]. However, efficient and timely dissemination of diverse knowledge into health practice and policy-making requires a new framework for organizing and disseminating the evidence. Central to this framework is the promotion of active participation among physicians and healthcare practitioners in creating and exchanging knowledge, as well as attaining consensus on its practical application [ 10 , 11 ]. For instance, Chan et al. showed that improving the quality of drug prescriptions in the geriatrics department requires effective communication and collaboration between various specialties in multidisciplinary knowledge networks [ 12 ]. Moreover, during the COVID-19 crisis in Canada, inter-organizational collaboration among experts through knowledge exchanges proved to be an effective strategy for collectively shouldering public health responsibilities [ 13 ].
However, infectious diseases still pose a threat to people’s health. This risk is exacerbated by the emergence of new pathogens, re-emergence of infections, and environmental and lifestyle-related challenges to disease control [ 14 , 15 ]. The COVID-19 pandemic has been unprecedented in recent years, with the World Health Organization (WHO) reporting more than 153 million cases and 2.3 million deaths by mid-2021 [ 16 ].
Effectively combating infectious diseases requires strategic collaboration and a broad knowledge base. The scientific community, government bodies, non-governmental organizations, and health policymakers must engage in extensive cooperation. However, the lack of coordination and cooperation among all stakeholders and authorities in the control of infectious diseases is one of the most important weaknesses of the care systems in developing countries, such as Iran [ 15 , 17 ] According to a study conducted in southern Nigeria, managing endemic infectious diseases is significantly hindered by the inadequate application of research findings in policy-making and practice. The creation of knowledge networks and strengthening collaboration between research producers and evidence users have been proposed as possible solutions to this problem. This finding highlights the crucial role of knowledge networks in achieving evidence-based healthcare delivery in these diseases [ 18 ].
Although in the field of infectious diseases, there are knowledge networks based on technological tools such as the Global Public Health Information Network (GPHIN), Global Outbreak Alert and Response Network (GOARN), Google Flu Trends, BioCaster, GET WE, and MedISys. These networks use a variety of technologies to collect, analyze, and disseminate data on infectious diseases. They focus on facilitating the exchange of information and expertise among a wide range of researchers, healthcare professionals, policymakers, and the public. However, these networks are transactional, and their members share information and resources based on need [ 17 ]. In contrast, networks such as Communities of Practice (CoPs) emphasize the strengthening of cooperation between specialists with common interests and expertise in a particular field. This leads to more stable and collaborative relationships and creates a space for members to engage in meaningful discussions, share their experiences, and develop collective knowledge and skills [ 1 ]. In other words, while researchers and other experts play a pivotal role in these efforts, a lack of communication between research findings and health policy can hinder disease control efforts despite the existence of prevention and treatment protocols. Therefore, enhanced coordination and collaboration among healthcare practitioners through the creation and implementation of interactive knowledge networks such as Communities of Practice (CoPs) are essential for overcoming these obstacles in infectious diseases [ 19 ].
In this regard, McKellar et al. also emphasized the importance of networked knowledge exchange and capacity building. They discussed the potential costs and benefits of these activities, as well as ways to maximize the benefits [ 20 ]. Communication can be improved within and across national borders by strengthening knowledge exchange and forging stronger partnerships between research institutions and healthcare organizations. In turn, this will enhance initiatives aimed at tackling infectious diseases [ 21 ].
The purpose of this research was to evaluate the factors that enable or hinder the need and significance of establishing interactive knowledge exchange networks and communities of practice (CoPs) among infectious disease specialists. Given the nature and rapid spread of infectious diseases, the sharing of information, knowledge, and experiences among experts in this field is highly valuable. As a result, the findings of this research are particularly important for infectious disease managers and policymakers, as well as for promoting a community of specialists and enhancing healthcare providers’ grasp of collective knowledge and practices.
Participants
This qualitative study employing content analysis was carried out in Iran. Semi-structured interviews were conducted with 25 experts, including infectious disease specialists (clinical and non-clinical), epidemiologists, knowledge management experts, and executives of knowledge-based firms) Like companies that produce new services such as medicine, vaccine, medical equipment, etc.). The participants were selected based on purposive and snowball sampling. They met the following criteria: willingness to engage with the research team, holding a degree in fields such as infectious diseases (e.g., a Ph.D. In Medical Virology, Medical Bacteriology, Medical Microbiology, or Epidemiology) or health knowledge management (e.g., a Ph.D. In the Medical Library of Information Sciences), and being actively involved in their respective field. The interviews were continued until data saturation. We conducted a total of 23 interviews, with one interview conducted per participant. After we reached data saturation, two more interviews were conducted to ensure that no new piece of data pertaining to the research topic would be obtained. The interviews were conducted either in person (face to face) at the specialists’ workplaces or online through social networks such as Skype, WhatsApp, and Google Meet.
Data collection
The study used a semi-structured interview guide with close-ended questions to gather information about the study’s goals and limitations (see Appendix 1). The interview guide consisted of two parts: demographic data and the knowledge network and factors affecting it. The interviews were conducted from October to December 2022 and took an average of 30 to 45 min. The participants provided informed consent before interviews after being ensured of the confidentiality of the information. They were also asked to recommend other individuals whom they believed were pertinent to the investigation. In addition, they offered consent to have their voices recorded and were assured of their ability to withdraw from the study at any time.
Data analysis
The data collected from the interviews was analyzed based on thematic analysis. At the end of each interview, we meticulously transcribed the interview in Microsoft Word 2019. Upon reviewing the transcripts multiple times, the initial themes and sub-themes were identified using MAXQDA 20. The coding into major and minor themes was done independently by MO, M, and NA, L. Discrepancies were resolved through discussion with a third reviewer (A, SH). The member check strategy [ 22 ] was used to ensure the validity and reliability of the data. Initial codes were shared with some participants to compare and verify the consistency of the ideas generated from the data with their content. Inter-coder agreement was checked to control the validity of the data. After the initial analysis, the researchers re-analyzed the interview texts and combined similar codes and subsets. In the next step, the relationships between subsets were identified and categorized into larger themes. Finally, the main themes of this study were extracted.
The study involved interviews with 25 participants, 20 of whom were male. All the participants were subject specialists. They were actively working as professors and researchers at Iranian universities of medical sciences, and two were managers of knowledge-based companies in the field of health systems. Table 1 lists the participants’ demographics. Initially, 871 codes were examined and then refined to 564 unique codes by eliminating repetitions and consolidating similar codes. Subsequently, through expert assessment, these codes were further narrowed to 437 definitive codes. These codes were categorized into two groups: influential facilitators and barriers (Table 2 ).
Based on the data gathered from interviews, the facilitators shaping the knowledge network for infectious diseases were classified into three main categories: individual factors, organizational factors, and communication mechanisms. In total, these categories encompassed seven key themes.
Individual factors involved two themes: “strengthening knowledge exchange between experts in infectious diseases” and “personal characteristics” such as the criteria for network membership.
Organizational factors encompassed factors related to the organization and involve three themes: “organizational and trans-organizational factors”, “management strategies”, and “interactions with non-governmental sectors”. Communication mechanisms involved two themes: the use of “information technology” and “knowledge brokers”. In addition, three important challenges were identified as barriers to the knowledge network: “administration and policy-making challenges”, “organizational and trans-organizational challenges”, and “individual challenges”. Detailed descriptions of these influential facilitators and barriers are provided to offer a comprehensive understanding of their role in shaping the knowledge network for infectious diseases.
Facilitators related to the knowledge network of infectious diseases
Individual factors, theme 1: strengthening knowledge exchange between experts in infectious diseases.
A primary concern impacting all types of knowledge networks the knowledge network of infectious diseases is the need to improve connections between specialists. According to many interviewees, building and maintaining a knowledge network in this field depends on establishing a robust scientific framework strong scientific platform for specialists to communicate and share knowledge while recognizing different viewpoints, areas of expertise, and skills. By promoting collaboration and interdisciplinary communication, knowledge networks can benefit from diverse perspectives and expertise. This approach allows for a more comprehensive understanding of infectious diseases as specialists can share unique insights and contribute to developing innovative solutions. One participant highlighted the significance of taking immediate action based on the exchange of knowledge among specialists:
“A scientific platform that caters to the elite is highly beneficial. It allows for the scientific communication and acceptance of diverse tastes and specialties , such as microbiology , laboratory sciences , and academic professors of traditional medicine from prestigious universities. This applies to all areas and activities within the platform , ensuring a specific and valid focus.” (P5) .
Theme 2: Personal characteristics (Criteria for membership)
The second theme of individual factors among facilitators of the knowledge network of infectious diseases is personal characteristics, e.g., personality, beliefs, behaviors, and attitudes shaping interactions and knowledge sharing within infectious diseases knowledge networks. By recognizing and utilizing these factors, we can enhance knowledge flow, support collaboration, and effectively combat the complexities of infectious diseases.
“It is important to acknowledge the importance of individual agents in every discipline. Despite our perceived abilities and knowledge , it’s crucial to acknowledge the boundaries of our understanding. The desire for group interaction and involvement can significantly affect motivation. Thus , when choosing members , it is crucial to carefully consider individual factors.” (P5) .
Organizational factors
Theme 1: organizational and trans-organizational factors.
One of the main facilitators of establishing knowledge networks for infectious diseases is the influence of organizational and trans-organizational factors. These factors encompass the processes, structures, and mechanisms directly impacting the formation and enhancement of knowledge networks. These factors are critical to developing successful knowledge networks that can effectively respond to outbreaks, conduct research, and inform public health policy. As stated by the contributors, these factors are essential for shaping successful knowledge networks. Infectious disease stakeholders can collaborate more effectively to address current challenges and prepare for future threats by recognizing and addressing these factors.
“…Organizational factors are the same as hierarchy and definition of processes , which are very much needed in such teamwork…” (P3) . “To ensure the legal approval of these networks , it is necessary to establish clear organizational frameworks. These frameworks should outline permissible exchanges within each area , ensuring the usefulness of the network for all parties involved. It is crucial to identify and clarify the specific points relevant to individuals and their associated institutions.” (P14) .
Theme 2: Management strategies
Effective management strategies can direct the network path, determine the input and output of processes, and regulate them. A key aspect of effective management strategies is clearly defining the tasks and roles of individuals within an organization. This can significantly impact the outcomes of knowledge networks. By assigning specific responsibilities to individuals based on their skills, expertise, and knowledge, managers can ensure that each member effectively contributes to the network’s goals. This can foster a sense of ownership, accountability, and collaboration among team members, thereby improving coordination and communication within the network. Participants P2 and P5 stated the following:
“Effective management policies are crucial for success. Without targeted guidance , leadership , and excellent management , nothing would come to fruition. Conflicts are inevitable when different views and experiences collide.” (P2) . “The management must know how to manage , divide the tasks , hold multiple meetings… interact with them more… If the network management is strong and capable , it can lead the affairs , and , most importantly , the support of top managers from Take these processes “. (P11)
Theme 3: Strengthening interactions with non-governmental sectors
An important factor for the successful formation of knowledge networks is establishing a stronger relationship with non-governmental sectors. Input from those interviewed shows that the non-profit sector can provide several advantages: More efficient and effective research in healthcare can be achieved by working collaboratively with the non-governmental sector, thus facilitating the creation of new products and the provision of high-quality healthcare. This collaboration allows for the transformation of research knowledge into tangible actions and policies that serve to benefit society at large. Moreover, the partnership between knowledge institutions and non-governmental organizations encourages the development of innovative and comprehensive approaches to problem-solving. This partnership empowers societies by driving positive change and promoting inclusiveness. Participant P12 specifically emphasized these advantages:
“The exchange of knowledge between non-governmental companies and academic professionals is currently limited. While there is some exchange on face-to-face platforms , it is mostly through trial and error in the production of products. However , external interactions with companies can greatly impact the effectiveness of research results and the achievement of the knowledge network’s main goals , ultimately promoting network performance.” (P12) .
Communication mechanisms
Theme 1: use of information technology.
The use of information technology significantly influences knowledge networks in infectious diseases. The participants emphasized that information technologies help the knowledge network by facilitating the acquisition, assimilation, sharing, and dissemination of knowledge, particularly in generating new knowledge. Information technology has revolutionized research by facil.itating collaboration and knowledge sharing among researchers globally. Online platforms and databases allow researchers to access and contribute to a vast repository of data on infectious diseases, accelerating the spread of fresh research and treatment advancements. They also stated that technological tools supporting a knowledge network should offer a robust infrastructure for managing a comprehensive knowledge base and creating collaborative workspaces. This viewpoint was shared by P10 and P22.
“Technological infrastructure is a significant factor shaping the growth of networks. It is essential for the formation of networks and is widely used in today’s world.” (P 10) . “Specialists rely on this infrastructure to effectively apply technology and build a comprehensive knowledge base through collaborative efforts.” (P 22) .
Theme 2: Use of knowledge brokers
Knowledge brokers facilitate and enhance the transmission and communication of knowledge among individuals. Their presence in networks can lead to better communication between members and teams, resulting in successful cooperation. Librarians, as experts in connecting people and information, have a vital role in the knowledge ecosystem of infectious diseases. They facilitate communication and understanding among researchers, healthcare practitioners, policymakers, and the wider community. As intermediaries, librarians facilitate communication and knowledge exchange, ensuring that diverse perspectives and expertise are considered in understanding and addressing infectious disease challenges. For instance, Participants P8 and P1 stated the following:
“The profession of librarianship plays a crucial role in using technology to effectively mediate networks. Librarians can use these resources to form networks and play a significant role in their development.” (P8) . “Unfortunately , our librarians were introduced mostly as book repositories… while as a good source of knowledge , they can be facilitators for these scientific networks… Informants can help in informing and publishing.” (P17) .
Barriers to the knowledge network for infectious diseases
Theme 1: administration and policy-making challenges.
According to the participants, a significant barrier to developing a successful infectious knowledge network is the limited understanding of infectious diseases, management difficulties, and policy-making. They emphasized that without a solid understanding of infectious diseases, it becomes challenging to effectively manage and make informed policy decisions. They noted that health organizations struggle to manage and create policies in the field of infectious diseases because managers and policymakers often overlook the shortcomings of these areas. Considering these challenges, the participants suggested that focusing on elements, such as policy and management frameworks, can lead to successful outcomes. For instance, two participants said,
“We have identified a weakness in knowledge transfer within our organization. Therefore , organizations must prioritize both organizational and managerial policies in addressing this issue” (P 15). “The role of management is important here , and it is better to choose managers who have a lot of general expertise , are impartial , and can invite different people from different disciplines to interactions. The result is empathy and effectiveness of interactions to control and find solutions to challenges for patients and the health system… , something that we do not see in our health system at the moment…” (P10) .
Theme 2: Organizational and trans-organizational challenges
Organizational and trans-organizational challenges are the second obstacle to the knowledge network of infectious diseases. Many participants identified structural problems, weak organizational culture, and inadequate infrastructure as major obstacles to forming societies and knowledge exchange networks in infectious diseases. A comprehensive strategy is needed to improve collaboration, communication, and efficiency in knowledge sharing within an infectious disease knowledge network. This includes promoting a culture that values collaboration across different organizations, establishing better ways to communicate and share information, simplifying administrative procedures to facilitate knowledge sharing, and providing enough funding and resources to support the network’s activities. By overcoming these challenges, the network can improve its knowledge-sharing capabilities, resulting in more effective prevention and management of infectious diseases. For example, two participants stated the following:
“We have structural problems and no culture of information exchange and sharing within our organization… Important individuals with knowledge and experience are not given proper attention.” (P 21) . “When we talk about the knowledge network , the infrastructure must be provided first because it strengthens the knowledge in the system. Lack of infrastructure , culture , process , and support from organizational managers for these processes are challenges. If these issues are resolved , it can lead to the flourishing of networks and increased interactions , and we can witness its positive effects on society in the field of infectious disease control and treatment…” (P9) .
Theme 3: Individual challenges
One of the main barriers to developing knowledge networks in infectious diseases is individual challenges. The participants identified several problems related to these challenges that hinder the formation of knowledge networks, such as the heavy workload, a lack of a clear definition of knowledge networks for individuals, and social factors. Overcoming these obstacles will foster a collaborative and innovative atmosphere. This environment will enhance our abilities to prevent, identify, and manage infectious diseases effectively.
“Most people don’t want to participate in activities like knowledge networks due to a lack of time… Time limitations can explain why people don’t participate and prefer to do things that are their main responsibility. …“(P4) . “… In my view , this matter remains incompletely comprehended , and what I have failed to grasp is that while many individuals may possess familiarity with the discourse from a scientific perspective , they may lack any practical insights on the subject matter…. They have no background regarding the issue. Now , how can this network be implemented?” (P8) . “Social factors are also important… People’s expectations can affect the quality and amount of knowledge exchange.” (P19) .
This qualitative study evaluated knowledge networks and identified facilitators and barriers influencing knowledge sharing in infectious diseases. Since infectious diseases are important not only in Iran, but also worldwide, it is crucial to strengthen the scientific foundation of specialists and promote their participation in sharing information and knowledge. The results showed that facilitating collaboration among individuals with diverse expertise promotes knowledge exchange, learning, and innovation. This, in turn, enhances therapeutic approaches and facilitates forming a professional knowledge network and innovation to enhance therapeutic approaches.
The findings demonstrated a pressing problem in healthcare regarding the consistent and effective application of research findings in practice, a dilemma that has persisted for many years [ 23 ]. A deficiency in translating research into clinical practice has detrimental impacts, compromising clinical outcomes and the overall quality of healthcare services. Additionally, it leads to the squandering of resources and perpetuates an inefficient and unproductive health system. Deploying effective strategies is crucial to bridging this gap in knowledge applications. Facilitating the exchange of knowledge by creating wide networks with different goals, such as control, prevention, and the development of new treatment methods that include different organizations, professions, and people, is vital to addressing these challenges in infectious diseases [ 24 , 25 , 26 ].
Personal attributes are another critical factor influencing the development of knowledge networks in infectious diseases. Individual characteristics that vary and influence engagement in a knowledge network have been evaluated in several studies for their impact on knowledge sharing and exchange ( 27 – 28 ).
The findings revealed that organizational and trans-organizational factors are among the most important aspects influencing the development of knowledge networks in infectious diseases. Findings from earlier studies align with this, showcasing that characteristics within organizations significantly influence the distribution and exchange of knowledge between organizations [ 29 , 30 , 31 ]. This study demonstrates that management strategies and practices play a crucial role in fostering the development and enhancement of interconnected knowledge-sharing networks within an organization. The effective implementation of these processes can significantly enhance the flow of knowledge within the network, making it a crucial factor for the successful launch of knowledge networks [ 32 ]. This study highlights the significant role of management practices in driving the effectiveness of knowledge networks. Similar findings have been observed in multiple previous studies [ 33 , 34 , 35 ].
A recent study suggests that the network of information in infectious diseases can aid a reflective and inclusive approach to improving primary healthcare. It can also provide a platform for discussions, support the transmission process, and facilitate knowledge exchange [ 13 ]. The network should include representatives from public and non-public sectors, requiring a mixed resource allocation model involving all stakeholders. Partnering with the non-public sector can provide valuable features, such as skill effectiveness, innovation, technology, motivation, financing, and risk sharing. Public-private partnership is a sustainable financial mechanism for health development initiatives and improves the quality of public services [ 36 ]. Hernandez and Zaragoza’s investigation corroborates the desirability of public and non-public collaboration in mitigating healthcare challenges and advancing service provision [ 37 ].
The analysis revealed that using information technology, specifically tools that enable streamlined communication and information sharing, holds considerable sway over the knowledge exchange network in the field of infectious diseases. These technologies are pivotal for promoting knowledge sharing, forging connections between individuals and various organizational departments, and mediating knowledge transfer across disparate entities. Therefore, access to these technologies is imperative for the efficiency of knowledge networks, providing the necessary infrastructure to expedite knowledge sharing and transfer within a network [ 38 , 39 ]. Although information technologies can present risks or threats, such as diminishing in-person interactions and potentially undermining trust and collaboration, their benefits are much more significant [ 40 ]. Goh’s research emphasizes the substantial role that information systems and informatics play in enabling expertise sharing and dissemination among professionals [ 41 ].
This study suggests that using brokers such as librarians and medical informants can enhance the effectiveness of a knowledge network. These intermediaries act as bridges between scientists, leaders, and policymakers, making communication and comprehension easier. As a result, there is more teamwork, and knowledge is shared more widely. The study recommends that knowledge networks use these mediators more frequently to enhance their overall effectiveness [ 42 ]. Several studies have indicated that knowledge brokers facilitate social interactions and participatory processes by focusing on tasks such as identifying, appraising, and translating evidence. These professionals identify emerging problems that can be resolved using the knowledge contained in evidence and documentation [ 43 , 44 , 45 ].
Regarding the barriers to the formation of the infectious disease knowledge network, the findings from this study suggest that the health system must implement management policies and open scientific frameworks to enhance knowledge sharing and exchange among specialists. Misaligned management strategies or a lack of coherence with organizational goals can be primary factors in the failure of networks and knowledge communities to fulfill their knowledge-sharing and professional expertise goals. Elements such as the provision of organizational facilities, the overarching environment, and the prevailing culture exert significant influence on the efficacy of the knowledge network’s operational capabilities [ 46 , 47 , 48 ]. Furthermore, this study suggests that specific personal traits can make it harder to build knowledge networks and share knowledge with others. This decreased knowledge sharing can harm collaborative efforts. This presents a significant challenge, as has been highlighted in previous studies.
This study encompasses a limited segment of the health research community. Consequently, future studies should meticulously assess and scrutinize diverse dimensions of research in this domain while also considering the perspectives of a wider array of individuals and specialties. Policies governing the use of this network and crucial elements contributing to its advancement should be established based on these evaluations.
The findings underscore the importance of expanding scientific evidence and research in the field of infectious disease. A strong network linking different departments, specialties, and experts from various disciplines is critical for rapid response. Creating such a network requires strategic partnerships with healthcare institutions and non-governmental sector entities while maintaining consistent communication through direct and digital interactions. This study identifies the facilitators and barriers to implementing a knowledge network specifically for infectious diseases. By carefully evaluating the effectiveness and practical applications of each aspect, we can significantly enhance the likelihood of successfully establishing, growing, and maintaining a network. It is essential to recognize and successfully navigate challenges that arise to guarantee a network’s successful establishment and ongoing functionality.
Data availability
The datasets formed and analyzed during the current study are available from the corresponding author upon reasonable request.
Pyrko I, Dörfler V, Eden C. Thinking together: what makes communities of practice work? Hum Relat. 2017;70(4):389–409.
Article PubMed Google Scholar
Mahami-Oskouei M, Nemati-Anaraki L, Panahi S, Asadzandi S. Factors influencing knowledge sharing between scientific specialists in knowledge networks and communities of practice: a systematic literature review. J Educ Health Promotion. 2024;13(1):136.
Article Google Scholar
Hildreth PM, Kimble C, editors. Knowledge networks: Innovation through communities of practice. Igi Global; 2004.
Schönström M. Creating knowledge networks: lessons from practice. J Knowl Manage. 2005;9(6):17–29.
Fishchuk I, Evsyukova O, Smalskys V. The information and communication support of public authorities in Ukraine in the context of using knowledge management in human resources. Viešoji Politika Ir Administravimas. 2021;20(2):260–70.
Google Scholar
Peña I. Knowledge networks as part of an integrated knowledge management approach. J Knowl Manage. 2002;6(5):469–78.
Tetroe JM, Graham ID, Foy R, Robinson N, Eccles MP, Wensing M, Durieux P, Légaré F, Nielson CP, Adily A, Ward JE. Health research funding agencies’ support and promotion of knowledge translation: an international study. Milbank Q. 2008;86(1):125–55.
Article PubMed PubMed Central Google Scholar
Armstrong K, Kendall E. Translating knowledge into practice and policy: the role of knowledge networks in primary health care. Health Inform Manage J. 2010;39(2):9–17.
Prowse M. Aid effectiveness: the role of qualitative research in impact evaluation. Background note. London: ODI; 2007.
Kalucy EC, Pearce CM, Beacham B, Lowcay BL, Yates RE. What supports effective research links between divisions of General Practice and universities? Med J Aust. 2006;185(2):114–6.
Kilpatrick S, Barrett M, Jones T. Defining Learning Communities.2003. www.crlra.utas.edu.au/files
Chan B, Reeve E, Matthews S, Carroll PR, Long JC, Held F, et al. Medicine information exchange networks among healthcare professionals and prescribing in geriatric medicine wards. Br J Clin Pharmacol. 2017;83(6):1185–96.
McNally M, Rock L, Gillis M, Bryan S, Boyd C, Kraglund F, Cleghorn B. Reopening oral health services during the COVID-19 pandemic through a knowledge exchange coalition. JDR Clin Trans Res. 2021.
McKeown RE. The epidemiologic transition: changing patterns of mortality and population dynamics. Am J Lifestyle Med. 2009;3(1suppl):S19–26.
Parhizgari N, Gouya MM, Mostafavi E. Emerging and re-emerging infectious diseases in Iran. Iran J Microbiol. 2017;9:122–42.
PubMed PubMed Central Google Scholar
Magee MJ, Narayan KM. Global confluence of infectious and non-communicable diseases-the case of type 2 diabetes. Prev Med. 2013;57:149–51.
Article PubMed CAS Google Scholar
Velasco E, Agheneza T, Denecke K, Kirchner G, Eckmanns T. Social media and internet-based data in global systems for public health surveillance: a systematic review. Milbank Q. 2014;92(1):7–33.
Ezenwaka U, Mbachu C, Etiaba E, Uzochukwu B, Onwujekwe O. Integrating evidence from research into decision-making for controlling endemic tropical diseases in South East Nigeria: perceptions of producers and users of evidence on barriers and solutions. Health Res Policy Syst. 2020;18(1):1–0.
Ellwanger JH, Veiga AB, Kaminski VD, Valverde-Villegas JM, Freitas AW, Chies JA. Control and prevention of infectious diseases from a one health perspective. Genet Mol Biology. 2021;44.
McKellar KA, Pitzul KB, Juliana YY, Cole DC. Evaluating communities of practice and knowledge networks: a systematic scoping review of evaluation frameworks. EcoHealth. 2014;11(3):383–99.
Yamey G, Schäferhoff M, Aars OK, Bloom B, Carroll D, Chawla M, et al. Financing of international collective action for epidemic and pandemic preparedness. Lancet Global Health. 2017;5(8):e742–4.
Korstjens I, Moser A, Series. Practical guidance to qualitative research. Part 4: trustworthiness and publishing. Eur J Gen Pract. 2018;24(1):120–4.
Fazey I, Fazey JA, Fazey DM. Learning more effectively from experience. Ecol Soc. 2005;10(2).
Ozor N, Kwanya T, Ozor GN. Learning what works: knowledge exchange and networking among the science system actors in sub-saharan Africa. United Kingdom: RECIRCULATE Programme of The Lancaster University; 2020.
Yazdi-Feyzabadi V, Amini-Rarani M. Evidence informed policymaking in infectious disease epidemics: implications for coronavirus disease 2019 (COVID-19). Health Inform Manage. 2020;17(1):39–42.
Osborne J. Improving your data transformations: applying the Box-Cox transformation. Practical Assess Res Evaluation. 2010;15(1):12.
Akhavan Behbahani A, Masoudi Asl I, Hesam S, Najafikhah M. Challenges for public participation in health legislation in Iran: a qualitative study. Archives Rehabilitation. 2020;21(4):454–69.
Zare R, Bahmani Choob Bastani A, Fathizadeh A. Conceptualize and identify dimensions and Indicators of Organizational Democracy in Public Sector Organizations. Public Organ Manage. 2016;4(3):29–48.
Choo CW. Information culture and organizational effectiveness. Int J Inf Manag. 2013;33(5):775–9.
Fullwood R, Rowley J, Delbridge R. Knowledge sharing amongst academics in UK universities. J Knowl Manage. 2013;17(1):123–36.
Zhen L, Jiang Z, Song HT. Distributed knowledge sharing for collaborative product development. Int J Prod Res. 2011;15(10):2959–76.
Rezaeian A, Bagheri R, Fartoukzadeh H. Identifying, prioritizing and modeling the factors of formation of knowledge networks in knowledge-based organizations with an interpretive structural approach. J Manage Improv. 2017;3(37):1–24.
Mayer RC, Davis JH, Schoorman FD. An integrative model of organizational trust. Acad Manage Rev. 1995;20(3):709–34.
Reychav I, Weisberg J. Bridging intention and behavior of knowledge sharing. J Knowl Manage. 2010 Apr 6.
Huang CC. Knowledge sharing and group cohesiveness on performance: an empirical study of technology R&D teams in Taiwan. Technovation. 2009;29(11):786–97.
Lueg C. Information, knowledge, and networked minds. J Knowl Manage. 2001;5(2):151–60.
Hernandez-Aguado I, Zaragoza GA. Support of public–private partnerships in health promotion and conflicts of interest. BMJ Open. 2016;6(4):e009342.
Fenton E, Harvey J, Sturt J. Evaluating primary care research networks. Health Serv Manage Res. 2007;20(3):162–73.
Seaman M. Birds of a feather? Communities of practice and knowledge communities. Curriculum Teach Dialogue. 2008;10(1/2):269.
Cyril Eze U, Guan Gan Goh G, Yih Goh C, Ling Tan T. Perspectives of SMEs on knowledge sharing. Vine. 2013;43(2):210–36.
Goh SC. Managing effective knowledge transfer: an integrative framework and some practice implications. JKM. 2002;6(1):23–30.
Bornbaum CC, Kornas K, Peirson L, Rosella LC. Exploring the function and effectiveness of knowledge brokers as facilitators of knowledge translation in health-related settings: a systematic review and thematic analysis. Implement Sci. 2015;10:162.
Ward VL, House AO, Hamer S. Knowledge brokering: exploring the process of transferring knowledge into action. BMC Health Serv Res. 2009;9:1–6.
Conklin J, Lusk E, Harris M, Stolee P. Knowledge brokers in a knowledge network: the case of Seniors Health Research Transfer Network knowledge brokers. Implement Sci. 2013;8(1):1–0.
Gagnon M. Knowledge dissemination and exchange of knowledge. In Knowledge Translation in Health Care: Moving from Evidence to Practice. Edited by Strauss S, Tetroe J, Graham ID. Oxford: Wiley-Blackwell; 2009:235–245.
BALLI Aİ, ÜSTÜN F. The relationship between organizational trust levels and demographic characteristics of employees: a case study. Int J Social Humanit Sci. 2017;1(2):20–38.
Svard P. The impact of information culture on information/records management: a case study of a municipality in Belgium. Records Manage J. 2014;24(1):5–21.
Ardichvili A. Learning and knowledge sharing in virtual communities of practice: motivators, barriers, and enablers. Adv Developing Hum Resour. 2008;10(4):541–54.
Download references
Acknowledgements
We appreciate of Iran University of Medical Sciences for financial support with Grant NO: IUMS/SHMIS-1401-1-37-23099.
This work was supported by Partial financial from Iran University of Medical Sciences with Grant NO: IUMS/SHMIS-1401-1-37-23099.
Author information
Authors and affiliations.
Ph.D in Medical Library & Information Science, School of Health Management & Information Sciences, Student Research Committee, Iran University of Medical Sciences, Tehran, Iran
Mina Mahami-Oskouei
Health Management and Economics Research Center, Health Management Research Institute, Iran University of Medical Sciences, Tehran, Iran
Leila Nemati-Anaraki
Department of Medical Library and Information Science, School of Health Management and Medical Information Sciences , Iran University of Medical Sciences, Tehran, Iran
Sirous Panahi & Shadi Asadzandi
You can also search for this author in PubMed Google Scholar
Contributions
All authors contributed to the study’s conception and design. The interviews were collected by [Mina Mahami-Oskouei]; implementation, and analysis by [Leila Nemati Anaraki], [Sirous Panahi], and [Shadi Asadzandi]. The first draft of the manuscript was written by [Mina Mahami-Oskouei]. Writing – review and editing final of the manuscript was written by [Mina Mahami-Oskouei], [Sirous Panahi], [Leila Nemati Anaraki], and all authors commented on previous versions of the manuscript. All authors read and approved the final manuscript.
Corresponding author
Correspondence to Leila Nemati-Anaraki .
Ethics declarations
Ethics approval and consent to participate.
The study procedure was approved by the Medical Ethics Committee of Iran University of Medical Sciences [date: Mar 2022, ID: IR.IUMS.REC.1400.1082] As part of the doctoral dissertation “Developing a Conceptual Model for a Knowledge Network in the Infectious Disease Field in Iran”. All participants’ information was private and nameless; there was no personal information that could link the answers with any of the participants in the present study.
Consent for publication
Not applicable.
Competing interests
The authors declare no competing interests.
Additional information
Publisher’s note.
Springer Nature remains neutral with regard to jurisdictional claims in published maps and institutional affiliations.
Electronic supplementary material
Below is the link to the electronic supplementary material.
Supplementary Material 1
Rights and permissions.
Open Access This article is licensed under a Creative Commons Attribution-NonCommercial-NoDerivatives 4.0 International License, which permits any non-commercial use, sharing, distribution and reproduction in any medium or format, as long as you give appropriate credit to the original author(s) and the source, provide a link to the Creative Commons licence, and indicate if you modified the licensed material. You do not have permission under this licence to share adapted material derived from this article or parts of it. The images or other third party material in this article are included in the article’s Creative Commons licence, unless indicated otherwise in a credit line to the material. If material is not included in the article’s Creative Commons licence and your intended use is not permitted by statutory regulation or exceeds the permitted use, you will need to obtain permission directly from the copyright holder. To view a copy of this licence, visit http://creativecommons.org/licenses/by-nc-nd/4.0/ .
Reprints and permissions
About this article
Cite this article.
Mahami-Oskouei, M., Nemati-Anaraki, L., Panahi, S. et al. Iranian experts’ perspectives on facilitators and barriers influencing the infectious disease knowledge network: A qualitative study. BMC Health Serv Res 24 , 1040 (2024). https://doi.org/10.1186/s12913-024-11525-8
Download citation
Received : 23 March 2024
Accepted : 02 September 2024
Published : 07 September 2024
DOI : https://doi.org/10.1186/s12913-024-11525-8
Share this article
Anyone you share the following link with will be able to read this content:
Sorry, a shareable link is not currently available for this article.
Provided by the Springer Nature SharedIt content-sharing initiative
- Knowledge Network
- Knowledge management
- Knowledge exchange
- Knowledge sharing
- Infectious diseases
BMC Health Services Research
ISSN: 1472-6963
- General enquiries: [email protected]
- Open access
- Published: 05 September 2024
Strengthening the effectiveness of community health system: Assessing the factors that enhance or constrain the delivery of health services within communities in Nigeria
- Okechukwu Ozor 1 ,
- Enyi Etiaba 1 &
- Obinna Onwujekwe 1
Health Research Policy and Systems volume 22 , Article number: 124 ( 2024 ) Cite this article
Metrics details
Introduction
Sub-optimal community health service delivery (CHSD) has been a challenge constraining community health systems (CHS) globally, especially in developing countries such as Nigeria. This paper examined the key factors that either enhance or constrain CHSD in Nigeria at the individual, community/facility and governmental levels while recommending evidence-based solutions for sustaining and improving CHSD within the framework of CHS.
Data were collected through a qualitative study undertaken in three states (Anambra, Akwa-Ibom and Kano) in Nigeria. Respondents were formal/informal health providers, community leaders and representatives of civil society organizations all purposively sampled. There were 90 in-depth interviews and 12 focus group discussions, which were audio-recorded, transcribed verbatim and analysed thematically using codes to identify key themes.
Factors constraining community health service delivery at the individual level were poor health-seeking behaviour, preference for quacks and male dominance of service delivery; at the community/facility level were superstitious/cultural beliefs and poor attitude of facility workers; at the governmental level were inadequate financial support, embezzlement of funds and inadequate social amenities. Conversely, the enabling factors at the individual level were community members’ participation and the compassionate attitude of informal providers. At the community and facility levels, the factors that enhanced service delivery were synergy between formal and informal providers and support from community-based organizations and structures. At the governmental level, the enhancing factors were the government’s support of community-based formal/informal providers and a clear line of communication.
Conclusions
Community health service delivery through a functional community-health system can improve overall health systems strengthening and lead to improved community health. Policy-makers should integrate community health service delivery in all program implementation and ultimately work with the community health system as a veritable platform for effective community health service delivery.
Peer Review reports
Poor community healthcare service delivery (CHSD) has adverse effects on both the individual and the economy of the state [ 1 , 2 ], while improved community healthcare service delivery has been shown to relate to more productivity in people [ 3 ]. Therefore, ensuring an efficient community health service delivery has implications for not only improving the health of the citizens but also the economy of the country. Achieving universal health coverage (UHC) and the Sustainable Development Goals (SDGs), for any country relies on the effectiveness of the country’s health service delivery to its communities; this effectiveness is dependent on how well factors that constrain and enhance health service delivery are identified, addressed and sustained [ 4 , 5 ]. The promotion of effective community health service delivery that would enhance the community health system in Nigeria has been a key policy objective of the Nigerian government, as contained in the Nigerian 2016 National Health Policy document [ 6 ]. This is because ensuring an efficient community health system has been a major global issue for countries across the globe [ 7 , 8 ]. According to the Nigerian 2016 National Health Policy document, the improvement of health service delivery at the community level has been identified as one of the key strategies for improving community health systems (CHS). Although there is no consensus for defining the concept of community health system because it is still evolving, Schneider and Lehmann in 2016 defined it as the set of local actors, relationships and processes engaged in producing, advocating for and supporting health in communities and households outside of, but existing in relationship to, formal health structures [ 9 ]. Secondly, Nigeria has not yet formally defined a community health system outside of the primary healthcare (PHC) system but, on occasions, has used these terms interchangeably [ 30 ]. However, some authors have also framed the CHS as the space between the “local health system” and the community, filled by the community health workers, who form a critical component of the CHS, acting as service extenders, cultural brokers and social change agents, extending health services to households [ 36 , 37 , 38 , 39 ]. It has also been conceptualized as the “grey zone” between the public health system, non-governmental organizations (NGO) and the private health system [ 40 , 41 ].
In this study, the authors a conceptualize community health system to refer to the actors (and their actions and relationships) in the formal and informal sectors found within the Nigerian local communities that influence healthcare service provision, utilization and advocacy. This includes, but is not limited to, actors such as the ward development committee (WDC), community security personnel, bonesetters, herbalists, traditional birth attendants (TBA), patent medicine vendors (PMVs) and formal actors such as the PHC centres and other facilities that provide formal healthcare services in the communities, such as the health posts. The concept within the Nigerian context also captures the intricate relationships and processes that may exist between these actors that influence the quality of healthcare service provision for the communities they serve.
Nigeria established the PHC system in 1976, which designated the local government areas (LGAs), the second smallest administrative level in the country, the responsibility of managing and implementing health services at the community level [ 30 ]. Subsequently, the National Primary Health Care Development Agency (NPHCDA) was established in 1992 to ensure the sustainability of previous gains and better manage the PHC system in the country [ 33 ], with the agency revising the PHC system in 1993 [ 10 ]. Despite these efforts, it is regrettable to see Nigeria rank 157th out of 167 countries in a 2023 world health and health system ranking [ 11 ]. The CHS operates just below the PHC system but is intrinsically linked to it, and its optimal functionality is expected to help in improving the country’s ranking amongst global health systems.
Studies have shown that the CHS in Nigeria has been deteriorating and needs to be enhanced as a way of improving the overall health system as well as boosting the country’s health profile [ 12 , 13 ]. This deterioration was attributed to a lack of adequate community health workers (CHWs) and a lack of re-training for the few available [ 13 ], poor female representation, poor collaboration with pre-existing community structures and hence poor community participation [ 12 ]. However, to improve community health system delivery in Nigeria within the construct of the CHS, it is important to assess the factors that constrain as well as promote healthcare service delivery at different levels across communities to mitigate the constraining factors while ensuring the sustenance of the promoting factors. Identifying and addressing the key factors that impede community health service delivery in Nigeria have strong implications for the improvement of CHS in the country as well as the attainment of the UHC and SDGs. This paper examined the key factors that either enhance or constrain CHSD in Nigeria at the individual, community/facility and governmental levels while recommending evidence-based solutions for sustaining and improving CHSD within the concept of CHS. It is a portion of a larger study on improving health at the community level in Nigeria, towards UHC. The findings will be useful for decision-makers in relation to possible solutions to tap into the latent resources of communities, especially within the context of CHS to significantly improve community health.
Study area and design
This study was conducted in three states across three geopolitical zones in Nigeria, namely Akwa-Ibom (south-southern zone), Anambra (south-eastern zone) and Kano (located in the north-western region). The areas were purposively chosen to achieve a geographical representation of the country and ensure more spread of the result findings. Data were collected through face-to-face semi-structured interviews and focus group discussion (FGD) with open-ended questions and prompts from key informants. Purposive sampling was used to select two local governments areas (LGAs) in each of the three states. The sampled LGAs included one rural and one urban area. For Akwa-Ibom State, the urban LGA was Uyo, while the rural LGA was Itu. For Anambra State, the urban LGA was Awka South LGA, while the rural LGA was Aguata. For Kano State, the urban LGA was Nasarawa Local Government Area, while the rural LGA was Kumbotso. The purposive sampling technique was used to select respondents for the study. The factors considered in their identification and selection were their level of participation and involvement in health-related activities in the communities, their occupation, leadership roles and length of service to the community or the government. The respondents cut across different groups – policy-makers; civil society organizations (CSOs); non-governmental organizations (NGOs); traditional, religious and community leaders; community groups; formal health providers; and informal health providers. A total of 90 in-depth interviews (IDIs) and 12 focus group discussions (FGDs) were conducted (Table 1 ). Data were collected between October and November 2022.
Data analysis
The analysis followed a systematic approach, including familiarization with the data, coding, identification of themes and interpretation. Regular meetings were held to discuss the coding and resolve any discrepancies among the researchers. Analyses focused on exploring the factors that both encourage as well as hinder community health service delivery in three sub-themes or levels – individual, community/facility and governmental. The analysis process involved double-coding each of the transcripts to ensure the relevance and comprehensiveness of the findings.
Ethical considerations
Ethical approval for this study was obtained from the Health Research Ethics Committee of the University of Nigeria Teaching Hospital, Ituku-Ozalla Enugu State. Permission to conduct the study was sought and obtained from the respondents and traditional leaders of the communities. Written informed consents were obtained from all study participants after they had demonstrated an understanding of study procedures and voluntariness. The confidentiality of all the participants was assured and maintained during and after the study by assigning unique identifiers to each participant during data analysis and reporting.
The result of the analysis is presented in two main themes which are factors that (1) enable or (2) constrain community health service delivery. Each of the main themes is further broken down into three sub-themes or levels: individual, community/facility and governmental.
Factors that promote CHSD
The following factors were found in the study to enhance community health service delivery at different levels:
Individual level
Selfless participation of community members
We found that one of the factors encouraging health service delivery in the communities is community members’ selfless participation in activities that improve CHSD. The members of the community were found to engage in personal activities such as carrying out sanitary activities in the primary healthcare centres as well as providing health equipment such as handwashes, gloves, etc., in a bid to improve the quality of health service provision in the communities. For example, a respondent in Kano State during the interview noted:
We carry out many services in the society like cleaning gutters and sweeping our surroundings, washing and disinfecting hospital, etc. All of this are done voluntarily by the people without expecting any payment at the end.” [WDC member, Kano].
Another respondent in Akwa Ibom noted:
In fact, it is the effort of ourselves that make things work here. The government is less concerned.” [civil servant, Akwa Ibom)].
Synergy between formal and informal providers with community members
At the individual level, informal providers expressed that they were in constant interaction with the formal providers to ensure adequate health service delivery for the community members. This interaction involves informal providers individually referring cases they cannot handle to formal providers to ensure maximum treatment output. The informal providers acknowledged that some cases they encounter are sometimes beyond them and that the best practice is to refer these immediately to the formal providers and that this has helped in ensuring adequate health service delivery output. While trying to buttress this point, a herbalist in Akwa Ibom noted:
Yes, I do refer some injuries that are beyond my ability to a health center because I believe there are some injuries that I can cure and others that I cannot and must refer to health centers. [herbalist, Akwa Ibom].
Compassionate attitude of informal providers
Another factor found to aid health service delivery in the communities at the individual level was the informal provider’s compassion and willingness to help the patients at little or no cost. These providers noted that their priority is ensuring that the patients recover from their illness rather than making monetary gains. This ensured that the patients kept coming to receive services at a reduced rate. A bonesetter in Akwa Ibom in a bid to elucidate this noted:
I’m not sure, but according to information I received from one of the clients referred to me, he should pay the hospital 650,000 naira. I was also considerate with him, and I didn’t take much from him because he was poor. I only charged him 130,000 naira because I was being considerate... the majority of them stay because I have a large house and I don’t mind them staying until they finish their treatment, and while at my place, they feed themselves out of their pockets, while the majority of them come from their homes for their treatment and then return [bonesetter, Akwa Ibom, IDI].
Another respondent in Kano recounts that, although the providers make little money from the job compared with the amount of work they do, it is the passion they have for the job that keeps them going. The respondent noted:
Most of them have passion for it because the little they get from it is really helping them [informal health worker, Kano].
Community/facility level
Synergy between formal and informal providers
At this level, a key factor we identified to be aiding health service delivery in the communities was cooperation between the formal and informal providers. Informal providers were found to cooperate with formal providers to the extent of having a union in the community that serves to regulate their practices. In these meetings, it was found that they shared ideas about how to promote their services. The informal providers were also reported to help in preparing patients for the formal providers to administer treatments. One of the respondents during an interview in Kano while trying to elucidate this synergy among the providers said:
During disease outbreaks, our members help the health workers in managing patients, for instance, we help in moving patients to their respective wards, putting them on beds, and getting drips ready so that by the time the health worker comes to them all is set he/she is just going to start administering treatment... all the health workers in the hospital including the in-charge respect and give us their maximum cooperation [WDC member, Kano, IDI].
Another respondent in Kano noted:
As you can see we (TBAs) are in the antenatal station so we work with them [TBA, Kano, IDI].
An FGD respondent in Kano also added:
Yes, we have linkage and from the ward head up to the district head. We the VCM, normally hold a meeting every month with district heads, to discuss about the health-related problems and if there is need for further assistance the ward head will also report to district head. [volunteer community mobilizer, Kano].
Support from community-based organizations and structures
Support from community-based organizations was also found to aid CHSD at the community/facility level. The existing community structures were found to facilitate the provision of service delivery through directly/indirectly influencing community participation or the provision of health service delivery. These community structures were: faith-based organizations (FBOs), ward development committees (WDC), health facility committees (HFCs) and broader community leadership. These groups were found to be involved in various health activities and other non-health activities in the communities, ranging from capital projects such as the erection of new health centres, after which they handed them over to the government to manage. They organize community empowerment programs, sensitization and health education activities through regular meetings, convening at community ceremonies such as weddings and church/mosque. For example, in one of the interviews in Kano, one of the respondents noted:
For us to achieve our objectives in the communities, we engage the services of the President Generals of the communities; [CHWs], Traditional leaders, faith-based organizations, the Market Women Association, the youths, etc... we normally draw the attention of the community representatives such as the TR, PG, youth leaders, market women leaders, etc. of the need to gather the community members for awareness creation [civil servant, Anambra, IDI].
The ward development committee has really helped to breach the gap between the community and the health facility so whatever you need to do in terms of mobilization and sensitization, they are always there, they help when we’re planning for advocacy visits, anytime you call them they render their support [officer-in-charge1, Akwa Ibom, IDI].
Governmental level
Government/partner’s support to community-based formal and informal providers
At this level, the support from both government and non-governmental organizations to community-based formal and informal providers was seen to aid CHSD. For example, informal providers were trained, supported and incentivized by NGOs that provide formal services to identify and refer illness cases to a central facility for treatment in Kano. Also, the TBAs noted that they receive items such as gloves, cleaning agents, etc., from the local government health authorities as a way to support them in attending to cases within their capacities and incentivize them to make quick referrals to the formal providers. A respondent in Anambra noted:
My organization trained [CHWs] and community volunteers on how to handle compassion fatigue… Yes of course, we have to educate them on what to do in the community before they start, this will help to guide them against abuse” [medical doctor, NGO, Anambra, IDI].
Another respondent also said:
We organize training, we train the CORPs, we train the CVs – the community volunteers and the community resource persons [civil servant, Anambra, IDI].
Speaking during an FGD in Kano, a TBA reflects:
...we’re really enjoying the training because the training is encouraging us, and like before most of the women, when they give birth at home they don’t care to go to the hospital but now as a result of our work they do come to the hospital, and all happens as a result of the training, and all these are among our work, and now even giving birth at home is very rare... [TBA, Kano, R6, FGD].
Clear line of communication
We also found that another factor that enhances CHSD at the governmental level is ensuring a clear line of communication among the health partners, the government and the community. This made it easy to ensure that interventions were directed to the right people. A respondent in Anambra during the IDI threw more light on this when the respondent said:
If any partner is coming to the state to support the state, for family planning programme, they will come through me. Then we go down to the LGAs. The LGA have the RA supervisor at the LGA level, and then we move down to the community, then we have facilities where we implement all these programmes” [civil servant, Anambra, IDI].
Factors that constrain CHSD
These factors were found to impede efficient community health service delivery at different levels.
Poor health-seeking behaviours/preference for quacks
The result of the study revealed that one of the factors impeding CHSD was the inability of the community members to seek health advice from experts; poor health-seeking behaviour, quack patronage, the tendency to receive services from people that are proximal to their homes and people they know rather than professionals; and the inability to go to the hospital on time to access care until the illness worsened. One of the respondents during the interview said:
That has been a challenge, honestly, we need to work on that actually. Even at that, people are still patronizing the quack…There is a PHC there, but they prefer to go to the quack and to the TBAs to deliver... [civil servant, Anambra, IDI].
In Akwa-Ibom, a healthcare provider noted that, after visiting a quack, the patients would then come to them when the illness got worse and the quack could no longer handle it. Below is an excerpt from the interview with the respondent.
They go to Chemist and treatment will fail, then they return to us. Some go to the Chemist with the name of the drugs and the chemist will hardly ask them questions [officer-in-charge1, Akwa Ibom].
Male dominance
Male dominance was found to be a problem, especially in Kano. This factor involved males refusing to comply with health workers/providers in the community. These males were also found to prevent their wives from receiving care such as going for antenatal and having their children immunized. A respondent in Kano elucidated this problem when she noted:
The main problem is noncompliance from the men in the community, in many cases, the women give their full support to us but their husbands prevent them from attending ante-natal or accepting immunizations for their babies [TBA, Kano].
Superstitious/cultural belief
The results also revealed that superstitious belief/cultural belief was a factor impeding CHSD at this level. The belief was found to revolve around local and religious perceptions about different aspects of health such as birthing and illnesses. These beliefs were observed to cut across societal spiritual stereotypes attached to health, political and fetish interferences and lack of community awareness. This belief was captured by one of the respondents during the interview in Akwa-Ibom when the respondent said:
You know our people believe prophesy, that woman can see well if the person will not be able to deliver, she used to pray, so many TBAs have prayer houses which our women can live there for 1 month before the delivery time [officer-in-charge1, Akwa Ibom]
While reacting to the issue of the cultural belief, a respondent in Anambra also noted:
Again, is what people say about ntutu (a native malicious charm characterized by the mysterious injection of tiny metal pin-like objects into the victim’s body) – all these things contribute to the disruption of healthcare. Because when someone has serious typhoid and malaria, or serious illness inside the person, the person will focus on going to remove the ntutu. By the time they will come to hospital, things have gotten worst” [public servant, Anambra].
Poor attitude of facility workers
Another factor we found to be constraining service delivery in the communities at this level is the poor attitude of the formal providers in the facility which is mostly reflected in absenteeism. This was elucidated by a community leader in Anambra when he noted:
When you get there, you will only see a nurse there and the doctor might be working in Awka. He won’t be coming all the time. When you get there the nurse will be giving you first aid treatment while waiting for the doctor. Will you say that they are not there? The nurse will press you water while you wait for the doctor and the doctor might end up not coming that day while the person keep waiting. So that is the challenge [community leader, Anambra].
During an FGD in Akwa Ibom, a respondent also noted:
This patronage to PMVs happen because there aren’t enough workers at the health centres and the health centers are closed early or not even open sometimes, especially during weekends. Most people use the PMVs and TBAs when they have no other option because there aren’t enough workers at the health center. This means that there should be enough staff at the health center and people will see reasons to visit the health center instead of using the PMVs [trader, Akwa Ibom].
Lack of adequate financial support from the government
The result revealed that one of the major factors constraining health service delivery was the lack of adequate financial support for healthcare providers to carry out their activities. This lack of funds was, for example, seen in poor remuneration for the field workers, the problem of owing health workers by the government, delay in payment of monthly salary, lack of funds for nutrition officers, lack of a Community-based Management of Acute Malnutrition (CMAM) centre in Akwa Ibom where acute malnutrition can be managed, etc. One of the respondents in Kano while trying to buttress the issue noted:
Yes, our biggest challenge is finance. We have the zeal to do many things but our constraint is finance. We are able to achieve some of our aims...the biggest challenge we face so far is lack of financial support from the government [WDC Member, Kano, IDI].
Another respondent in Anambra also noted:
Our major challenge has been lacking the funds to carry out some of the activities we want to do [chief security officer, Anambra].
The lack of financial support was also seen to affect the availability of cash to pay volunteers adequately, which also caused setbacks; this problem was captured by a respondent in Anambra:
The meagre amount paid to the volunteers is a serious setback… [volunteer health worker, Anambra,
Embezzlement of funds
Another major problem we found in the study constraining CHSD at the governmental level was the embezzlement of funds budgeted for healthcare in the communities. This corrupt practice cuts across the whole health system down to government officials and elites in society. To elucidate this problem, a formal provider in Anambra noted that:
There was one man that came here, that time was like they are doing sickle cell program for the State. It is government-funded or foreign government-funded. During the Mr. X’s governorship regime, they brought the money, but a sitting government official took it [medical doctor, NGO, Anambra].
Lack of social amenities
From the result, we found that another governmental-level factor limiting the progress of community health service delivery is the lack of social amenities in the communities such as water, electricity, security, adequate living quarters for healthcare workers, etc. The lack of electricity was emphasized, as it prevents pregnant women from accessing the healthcare centre at night. An example of how this constrains service delivery was captured by one of the respondents during the interview in Kano:
These sectors can because if there is no water in the hospital, there is a big problem, you see, water is very important because whatever that you are going to take, you have to use water, in conducting delivery, in the lab, because you have to wash some items before use or after use, in the hospital environment even the toilet, the staff toilet, outpatient toilet, everything, you have to use water. Water is very essential for the community health [matron, Kano, IDI].
While reacting to the issue of security, a respondent in Akwa Ibom noted:
But the fear is when you do all these things without security, something can happen cos when I came in here. I had a little experience, thieves came and removed all the fans that were here, about 8 fans, and picked all the drugs [officer-in-charge2, Akwa Ibom].
The lack of accommodation or living quarters inside the healthcare facilities for healthcare workers was also buttressed by another respondent in Akwa Ibom:
...but we are still praying that one faithful day, those ones they have not been giving us, they will give, because here now, we are supposed to have a quarters... one thing is the quarters here, the space is very big, they supposed to build quarters here and they are complaining, they supposed to build quarters, the community gave the government this land to build of which they have not [officer-in-charge1, Akwa Ibom, IDI].
Poor data record/data management
The result also revealed that the problem with health data collection and management was another factor constraining CHSD by affecting the utilization of health data for planning and decision-making. This problem was found to accrue from limited human resources and lack of training of data officers by the government. In a bid to elucidate this problem, a respondent said:
We have issue with data collection because we don’t have enough human resources for health. So, data collection has been a problem. You know, they are trying, but need training. At times, they lack the tools to collect data, but we are trying to address it. If we have enough human resources for health, we will deploy them to these facilities to help to generate every data and assign that role to them [civil servant, Anambra, IDI].
Result summary Table 2 presents the identified factors enhancing CHSD in the communities respectively at individual, community/facility and governmental levels. Table 2 presents a summary of identified factors constraining CHSD in the communities respectively at individual, community/facility and governmental levels.
The findings that, although CHSD within Nigerian communities is plagued with multi-level challenges, there are also multi-level factors playing roles in enhancing CHSD underscores the point that efforts are still being made to ensure an improved CHSD within the communities at different levels. These efforts were seen predominantly at the individual level, which highlights the power community members wield in improving healthcare in their communities rather than waiting on others, thus, highlighting the imperative role of community participation as noted in a recent study [ 23 ].
The synergy between formal and informal providers was found to be a key factor in enhancing CHSD both at individual and community/facility levels. This finding further supports the importance of ensuring a sustained healthy relationship between the informal and formal healthcare providers in ensuring improved healthcare service provision in communities as previously emphasized by other researchers [ 24 , 34 ]. Furthermore, at the community and facility level, support from community-based organizations (CBOs) was found as an enabler, as has been also identified in Nepal, although these were challenged by weak governance [ 31 ], and in China, where a community delivery model based on community structures was found to improved user satisfaction [ 32 ].
At the governmental level, NGOs partnering with the government to support formal and informal providers in the communities were also seen to help improve health service delivery in the communities. This finding further supports the findings of [ 35 ] that NGOs play a vital role in strengthening healthcare service delivery in low- and middle-income countries around the world.
The results are also consistent with the work of other researchers within Nigeria. For example, the current study supports the findings from the works of Amedari and Ejidike [ 18 ], Obansa and Orimisan [ 19 ], Okereke et al. [ 13 ] and Welcome [ 20 ], which identified factors constraining health service delivery in Nigerian communities as centring on corruption across the health system, poor management of human resources and lack of funding from the government. This raises the concern that these problems may have lingered because of negligence or enough not being done to curtail it by relevant stakeholders, hence pointing to the urgent need to create more awareness of the dangers of these constraining factors on CHSD and CHS as well as calling for more concerted effort to address these challenges. This also highlights the need for the possible adoption of a different approach to addressing these challenges. One such different approach could be the exploration of a joint regional effort from African leaders to effectively address these challenges since some of the factors found to impede CHSD within Nigeria such as lack of funding and basic amenities from the government are also those reported by the Nigerian National Population Commission [ 17 ] to affect healthcare service delivery in other African countries.
Although there are multi-level factors identified to be impeding CHSD in Nigeria by the present study, the majority of the factors were identified to stem from the governmental level. Interestingly, these challenges lead to others, indicating that successful eradication of a challenge can resolve other challenges. For example, the lack of adequate financial support, embezzlement of funds, lack of social amenities and poor data management were among the factors found to be impeding the progress of health services in the communities. By adequately addressing the issue of embezzlement of public funds, the issues of inadequate financial support, lack of amenities and poor data management can invariably be resolved to a reasonable extent; this is because the reason for the lack of social amenities, data management and other financial support could be because the funds provided for such are embezzled or mismanaged. Therefore, by setting up functional accountability structures involving the community members where every fund coming into the communities for health be it as a donation or government funding is accounted for, there is a possibility that this will also increase community participation in health as well as enable the communities to serve as “accountability police” for health projects, thus ensuring that funds are efficiently utilized in the execution and delivery of community health services.
The poor attitude of healthcare facility workers in Nigeria especially in the form of absenteeism has been reported by studies to be a cog in the wheel of development for CHSD in Nigeria [ 25 , 26 ]; our study finding was able to validate this by indicating that this facility-level constraint is still a key factor impeding the progress of CHSD in Nigerian communities. According to these studies [ 25 , 26 ], absenteeism is fueled by the government’s inability to provide adequate human resources in health facilities. This highlights the interrelatedness of the constraining factors across different levels in the current study. Furthermore, the unavailability of healthcare workers in the facility (a facility-level factor) may in turn explain why community members prefer to seek care among quacks (an individual-level factor) since these quacks are more proximal, are easy to access and affordable and easily share the same cultural preferences and beliefs with the community members [ 27 , 28 , 29 ].
Our study also revealed that cultural/superstitious beliefs negatively impact community health service delivery and consequently the community health systems in Nigeria. The influence of sociocultural factors on healthcare service delivery in Nigeria has been long documented by Odebiyi [ 30 ]. These cultural/superstitious beliefs (a community-level factor) may also explain the refusal of males to let their wives go for antenatal and immunization services, which the current study identified as a constraining factor at the individual level.
The interrelated nature of these constraining factors across the levels and the over-dependence on the government to tackle these challenges alone may explain why these factors have lingered. Therefore, involving more hands was seen as a key solution. The introduction of a multi-sectoral action where different health and non-health sectors can collectively work towards addressing these challenges at various levels may be more effective than expecting the government to do it alone. Since these challenges occur at different levels, different sectors that function at each level can focus on addressing challenges within their operational level first, before tackling them at a broader level. Evidence [ 21 , 22 ] has also shown that multi-sectorial collaboration is an effective approach to addressing different health challenges.
Limitation of study
Our study was not able to cover more states in the geopolitical zones already covered as well as new geopolitical zones in Nigeria such as Southwest due to limited resources.
Conclusion and recommendations
Achieving an enhanced CHS is possible. However, this is largely dependent on how well actors in both health and non-health sectors prioritize and adequately address the multi-level challenges constraining CHSD while sustaining the factors that promote it. CHSD through functional CHS can help improve strengthening of overall health systems and lead to improved health at the community level while ensuring the achievement of universal health coverage. Decision-makers should integrate CHSD in all program implementation that involves communities and ultimately work with CHS as a veritable platform for effective health service delivery.
Since the findings show that efforts are being made to improve community healthcare service delivery, the underlying argument therefore could be that these efforts are not substantial enough to produce the significant output needed for an optimal CHSD within the communities. This draws attention to the need for a joint approach that ensures the sustenance of the enabling factors while simultaneously addressing the identified challenges to boost the effort.
Also, it is possible that identifying and rewarding exceptional community actors that contribute to improving health service delivery in the communities by the leaders of the community and other stakeholders can encourage the sustenance of the identified enabling factors, especially at the individual level since studies have shown that reinforcements can help sustain positive attitudes [ 14 , 15 , 16 ].
Availability of data and materials
The dataset used for this study is available and can be obtained from the corresponding author upon request. No datasets were generated or analysed during the current study.
Abbreviations
Community health system
- Community health service delivery
Traditional birth attendants
In-depth interview
Officer-in-charge
Community-based management of acute malnutrition
Focus group discussion
Patent medicine vendors
- Universal health coverage
Sustainable Development Goals
Primary healthcare
Civil society organizations
Non-governmental organizations
Local government area
Community volunteers
Faith-based organizations
Ward development committee
Health facility committees
Informal health worker
Volunteer community mobilizer
De Nardi M, Pashchenko S, Porapakkarm P. The lifetime costs of bad health. Cambridge: Technical report, National Bureau of Economic Research; 2017.
Book Google Scholar
Dobkin C, Finkelstein A, Kluender R, Notowidigdo MJ. The economic consequences of hospital admissions. Am Econ Rev. 2018;108(2):308–52.
Article PubMed Google Scholar
Mitchell RJ, Ozminkowski RJ, Serxner S. Improving employee productivity through improved health. J Occup Environ Med. 2013;55(10):1142–8. https://doi.org/10.1097/JOM.0b013e3182a50037 .
Effiong FB, Ogbonna CP, Agughalam PI, Okwukwu MO, Dike IC, Elebesunu EE, Uwishema O. The role of community-based approaches in achieving universal health coverage: addressing the Nigerian narrative. Ann Med Surg. 2023;85(5):1769–73. https://doi.org/10.1097/MS9.0000000000000443 .
Article Google Scholar
Federal Ministry of Health. National Health Policy 2016: promoting the health of nigerians to accelerate socio-economic development. Abuja: Federal Ministry of Health; 2016.
Google Scholar
Rawat A, Pun A, Ashish KC, Tamang IK, Karlström J, Hsu K, Rasanathan K. The contribution of community health systems to resilience: case study of the response to the 2015 earthquake in Nepal. J Glob Health. 2023;13:04048. https://doi.org/10.7189/jogh.13.04048 .
Article PubMed PubMed Central Google Scholar
Kruk ME, Gage AD, Arsenault C, Jordan K, Leslie HH, Roder-DeWan S, Adeyi O, Barker P, Daelmans B, Doubova SV, English M, García-Elorrio E, Guanais F, Gureje O, Hirschhorn LR, Jiang L, Kelley E, Lemango ET, Liljestrand J, Malata A, Pate M. High-quality health systems in the sustainable development goals era: time for a revolution. Lancet Glob Health. 2018;6(11):e1196–252. https://doi.org/10.1016/S2214-109X(18)30386-3 .
Schneider H, Lehmann U. From community health workers to community health systems: time to widen the horizon? Health Syst Reform. 2016;2(2):112–8.
Egan KF, Devlin K, Pandit-Rajani T. Community health systems catalog country profile: Nigeria. Arlington: Advancing Partners & Communities; 2017.
Vankar P. Ranking of Health and Health Systems of Countries Worldwide in 2023. Statista. 2023. https://www.statista.com/statistics/1376359/health-and-health-system-ranking-of-countries-worldwide/ . Accessed 10 Dec 2023.
Iyanda OF, Akinyemi OO. Our chairman is very efficient: community participation in the delivery of primary health care in Ibadan Southwest Nigeria. Pan African Med J. 2017;27:258. https://doi.org/10.11604/pamj.2017.27.258.12892 .
Okereke E, Ishaku SM, Unumeri G, et al. Reducing maternal and newborn mortality in Nigeria – a qualitative study of stakeholders’ perceptions about the performance of community health workers and the introduction of community midwifery at primary healthcare level. Human Resour Health. 2019;17:102. https://doi.org/10.1186/s12960-019-0430-0 .
Adam P, Greg R, Sasan S, Alan W. Small sample field study: the effects of team-based recognition on employee engagement and effort. Manag Account Res. 2023;59:1044–5005. https://doi.org/10.1016/j.mar.2022.100829 .
Alkandi IG, Khan MA, Fallatah M, Alabdulhadi A, Alanizan S, Alharbi J. The impact of incentive and reward systems on employee performance in the Saudi primary, secondary, and tertiary industrial sectors: a mediating influence of employee job satisfaction. Sustainability. 2023;15(4):3415. https://doi.org/10.3390/su15043415 .
Mohsin B, Bradley E, Wright E, Shahidul H. The interactive influence of public service motivation, perceived reward equity, and prosocial impact on employee engagement: a panel study in Pakistan. Pub Manag Rev. 2021. https://doi.org/10.1080/14719037.2021.2013069 .
NPC Nigeria. Nigeria demographic and health survey 2018. Abuja: National Population Commission and ICF Macro; 2018.
Amedari MI, Ejidike IC. Improving access, quality and efficiency in health care delivery in Nigeria: a perspective. PAMJ One Health. 2021;5:3. https://doi.org/10.11604/pamj-oh.2021.5.3.28204 .
Obansa SA, Orimisan A. Health care financing in Nigeria: prospects and challenges. Mediterr J Soc Sci. 2013;4(1):221–36. https://doi.org/10.5901/mjss.2013.v4n1p221 .
Welcome MO. The Nigerian health care system: Need for integrating adequate medical intelligence and surveillance systems. J Pharm Bioallied Sci. 2011;3(4):470–8. https://doi.org/10.4103/0975-7406.90100 .
Rasanathan K, Atkins V, Mwansambo C, Soucat A, Bennett S. Governing multisectoral action for health in low-income and middle-income countries: an agenda for the way forward. BMJ Glob Health. 2018;3(Suppl 4): e000890. https://doi.org/10.1136/bmjgh-2018-000890 .
Bennett S, Glandon D, Rasanathan K. Governing multisectoral action for health in low-income and middle-income countries: unpacking the problem and rising to the challenge. BMJ Glob Health. 2018;3(Supple 4): e000880. https://doi.org/10.1136/bmjgh-2018-000880 .
Gholipour K, Shokri A, Yarahmadi AA, Tabrizi JS, Iezadi S, Naghibi D, Bidarpoor F. Barriers to community participation in primary health care of district health: a qualitative study. BMC Prim care. 2023;24(1):117. https://doi.org/10.1186/s12875-023-02062-0 .
Sieverding M, Beyeler N. Integrating informal providers into a people-centered health systems approach: qualitative evidence from local health systems in rural Nigeria. BMC Health Serv Res. 2016;16:526. https://doi.org/10.1186/s12913-016-1780-0 .
Odii A, Onwujekwe O, Hutchinson E, et al. Absenteeism in primary health centres in Nigeria: leveraging power, politics and kinship. BMJ Glob Health. 2022;7: e010542.
Oche MO, Oladigbolu RA, Ango JT, Okafoagu NC, Ango UM. Work absenteeism amongst health care workers in a tertiary health Institution in Sokoto, Nigeria. J Adv Med Med Res. 2018;26(2):1–9. https://doi.org/10.9734/JAMMR/2018/40467 .
Bloom G, Standing H, Lucas H, Bhuiya A, Oladepo O, Peters DH. Making health markets work better for poor people: the case of informal providers. Health Policy Plan. 2011;26(suppl 1):i45-52.
Durham J, Michael M, Hill P, Paviignani E. Haïti and the health marketplace: the role of the private, informal market in filling the gaps left by the state. BMC Health Serv Res. 2015;15(1):424.
Article CAS PubMed PubMed Central Google Scholar
Rao PH. Profile and practice of private medical practitioner in rural India. Health Popul Perspect Issues. 2005;28:40–9.
Odebiyi AI. The socio-cultural factors affecting health care delivery in Nigeria. J Trop Med Hyg. 1977;80(11):249–54.
CAS PubMed Google Scholar
Egan KF, Devlin K, Pandit-Rajani T. Community health systems catalog country profile: Nigeria. 2017
Acharya KK, Zafarullah H. Community governance and service delivery in Nepal: an assessment of influencing factors. Commonw J Local Gov. 2019. https://doi.org/10.5130/cjlg.v0i21.6739 .
Liu Y, Yuan Z, Liu Y, et al. Changing community health service delivery in economically less-developed rural areas in China: impact on service use and satisfaction. BMJ Open. 2014;4: e004148. https://doi.org/10.1136/bmjopen-2013-004148 .
National Primary Healthcare Development Agency. Brief overview . 2024. https://nphcda.gov.ng/about/ . Accesssed 17 July 2024
Christian BN, Christian NG, Keshinro MI, Olutade-Babatunde O. How to build bridges for Universal Health Coverage in Nigeria by linking formal and informal health providers. BMJ Glob Health. 2023;8(11): e014165. https://doi.org/10.1136/bmjgh-2023-014165 .
Besançon S, Sidibé A, Sow DS, Sy O, Ambard J, Yudkin JS, Beran D. The role of non-governmental organizations in strengthening healthcare systems in low- and middle-income countries: lessons from Santé Diabète in Mali. Glob Health Action. 2022;15(1):2061239. https://doi.org/10.1080/16549716.2022.2061239 .
Kane S, Kok M, Ormel H, Otiso L, Sidat M, Namakhoma I, Nasir S, Gemechu D, Rashid S, Taegtmeyer M. Limits and opportunities to community health worker empowerment: a multi-country comparative study. Soc Sci Med. 2016;164:27–34.
Baatiema L, Sumah AM, Tang PN, Ganle JK. Community health workers in Ghana: the need for greater policy attention. BMJ Glob Health. 2016;1: e000141.
Schaaf M, Warthin C, Freedman L, Topp SM. The community health worker as service extender, cultural broker and social change agent: a critical interpretive synthesis of roles, intent and accountability. BMJ Glob Health. 2020;5: e002296.
Leban K, Kok M, Perry HB. Community health workers at the dawn of a new era: 9. CHWs’ relationships with the health system and communities. Health Res Policy Syst. 2021;19:1–19.
Zulu JM, Goicolea I, Kinsman J, Sandoy IF, Blystad A, Mulubwa C, Makasa MC, Michelo C, Musonda P, Hurtig AK. Community-based interventions for strengthening adolescent sexual reproductive health and rights: how can they be integrated and sustained? A realist evaluation protocol from Zambia. Reprod Health. 2018;15:1–8.
Zulu JM, Perry HB. Community health workers at the dawn of a new era. Berlin: Springer; 2021.
Download references
Acknowledgements
We wish to acknowledge the Bill & Melinda Gates Foundation as well as the African Health Observatory Platform (AHOP) for their support in helping to ensure the completion of this study.
The research project which led to the results included in this manuscript received funding from the Bill & Melinda Gates Foundation. However, the funding does not cover publications. The funders did not participate in designing the study, collecting and analysing data, or writing and reviewing the manuscript. The views expressed in this manuscript belong exclusively to the authors and do not necessarily represent the funders’ opinions.
Author information
Authors and affiliations.
Health Policy Research Group, University of Nigeria, Enugu, Nigeria
Okechukwu Ozor, Enyi Etiaba & Obinna Onwujekwe
You can also search for this author in PubMed Google Scholar
Contributions
Enyi Etiaba and Obinna Onwujekwe conceptualized and designed the study. Okechukwu Ozor and Enyi Etiaba participated in data collection. All authors took part in data analysis. Okechukwu Ozor wrote the first draft of the manuscript. All authors reviewed and approved the final version of the manuscript.
Corresponding author
Correspondence to Okechukwu Ozor .
Ethics declarations
Ethics approval and consent to participate.
This study received ethics approval from the Health Research Ethics Committee of the University of Nigeria Teaching Hospital Enugu with reference number NHRECAS/01/2008-WA00002458-IRB00002323. All methods were conducted according to relevant guidelines and regulations. All participants were informed of the purpose of the research, the rights of participants and measures to protect them and their data. Written, signed and verbal informed consent was also obtained from all the participants. Participation in the study was voluntary and confidentiality was ensured.
Consent for publication
Not Applicable.
Competing interest
There is no competing interest. We confirm that the manuscript has been read and approved by all named authors. We confirm that the order of authors listed in the manuscript has been approved by all named authors.
Additional information
Publisher’s note.
Springer Nature remains neutral with regard to jurisdictional claims in published maps and institutional affiliations.
Rights and permissions
Open Access This article is licensed under a Creative Commons Attribution-NonCommercial-NoDerivatives 4.0 International License, which permits any non-commercial use, sharing, distribution and reproduction in any medium or format, as long as you give appropriate credit to the original author(s) and the source, provide a link to the Creative Commons licence, and indicate if you modified the licensed material. You do not have permission under this licence to share adapted material derived from this article or parts of it. The images or other third party material in this article are included in the article’s Creative Commons licence, unless indicated otherwise in a credit line to the material. If material is not included in the article’s Creative Commons licence and your intended use is not permitted by statutory regulation or exceeds the permitted use, you will need to obtain permission directly from the copyright holder. To view a copy of this licence, visit http://creativecommons.org/licenses/by-nc-nd/4.0/ .
Reprints and permissions
About this article
Cite this article.
Ozor, O., Etiaba, E. & Onwujekwe, O. Strengthening the effectiveness of community health system: Assessing the factors that enhance or constrain the delivery of health services within communities in Nigeria. Health Res Policy Sys 22 , 124 (2024). https://doi.org/10.1186/s12961-024-01204-9
Download citation
Received : 22 May 2024
Accepted : 30 July 2024
Published : 05 September 2024
DOI : https://doi.org/10.1186/s12961-024-01204-9
Share this article
Anyone you share the following link with will be able to read this content:
Sorry, a shareable link is not currently available for this article.
Provided by the Springer Nature SharedIt content-sharing initiative
- Community health systems
- Multisectoral collaboration
Health Research Policy and Systems
ISSN: 1478-4505
- Submission enquiries: Access here and click Contact Us
- General enquiries: [email protected]

IMAGES
VIDEO
COMMENTS
Doing qualitative research is not easy and may require a complete rethink of how research is conducted, particularly for researchers who are more familiar with quantitative approaches. There are many ways of conducting qualitative research, and this paper has covered some of the practical issues regarding data collection, analysis, and management.
Qualitative data is a type of data that is collected and analyzed in a non-numerical form, such as words, images, or observations. It is generally used to gain an in-depth understanding of complex phenomena, such as human behavior, attitudes, and beliefs.
Qualitative research involves collecting and analyzing non-numerical data (e.g., text, video, or audio) to understand concepts, opinions, or experiences. It can be used to gather in-depth insights into a problem or generate new ideas for research. Qualitative research is the opposite of quantitative research, which involves collecting and ...
How to conduct qualitative research? Given that qualitative research is characterised by flexibility, openness and responsivity to context, the steps of data collection and analysis are not as separate and consecutive as they tend to be in quantitative research [13, 14].As Fossey puts it: "sampling, data collection, analysis and interpretation are related to each other in a cyclical ...
Planning Qualitative Research: Design and Decision Making ...
Qualitative research involves the studied use and collection of a variety of empirical materials - case study, personal experience, introspective, life story, interview, observational, historical, interactional, and visual texts - that describe routine and problematic moments and meanings in individuals' lives.
Qualitative Data Analysis: What is it, Methods + Examples
Qualitative data describes findings beyond clear-cut numbers from research across various methods and types. If you're wondering how qualitative data can help your business or innovative research efforts, keep reading. This is the ultimate guide to understanding what it is, how it works, and where to begin in collecting and analyzing data.
Qualitative Research. Qualitative research is a type of research methodology that focuses on exploring and understanding people's beliefs, attitudes, behaviors, and experiences through the collection and analysis of non-numerical data. It seeks to answer research questions through the examination of subjective data, such as interviews, focus groups, observations, and textual analysis.
Still other definitions focus on the process and context of data collection: Qualitative research is a situated activity that locates the observer in the world. It consists of a set of interpretive, material practices that makes the ... tive research that divides qualitative data into its three main forms—text, images, and sounds (Figure 1.1 ...
Qualitative Data Collection: What it is + Methods to do it
Methods of data collection in qualitative research
Qualitative Data, Analysis, and Design
The three core approaches to data collection in quali-tative research—interviews, focus groups and observa-tion—provide researchers with rich and deep insights. All methods require skill on the part of the researcher, and all produce a large amount of raw data. However, with careful and systematic analysis12 the data yielded with these ...
Data collection in qualitative research
Qualitative data is descriptive information that captures observable qualities and characteristics not quantifiable by numbers. It is collected from interviews, focus groups, observations, and documents offering insights into experiences, perceptions, and behaviors. Qualitative data analysis cannot be counted or measured because it describes ...
The SAGE Handbook of. tive Data AnalysisUwe FlickMapping the FieldData analys. s is the central step in qualitative research. Whatever the data are, it is their analysis that, in a de. isive way, forms the outcomes of the research. Sometimes, data collection is limited to recording and docu-menting naturally occurring ph.
of qualitative research, it distinguishes itself as a how-to guide to be used in the field. We have designed the guide as a tool for training the data collection staff members of multisite and team-based public health projects, but it easily has application for smaller-scale or multidisciplinary projects as well.
Learning to Do Qualitative Data Analysis: A Starting Point
2005 — This how-to guide covers the mechanics of data collection for applied qualitative research. It is appropriate for novice and experienced researchers alike. It can be used as both a training tool and a daily reference manual for field team members. The question and answer format and modular design make it easy for readers to […]
Collecting and Analyzing Qualitative Data
Methods of Data Collection in Quantitative, Qualitative, and ...
of data collection, it is important to decide the target population that is to be used for the investigation. It. includes those firms, individuals, groups or elements that will be represented in ...
The Grounded Theory Approach is a systematic methodology used in qualitative analysis methods that allows researchers to generate theories based on collected data. This technique considers data collection, coding, and analysis as interrelated processes, helping researchers identify patterns and develop insights that emerge from the data.
Data for the study were collected from students (N = 16) through semi-structured interviews and analyzed using a qualitative content analysis. Results indicated that the students perceived the use of the learning analytics dashboard as an opportunity for versatile learning support, providing them with a means to control and observe their ...
This qualitative study employed content analysis and used purposive and snowball sampling. The data were collected via online or face-to-face interviews with 25 participants with diverse expertise in infectious diseases (both clinical and non-clinical), epidemiology, knowledge management, and knowledge-based business management in Iran.
Data were collected through a qualitative study undertaken in three states (Anambra, Akwa-Ibom and Kano) in Nigeria. ... who form a critical component of the CHS, acting as service extenders, cultural brokers and social change agents ... Ethical approval for this study was obtained from the Health Research Ethics Committee of the University of ...